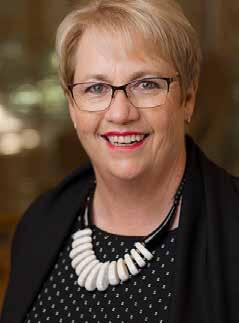
6 minute read
ROBOTIC PROCESS AUTOMATION (RPA) is the Path to AI
Lenore Keerigan UIPath’s Country Director for South Africa, talks exclusively to Synapse Magazine about the path to AI using RPA
WHAT ARE some of the main things that African or African-based businesses looking to introduce artificial intelligence (AI) should consider?
We are seeing the biggest implementation of AI in business processes related to Customer Experience e.g. processing of claims, complaints, call centres, applications, etc. Improved customer experience is one of the highest focus areas for CEOs in a competitive and economically constrained market. There is plenty of hype around AI, but a word of warning – many current AI initiatives work in isolation and have shown limited success. So, business leaders are still wondering where to start. This is the beauty of Robotic Process Automation (RPA) - companies can leverage the automation platforms whose software robots are already using AI and Machine Learning algorithms, making RPA the enabler for organisations to introduce AI into the standard business processes. RPA is the path to AI.
Which industries / sectors stand to benefit the most from introducing RPA or AI to their business processes and which ones should definitely be thinking about implementing this technology?
While the banking and financial services have pioneered the field of automation, at UiPath we have seen deployments in every industry, from manufacturing to retail, to aviation and the hotel industry, to the legal profession and the public sector. Functions like human resources, finance and accounting, contact centres, accounts payable, claims processing which are common across industries abound in repetitive, rules-based tasks that can and should be automated as a means to not only improve customer service, but also boost employee engagement and satisfaction. UiPath is promoting an Automation First mindset to organisations of all sizes, meaning that we offer them an end-to-end hyper-automation platform to practically eradicate the mundane and boring tasks from the job descriptions of the human employees allowing them to get involved in the more valuable and creative aspects of their jobs. Let the robots count the beans, and the humans analyse the data brought forth by the robots.
What could go wrong when AI is not implemented well, can you give some real-world examples?
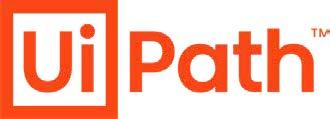
In the area of Machine Learning (ML) there have been some high-profile cases of undesirable outcomes. The first was when a leading software company let a chatbot out ‘in the wild’ and left it to learn based on conversations people had with the robot. It took less than 24 hours for users on Twitter to corrupt the robot resulting in it spewing out offensive content. Another ML-related high-profile case was a CV screening algorithm that a leading Cloud Platform company implemented being scrapped immediately after it showed a bias against women. Both these examples show that you need to control the data that is being fed to the algorithms for their training. If the training data can be influenced by outsiders, and they have malicious intentions, they can affect the behaviour. In the CV screening case, the question comes up around how fair the training set is, and if it contains any bias, or is disproportionate. Care needs to be taken when selecting which factors you want the ML algorithm to be able to use when being trained, and later when making its predictions. The old saying of ‘garbage in, garbage out’ applies very strongly to the Machine Learning world.
But there’s also another way to look at what can go wrong, not specifically because of the model, but on how people perceive it. One is that you cannot explain exactly how it’s working, what influences what. So, for example, if you’re using a deep learning algorithm to decide if a customer is suitable or not for a loan, if the answer is no, you cannot explain what influenced that answer. There is no formula saying 37% of the result is influenced by salary, 15% by the area where you live, 22% by the profession, etc.
The second is with the case of instant answers which are considered amazing most of the times except when they’re negative. For example an insurance company using AI had to delay the response with 24 hours if it was no, because 90% of the time they received complaints when after a few minutes of submission the customer received a response saying the insurance won’t cover that. With a 24 hour delay, the complaints dropped to 20-30%.
What would you say are the top three things to avoid or not do when implementing AI in a business? In other words, what are some of the common mistakes that businesses often make in implementing the technology?
Set expectations too high – The term ‘Artificial Intelligence’ sets people’s expectations very high and they expect autonomous, sentient beings able to perform anything better than humans. We are not close to that in reality. It is key that expectations are managed, the use case is explained properly to all the teams involved, and specific metrics and KPIs are set up to ensure we understand what is regarded as success, and how we can measure it.
Too big, too soon – Often projects try and automate too much of a process, or try to go too broad with the scope of their AI solution from the outset. It is usually best practice to always start with a smaller subset of a process or area and demonstrate a live example that brings in value before expanding.
Not plan for change management – like any technology solution there needs to be planning for changes. In the RPA world changes to underlying system can lead to automations breaking and needing upgrades. This needs to be catered for in the setup of the operating model. In the Machine Learning world, the quality and performance of a model can deteriorate over time and needs to be measured. There needs to be plans around how and when models will be retrained if their performance deteriorates beyond a certain threshold.
How is the Return On Investment (ROI) for AI or RPA measured? How should companies go about this and what should they look out for?
Some of the benefits are very tangible and quantifiable, such as human hours saved by automation. It is important to capture the metrics around the process before the automation has been implemented, to allow a post-change measurement and thus the benefit calculation to be completed. This sort of calculation is something companies are familiar with from the inception of software solutions. UiPath has tools in the area of task and process mining that can help with all of this.
It’s no secret that when given a repetitive task such as invoice processing, the software robot will pay the same attention to the 500th invoice while a human employee might have errors. So, accuracy is definitely a key benefit of automation. Compliance to standards and governance models is another area where significant benefits are realised.
More difficult benefits to measure and quantify are things like increased customer satisfaction as customers, for example, are able to get information or technical support or counseling faster.
A key area in which RPA is having a tangible impact, that is not always easy to measure, is employee happiness. We are finding those companies who have embraced the mantra of ‘A Robot for Every Person’ and are freeing up their employees from mundane tasks are making them happier in the workplace. ai “