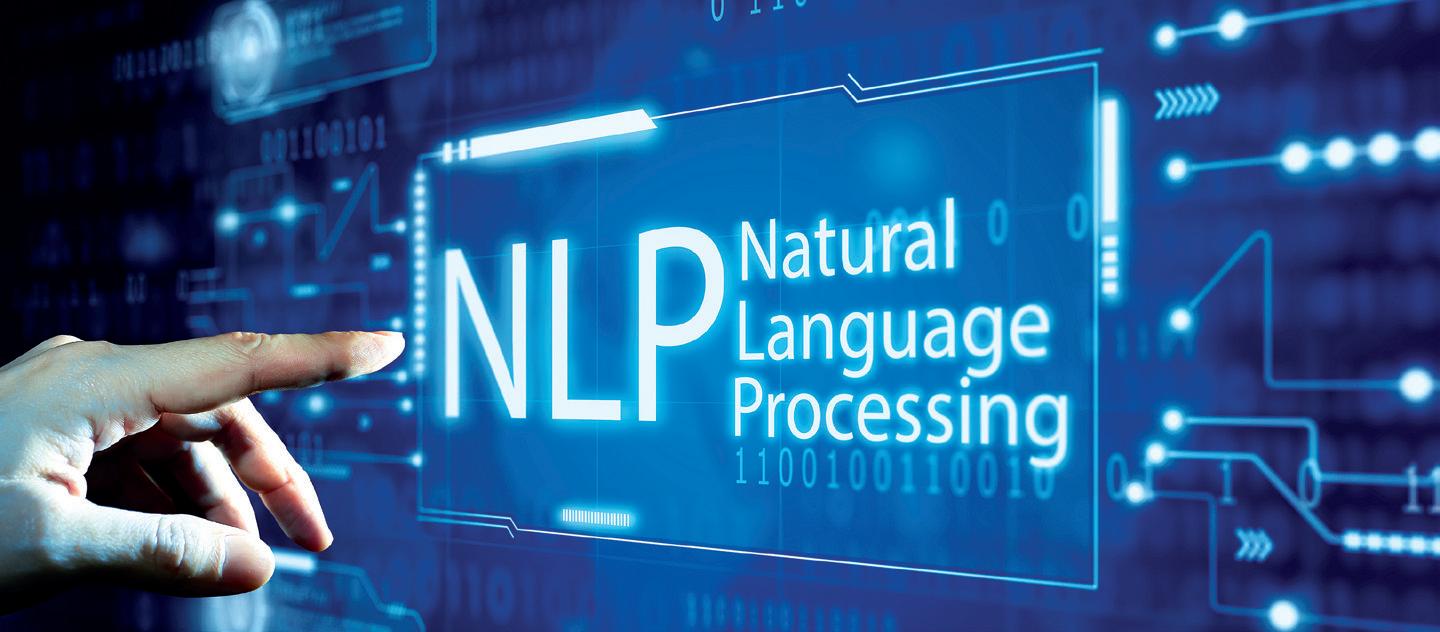
11 minute read
AI see the future
As Martinez explains: “If someone starts funding every day, let’s say it’s 12 o’clock that they make their payments, and then it slips to 12.15, then 12.30, one o’clock… is there something in those events that is saying ‘this counterparty is starting to have some liquidity issues and maybe they should be shut off?’.
“Instead of a human saying, ’I just got a call from risk to tell us to stop making those payments’, is there something that could flash red on the screen that says, ’wait, don’t do anything’?”
Another model SmartStream is examining is more general in nature – the objective being to provide as much clean
The clarity of data: Natural language processing can sharpen-up anti-fraud measures customers sign off on, so they can extract all of those fees for doing the trades,” says Martinez. “We can onboard these schedules much faster, and then get those savings to our customers, which then goes to their customers; if they’re paying less in fees, because they’re doing more transactions with a certain broker, both sides are getting the benefit.” will return ‘here are possibilities’. Before, without that technology, it had to match or it didn’t. Now, it doesn’t have to match; it can just sound the same, because the AI will conduct phonetic searches as well as text searches. We have a bank in Asia, that we’re testing this with now.” reference data for the markets as possible so that their own engines can produce better quality algorithms.
“If what goes in is garbage, that’s what comes out. If you have a lot of breaks because your identifiers aren’t correct, you need to get a provider, such as us, to give you clean data and ensure your algorithms work properly,“ says Martinez.
“SmartStream uses the three Vs. Data has to be verified, so it has to be data that we know is trusted from an organisation. There has to be value to that data – it has to be something that we know will help us get somewhere. And, finally, there has to be volume – it just can’t be one record, there have to be several records.”
It’s also starting to explore AI applications around its fees and expense management product. “One is to use it to understand and read the documents that
An entirely new area of work for SmartStream AIR itself could be in natural language processing for text reconciliation when compiling sanctions lists to reduce the likelihood, for example, of an individual or institution finding themselves on a list because a single letter has erroneously been mis-entered. It’s what Martinez refers to as ‘fuzzy matching’.
“While we were working internally, with AIR, and some of its offshoot projects, internally, we realised that text is a big topic of reconciliation,” says Martinez.
“The point is, we can use that technology not just for numbers, but also for text. We can do anti-money laundering, know your customer. When someone gives you a name to match against a sanctions list, you can ask the AI ‘is this person on any of these lists that we are aware of?’ and it
Looking ahead, Martinez predicts large language models and generative AI like ChatGPT will impact some areas of the financial services industry in a fundamental way.
““In my opinion, artificial intelligence is not going to be finding better algorithms. We’re there already. But, similar to what we’re seeing with ChatGPT, the way AI is going to start changing finance is by processing data in a way that allows new securities and products to be put together. With technologies like GPT, you could say, ‘I’d like to have some kind of interest-type product‘, and the technology will say, ’here are the 10 ways you can do something like that’. That’s what this new technology is doing: using neural networks to make attachments to things that a human may not have thought of because we’ve never been able to put those neurons together.
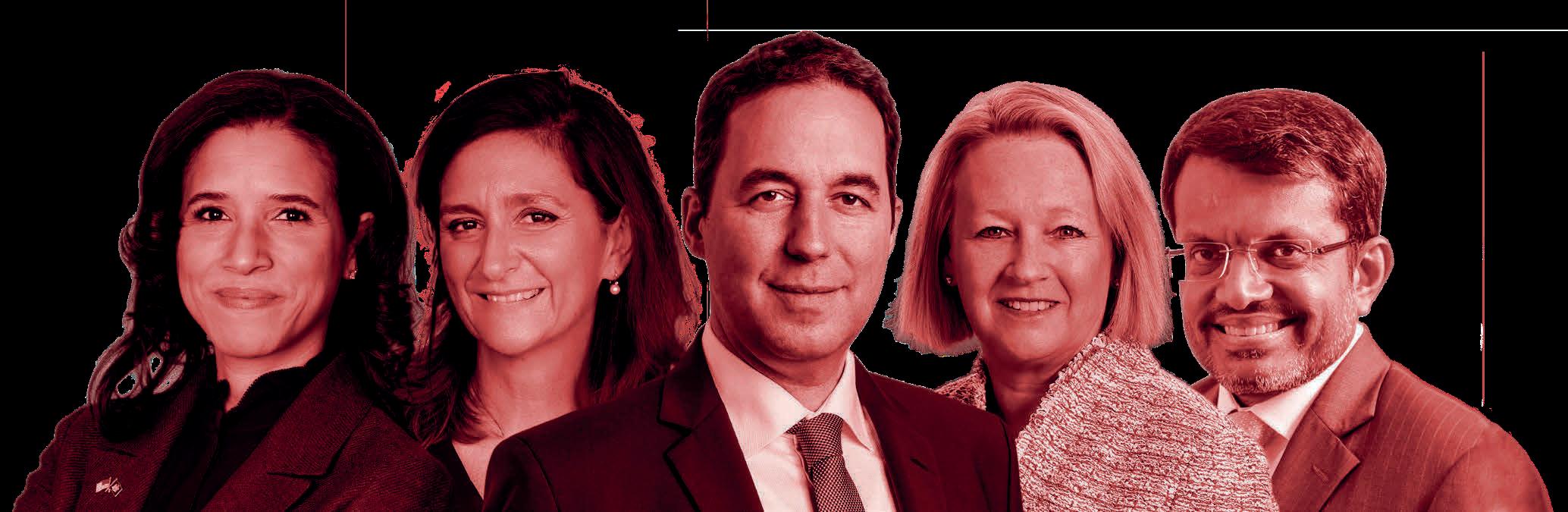
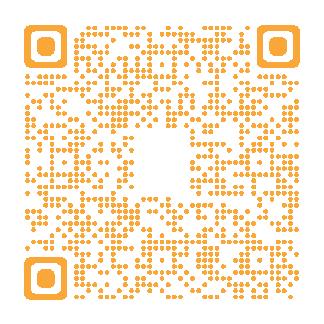
Tech billionaire Elon Musk has pledged to create a ‘TruthGPT’ to address the bias that has plagued machine learning and led to perverse outcomes for consumers. Meanwhile, the CEO of OpenAI, which created ChatGPT, has called on US legislators to regulate artificial intelligence.
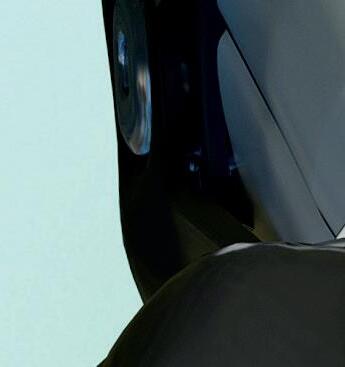
So, given that AI is now an indispensable technology in financial services, how does the industry ensure it’s working to the benefit of everyone?
We put together a global panel of experts to find out. And we started by asking for a definition of AI…
That proved to be a tricky question. Ash Booth, a researcher in machine learning now working for HSBC bank, said that, despite AI being around for six decades, there still isn’t consensus among academics.
“A loose definition that many of us like to use is that it’s a field of computer science that focusses on the development of machines that can think, reason, and perform tasks that humans usually do. To do that, it encompasses different technologies, like machine learning, natural language processing, computer vision, even robotics. Generative AI is part of that subset: it refers to AI systems specifically that create new content, or generate new ideas, based on data they’ve been trained on, and a prompt that’s provided at the time of use.”
While generative AI is stealing all the headlines currently, there have been other major advances in more traditional areas of machine learning that are enabling better decision-making, improved automation, and more personalised customer experiences.
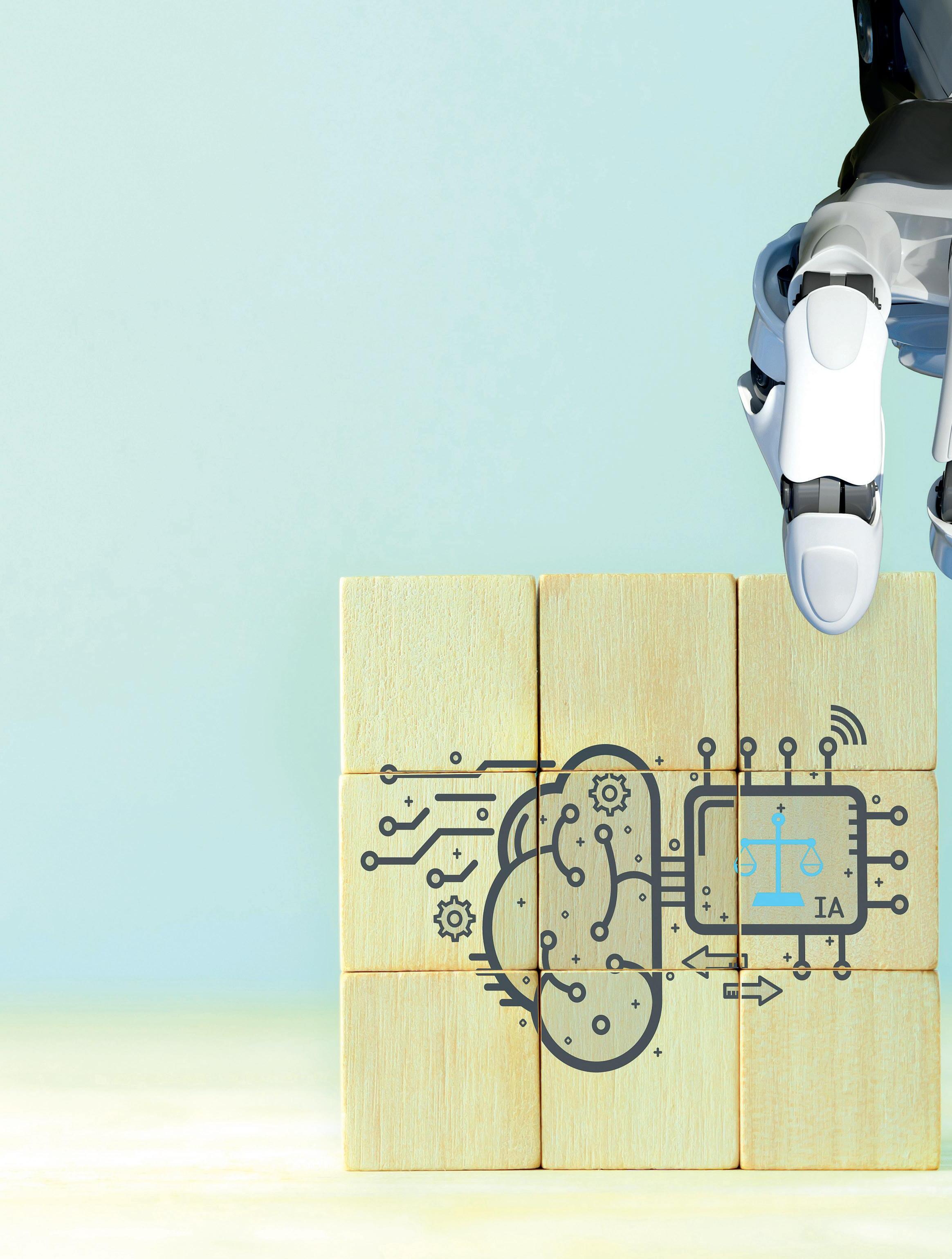
One of those is incremental learning, a machine learning method that is well suited for environments in which data changes constantly, making it difficult for other models to keep up – in weather modelling, for example... or fighting fraud.


In 2021, payments solutions company ACI Worldwide received full patent approval for an incremental learning technology that had been developed specifically to give enhanced fraud protection for merchants and financial institutions, and which was embedded into its ACI Fraud Management suite. It’s designed to give the payments industry a fighting chance in a threat landscape where the bad actors’ agile modus operandi, armies of well-remunerated data scientists and superior tech, challenge financial crime teams every day.
ACI’s Tanya Kopytina, took up the story: “Incremental learning allows artificial intelligence to
The future is now: AI is embedded into much of our daily financial lives continuously learn and adapt as new data comes into the system, so, in the fraud context, that’s as soon as new fraudulent patterns have been established.
“In the hands of ACI, it’s used to address a number of threats. The first one is in account takeover fraud where criminals gain access to the customer’s account or internet banking details, with the purpose of making unauthorised transactions.
“Another is card fraud. That could include card-not-present fraud, or card-present fraud, using a stolen or counterfeit card, which could be the result of online data breaches, some other point of compromise such as BIN attacks, card testing events, or ATM skimming.
“Last but not least is phishing and social engineering scams. But in all cases, the main benefit is that technology enables us to analyse large volumes of data in real time, and to identify fraudulent patterns as they emerge in real time.”
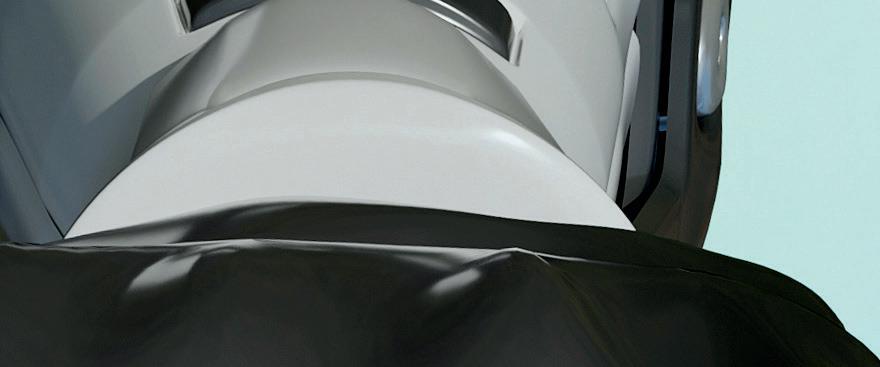
A Biased Perspective
Beyond fraud detection, there is an ongoing debate, though, as to the inherent risks and advantages in incremental learning models, which highlights wider concerns around bias in artificial intelligence. Ash Booth highlighted some of them: “When you’re learning on the fly, as new data comes in, you have to be particularly careful about monitoring things like data bias or model bias in real time. That’s not to say it’s not possible, but you have to be very careful about that. Because the algorithm might start to focus too much on recent examples, or you’re just not detecting shifts in bias, that sort of thing.
“Another potential issue is ‘catastrophic forgetting’, which is where your model is focussing too much on that recent data, and can ‘forget’ important trends that it hasn’t seen for a while, particularly when new information and old information start to conflict.”
He acknowledged that there are ways to mitigate some of those downsides.
Tanya Kopytina explained ACI’s approach: “In order to enable the incremental machine learning technology for fraud detection, first and foremost we need to have the technological capabilities of a fraud detection platform to receive and process data in real time, but also be able to develop machine learning models within the same platform.
“Secondly, to mitigate data bias and ‘catastrophic forgetfulness’ we need to develop features more precisely and more accurately, as part of the model development process. We need to create dynamic features that would be capable to adapt automatically to the changes in fraudsters’ behaviour. When we put these features into the model, the model will be continuously retrained, in a natural manner, and fraudsters basically will be telling us how the model will develop and evolve over time.
“I think it will depend on a combination of technology, fraud, and data science expertise, to be able to apply AI for fraud detection, and ACI has patented its technology for the implementation of that solution.”
Gürer Kural from Rabobank believed that incremental learning was adding a lot of value to AI systems, but cautioned that, beyond fraud detection, there were inherent problems in applying it to other financial use cases: “When it comes to, let’s say, applying it to credit loans it’s trickier, because you cannot say to a customer, ‘your loan request is declined. But if you try tomorrow, maybe you will get it, because our AI system keeps learning!’.
“That’s something that we need to be careful about, because the financial environment, and the trust in the financial environment, is quite fragile, and we know how easy it is to break things.
“So, maybe we can adjust the use cases, and the learning frequency, according to the topic and use case. Because, as Ash mentioned – if you train something on its own data, it will favour its own data and then, once you lose control of the incremental learning, maybe it’s too late, and you will see a model that’s drifted away from its first course – and we don’t want to have model drift or ‘catastrophic forgetfulness.’”
One way to safeguard against such an eventuality and make AI accountable is through an obligation on developers to produce specific AI software engineering documentation that validates procedures and will help in identifying unintended bias and explain decisions that a machine is making.
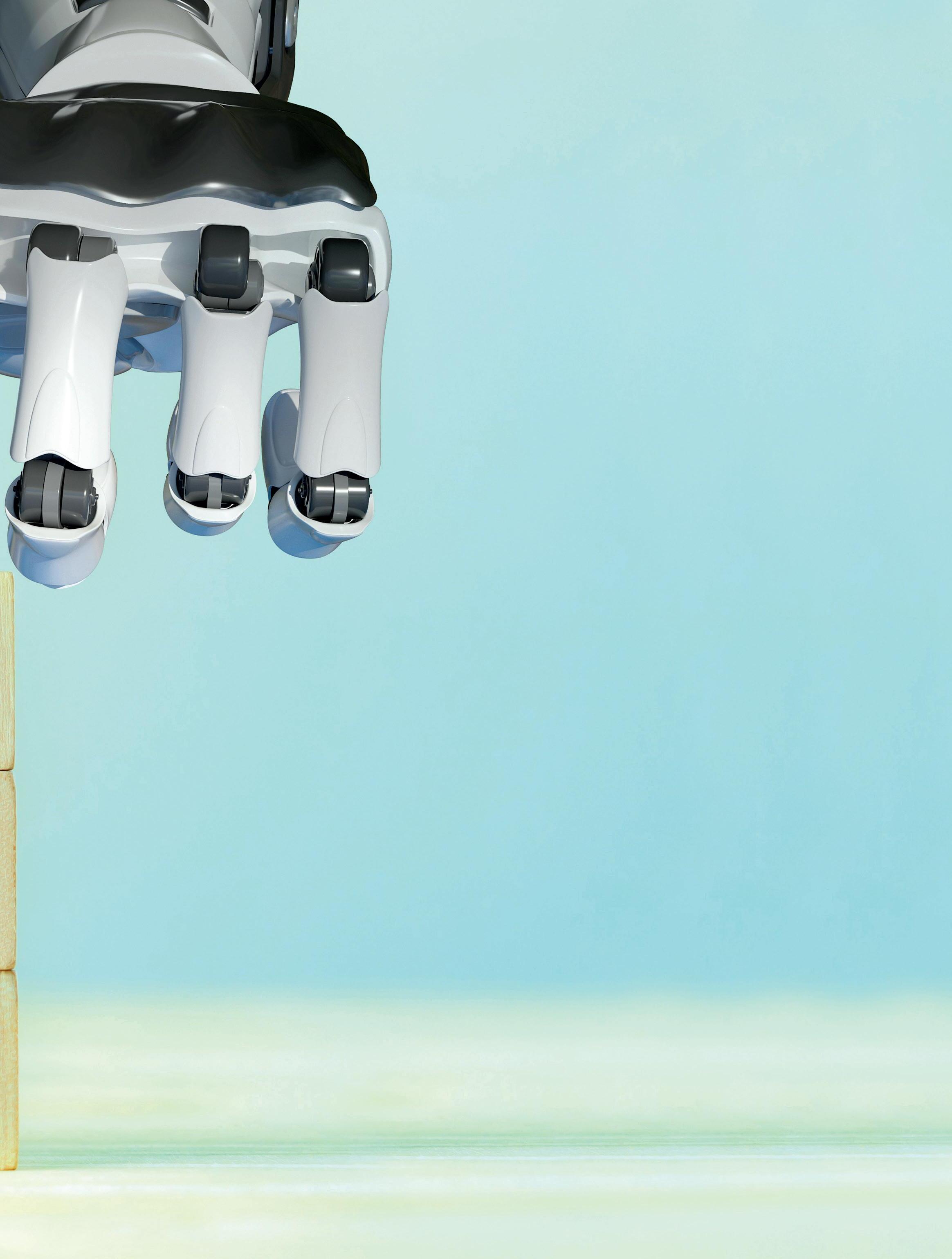
Our Speakers
Tanya Kopytina is an experienced fraud consultant, based in Australia, with a specific interest in automation, advanced analytics and machine learning. She works with real-time payment software company, ACI Worldwide.
Gürer Kural is an analytics consultant at Rabobank, in the Netherlands, where he deals with strategic change and risk management. He’s currently involved in ethical and generative AI.
Ash Booth is head of artificial intelligence for the markets and securities services business at HSBC bank in London, working with the trading, sales, structuring, quant research and operations team in the bank’s markets business.
There are well-known examples of groups of people discriminated against by AI, not because the programmer intended it, but because the data on which the AI was based itself obscured a bias. That results in a clear pattern of unfairness emerging. But it’s harder to identify where a random bias has occurred and challenge it.
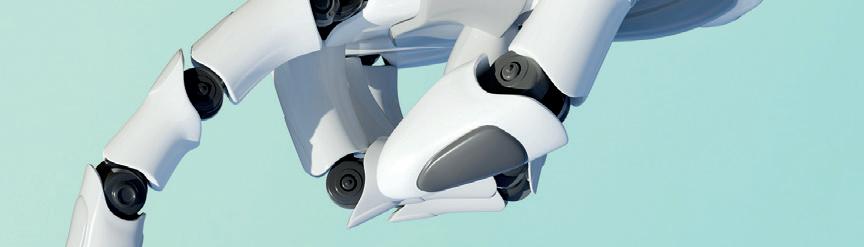
Take the real case of a COO at a UK financial institution who, to his dismay, discovered that his own company’s AI had flagged him as a possible gambler. It had based that on the fact that he frequently used an ATM that happened to be next to a betting shop. Most ordinary people would never find out why a system had assigned them the label it had.
Such cases highlighted the need for true focus on fairness and explainability, said Ash Booth: “Explainability, in the sense of ‘what are the things that are driving my model?’. In this case, it seemed to be really focussing on where cash was withdrawn. And then you should ask, ‘is that fair? Is that a reasonable metric? What spillover might that have? Do I, as a person creating this model, believe that basing our decision on the location of cash machines is fair,
Where we see it falling down is when the right governance isn’t in place.” as possible, and the most structured, easy-to-work-with. So, I think smaller banks can benefit from using machine learning focussed on specific use cases where they have a competitive advantage, or a unique expertise, whether that’s small business lending or niche markets.
That struck a chord with Tanya Kopytina: “I absolutely agree. It’s up to the data scientists to be able to interpret this data – to collect and aggregate the data in a way that helps us to generate some insights from the model development.
“If I come back to fraud, we need to have some fraud expertise, to be able to aggregate the data in a way that we could gather some insights about a typical customer’s trusted behaviour, so we can compare that to the current suspicious or unknown activity. Those insights could be done with the data, though – that’s basically the beauty and the art of machine learning model development.
“They could take advantage of this early stage of AI by organising their data better than banks that have to contend with a lot of tech debt, and by building their data architecture in an AI-first way.”
Gürer Kural agreed: “There’s a tendency to think that the more data you have, the better your model will be. This is wrong. The better the data you have, the better your model will be. When it comes to data, it’s quality over quantity.
“That means the larger datasets that international banks have, for instance, might be a problem for the developers, because you will lose time and effort
Ash Booth, HSBC reasonable, and ethical?
What other implications might that have?’.
“You need to be able to unpack your model in that way, and you should be doing it when you have a human training a model. When these models go live and they’re updated in real time, we need to have the systems in place to monitor for these sorts of biases in real time, too.
“There’s so much you can do to measure for and mitigate bias. The statistics and the practices exist to make sure this bias doesn’t happen. But, ultimately, it’s about thoughtful and sensible governance around training and deploying of AI models, that look for things like these proxy measures.
“Another important point with regards to the fraud detection and incremental learning, is the need for accurate, consistent and complete fraud automapping. If we can provide the feedback about what fraud looks like to the system, our models will be able to generate better insights, as a result.”
Finally, we wanted to know if this was inevitably an area of financial services where the biggest institutions that had access to the largest repositories of data were at an advantage.
Yes – and no – said Ash Booth: “Larger financial institutions have more data available to them, and perhaps that’s giving them an advantage in training larger or more widely representative machine learning models. However, managing massive numbers of diverse datasets is a tricky technological problem.
“If I’m trying to solve a problem, I want to use the least amount of data untangling it, whereas with the smaller banks, maybe it’s easier.”
For Tanya Kopytina, working in the field of financial crime, there was more advantage in large and small banks sharing data – or at least patterns of data: “ACI has developed a Network Intelligence Module that enables financial institutions to collaborate. So, when a big bank detects fraudulent activity or identifies some point of compromise, they can share these fraud signals with smaller banks, which can use that information in a preventative manner.
“We are not sharing private or sensitive information, it’s more a mathematical formula, but it could really help a smaller organisation to fight fraud more effectively by utilising insights they don’t possess.
“That could also promote fairness and accountability because they can avoid their AI models alerting on an unfairly targeted audience, such as specific demographics. And that actually promotes transparency as well as really disrupting the whole chain of fraudulent activity.”
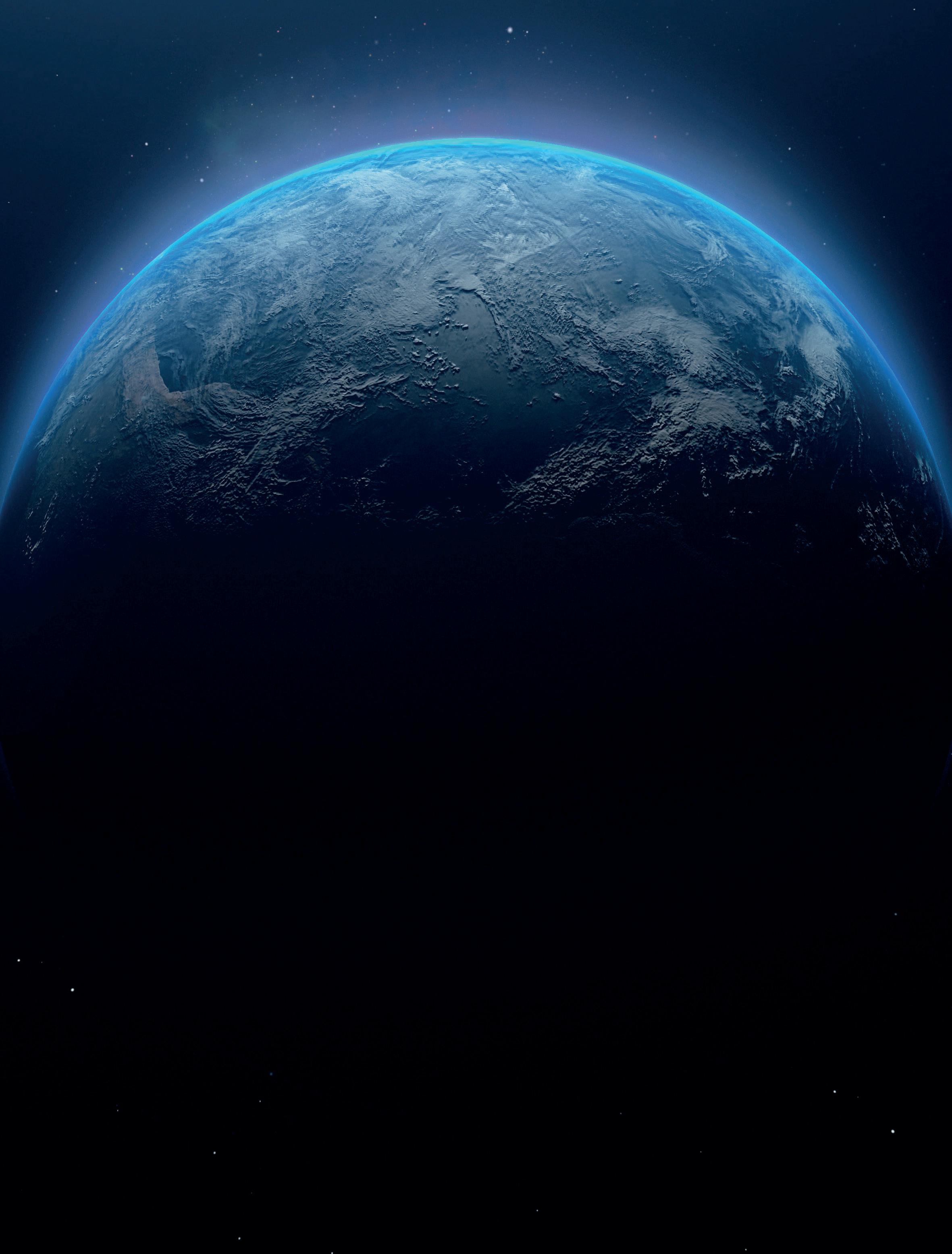