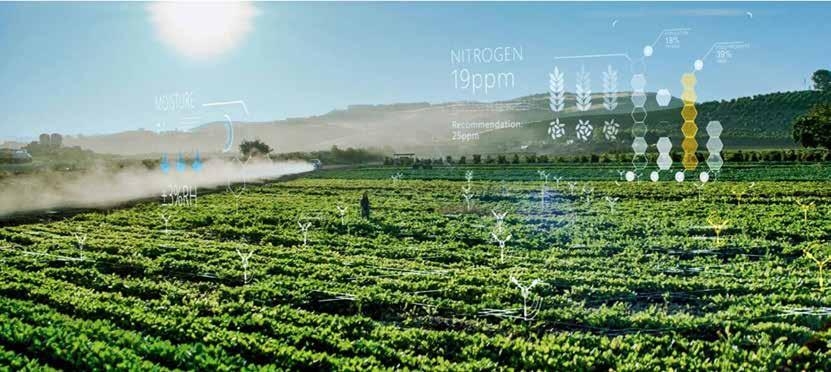
8 minute read
Lacuna Fund invests in African agricultural datasets for AI
LACUNA FUND BACKS SIX AFRICAN PROJECTS BUILDING AGRICULTURAL DATASETS FOR AI
Lacuna Fund, the world’s first collaborative fund aiming to correct gaps and biases in data for artificial intelligence (AI), in January announced the first round of funding to support datasets for more equitable and accessible AI for agriculture.
Lacuna Fund was co-founded in 2020 by The Rockefeller Foundation, Google.org, and Canada’s International Development Research Centre with a mandate to provide data scientists, researchers, and social entrepreneurs in low-and middle income contexts globally with the resources they need to produce labeled datasets that address urgent problems in their communities. Lacuna Fund is working to fill data gaps in three main domains, namely language, agriculture, and health.
The fund said its first round of funding in the agricultural AI for social good domain will support pioneering efforts solving urgent local problems in African countries while leading to a step change in machine learning’s potential around the world. A representative from Lacuna Fund said the six projects represent a total of $1.1-million in funding.
“The recipients of this first round of funding are unlocking the power of machine learning to alleviate food security challenges, spur economic opportunities, and give researchers, farmers, communities, and policymakers access to superior agricultural datasets. We are proud to support their work,” the fund said on its website.
Lacuna Fund said it had received over 100 applications from , or in partnership with organisations across Africa. Lacuna Fund’s Technical Advisory Panel (TAP) selected the six recipients using criteria based on the fund’s principles, namely: quality, transformational impact, equity, participatory approach, ethics, efficiency, feasibility, accessibility, and sustainability.
“It’s clear that the demand for high-quality and representative labeled training data is high, and the expertise and groundwork needed to collect and deploy it is growing by the day,” said Lacuna Fund. The fund said all datasets produced will be locally developed and owned and will also be openly accessible to the international data community.
Lacuna Fund explained that it aims to match project teams with in-kind capacity building, and technical support as needed and available.
Mutembesa Daniel, research scientist at the Artificial Intelligence Research lab at Makerere University and a member of the Technical Advisory Panel, said he was very proud of the work being done by the cohort. “I look forward to seeing the glass ceiling between francophone and anglophone parts of Africa being broken, to seeing data scientists from these different parts of Africa starting to work with each other across regions,” he added.
Another member of the Technical Advisory Panel, AllSightsAfrica executive chairman Youssef Travaly said Lacuna Fund had managed to reach a consensus on a well-balanced portfolio, both in terms of geography and type. “This is a great step forward toward more inclusive AI,” said Travaly.
The six projects selected for funding address a range of agricultural needs, including livestock and fisheries management, as well as crop identification, yield estimation, and disease detection in crops that shore up food security efforts in the region—namely cassava, maize, beans, bananas, pearl millet, and cocoa. They are:
Helmets Labeling Crops
This project is a collaborative initiative between the University of Maryland/NASA Harvest Africa Program, The Regional Universities Forum for Capacity Building in Agriculture (RUFORUM), Center for Earth Observation and Citizen Science at the International Institute for Applied Systems Analysis, The Regional Center for Mapping of Resource for Development (RCMRD), The Eastern Africa Grain Council (EAGC), Lutheran World Relief Mali, International Maize and Wheat Improvement Center (CIMMYT), and Radiant Earth Foundation.
The project will create unprecedented ML-ready labeled datasets for crop type, crop pest and disease, and market prices in the main food production regions in Kenya, Mali, Rwanda, Tanzania, and Uganda.
Collectively, the team will use novel and innovative approaches that include rapid point data collection with cameras mounted on the hoods of vehicles—“helmets”—combined with crowdsourcing to create point and polygon labels. By partnering with local universities -- including Makerere Univesity Uganda, Sokoine University Tanzania, as well as others that are part of the Regional Universities Forum for Capacity Building in Agriculture (RUFORUM) --, this project will create opportunities for training future African researchers to use remote sensing and machine learning.
Catherine Lilian Nakalembe, a Ugandan remote sensing scientist and assistant research professor at the University of Maryland, as well as NASA Harvest Africa programme director described Helmets Labeling Crops as a one of a kind opportunity to address a critical gap, to learn and develop a cost-effective scalable approach to labeled data needed to improve the basis of agricultural monitoring.
“We’ll also get a chance to train and work with students across Africa which is an exciting opportunity to encourage students into remote sensing, data science, and machine learning,” added Nakalembe.
IoT Water Quality Monitoring System for Freshwater Aquaponics Fish Ponds
The University of Nigeria Nsukka will build a remotely monitored and controlled Internet of Things (IoT) water quality management system for conventional ponds and the aquaponics fish pond systems to generate labeled datasets, and partner with local farmers to test the system in the field. These datasets will enable machine learning researchers to build models for predicting fish yield in terms of weight gain, water quality parameters, and feed consumption.
Collins Udanor, deputy director of the Education Innovation Centre at the University of Nigeria Nsukka, said he was hopeful the project is going to open a open a vista of opportunities to local fish farmers as it will throw an avalanche of light into what happens inside the fish pond. “This will indeed explain many things to the farmers and improve yields, as well as make available local datasets for the machine learning community,” he added.
Machine Learning Datasets for Crop Pest and Disease Diagnosis based on Crop Imagery and Spectrometry Data
This project will produce quality open and accessible image and spectrometry datasets from Uganda, Tanzania, Namibia, and Ghana for several crops that contribute to food security in Sub-Saharan Africa, including cassava, maize, beans, bananas, pearl millet, and cocoa.
The team -composed of data scientists and researchers from Makerere University, The Nelson-Mandela African Institution of Science and Technology, Namibia University of Science and Technology, and the karaAgro AI Foundation – expect the image and spectral datasets will be used for early disease identification, disease diagnosis, and modelling disease spread, which will ultimately help in breeding resistant crop varieties.
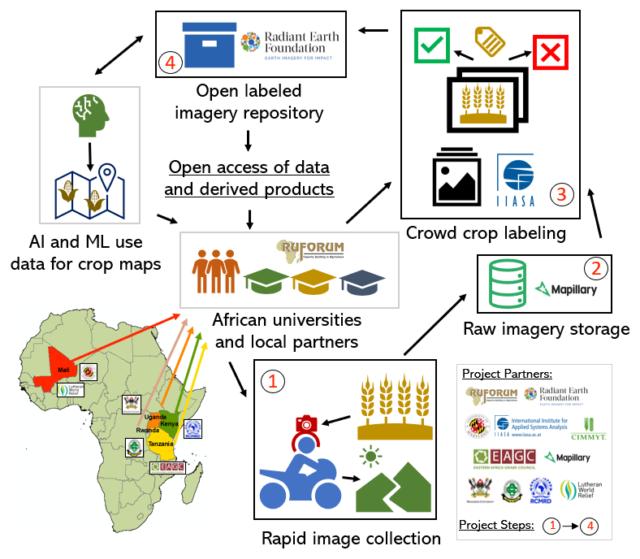
Makerere University lecturer Joyce Nakatumba-Nabende explained that the project is a unique collaboration across four countries in sub-Saharan Africa with the aim of delivering crop imagery and spectrometry datasets for six important food security crops. “The datasets are necessary for building machine learning models for early disease diagnosis and will be relevant for not only the AI and machine learning communities but also for the smallholder farmers and agricultural experts,” she added.
Decision Support Tool for Community-led Land Use Plans
This project, which is led by The Nelson-Mandela African Institution of Science and Technology, University of Glasgow, University of Hohenheim, and NOTTECH Company Limited, will apply participatory methods and telemetry data to generate labeled datasets of landscape features. These labeled datasets will then be used to develop a community-led land use plan in Tanzania to support the management of livestock resources and reduce conflicts with crop cultivation.
Locational Offset Correction
The utility of existing crop-cut yield datasets is often compromised due to location inaccuracies arising from the data collection process. To address this, the Locational Offset Correction project will create a method for correcting location inaccuracies using available satellite data. This method will then be used to create a new, clean version of a crop-cut yield dataset for maize crops in East Africa, which will be released along with a tool to allow others to re-create the process using additional datasets. This research will be conducted by a team from Zindi and the Big Data Platform of the CGIAR.
Zindi CEO and co-founder Celina Lee said the data science competition platform will call on data science talent from across Africa and around the world to crowd-source a machine learning solution for correcting location errors which are a common problem in agriculture data sets. “ This solution will allow us to correct and publicly release one of the most expansive crop cut yield estimation datasets for maize in Eastern Africa,” she added.
Eyes on the Ground: Providing Quality Model Training Data through Smartphones
This project will see the team from ACRE Africa and the International Food Policy Research Institute (IFPRI) use smartphones to create a unique dataset of geo-referenced crop images along with labels on input use, crop management, phenology, crop damage, and yields.
Images will be collected in 11 counties in Kenya. Funding will be used to support data collection, standardisation of methods for submitting images, and curation of datasets so they meet best practices for ground reference data collection and cataloguing, while safeguarding ethics considerations.
Lilly Waithaka, Agri-climate Data Analyst at ACRE Africa, said the project is a novel concept that aims to provide smallholder farmers with risk mitigation and adaptation strategies through satellites and smartphones to ensure that they invest in high productivity agriculture investments.
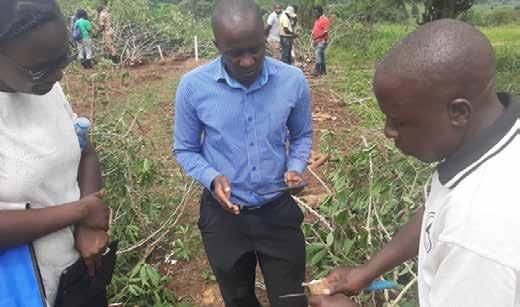
“The ground pictures not only provide ACRE Africa with Eyes on the Ground to fine-tune insurance products/models and minimise basis risk, but also enable us to observe management practices that promote the adoption of productivity-enhancing yet resilient technologies through bundling with stress-tolerant seeds and remote advisories. To bridge the gap between insurance products, resilient technologies and smallholder farmers we leverage on the Village Extension Service Providers (VESPs) model, a high touch and robust route to market model to help create awareness, capacitate and distribute our products. This model ultimately intends to create entrepreneurial opportunities,” added Waithaka.
“We co-founded Lacuna Fund recognising that AI has the potential to increase food and financial security for vulnerable populations around the world but that the only way to achieve this is through community-driven innovation,” said Evan Tachovsky, director and lead data scientist at The Rockefeller Foundation. Tachovsky added that Lacuna Fund exists to support the AI community across Africa as they build tools for farmers, extension workers, and policy makers. “This exceptional cohort of projects is a testament to their ingenuity, expertise, and commitment to impact and we look forward to seeing them build and open source these critical datasets,” he said.
“The goal is open datasets. But this is bigger than just creating the datasets,” said Daphne Luong, director of Engineering at Google AI Research. “We want to create innovative, scalable and replicable data protocols, so they can be applied to different data domains as well as other geographical regions. Eventually we hope that more representative and accessible data will allow machine learning to better serve communities worldwide.” ai