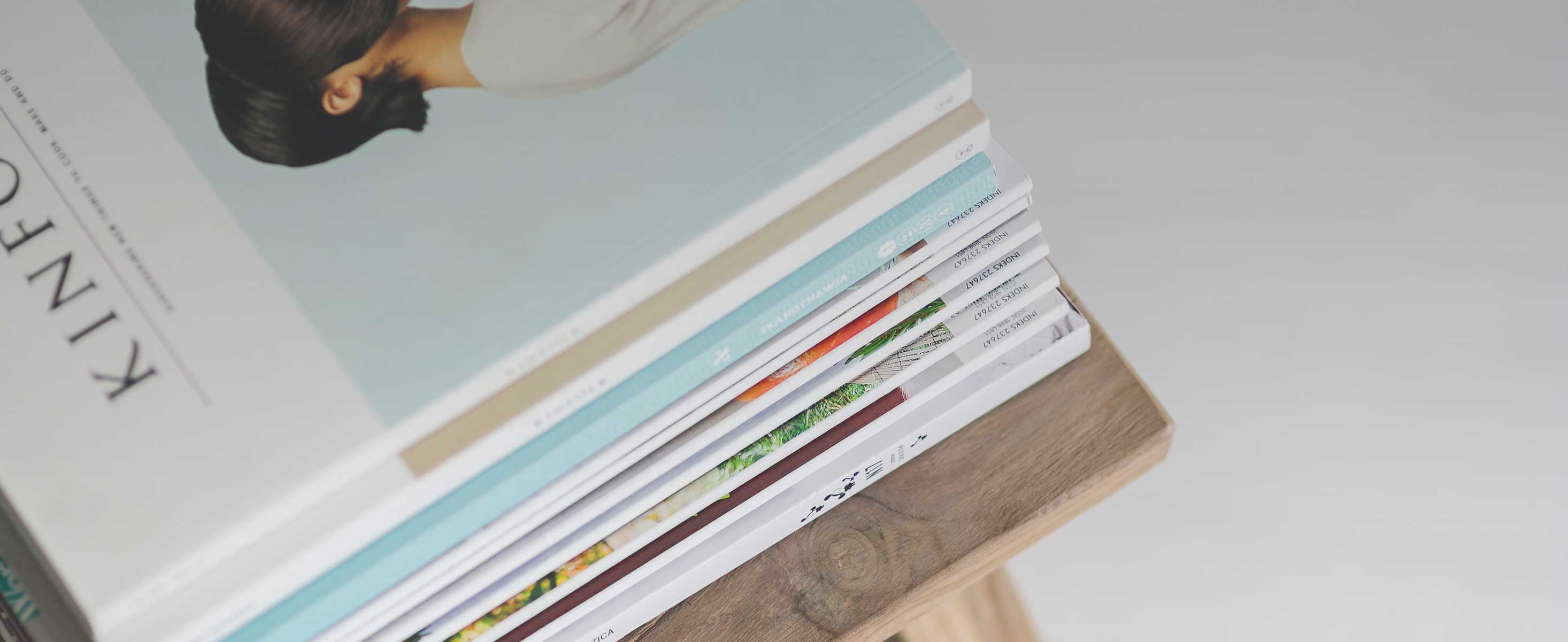
18 minute read
Chapter 13Experiments and Quasi -Experiments
from TEST BANK for Introduction to Econometrics 2nd Second Edition by M. W. Watson and J. H. Stock.
by ACADEMIAMILL
13.1Multiple Choice
1)The following are reasons for studying randomized controlled experiment in an econometrics course, with the exception of
A)at a conceptual level, the notion of an ideal randomized controlled experiment provides a benchmark against which to judge estimates of causal effects in practice.
B)when experiments are actually conducted, their results can be very influential, so it is important to understand the limitations and threats to validity of actual experiments as well as their strength.
C)randomized controlled experiments in economics are common.
D)external circumstances sometimes produce what appears to be randomization.
Answer:C
2)Program evaluation
A)is conducted for most departments in your university/college about every seven years.
B)is the field of study that concerns estimating the effect of a program, policy, or some other intervention or “treatment.”
C)tries to establish whether EViews, SAS or Stata work best for your econometrics course.
D)establishes rating systems for television programs in a controlled experiment framework.
Answer:B
3)In the context of a controlled experiment, consider the simple linear regression formulation Y i = β 0 + β 1 Xi + ui . Let the Y i be the outcome, Xi the treatment level, and ui contain all the additional determinants of the outcome.
Then
A)the OLS estimator of the slope will be inconsistent in the case of a randomly assigned Xi since there are omitted variables present.
B) Xi and ui will be independently distributed if the Xi be are randomly assigned.
C) β 0 represents the causal effect of X on Y when X is zero.
D) E(Y X = 0)is the expected value for the treatment group.
Answer:B
4)In the context of a controlled experiment, consider the simple linear regression formulation Y i = β 0 + β 1 Xi + ui
Let the Y i be the outcome, Xi the treatment level when the treatment is binary, and ui contain all the additional determinants of the outcome. Then calling β 1 ^ a differences estimator
A)makes sense since it is the difference between the sample average outcome of the treatment group and the sample average outcome of the control group.
B)and β 0 ^ the level estimator is standard terminology in randomized controlled experiments.
C)does not make sense, since neither Y nor X are in differences.
D)is not quite accurate since it is actually the derivative of Y on X.
Answer:A
5)The following does not represent a threat to internal validity of randomized controlled experiments:
A)attrition.
B)failure to follow the treatment protocol.
C)experimental effects.
D)a large sample size.
Answer:D
6)The Hawthorne effect refers to
A)subjects dropping out of the study after being randomly assigned to the treatment or control group.
B)the failure of individuals to follow completely the randomized treatment protocol.
C)the phenomenon that subjects in an experiment can change their behavior merely by being included in the experiment.
D)assigning individuals, in part, as a result of their characteristics or preferences.
Answer:C
7)The following is not a threat to external validity:
A)the experimental sample is not representative of the population of interest.
B)the treatment being studied is not representative of the treatment that would be implemented more broadly.
C)experimental participants are volunteers.
D)partial compliance with the treatment protocol.
Answer:D
8)Assume that data are available on other characteristics of the subjects that are relevant to determining the experimental outcome. Then including these determinants explicitly results in
A)the limited dependent variable model.
B)the differences in means test.
C)the multiple regression model.
D)large scale equilibrium effects.
Answer:C
9)All of the following are reasons for using the differences estimator with additional regressors, with the exception of
A)efficiency.
B)providing a check for randomization.
C)providing an adjustment for “conditional” randomization.
D)making the difference estimator easier to calculate than in the case of the differences estimator without the additional regressors.
Answer:D
10)Experimental data are often
A)observational data.
B) binary data, in that the subject either does or does not respond to the treatment.
C)panel data.
D)time series data.
Answer:C
11)With panel data, the causal effect
A)cannot be estimated since correlation does not imply causation.
B)is typically estimated using the probit regression model.
C)can be estimated using the “differences - in- differences” estimator.
D)can be estimated by looking at the difference between the treatment and the control group after the treatment has taken place.
Answer:C
12)Causal effects that depend on the value of an observable variable, say Wi ,
A)cannot be estimated.
B)can be estimate by interacting the treatment variable with Wi
C)result in the OLS estimator being inefficient.
D)requires use of homoskedasticity - only standard errors.
Answer:B
Stock/Watson 2e CVC2 8/23/06 Page 310
13)To test for randomization when Xi is binary,
A)you regress Xi , on all W’s and compute the F- statistic for testing that all the coefficients on the W’s are zero. (The W’s measure characteristics of individuals, and these are not affected by the treatment.)
B)is not possible, since binary variables can only be regressors.
C)requires reordering the observations randomly and re - estimating the model. If the coefficients remain the same, then this is evidence of randomization.
D)requires seeking external validity for your study.
Answer:A
14)The following estimation methods should not be used to test for randomization when Xi , is binary:
A)linear probability model (OLS) with homoskedasticity - only standard errors.
B)probit.
C)logit.
D)linear probability model (OLS) with heteroskedasticity - robust standard errors.
Answer:A
15)In a quasi- experiment
A)quasi differences are used, i.e., instead of △Y you need to use ( Y after - λ × Y before), where 0 < λ < 1.
B)randomness is introduced by variations in individual circumstances that make it appear as if the treatment is randomly assigned.
C)the causal effect has to be estimated through quasi maximum likelihood estimation.
D)the t- statistic is no longer normally distributed in large samples.
Answer:B
16)Your textbooks gives several examples of quasi experiments that were conducted. The following is not an example of a quasi experiment:
A)labor market effects of immigration.
B)effects on civilian earnings of military service.
C)the effect of cardiac catheterization.
D)the effect of unemployment on the inflation rate.
Answer:D
17)A repeated cross- sectional data set
A)is also referred to as panel data.
B)is a collection of cross - sectional data sets, where each cross - sectional data set corresponds to a different time period.
C)samples identical entities at least twice.
D)is typically used for estimating the following regression model
Y it = β 0 + β 1 Xit + β 2 W1, it + + β 1 + rWr,it + uit
Answer:B
18)For quasi - experiments,
A)there is a particularly important potential threat to internal validity, namely whether the “as if” randomization in fact can be treated reliably as true randomization.
B)there are the same threats to internal validity as for true randomized controlled experiments, without modifications.
C)there is little threat to external validity, since the populations are typically already different.
D)OLS estimation should not be used.
Answer:A
19)Experimental effects, such as the Hawthorne effect,
A)generally are not germane in quasi - experiments.
B)typically require instrumental variable estimation in quasi - experiments.
C)can be dealt with using binary variables in quasi - experiments.
D)are the most important threat to internal validity in quasi - experiments.
Answer:A
20)Heterogeneous population
A)implies that heteroskedasticity- robust standard errors must be used.
B)suggest that multiple characteristics must be used to describe the population.
C)effects can be captured through interaction terms.
D)refers to circumstances in which there is unobserved variation in the causal effect with the population.
Answer:D
21)If the causal effect is different for different people, then the population regression equation for a binary treatment variable Xi , can be written as
A) Y i = β 0 + β 1 Xi + ui .
B) Y i = β 0 + β 1 i Xi + ui .
C) Y i = β 0 i + β 1 i Xi + ui .
D) Y i = β 0 + β 1 Gi + β 2 Dt + ui .
Answer:C
22)In the case of heterogeneous causal effects, the following is not true:
A)in the circumstances in which OLS would normally be consistent (when E(ui Xi ) = 0), the OLS estimator continues to be consistent.
B)OLS estimation using heteroskedasticity - robust standard errors is identical to TSLS.
C)the OLS estimator is properly interpreted as a consistent estimator of the average causal effect in the population being studied.
D)the TSLS estimator in general is not a consistent estimator of the average causal effect if an individual’s decision to receive treatment depends on the effectiveness of the treatment for that individual.
Answer:B
23)One of the major lessons learned in the chapter on experiments and quasi - experiments
A)is that there are almost no true experiments in economics and that quasi - experiments are a poor substitute.
B)you should always use TSLS when estimating causal effects in quasi - experiments.
C)populations are always homogeneous.
D)is that the insights of experimental methods can be applied to quasi - experiments, in which special circumstances make it seem “as if” randomization has occurred.
Answer:D
24)Quasi- experiments
A)provide a bridge between the econometric analysis of observational data sets and the statistical ideal of a true randomized controlled experiment.
B)are not the same as experiments, and lessons learned from the use of the latter can therefore not be applied to them.
C)most often use difference - in- difference estimators, which are quite different from OLS and instrumental variables methods studied in earlier chapters of the book.
D)use the same methods as studied in earlier chapters of the book, and hence the interpretation of these methods is the same.
Answer:A
25)The major distinction between the experiments and quasi - experiments chapter and earlier chapters is the
A)frequent use of binary variables.
B)type of data analyzed and the special opportunities and challenges posed when analyzing experiments and quasi- experiments.
C)superiority of TSLS over OLS.
D)use of heteroskedasticity- robust standard errors.
Answer:B
26)A potential outcome
A)is the outcome for an individual under a potential treatment.
B)cannot be observed because most individuals do not achieve their potential.
C)is the same as a causal effect.
D)is none of the above.
Answer:A
27)A causal effect for a single individual
A)can be deduced from the average treatment effect.
B)cannot be measured.
C)depends on observable variables only.
D)is observable since it is used as part of calculating the mean of individual causal effects.
Answer:B
28)Randomization based on covariates is
A)not of practical importance since individuals are hardly ever assigned in this fashion.
B)dependent on the covariances of the error term (serial correlation).
C)a randomization in which the probability of assignment to the treatment group depends on one of more observable variables W.
D)eliminates the omitted variable bias when using the difference estimator based on Y i = β 0 + β 1 X i + ui , where Y is the outcome variable and X is the treatment indicator.
Answer:C
29)Testing for the random receipt of treatment
A)is not possible, in general.
B)entails testing the hypothesis that the coefficients on W1i, …, Wri are non - zero in a regression of Xi on W1i, …, Wr
C)is not meaningful since the LHS variable is binary.
D)entails testing the hypothesis that the coefficients on W1i, …, Wri are zero in a regression of Xi on W1i, …, Wr
Answer:D
30)Failure to follow the treatment protocol means that
A)the OLS estimator cannot be computed.
B)instrumental variables estimation of the treatment effect should be used where the initial random assignment is the instrument for the treatment actually received.
C)you should use the TSLS estimator and regress the outcome variable Y on the initial random assignment in the first stage to get predicted values of the outcome variable.
D)the Hawthorne effect plays a crucial role.
Answer:B
Stock/Watson 2e CVC2 8/23/06 Page 313
31)Small sample sizes in an experiment
A) biases the estimators of the causal effect.
B)may pose a problem because the assumption that errors are normally distributed is dubious for experimental data.
C)do not raise threats to the validity of confidence intervals as long as heteroskedasticity - robust standard errors are used.
D)may affect confidence intervals but not hypothesis tests.
Answer:B
32)A repeated cross- sectional data set is
A)a collection of cross - sectional data sets, where each cross - sectional data set corresponds to a different time period.
B)the same as a balanced panel data set.
C)what Card and Krueger used in their study of the effect of minimum wages on teenage employment.
D)time series.
Answer:A
33)In a sharp regression discontinuity design,
A)crossing the threshold influences receipt of the treatment but is not the sole determinant.
B)the population regression line must be linear above and below the threshold.
C) Xi will in general be correlated with ui
D)receipt of treatment is entirely determined by whether W exceeds the threshold.
Answer:D
34)Threats to internal validity of quasi - experiments include
A)failure of randomization.
B)failure to follow the treatment protocol.
C)attrition.
D)all of the above with some modifications from true randomized controlled experiments.
Answer:D
13.2Essays and Longer Questions
1)You want to study whether or not the use of computers in the classroom for elementary students has an effect on performance. Explain in some detail how you would ideally set up such an experiment and what threats to internal and external validity there might be.
Answer:Answer will differ by students. Students have the choice to suggest a quasi - experiment or a controlled experiment. Although it is possible to focus on states where elementary schools have introduced the use of computers in the classroom, and compare the change in test scores with those in states which have not done so, it is more likely that students will concentrate on a controlled experiment design here. The answer should emphasize the initial random selection of pupils, classrooms, or schools, from the population of a state, or the nation, and the random assignment to a treatment group. Furthermore, teachers must also be randomly assigned. In essence, the random selection and assignment is to ensure that E(ui Xi ) = 0 holds. X could be a binary variable, indicating whether computers were introduced in the classroom, or it could indicate the intensity with which computers were used.
Answers should mention each of the threats to internal and external validity. Failure to randomize might occur because the treatment group could be assigned according to the performance level of students or computers already being used in classrooms, or the previous experience students had with computers. Teachers may be chosen depending on their knowledge of computers and software. Failure to follow treatment protocol is less of a risk here. Attrition is a problem if parents move to another school district or private schools as a result of the assignment to the treatment and control groups. Experimental effects are hard to avoid in this situation since it does not make sense to have a double blind experiment. Small samples should not be a problem in this set - up. Threats to external validity include nonrepresentative samples, which are unlikely to occur here unless there are a large number of volunteers. Similarly, nonrepresentative programs or policy should not pose a problem. There may be general equilibrium effects if more technically oriented teachers have to be hired or others have to be reeducated. Finally, there may be treatment vs. eligibility effects if there is a choice to opt in or out of the treatment and control group.
2)Canada and the United States had approximately the same aggregate unemployment rates from the 1920s to 1981. In 1982, a two percentage point gap appears, which has roughly persisted until today, with the Canadian unemployment rate in the third quarter of 2002 being 7.6 percent while the American rate stood at 5.9 percent in the same period. Several authors have investigated this phenomenon. One study, published in 1990, contained the following statement: “It is a clichė that, as compared to analysis in the physical sciences, economic analysis is hampered by the lack of controlled experiments. In this regard, study of the Canadian economy can be much facilitated by comparison with the behaviour of the US …” Discuss what the authors may have had in mind. List some potential threats to internal and external validity when comparing aggregate unemployment rate behavior between countries.
Answer:It should be clear that the authors were not really talking about a controlled experiment, but instead had in mind a quasi - experiment or natural experiment. In a randomized controlled experiment to study the effect of unemployment insurance benefits on unemployment, for example, unemployed workers would be “treated” with various degrees of unemployment insurance generosity, such as the amount by which their former wages are replaced by unemployment insurance benefits (“replacement rate”), the duration of benefits, the scrutiny of the agency monitoring the job search effort, etc. Instead the authors must have thought that the two economies were similar in many aspects, and that because of an external event, either in Canada or in the U.S., one was subjected to a treatment, while the other was not, which resulted in the aggregate unemployment rate difference. It is the difference in location (living in the U.S. vs. in Canada) that gives the resemblance to a randomly assigned treatment. The above study is of the first type of quasi - experiments discussed in the textbook whereby the treatment received is viewed as if randomly determined.
One threat to external validity is to generalize the results from a U.S. - Canada comparison to other cultural and less developed economies. Also, consider unemployment insurance generosity as a treatment variable. (Canada liberalized unemployment benefits considerably in the early ‘70s). In that case E(ui Xi ) = 0 is unlikely to hold, and additional regressors and instrumental variable techniques should be used.
3)Earnings functions provide a measure, among other things, of the returns to education. It has been argued these regressions contain a serious omitted variable bias due to differences in abilities. Furthermore, ability is hard to measure and bound to be highly correlated with years of schooling. Hence the standard estimate of about a 10 percent return to every year of schooling is upward biased. Suggest some ways to address this problem. One famous study looked at earnings of identical twins. Explain how this can be viewed as a quasi- experiment, and mention some of the threats to internal and external validity that such a study might encounter.
Answer:Answers will vary by student. The omitted variable bias should play a central part in the discussion.
Xi , W1 i ,..., Wri ) = 0 will not hold if one of the W’s is years of education and u contains unobserved ability. If ability causes individuals to have higher earnings and longer years of education, perhaps through obtaining university scholarships easier, then the returns to education are biased upward. One way to circumvent this problem is, as some studies have done in the past, to approximate ability by IQ scores. If IQ scores measure ability with error, then instrumental variable techniques can be employed. These were discussed in Chapter 10 of the textbook. Another possibility is to model ability as an omitted variable that remains constant over time. In that case, panel estimation methods with fixed effects, presented in Chapter 8 of the textbook, can be used. Data can be differenced to eliminate the entity fixed effects or binary variables can be added to capture them. At any rate, this approach requires data being available for more than a single point in time. The use of data from identical twins is fascinating since these have identical genes and, typically, identical family backgrounds. The suggestion is therefore to assume that they have identical ability as well. If some twins have different years of schooling while others do not, then this can be treated as a quasi - experiment since the researcher can view this choice as if it had been randomly assigned. Obviously it cannot count as a randomized controlled experiment, since the difference in schooling was not determined by the flip of a coin, say. But it may also run into problems in providing an as if randomization. The text flagged some of the potential problems in section 11.1: “Initially, one might think that an ideal experiment would take two otherwise identical individuals, treat one of them, and compare the difference in their outcomes while holding constant all other influences. This is not, however, a practical experimental design, for it is impossible to find two identical individuals: even identical twins have different life experiences, so they are not identical in every way.” Finally, if identical twins are “different” from the general population, then there is also a threat to external validity by generalizing the results for the population of all individuals.
4)Describe the major differences between a randomized controlled experiment and a quasi - experiment.
Answer:Answers will vary by student. Some of the following points should appear.
A randomized controlled experiment relies on the random selection of entities from a population of interest, and the random assignment of these individuals into either a treatment or control group. To study the causal effects, a simple regression model with a single regressor can be specified. This regressor can either be a binary variable or a variable indicating treatment levels. Since E(ui Xi ) = 0 is guaranteed if the assignment and selection was random, then the causal or treatment effect can be measured through E(Y i X = x ) - E(Y i X = 0). The random selection and assignment assures that there is no omitted variable bias, and therefore the OLS estimator is unbiased. Adding additional regressors can result in increased efficiency. Alternatively a differences - in- differences estimator with or without additional regressors is also available if the entities have been observed for two periods, one before and one after the treatment. In the case of more than two observations per entity, panel methods can be employed. There are various threats to internal and external validity. These include failure to randomize, failure to follow treatment protocol, attrition, experiment effects, and small samples (threats to internal validity), and nonrepresentative sample, nonrepresentative program or policy, general equilibrium effects, and treatment vs. eligibility effects (threats to external validity).
A quasi - experiment is also called a “natural experiment” since the treatment of some entities resulted from an external event. The treatment is administered “ as if” it was random. The reason for observing quasi- experiments more often in economics is that they are less expensive and raise less of an ethical concern. The “as if” randomly assigned treatment is the result of, as the textbook puts it, “vagaries in legal institutions, location, timing of policy or program implementation, natural randomness such as birth dates, rainfall, or other factors that are unrelated to the causal effect under study.” There are two types of quasi- experiments, one whereby treatment is viewed as if randomly determined, the other whereby the “ as if” randomization provides an instrumental variable. Threats to internal and external validity are the same as for randomized controlled experiments once they are modified. For example, experimental effects are typically absent since individuals are not aware that they are part of an experiment. Small samples is replaced by instrument validity in quasi - experiments.
5)Roughly ten percent of elementary schools in California have a system whereby 4 th to 6th graders share a common classroom and a single teacher (multi - age, multi - grade classroom). Suggest an experimental design that would allow you to assess the effect of learning in this environment. Mention some of the threats to internal and external validity and how you would attempt to circumvent these.
Answer:Students should be selected randomly within a school and should be randomly assigned to a treatment group (multi - age, multi - grade classroom) and a control group (traditional grade assignment; 4 th , 5th , and 6 th grade only per room). Alternatively, and depending on the size of the experiment, a subset of schools could be chosen and some pupils would randomly be assigned to traditional grade assignments while others would be moved into multi - age, multi - grade classrooms. Another alternative would be to simply choose some schools randomly which would have multi - age, multi - grade classrooms only. The causal effect could then be estimated in a simple regression model with a binary regressor. Random selection and random assignment would assure E(ui Xi ) = 0 and thereby eliminate one threat to internal validity through omitted variable bias.
Another threat to internal validity would be if the worst or best performing schools were chosen instead of using a random selection, or if parents in the district were allowed to vote whether or not to have the school selected for the experiment. This would imply a failure to randomize. If students were allowed to refuse to participate by transferring to a neighboring school, then this would represent failure to follow treatment protocol. Double blind experiments are obviously not feasible since both instructors and students know into which setting they are being placed (“experimental effects”). There are few threats to external validity except for the situation whereby students would be allowed to opt in or out of the experimental group (“treatment vs. eligibility effect”).
Stock/Watson 2e CVC2 8/23/06 Page 318
6)Assume for the moment that the student - teacher ratio effect on test scores was large enough that you would advocate reducing class sizes in elementary schools. In 1996, the State of California reduced class sizes from K- 3 to no more than 20 students across all public elementary schools (Class Size Reduction Act) at a cost of approximately $2 billion. In a short essay, discuss why the general equilibrium effects might differ from the results obtained using experiments.
Answer:The General Equilibrium effects are the result of the additional demand for teachers. Each elementary school needed additional teachers in order to reduce the class size to 20 or less think of a school that had perhaps 3 Kindergarten classes of 25 students each. In that case, one additional classroom had to be created typically some temporary structure. The question arises where the additional teacher came from. If your school district was a desirable district to teach in, perhaps because of having a reputation of well behaved children or classrooms that were well equipped, then teachers from other districts, perhaps less desirable ones, would apply to the better school district. Presumably the desirable school district would pick the best teacher(s) available, leaving the less desirable school district with a lower level of teacher quality. The same phenomenon would repeat itself at the lower level school district, and so forth, until you would get to the least desirable school district, which would have to hire new teachers from a cohort that could not find a job elsewhere. Given the size of the State of California, the General Equilibrium effect could be substantial, perhaps even drawing quality teachers from other states.