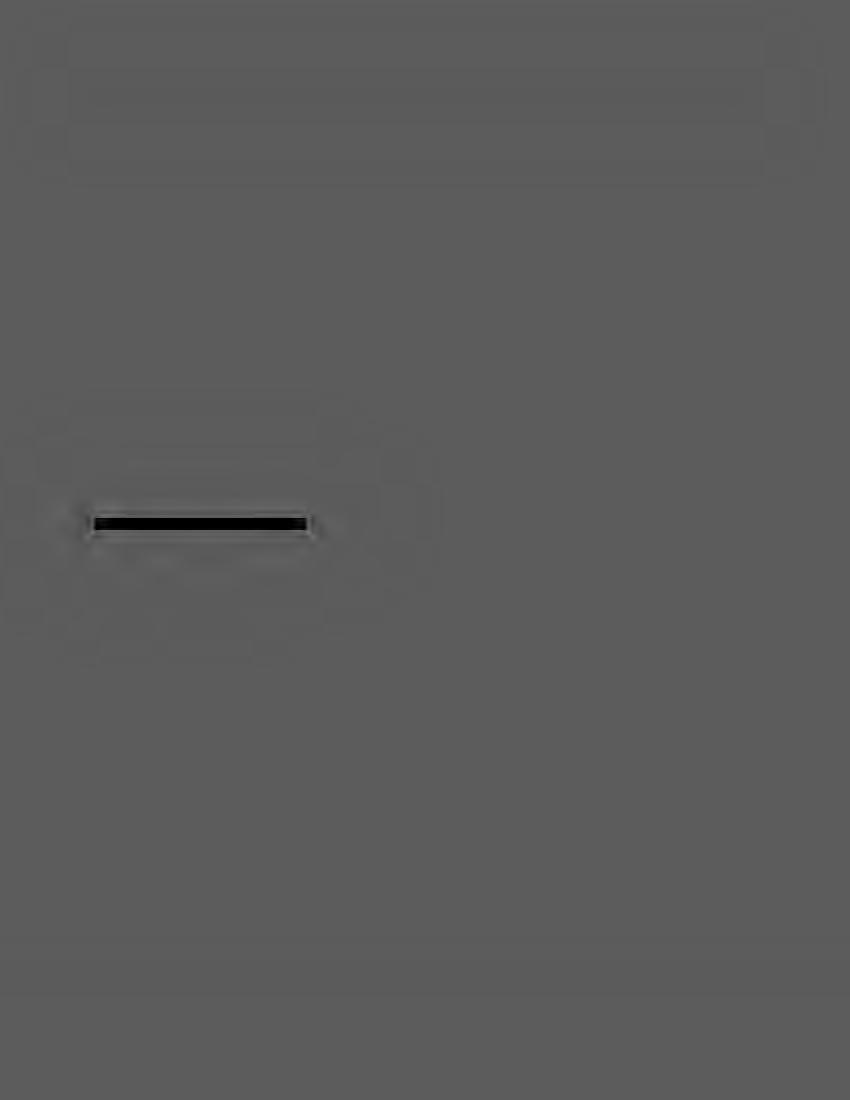
6 minute read
A. Deceptive and fraudulent content intended to scam or otherwise harm individuals
of particular content and whether it has been altered. These tools — which could involve blockchain, among other things — can be especially helpful in dealing with the provenance of audio and video materials. Like detection tools, however, authentication measures have limits and are not helpful for every online harm.
Finally, in the context of AI and online harms, any laws or regulations require careful consideration. Given the various limits of and concerns with AI, explicitly or effectively mandating its use to address harmful content — such as overly quick takedown requirements imposed on platforms — can be highly problematic. The suggestion or imposition of such mandates has been the subject of major controversy and litigation globally. Among other concerns, such mandates can lead to overblocking and put smaller platforms at a disadvantage. Further, in the United States, such mandates would likely run into First Amendment issues, at least to the extent that the requirements impact legally protected speech. Another hurdle for any regulation in this area is the need to develop accepted definitions and norms not just for what types of automated tools and systems are covered but for the harms such regulation is designed to address.
Putting aside laws or regulations that would require more fundamental changes to platform business models, the most valuable direction in this area — at least as an initial step — may be in the realm of transparency and accountability. Seeing and allowing for research behind platforms’ opaque screens (in a manner that takes user privacy into account) may be crucial for determining the best courses for further public and private action.24 It is hard to craft the right solutions when key aspects of the problems are obscured from view.
III. USING ARTIFICIAL INTELLIGENCE TO COMBAT ONLINE HARMS
A. Deceptive and fraudulent content intended to scam or otherwise harm individuals
Of the harms specified by Congress, deception is the most central to the Commission’s consumer protection mission. Public and private sector use of AI tools to combat online scams is still in its relative infancy, and such tools may be hard to develop. While some scams may be detected by relatively clear and objective markers, many are context-dependent and not obvious on their face. After all, the nature of a scam is to deceive people into thinking it’s not a scam. For example, the initial part of a scheme may involve a seemingly legitimate online ad, with key fraud indicators hidden offline and revealed only later. These factors may make it difficult for
24 Commission staff is currently analyzing data collected from several large social media and video streaming companies about their collection and use of personal information as well as their advertising and user engagement practices. See https://www.ftc.gov/reports/6b-orders-file-special-reports-social-media-video-streaming-serviceproviders. In a 2020 public statement about this project, Commissioners Rebecca Kelly Slaughter and Christine S. Wilson remarked that “[i]t is alarming that we still know so little about companies that know so much about us” and that “[t]oo much about the industry remains dangerously opaque.” https://www.ftc.gov/system/files/documents/public statements/1584150/joint statement of ftc commissioners cho pra slaughter and wilson regarding social media and video.pdf.
machines to predict the veracity of claims about a product or service. 25 Automated tools may thus be less likely, at least in the near term, to help address online fraud as opposed to other harms.26
Despite the challenges, the use of AI to combat fraud is certainly an area for further research. Perhaps AI-based tools could help law enforcement agencies, researchers, platforms, and others reveal patterns of fraud and hidden connections between bad actors. A few consumer protection agencies have indeed started to look into whether AI can help in specific areas of fraud. For example, Japan’s Consumer Affairs Agency sought funds to create an AI tool to identify websites selling products with deceptive COVID-19 prevention claims.27 Poland’s Office of Competition and Consumer Protection began a project to develop an AI tool that automatically detects unlawful clauses in business-to-consumer contracts.28 Multiple Austrian government agencies have funded the development of an AI tool that would help consumers detect whether a website is a fake online shop.29
Facebook states that it uses AI tools to address various types of fraud, though, as the FTC has reported, scams on Facebook and other social media sites have continued to rise.30 Specifically, Facebook says that it uses machine learning to identify scams and imposters on Messenger31 and generally that it demotes content associated with fraud, including: links to suspected cloaking domains (which might involve financial scams); pages predicted to be spam (which might involve false ads, fraud, and security risks); and exaggerated health claims.32 It also uses
25 At the same time, scammers can use their own automated tools to commit fraud or can use the automated systems of social media platforms to amplify and target false advertising. See, e.g., Jon Bateman, Get Ready for Deepfakes to Be Used in Financial Scams, Techdirt (Aug. 10, 2020), https://www.techdirt.com/2020/08/10/get-ready-deepfakesto-be-used-financial-scams/; https://www ftc.gov/business-guidance/blog/2021/11/ftc-analysis-shows-covid-fraudthriving-social-media-platforms; https://www.ftc.gov/news-events/data-visualizations/data-spotlight/2022/01/socialmedia-gold-mine-scammers-2021. 26 In contrast, AI is a common feature of anti-fraud measures in the credit card context, in which markers of fraud may be easier to detect. See PYMNTS and Brighterion, AI in Focus: The Rise against Payments Fraud (2021), https://www.pymnts.com/wp-content/uploads/2021/12/PYMNTS-AI-In-Focus-Waging-Digital-Warfare-AgainstPayments-Fraud-December-2021.pdf; https://usa.visa.com/visa-everywhere/security/outsmarting-fraudsters-withadvanced-analytics html; https://www.paypal.com/us/brc/article/enterprise-solutions-paypal-machine-learning-stopfraud. Payment firms also have the benefit of robust, accurate data about their customers and strong financial incentives to combat such fraud. 27 See https://www.caa.go.jp/policies/budget/assets/policies budget 201225 0002.pdf. 28 See https://ec.europa.eu/info/funding-tenders/opportunities/portal/screen/how-to-participate/orgdetails/949814883/project/899954/program/31061273/details. 29 See https://www.ait.ac.at/en/news-events/single-view/detail/6860?cHash=250795314de77d44fa029af1a1310da2 and Louise Beltzung, et al., Real-Time Detection of Fake-Shops through Machine Learning, 2020 IEEE International Conference on Big Data (Dec. 2020), https://doi.org/10.1109/BigData50022.2020.9378204. 30 See https://www ftc.gov/news-events/press-releases/2022/01/ftc-finds-huge-surge-consumer-reports-about-losingmoney-scams; https://www ftc.gov/news-events/blogs/business-blog/2021/11/ftc-analysis-shows-covid-fraud-
31 See https://messengernews fb.com/2020/05/21/preventing-unwanted-contacts-and-scams-in-messenger/. 32 See https://transparency.fb.com/en-gb/features/approach-to-ranking/types-of-content-we-demote/.
thriving-social-media; https://www ftc.gov/news-events/blogs/data-spotlight/2020/10/scams-starting-social-mediaproliferate-early-2020.
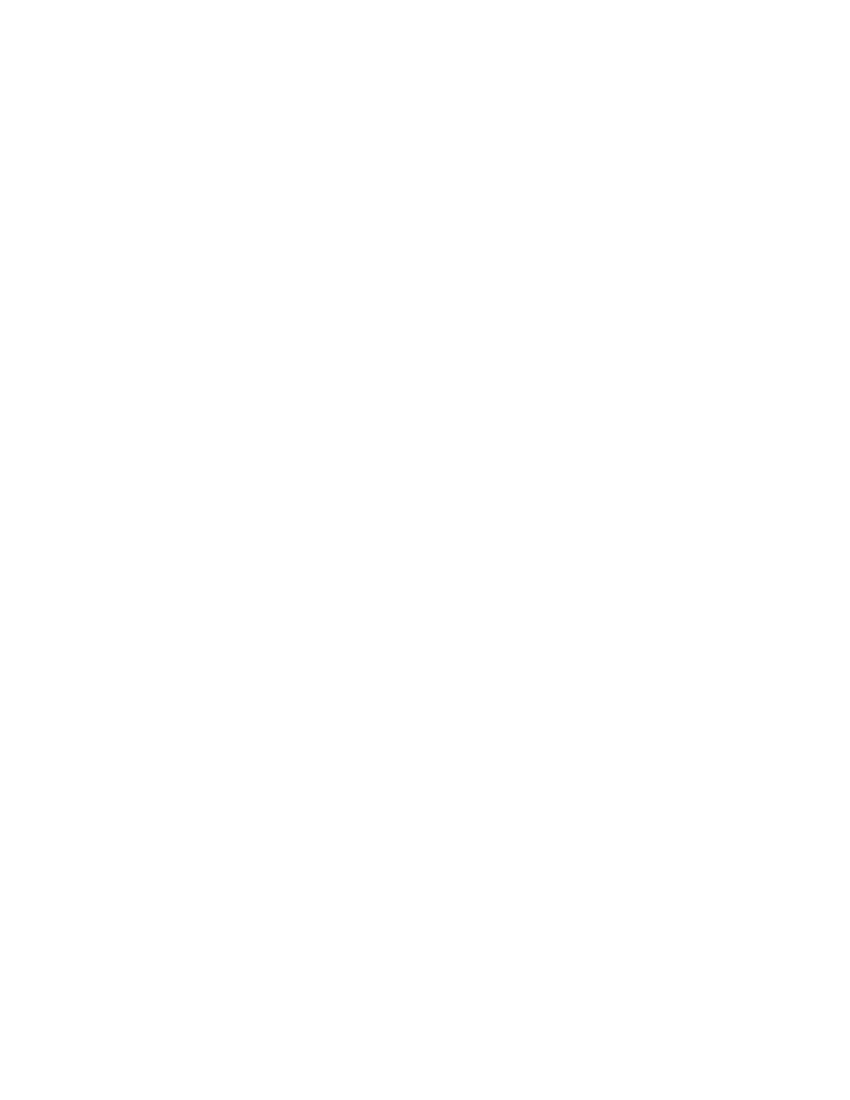
automated detection systems for scams in the Facebook Marketplace, though their efficacy is uncertain at best.
33
Other companies reporting similar usage of AI include Google, which uses it to detect online frauds and spam in search results and to filter spam, malware, and phishing in Gmail.34 Microsoft makes similar use of AI for phishing and spam in Outlook.35 Representatives of cybersecurity companies state that AI tools can also “track patterns in scam emails using large datasets and delete scam emails from people’s inboxes.”36 Third-party vendors may offer AI-based scam detection as well, such as tools to find tax scam websites.37
Some academic research has also focused on the use of AI to address this harm, including a publicly funded project in the United Kingdom to detect fake dating profiles,38 two connected studies on detecting undisclosed influencer affiliations, 39 and studies on detecting email spam.40
It is also worth noting that AI tools could aid in the investigation of whether companies are engaged in online conduct that harms competition. In 2021, for example, investigative journalists
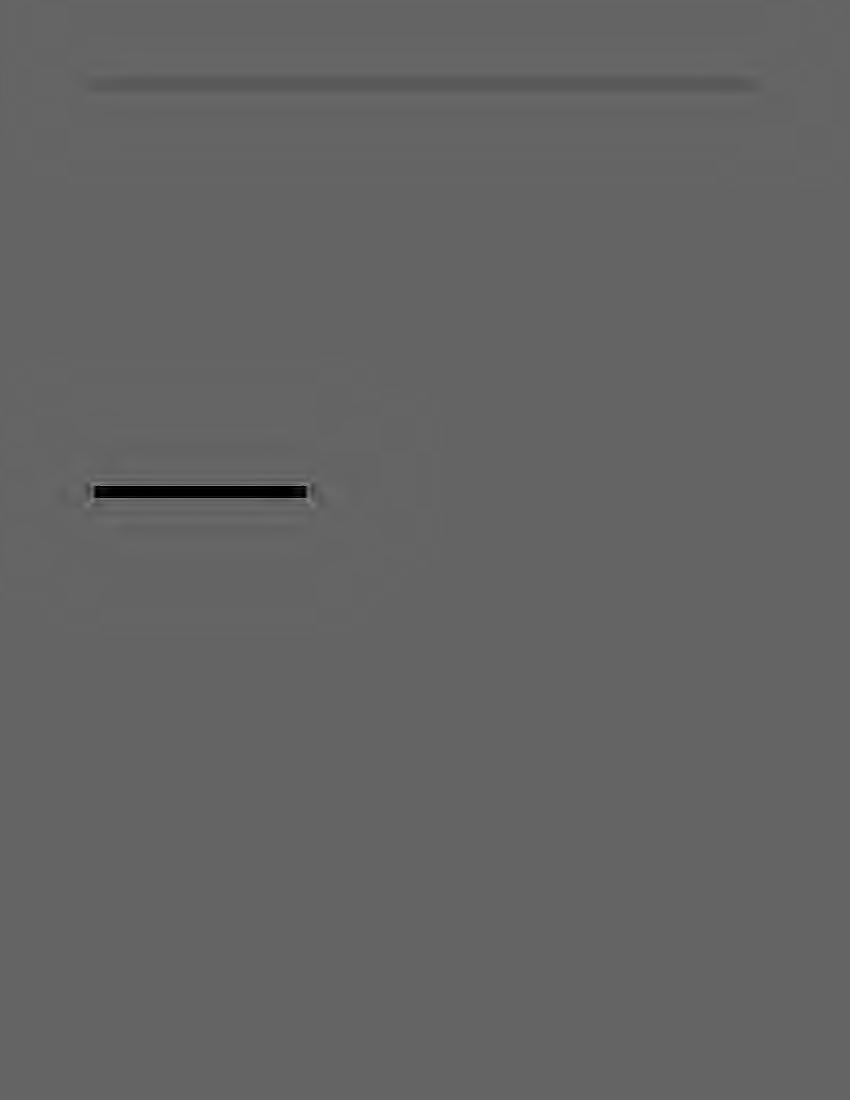
33 See Craig Silverman, et al., Facebook Grew Marketplace to 1 Billion Users. Now Scammers Are Using It to Target People Around the World, ProPublica (Sep. 22, 2021), https://www.propublica.org/article/facebook-grewmarketplace-to-1-billion-users-now-scammers-are-using-it-to-target-people-around-the-world. See also Colin Lecher and Surya Mattu, Facebook Scammers Are Schilling Fake Cryptocurrency Using Big Tech’s Biggest Names, The Markup (Feb. 22, 2022), https://themarkup.org/citizen-browser/2022/02/22/facebook-scammers-are-schillingfake-cryptocurrency-using-big-techs-biggest-names. 34 See https://developers.google.com/search/blog/2021/04/how-we-fought-search-spam-2020 and https://security.googleblog.com/2020/02/improving-malicious-document-detection html?m=1. 35 See https://www microsoft.com/en-us/insidetrack/office-365-helps-secure-microsoft-from-modern-phishingcampaigns; https://docs.microsoft.com/en-us/microsoft-365/security/office-365-security/anti-spamprotection?view=o365-worldwide. AlgorithmWatch has documented how some email spam filters using machine learning, including Microsoft’s, may seem uncontroversial but could in fact be discriminatory and remain largely unaudited. Nicolas Kayser-Bril, Spam filters are efficient and uncontroversial. Until you look at them, AlgorithmWatch (Oct. 22, 2020), https://algorithmwatch.org/en/spam-filters-outlook-spamassassin/. 36 See Social Catfish, State of Internet Scams 2021 at 52-53, https://spcdnblog.socialcatfish.com/uploads/2021/07/State-of-Internet-Scams-2021-2.pdf. 37 See Louise Matsakis, Filing Your Taxes? Watch Out for Phishing Scams, WIRED (Apr. 4, 2019), https://www.wired.com/story/filing-taxes-phishing-scams/. 38 See https://webarchive.nationalarchives.gov.uk/ukgwa/20200930155453/https://epsrc.ukri.org/ newsevents/news/aionlinedating/. 39 See Arunesh Mathur et al., Endorsements on Social Media: An Empirical Study of Affiliate Marketing Disclosures on YouTube and Pinterest, Proc. of the ACM on Human-Computer Interaction, Vol. 2, CSCW, Art. 119 (Nov. 2018), https://doi.org/10.1145/3274388; Michael Swart, et al., Is This an Ad?: Automatically Disclosing Online Endorsements on YouTube with AdIntuition, in CHI '20: Proc. of the 2020 CHI Conf. on Human Factors in Computing Systems (Apr. 2020), http://dx.doi.org/10.1145/3313831.3376178. 40 See Emmanuel Gbenga Dada, et. al., Machine Learning for Email Spam Filtering, Heliyon 5(6) (2019), https://www.sciencedirect.com/science/article/pii/S2405844018353404#bib127.
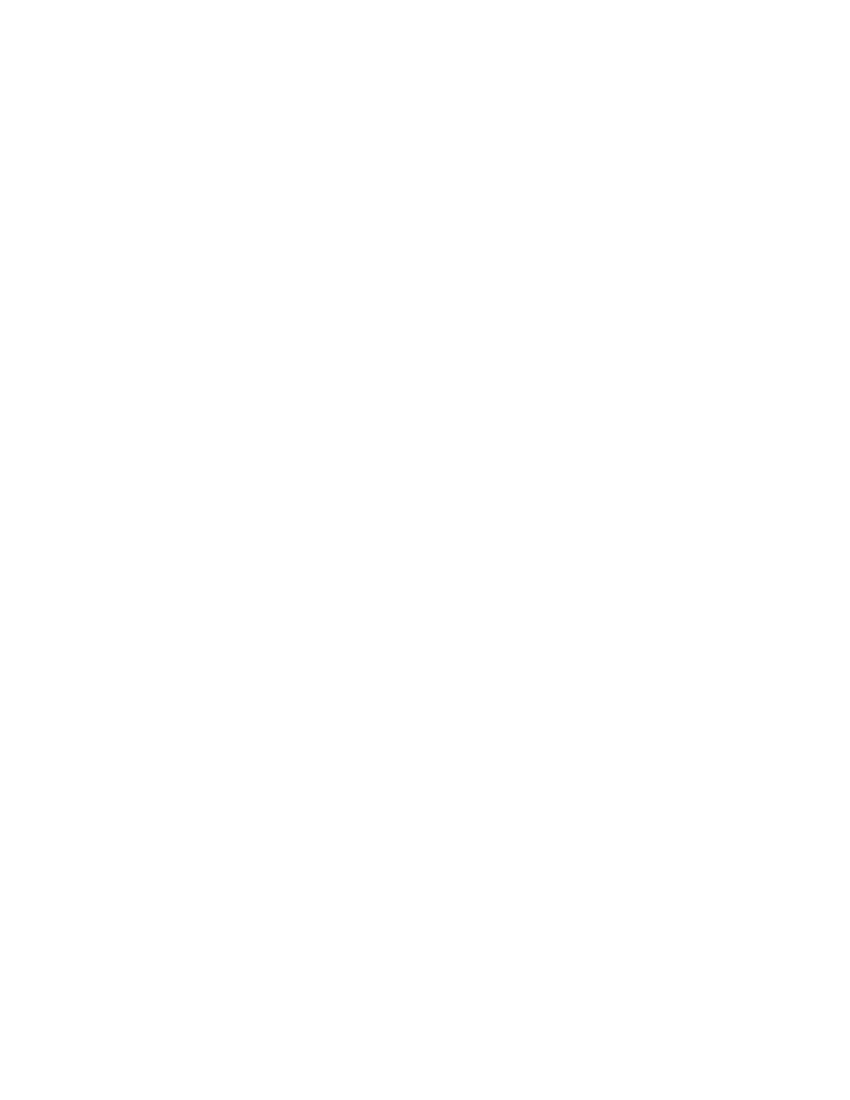