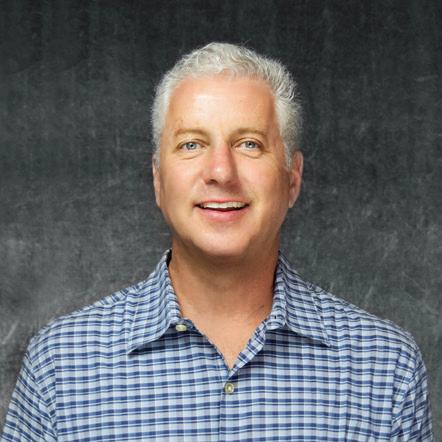
18 minute read
Innovator's Saga- An Interview with David Myers
Column Editor: Darrell W. Gunter (President & CEO, Gunter Media Group) <d.gunter@guntermediagroup.com>
DARRELL GUNTER: I’m pleased to interview a very longtime, industry friend and colleague, Mr. David Myers. He’s the CEO of the Data Licensing Alliance and the CEO of DMedia.
David, welcome to the Innovators Saga. I appreciate you coming on to talk about the Data Licensing Alliance.
DAVID MYERS: I appreciate it, Darrell. It’s always great to see you and have conversations. We’ve known each other for a long time, so happy to be on the show.
DG: Yeah, we didn’t have gray hair back then.
DM: Yes. Trials and tribulations of the industry, you know what I’m saying!
DG: There you go, there you go. So, if you could, for our audience, could you share a little bit about your education background so our audience can get to know you a little bit?
DM: Absolutely. I’ve had a really varied background and I think that, interestingly enough, I didn’t have a vision of how all the pieces would fit together, but it interestingly works for what I do. Education-wise, I have an undergraduate degree in genetics and business. I received my MBA from Pepperdine University and went to work for Texaco, a Fortune 10 company, doing strategic planning and then was a commodity trader for them.
At the same time, because of the timing of that, I was on the West Coast, I worked for them in the morning, and then I did investment banking at night and then went full-time investment banking for a number of years, came back to the East Coast and went to law school at night and started my first company. I was part of a great little venture in the dotcom era that we ended up selling to a New York company. Then I got recruited into the publishing industry where you and I got to know each other. That was in early 2000. I went to work for Wolters Kluwer. At the time it was Ovid, but I was one of their first hires when Ovid was acquired by Wolters Kluwer. I worked for them for seven years and then left and started my own consultancy, DMedia Associates, and still have that company to this day.
We’re a bespoke consultancy helping very large, or really companies of all sizes, but very large organizations with their data licensing needs as a service business. Well, let me take a step back. I know firsthand how complicated and inefficient the licensing of data is from my consultancy. And, so, during COVID, I came up with an idea — I’d been struggling with this idea actually for a long time — of how to make licensing more efficient. I came up with Data Licensing Alliance. It’s like the product side of my service business. It’s the yin to the yang, and it is a marketplace for licensing data specifically for AI. We’re focusing on AI. We have since the beginning, way before ChatGPT and all this generative AI noise that has come into the marketplace, but we’ve been doing it for a better part of three years. And that’s me in a nutshell.
DG: This is very interesting. You said that the Data Licensing Alliance is to make the process more, is it more transparent, would you say?
DM: That’s a great question. It’s certainly more transparent. Our tagline is we make AI smarter, but really what it is, is, it’s the marketplace that makes it easier for data science to license data for their efforts. And it’s applicable to any type of data. We happen to be focusing on the sciences and drug discovery as a first niche, but we have other niches as well. If you think about it, just like Amazon matches somebody selling T-shirts to somebody wanting to buy T-shirts, DLA will match buyers and sellers of data. So, just like you go on Amazon and you find different products that you want to add to your cart, and then you check out. It’s essentially the same thing on the DLA marketplace.
DG: Wow, that’s awesome. So, who sets the price? Does the publisher set their own price or is there a negotiation on the platform between the buyer and the seller?
DM: We put the power with a licensor, or seller in more layman’s terms. Absolutely. We make it more turnkey. There is no real negotiation. When you’re on Amazon and you see again a T-shirt for $12.95, you’re not negotiating with the seller. You either like that price or you don’t like that price. The licensor has the ability to price their products in multiple different ways on our platform, and they’re the one that sets the price. We really see ourselves as a channel to democratize data.
DG: I guess in one sense you’re also an aggregator of data that people can then search, find and decide they want a license.
DM: Absolutely, yes. An aggregator in that sense, just like Amazon is an aggregator of, again, I use that analogy of T-shirts and everything else. We are, too.
DG: Wow. And how long has the platform been in service?
DM: We started about three years ago, actually about three and a half years now. And great timing with COVID, of course, but it didn’t really affect us by and large because the whole industry is moving towards digital, it’s moving towards virtual. And so our problem doesn’t go away. My DMedia business, the DLA business, it’s all been pretty steady since then.
DG: Absolutely, absolutely. And if you can publicly disclose this, if you can’t, I understand, but how many customers do you have on the platform currently?
DM: I don’t want to get too far into that. We have a number of very prominent sellers like John Wiley and Sons, and American Medical Association and a bunch of others. We’ve been focusing really on the supply side and we’re onboarding a number of others before we really roll out too hard to the buy side.
DG: Okay. Wow. And so what do you see as your main selling point to the licensor?
DM: So, on the supply side, right? You’re asking why would they want to do that? Great question. There’s a number of issues that data owners have, and it’s because, number one, data licensing is very complicated. And I would say the majority do not have any experience or comfort level in licensing data. They understand how to sell subscriptions. People have been doing it for a long time, but licensing data, especially for AI, it’s a different animal. We offer expertise. We offer simplicity. We offer a channel to be able to target different layers of the ecosystem.
As you and I both know, many publishers or data owners sell to consortia and to institutions, but they stop at the institutional level. We have the ability to go down to the department or even the researcher themselves and target them. We can get much deeper, much more granular, because of the way the platform is structured. So, again, we are a channel and we offer those types of services to our partners. And lastly, what’s really kind of the salient point about AI is comprehensiveness. So, when somebody goes and wants to train their algorithm on data, they need data from a bunch of different sources to eliminate bias and improve diversity. And this is a one-stop shop for them. The rising tide, as they say, will raise all boats.
DG: Wow. And that’s fascinating. In an article I recently wrote for research information on AI, there was a story about the Samsung engineers who actually were trying to speed up their development process and they put Samsung code into chatGPT which meant that now everybody has access to their code. How do you manage those situations of when people license some data, and what are the limitations. What are the most common things that they need to be mindful of when licensing your data so that someone doesn’t take your data and then create another derivative product?
DM: Well, there was a number of questions within that question. On DLA, the whole process is regulated or controlled by contract. It’s all about contract. One of the steps in licensing data, you add your products to the cart, and when you check out, the first thing you do is sign a license electronically. And it’s that license that controls the relationship between the seller, or the licensor and the licensee, or the buyer. We are just the conduit, but we are not party to that contract. So, contract controls it, which is an important element. Part of the reason that’s an important element, under contract law, fair use is not an option.
So, just interestingly enough, but really the things that control the arrangement, it’s about the supplier. We have a governing law, we have the prohibitions and the rights that they have. Most of the people that license data for text and data mining or AI will be creating a derivative product. Barring that almost eliminates the need or the use case for licensing data. So, that is a right granted. What they can’t do certainly is utilize the underlying copyrighted product that the licensor has. That’s an important fact. And it’s controlled by contract. Certainly, when the contract’s over, they have to remove their data and a bunch of other items that I’m happy to talk about.
DG: Interesting you should mention that. A few years ago, when I was a consultant for STM and one very large open access publisher was dropping their membership to STM because they felt that, since they’re open access, that STM really wasn’t supporting open access as maybe they probably should have at that point. They do now. But, he felt that there was nothing for him because he wasn’t concerned about anybody stealing their material. And I said to him, “that’s interesting, but what if,” and this conversation was in 2016, I said, “what if someone based upon your product, they ingested all of your information, but they created a derivative product? Would you be concerned about that?” He goes, “oh, I never thought about that.” And now we see, of course, with AI, that happens. Have you seen any agreements lately where people are saying, “okay, you can use my product for a derivative product, but I want you to wet my beak. I want a piece of that pie.” Have you seen folks say okay?
DM: Oh, absolutely. And what I caution them on is how do you audit and even account for that? Because if you remember what I just said, it’s about the comprehensiveness. Somebody’s
creating a derivative product, but it’s most likely been trained on countless amounts of data, not only from one supplier. So, how do you know? ChatGPT has been trained on billions of data points. How do you split that up? How do you know the percentage? It is rife with it. What data owners should really think about is what is the value of this to that license and worry more so about that. Trying to get a long tail of payments off of derivative products is rife with problems. I’m not saying it’s not possible. And yes, I’ve seen it, I constantly see it, but it is problematic. However, if I may go off on a tangent for a second, if that’s okay with you.
DG: Absolutely.
DM: So, I have two soapbox issues. One of them is really about subscriptions. And within the subscription is a multitude of rights and responsibilities. And the second of them that is under a subscription is the rights for humans to read and potentially the right for machines to mine. And a lot of publishers, at least today, are giving away their text data mining rights as part of a subscription. My argument should be, those should be decoupled. So, a subscription is the right for humans to read. A license is that for machines to mine. And if you understand and agree with that concept, what ends up happening is you don’t necessarily have to charge. You can charge for both. You can charge for one and not the other. But, what’s interesting is it solves the OA problem, or at least one of the OA problems, especially with federally funded information.
What ends up happening is there’s this movement that any federally funded research that becomes an article, that article should be given away for free. And publishers are having a hard time getting their head around it because that’s where they make money. My argument is, great, no problem. It’s federally funded and you want us to give it away for free? Great. Here’s a repository. As a subscription, you can read it for free, but if you want to mine it, different use case, you can still charge for it. Now there’s an interesting answer to that problem. And, so, that’s my strategy, or at least my argument.
DG: I love this. First time I’m hearing this, this is great. But, so, how do you read something without... Now, it depends upon the definition of what is mining, right? Is that a fundamental search?
DM: I’m talking about machines to mine, right? So no human is looking at this. And machines will do it in a nanosecond where it could take humans days, weeks, months to ingest it.
DG: That’s right, that’s right. That’s right.
DM: It’s a completely different use case. It’s like if you remember way back in the day when we first got started, print was everything, and then digital came along. If you think about it as like a pyramid, the tip was the digital, and the big part was print. And then when digital came around, people said “Oh, it’s okay, we’ll just give away the digital to save print.” Well, as time went on, digital became more important, and that pyramid got flipped on its point. People were giving away print just to save the digital. We’re at the same inflection point now in the evolution of publishing where it’s digital and AI. Or when I say digital and print, I mean print’s still there, but it’s such a minor part for most, at least in professional and scholarly. But, what’s going to end up happening is this industry is moving all to machine learning.
DG: Absolutely.
DM: In my opinion.
DG: I agree. It reminds me of back in the day when I was at Dow Jones and we had Dow Jones News Retrieval, which is now called Factiva, and it was charging what, a buck thirty or a buck sixty per minute, and it was moving at the blazing speed of 150 baud per minute, something exceptionally slow. But what they wouldn’t allow you to do is store the information so that you can re-search it. You couldn’t create your own sub database of that. But I think this is kind of like deja vu with what’s happening with AI because of newspapers back in the day when the Internet came around. The newspapers allow Google to mine all of their data for free. And then you think about saying to Google, pay us for this because they didn’t want to do the investment.
And Peter Kahn of the Wall Street Journal, the chairman CEO, it was 1998, he had invited me back to Dow Jones. He wanted me to come back, but I was at Elsevier. I was happy, but he was sharing with me how they did it right with the Wall Street Journal.com and how he felt that the local and regional newspapers would really hurt themselves. So, now, I hope that they’re smart enough to realize that our data is very, very important, and we need to get some fee for it. I understand that there are discussions with Google about Google giving them some money because Google got all the money from the hard work of all of the newspapers.
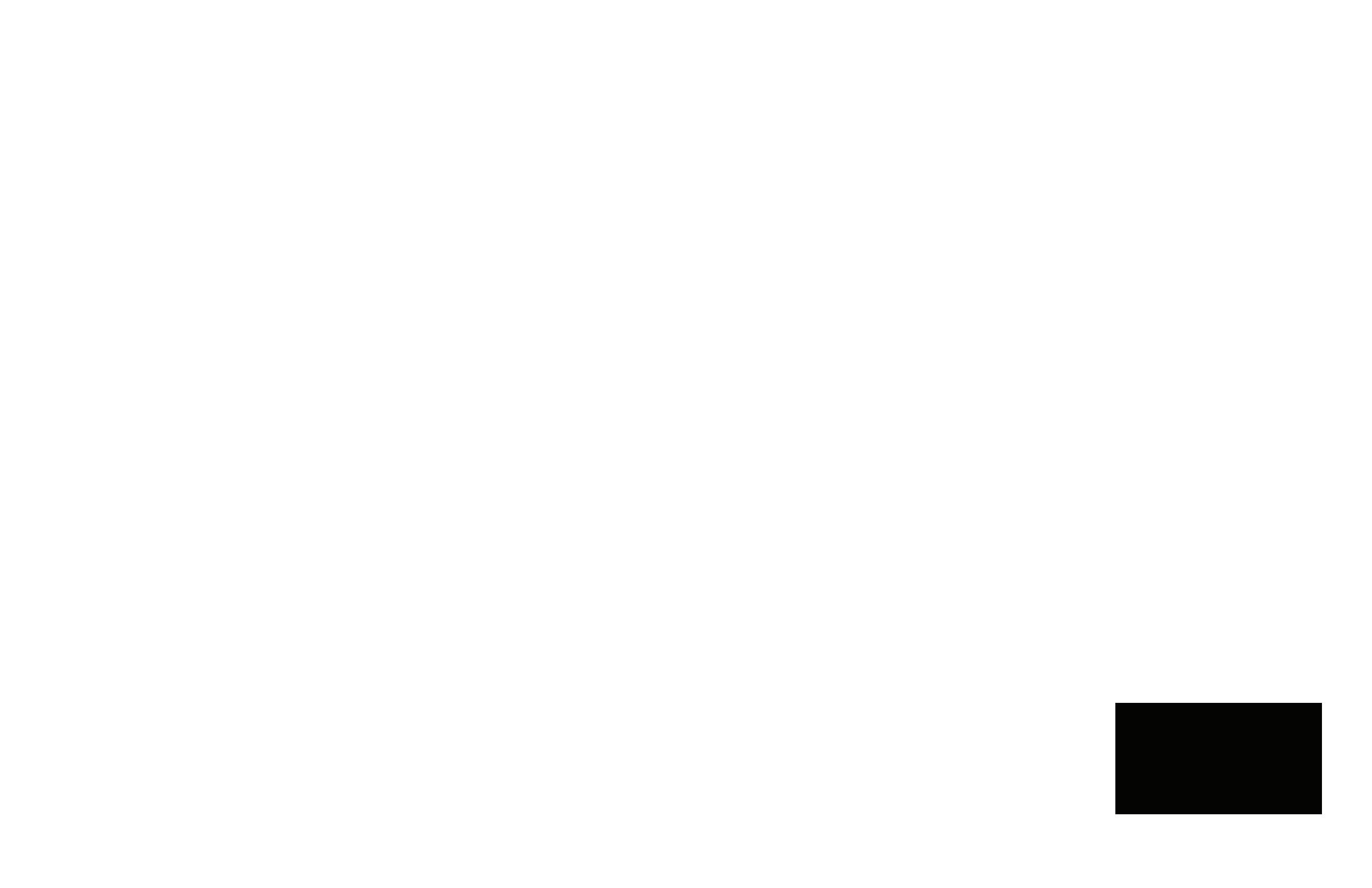
DM: Well, so you’ve undoubtedly heard that Google’s changed their terms of use; they basically are saying that we will mine any publicly available data on the Internet, and it
is free game for us to mine and own. And that’s caused some waves. As a matter of fact, I saw an announcement, I believe today, where Elon Musk adopted the same policy for his social media. So all his social media outlets, anything that’s publicly available, he claims he has the right to mine it for free. And I think that is problematic. I think that there’s certainly a public good that will come of it, but at the same time, there’s clearly going to be some violations to copyright and that’s an onion you can’t unpeel.
DG: Well, I guess Zoom has learned from that as well, because Zoom was taking all of our information from our individual video group and putting it into an AI machine.
DM: Yeah. It’s unbelievable. I mean, I’m so in awe of all the products that are being created with AI. It is unbelievable and those could not be accomplished without some of these activities that we’re discussing. But at the same time, there’s got to be a balance.
DG: That’s right.
DM: There’s risk and rewards. It’s interesting. So I created a facilitation course on licensing data for publishers, for them to create their AI policy for licensing their data to others.
DG: Oh, nice.
DM: So, it’s not a policy for internal use for what employees can do with generative AI and all. It’s about the policy for licensing your data out. There’s a lot of pitfalls and challenges that I discuss and I have senior management think about when they’re creating their policy. That’s not to say that they shouldn’t, and a lot of publishers have stopped doing that because they’re unsure or afraid, but the world’s changing and we need to keep pace.
DG: What you’re doing is so fascinating, David. Are you on course to do any speaking or panel discussions at any of the upcoming fall meetings?
DM: At the moment, I do not have plans to speak. I will be certainly at a number of them, but do not have plans at the moment. I normally facilitate a quarterly or biannual preview session for SSP, but other than that, at the moment, I don’t. But I’d love to. Anybody listening that needs somebody to talk about AI and licensing, I’d love to.
DG: Absolutely. That’s something I’ll pitch to someone and say, I got the perfect speaker for you.
DM: I appreciate it.
DG: You talked about your initial target market. Could you review that again in regards to your initial target market, as well as your plans for further expansion?
DM: I have a bunch of targets. In the short term, we’re looking to solve problems, especially drug discovery, because it’s quite huge. It’s quite a huge market. It’s quite an untapped market. As many people know, pharma has a hard time collaborating. Because of regulation, they can’t. But they have what’s called a treasure in the attic problem. They have tons of data that they have, and a lot of it they can’t use. So, failed clinical trials, for example. If there were a way to unleash some of those treasures, the world would benefit a lot. So, we’re looking at that. We’re looking at food science and crop safety. Solving big human problems is one of the things that we’re also looking at. The last one is public health policy. Problems that humanity needs to be solved. We’re not solving them. We see ourselves as the modern-day Levi Strauss. We’re standing at the mine entrance and giving everybody pick axes and shovels.
DG: There you go. Wow. Any final words you want to share with our audience about the Data Licensing Alliance and how they can get in touch with you? What is your website, et cetera, et cetera?
DM: We have two websites — our marketplace is open and ready for you at DLAdata.com and our marketing website is info.dladata.com. You can find us there or email me at <dave@ dladata.com>. Those are three ways you can certainly find me. And I would say as parting words, the thought of the day that I posted on LinkedIn was with AI, especially with data. Data, as they say, is the new oil. I mean, it’s really more than the new oil, it is the most prized commodity.
As Gordon Gecko said, information is the most important commodity I know of. With that, there’s new opportunities, new revenue opportunities. Certainly there’s challenges with that, but with those challenges, the opportunities can far outweigh it. The other side that I’ve seen is hiding your head in the sand like an ostrich, and that’s the current state of the dichotomy of publishing. And I urge everyone out there to consider experimenting a number of small bets out there. See what works. You have to push the envelope a little bit because the world is changing and the publishing industry is changing, and they say you’ll either be distinct or extinct.
DG: That’s right.
DM: With that, I’ll leave it be.
DG: I really appreciate it. Ladies and gentlemen, we’re here with my good friend, Mr. David Meyers, the CEO of Data Licensing Alliance and DMedia. David, thank you for coming on the program.
DM: Absolutely. Thank you for having me.
Transcribed from the radio interview Leadership with Darrell W. Gunter.