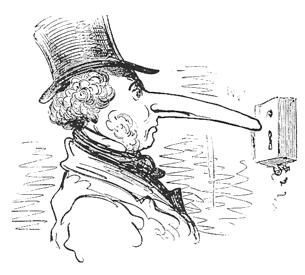
8 minute read
Chicago Library
Let’s Get Technical — Text and Data Mining Support at the University of Chicago Library
By Jessica Harris (Electronic Resource Management Librarian, University of Chicago Library) <jah1@uchicago.edu>
and Kristin E. Martin (Director of Technical Services, University of Chicago Library) <kmarti@uchicago.edu>
Column Editors: Kyle Banerjee (Sr. Implementation Consultant, FOLIO Services) <kbanerjee@ebsco.com> www.ebsco.com www.folio.org
and Susan J. Martin (Chair, Collection Development and Management, Associate Professor, Middle Tennessee State University) <Susan.Martin@mtsu.edu>
Abstract
The University of Chicago Library has long supported research projects using non-consumptive text and data mining (TDM) methods, including acquiring datasets, licensing platforms, and directing users to APIs. As the number and breadth of research projects utilizing TDM has grown, the Library has adapted to support this growing area of research. The article will cover the evolution of the process to support TDM within the Library, including the licensing work, types of resources available, and expertise needed to have a successful TDM program.
Introduction
The University of Chicago is a mid-sized doctoral university classified as Very High Research Activity. As of fall 2021, graduate students outnumber undergraduates, with FTE numbers of 10,279 and 7,618, respectively. Researchers have long had an interest in being able to explore corpora of text and data, both for exploratory purposes and for specific research questions.
To help understand the types of research the Library needs to support, we’ll begin with a definition of Text and Data Mining (TDM). A good overview, including a comprehensive definition can be found at the Carnegie Mellon University Libraries website: “The extraction of natural language works (books or articles, for example) or numeric data (i.e., files or reports) and use of software that read and digest digital information to identify relationships and patterns far more quickly than a human can.”1 The use of automated tools to process large volumes of digital content can identify and select relevant information and discover patterns or connections. In general parlance, text mining extracts information from natural language (textual) sources, while data mining extracts information from structured databases of facts. TDM incorporates both types of sources. TDM is also sometimes called non-consumptive research (e.g., not a “close reading” of the content). Recent legal rulings have supported this type of computational, non-consumptive research as acceptable under U.S. Copyright law, allowing corpora of text still under copyright, such as the full set of content available within HathiTrust, to be available for text mining.2
The Situation
Initial TDM research at the University of Chicago focused on collecting data sets or compiling corpora of text. For example, the Library is a member of the Linguistic Data Consortium (LDC),3 and collects linguistic data sets, first by CD-ROM, and later through downloadable websites. Early on, vendors often restricted licensing of TDM content to specific research projects; files could not be shared with the full University community. Researchers frequently approached projects with significant technical expertise and might even develop their own technical infrastructure to host and analyze the data directly, such as the ARTFL project of French-language texts.4 Researchers approached the Library with specific desires to obtain data sets digitized by commercial vendors. The Library then negotiated special access to obtain the data sets, which were often delivered via mail on hard drives. Large scale research projects, such as the Knowledge Lab,5 focused on collecting comprehensive data sets of full text from large scholarly publishers and metadata from academic research databases. While some providers were accommodating in supplying desired datasets, as the TDM market has grown, many “While some viewed it as an opportunity to providers were monetize a new research method, accommodating and pricing could be unaffordable. Other providers had concerns about sharing full data, viewing it as a in supplying desired datasets, loss of intellectual property, or if as the TDM aggregating content from other rights-holders, did not have rights to be able to supply data. market has grown, many As the idea and use of nonconsumptive research grew, new viewed it as an opportunity types of users began approaching to monetize a the Library for services, including many graduate students and some undergraduates as well. The new research method.” Electronic Resources Management (ERM) Librarian would partner with a subject specialist to help identify the research question and types of resources needed, and then work with content providers to attempt to obtain the data. Content providers often lacked timely methods for supplying the data, leaving disappointed students unable to wait for months for requests to go through a licensing review. Others, more technically savvy, might attempt to script and download large quantities of articles from databases and licensed websites, violating the University’s license agreements and potentially interrupting access for the entire campus community. Clearly, things needed to change. New mechanisms for obtaining data for TDM began to gain more traction. First, some content providers were willing to supply the full text XML and files of large, purchased archives, particularly for public domain materials, and license them for the entire university community. Other providers began offering APIs for TDM purposes on their website. This allowed researchers to extract the data they wanted and the publishers
to protect their intellectual property. The ERM Unit worked with the Digital Library Development Center to safely host and control access to large corpora of data. What the Library still lacked was robust technical help for researchers not fluent in skills needed to perform TDM, either when trying to manage the large sets of data or using the publisher APIs. In the next section, we’ll talk about our current approach to TDM support and how we are trying to leverage three facets to provide comprehensive support: • Licensing language that supports TDM • The acquisition of data sets and other desired content • TDM technical support for researchers
The Process
To help mitigate the delays that occurred when each individual TDM request required a specific license, the ERM Librarian negotiates license language that supports TDM when acquiring or renewing e-resources that can be used as blanket licensing across all requests. Many consortia have their own recommended licensing language that libraries can refer to and customize to make their own. For some examples, see the BTAA,6 CDL,7 and Liblicense8 standardized license agreements. CRL goes a step further by also creating a shared document9 of model license terms and specifications to use specifically when licensing data resources. While some content providers still require a statement of research for individual projects, having basic TDM rights established in the general license has helped speed up the time from inquiry to data delivery. Unfortunately, some content providers continue to refuse to include TDM language, or offer exorbitantly expensive options to support TDM access, leaving some resources out of reach. Some of the commonly requested and acquired data sets at the University of Chicago include geospatial data, linguistic corpora, research citation data and historical and current newspapers. Many of the newspapers for which we receive TDM requests were purchased under a perpetual license through ProQuest. Originally, ProQuest supplied the XML files of the full newspaper content, but it proved technologically challenging to supply files of many gigabytes. Additionally, the years of coverage frequently ended in the early 1930s, so the files did not address many research needs. In 2019, we piloted and later subscribed to their new TDM platform, TDM Studio, to provide access to current news sources. TDM Studio allows an unlimited number of “workbenches,” which must be requested by filling out a short form. Each workbench contains a researcher’s project and allows them to mine most of ProQuest’s content (including newspapers, journals, theses & dissertations) using Jupyter Notebooks. Researchers must have knowledge of either Python or R to use the workbench. Since we licensed TDM Studio, 22 workbenches have been created. As the number and type of TDM content has grown, we explored several avenues to increase the visibility and information regarding TDM resources. To provide for an overview and discovery in a single location, we created a LibGuide, which lists our text and data mining sources by type.10 This list includes resources for which we’ve successfully negotiated TDM rights. These terms are represented in our ERM (FOLIO) and links to content that has been intentionally purchased for TDM purposes are available in our online catalog. To provide technical support to researchers, it’s helpful to understand the processes that researchers use to text and data mine. For instance, what tools will be needed to extract, clean, and analyze the data? What skills will be required of the researcher? With vacancies in both positions that would be knowledgeable about TDM needs, we needed to expand the skill set of less experienced staff. To introduce text and data mining, several librarians enrolled in two Electronic Resources and Libraries (ER&L) 2021 TDM workshops: Fundamentals of Text Mining and Learning to Text and Data Mine with Jupyter Notebooks on Google Colab. Both workshops were excellent starting points for understanding the basics of TDM and how to get started with TDM using free, open source applications. In Spring 2021, the Library also began participating in ITHAKA’s Constellate beta program,11 which helps to empower librarians, faculty, and other instructors with the skills needed to teach text and data mining, including basics of how to program with Python. Twelve participants at the University of Chicago took the introductory course, Introduction to Text Analytics, including librarians, faculty, staff, and a PhD candidate. These training sessions have allowed us to more effectively engage with faculty and staff on their text and data mining needs and to direct them to the resources needed based on both their research topic and level of knowledge with programming languages.
Looking Forward
At the beginning of 2022, working through the Big Ten Academic Alliance, the Library acquired the Web of Science (WoS) Expanded API, and the WoS & Emerging Sources Citation Index (ESCI) backfile XML. We are also considering a license for Cadre,12 which was developed by Indiana University to help query and analyze large datasets, such as the WoS & ESCI XML files. We anticipate these new acquisitions, along with the Scopus API, will help meet the need for more complete citation data for large-scale research projects. As our program evolves, ongoing assessment of the TDM needs of our researchers will become paramount as we decide what avenues to take to provide further content and services. We’ve recently hired a new Director of Digital Scholarship and are actively recruiting for a Scholarly Communications Librarian. Both roles will be essential in shaping the future of our TDM program. Our ERM unit will continue to push for TDM rights in our licenses and expand the discovery and access to TDM content for all University of Chicago researchers.
endnotes for this column on page 60