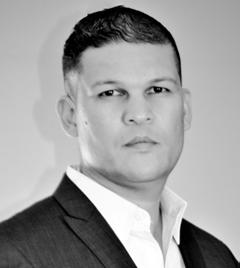
8 minute read
Why Eliminating Bias is Key to AI Success
By Rudeon Snell, Global Senior Director: Industries & Customer Advisory at SAP
2020 is forcing us to confront some hard truths about the world we live in. The Covid-19 pandemic has cast a sobering spotlight on some of the more questionable facets of our way of life and is making it clear, that continuing on this unsustainable path we have been on will inevitably lead to a very bleak future for our species.
ONE SUCH truth is symbolised by the global #BlackLivesMatter movement, which was sparked in the US, but has spread to cities all around the globe. This movement has once again highlighted the embedded biases in our interconnected social fabric, forcing us all, to not only rethink, but completely reevaluate long standing notions of morality, fairness and ethics.
It is worth taking pause, just for a moment, to consider whether the exponential technological progress we have been experiencing, and which does aim to create a better future for humanity, is not also amplifying some of the very same challenges we are trying to overcome as a global society.
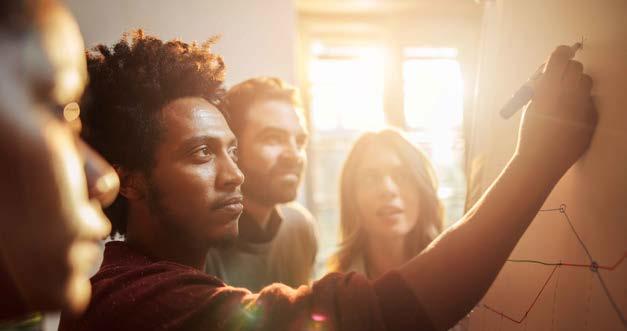
As we strive to meet the unmet and unarticulated needs of customers, we continuously look towards technology to fulfil the promise of ushering in a new era of human advancement. We see leading companies globally investing heavily in technologies such as Cloud Computing, Internet of Things, Advanced Analytics, Edge Computing, Virtual and Augmented reality, 3-D printing and of course Artificial Intelligence. And it is AI, which many experts tout as one of the most transformational technologies of our time, perhaps even more transformational than electricity or fire, in terms of sheer impact on humanity.
Global use of AI has ballooned by 270% over the past five years, with estimated revenues of more than $118-billion by 2025. AI powered technology solutions have become so pervasive, a recent Gallup poll found that nearly 9 in 10 Americans use AI based solutions in their everyday lives. Phones, apps, search engines, social media, email, cars and even appliances in our homes are all powered by AI infused technologies today, with this trend set to accelerate in future.
And yet, a dark side of AI is surfacing with alarming frequency as AI engrains itself in our daily lives. Questions that
are increasingly being posed and must be addressed, concern themselves with whether AI algorithms are indeed perpetuating various forms of bias to the detriment of under-represented communities and minorities? To what extent do AI-imbued solutions discriminate against exposed classes of our society due to embedded bias?
Bias in the machine
There are ample examples of algorithms displaying forms of bias.
In 2018, reports emerged of Gmail’s predictive text tool automatically assigning “investor” as “male”. When a research scientist typed “I am meeting an investor next week”, Gmail’s Smart Compose tool thought they would want to follow up with the question “Do you want to meet him?”.
That same year, Amazon had to decommission their AIpowered talent acquisition system after it appeared to favour male candidates. The software seemingly downgraded female candidates if their resumes included phrases with the word “women’s” in them, for example “women’s hockey club captain”.
Many of the large tech firms battle with diversity, with men much better represented than women in most major tech companies. Having gender bias embedded in algorithms designed to support the hiring process presents a significant risk to efforts at achieving greater diversity: Mercer’s Global Talent Trends report for 2019 highlights that 88% of companies globally, already use AI powered solutions in some way for HR, with 100% of Chinese firms and 83% of US employers relying on some form of AI for HR.
For Amazon, it has forced a rethink of how they recruit globally, no small feat for a company that employs more than 575 000 workers.
Persecuted by an algorithm
Errant algorithms can be responsible for greater harm than just a few missed employment opportunities.
In June 2020, the New York Times reported on an African American man wrongfully arrested for a crime he didn’t commit after a flawed match from a facial recognition algorithm. Experts at the time believed it was the first such case to be tested in US courts. I’d wager, it won’t be the last.
Recent studies by MIT found that facial recognition software, used by US police departments for decades, works relatively well on certain demographics, but is far less effective on other demographics, mainly due to a lack of diversity in the data that the developers used to train these algorithms.
Microsoft and Amazon have halted sales of their facial there are at least 21 different definitions of fairness, ranging recognition software until there is a better understanding across notions of individual fairness, group fairness, process and mitigation of their impact, on especially vulnerable or fairness, diversity and representation. Our individual and minority communities. IBM has even gone as far to halt offering, collective life experiences will largely determine what type of developing or researching facial recognition technology. fairness we favour, but the problem this creates is that one
But how does this happen in the first place? person’s fairness could be another’s discrimination. For example, what is the fair demographic representation How bias enters our algorithms of “Global CEO” when you enter that into an image search bar? McKinsey supports the view, that it is actually the underlying Is it a 50/50 split between male and female? Is it an equal split data that is the culprit in perpetuating bias, more so than the between White, Black, Hispanic and Asian CEOs? Or should its actual algorithm itself. In a 2019 paper, the firm argued that results simply be proportional to real-world data: if there are algorithms trained on data containing human decisions have a only four black CEOs of Fortune 500 companies, should only natural tendency toward bias. For example, using news articles 0.8% of search results be of black CEOs? for natural language processing could instil the common gender There is arguably a need for greater diversity in the stereotypes we find in society simply due to the nature of the development rooms where AI algorithms are created. A cursory language used. glance at the demographics of the big tech firms shows a
Many of the early algorithms were also trained using web disproportionate gender and demographic bias. More must data, which is often rife with our raw, unfiltered thoughts and be done to accelerate the synthesis of diverse and inclusive prejudices. A person commenting anonymously on an online perspectives in the AI creation process, so that AI algorithms forum arguably has more freedom to display prejudices without and the data they are trained on embody a broad range of much consequence. It’s not socially acceptable to admit to perspectives, allowing them to drive more optimal outcomes for being racist or sexist, but anonymity offered by the web means all those represented in society. many of these views proliferate mainstream and niche websites. What can we do to mitigate bias in the AI solutions we Any algorithm trained on this data is likely to assimilate the increasingly use to make potentially life changing decisions, such embedded biases. as arresting someone or hiring someone? Greater awareness
As Princeton researcher Olga Russakovsky observes: of bias can help developers see the context in which AI could “Debiasing humans is a lot harder than debiasing AI systems.” amplify embedded bias and guide them to put corrective
One example of this is Microsoft’s well-intentioned measures in place. Testing processes should also be developed experiment with their chatbot, Tay. Tay was plugged directly with bias in mind: AI creators should deliberately create into Twitter, where users across the world could interact with processes and practices that test for and correct bias. Design it. Users of the popular social media platform promptly got should always keep bias in mind. to work teaching the bot racist, misogynistic phrases. Within It’s probably impossible to have a completely unbiased one day, the bot started praising Hitler, forcing Microsoft society, but having more diverse voices and greater awareness researchers to pull the experiment. of the various forms of embedded bias within our societies can
The lesson: algorithms learn precisely what you teach them, help AI creators build greater fairness into their algorithms. consciously or unconsciously. And because algorithms learn Diversity is important and can lead to more meaningful and from data, data matters. helpful discussions around potential bias in human decisions.
Web data is also not fairly representative of society at large: Finally, AI firms need to make investments into bias issues with access to connectivity and the cost of smartphones research, partnering with other disciplines far beyond and data could exclude many - especially minorities - from technology such as psychology or philosophy, and share the engaging with online content. This means that data collected learnings broadly to ensure all the algorithms we use can from the web is naturally skewed to the demographics that operate alongside humans in a responsible and helpful manner. make most use of websites and social media. Fixing bias is not something we can do overnight. It’s a process, just like solving discrimination in any other part of Combating bias in our AI solutions society. However, with greater awareness and a purposeful One of the biggest challenges for the creators of AI algorithms approach to combating bias, AI algorithm creators have a hugely trying to eliminate bias, besides merely identifying it, is knowing influential role to play in helping establish a more fair and just what should replace it. If fairness is the opposite of bias, how do society for everyone. you define fairness? This could be one silver lining in the ominous cloud that is
Princeton computer scientist Arvind Narayanan argues 2020. ai