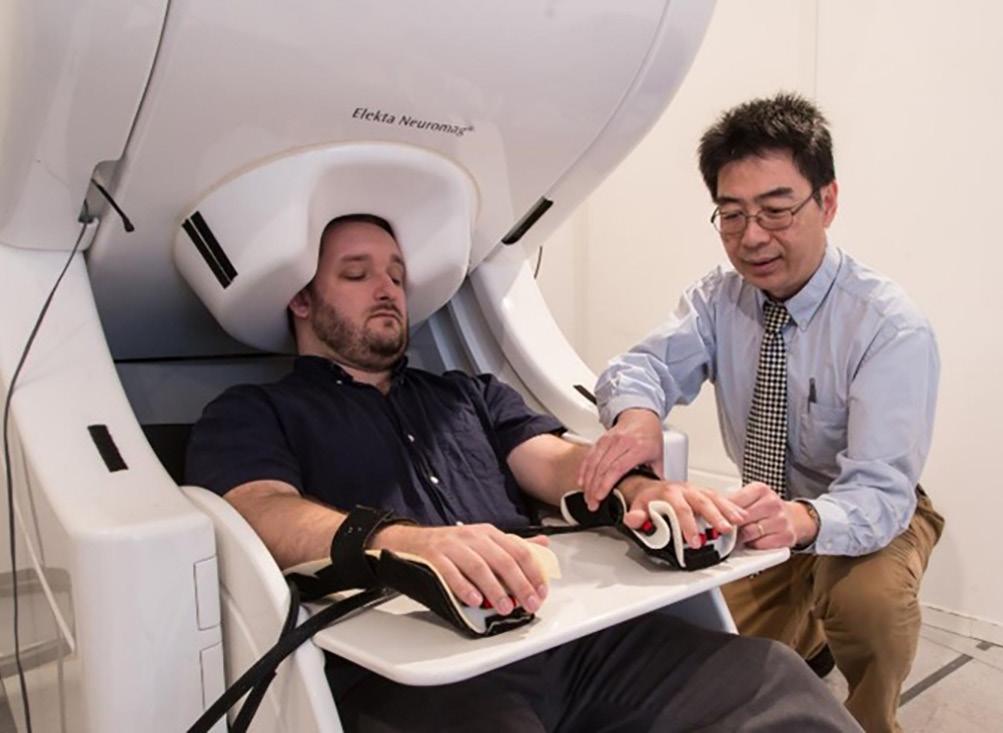
2 minute read
Brain injury and PTSD (Research Project
Developing new machine-learning based neuronal imaging markers for mild traumatic brain injury and posttraumatic stress disorder
By Mingxiong Huang, Ph.D.
Mild traumatic brain injury (mTBI) is a leading cause of sustained physical, cognitive, emotional, and behavioral deficits in OEF/OIF/OND Veterans and the general public. However, the underlying pathophysiology and recovery mechanisms, especially those associated with cognitive functioning in mTBI, are not completely understood. The neuronal mechanisms for the increased risk of PTSD after an mTBI are even less clear. Conventional MRI and CT images are generally negative even in patients with persistent post-concussive symptoms (PCS) and/or PTSD symptoms.
Diffusion-based MRI techniques have been developed to identify abnormalities in white-matter tracts, owing to the major role of diffuse axonal injury (DAI) in mTBI. Yet diffusion-based MRI techniques are not sufficiently sensitive for reliable clinical applications. Recent animal studies indicate that gray matter is also vulnerable to DAI, which leads to abnormal electromagnetic signals from the injured regions. In this regard, support is mounting for the sensitivity of resting-state magnetoencephalography (rs-MEG) source imaging markers for detecting neuronal abnormalities in mTBI.
MEG is a non-invasive neuroimaging technology available in San Diego (see Figure). We demonstrated that rsMEG delta-wave (1-4 Hz) markers were very sensitive in distinguishing mTBI patients with persistent PCS from neurologically intact individuals. We also found that high frequency rs-MEG gamma-band (30-80 Hz) markers show marked hyperactivity in mTBI, possibly due to injury of inhibitory interneurons. In addition, we found that task-evoked MEG (te-MEG) recordings during working memory (WM) task detected abnormal signals throughout the brain in mTBI that were related to worsened cognitive functioning.
Currently, we are conducting a research project funded by the US Department of Veterans Affairs at VA San Diego Healthcare System (VASDHS).
A main goal of this study is to develop highly sensitive diagnostic algorithms to differentiate Veterans with mTBI from those with comorbid mTBI and PTSD, and healthy control Veterans. The new approaches will use artificial neural network based machine-learning techniques to integrate rs-MEG and te-MEG imaging makers. We will study three groups of Veterans: (1) individuals with mTBI and persistent PCS (mTBI-only group); (2) individuals with comorbid mTBI and PTSD who have persistent PCS and PTSD symptoms; (3) healthy controls (HC).
We will establish a machine-learning based MEG diagnostic algorithm for mTBI that optimally integrates three MEG imaging markers (i.e., deltaband and gamma-band rs-MEG; WM evoked MEG) to differentiate Veterans with mTBI (mTBI-only and comorbid mTBI-PTSD) from HC Veterans with >90% accuracy. We will also develop a machine-learning MEG algorithm that integrates rs-MEG activity and te-MEG responses evoked by a negative emotion processing picture task to differentiate Veterans with mTBI-only from those with comorbid mTBI-PTSD with > 90% accuracy.
Furthermore, we will examine the correlates of abnormal MEG-based neurophysiological features in mTBI-only and comorbid mTBI-PTSD with clinical symptoms, cognitive impairments, and realworld quality of life. The success of this project will significantly improve neuroimaging-based techniques that can effectively aid in the diagnosis of mTBI and better characterize the relationships among neurobiological, neuropsychological, and neuropsychiatric effects of mTBI and PTSD.
Project contact information: Call (619) 925-3031 Principal Investigator: Mingxiong Huang, Ph.D., email: mxhuang@ucsd.edu Research Associates: Annemarie Quinto, Hayden Hansen, Jared Baumgartner, Jaqueline HernandezLucas