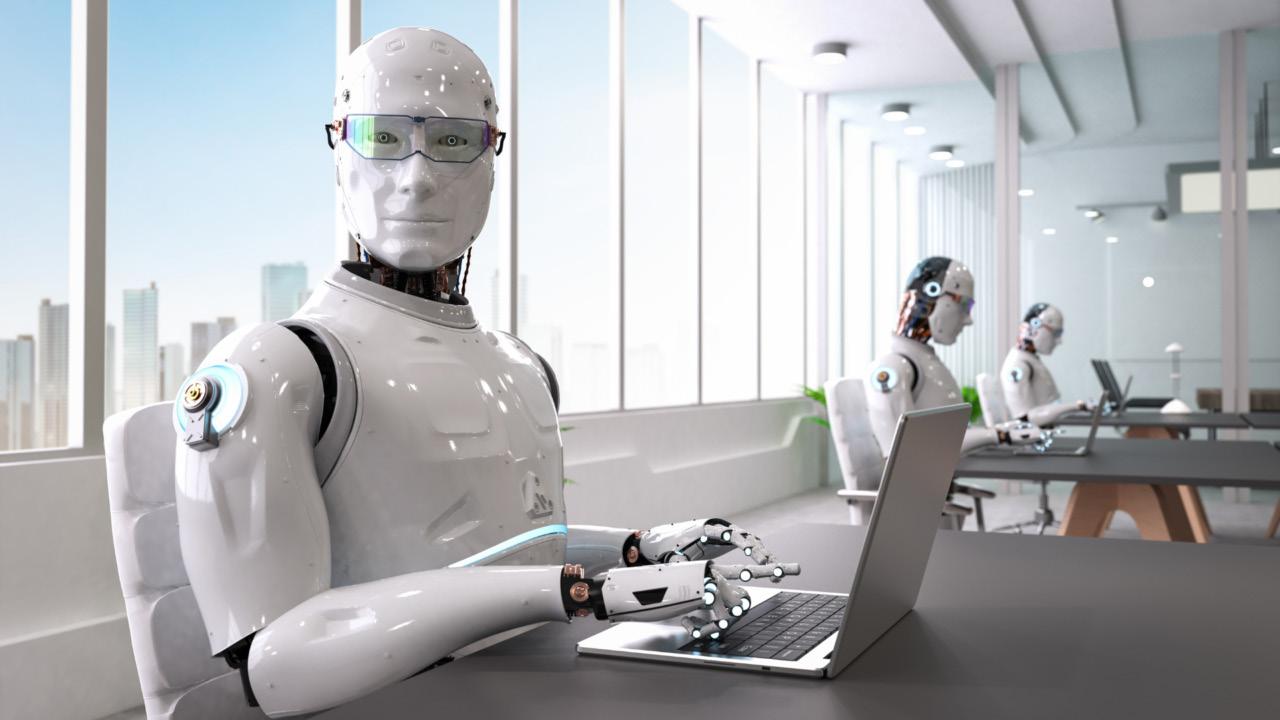
5 minute read
MODEL RISK MANAGEMENT FOR AI SYSTEMS
Model risk management is crucial to effectively using AI in various domains. As you know, AI has advanced rapidly and integrated into different areas of our lives, including healthcare, finance, and transportation. Even so, AI systems’ reliance on complex models makes them vulnerable to errors and biases, which could lead to significant risks for the individuals and organisations that use — or are impacted by — them.
What is model risk management?
Model risk management is the process of identifying, assessing, and mitigating risks associated with using complex models.
In the context of AI, managing risk involves evaluating the model’s accuracy, robustness, and potential biases. It also includes establishing effective governance frameworks, internal controls, and validation processes to ensure the models are reliable and safe.
Why is model risk management crucial for AI systems?
It helps to ensure that the models you’re using are accurate, reliable, and free from biases. The reality is that AI systems are only as good as the models they use. So, if those models are flawed, the system’s results can be unreliable, inaccurate, or even dangerous.
Let’s explore accuracy, bias, robustness, and compliance a bit more:
- Accuracy. AI systems rely on models to make predictions and decisions. If these models are inaccurate, the system may generate incorrect or misleading results.
- Bias. AI models can be biased in various ways, such as gender or racial bias, leading to unfair or discriminatory outcomes. Model risk management can help identify and mitigate biases like these.
- Robustness. AI systems can be vulnerable to attacks or unexpected data patterns. Model risk management can help ensure that models are robust and can withstand such scenarios.
- Compliance. Many industries and regulatory bodies have specific requirements for model risk management. Compliance with these requirements is essential to avoid legal and reputational risks.
Examples of model risk management applied to AI
Healthcare
The healthcare industry develops AI models to predict patient outcomes, diagnose diseases, and create personalised treatment plans. However, these models rely on large datasets, which probably contain biases. These biases, in turn, often lead to incorrect predictions. By implementing effective model risk management practices, healthcare organisations can ensure that their AI models are accurate, reliable, and safe.
Finance
The finance work uses AI models to create trading algorithms, risk models, and fraud detection systems. However, these models could be vulnerable to errors or biases, leading to significant financial losses. By establishing robust governance frameworks and validation processes, finance companies can mitigate these risks and ensure the accuracy and reliability of their AI models.
Transportation
An example of AI applied in the transportation sector is autonomous vehicles. These vehicles rely on complex machine learning models to make decisions on the road, such as when to accelerate, brake, or change lanes. To manage the risks associated with these models, transportation companies must thoroughly test and validate models before deploying them on the road. They must also monitor their ongoing performance in line with their approach to managing risk.
7 practical steps to implementing model risk management
It requires a comprehensive approach that involves various stakeholders, including data scientists, business leaders, and risk management professionals.
Here are some practical steps you can take to implement model risk management for AI systems:
1. Establish a clear governance structure. The first step is to establish a governance structure that outlines the roles and responsibilities of different stakeholders in the model risk management process. This includes identifying the individuals responsible for model development, testing, and validation and those responsible for monitoring and reporting on model performance.
2. Develop a robust model development process. The model development process should be robust and follow best practices, including data preprocessing, model selection, and evaluation.
In addition, this process should be documented and include appropriate checks and balances to ensure that models are developed in a controlled and transparent manner.
3. Conduct thorough model validation
Once you’ve deployed a mode, it should undergo thorough validation to ensure that it is accurate, reliable, and meets the business’s needs. This process includes:
- testing the model on different datasets,
- assessing its performance against various metrics, and
- evaluating its sensitivity to changes in input data and assumptions.
4. Monitor model performance. After deployment, you should monitor models to detect any issues or changes in performance. Further, this process includes regular monitoring of model output and monitoring changes in input data or assumptions that may impact model performance.
5. Implement a model governance framework. A model governance framework is a set of policies and procedures that govern the entire model lifecycle, from development to retirement. This framework should include controls for model development, validation, deployment, monitoring, and retirement, as well as mechanisms for escalation and resolution of issues.
6. Provide regular training and education. To ensure that all stakeholders understand the model risk management process and their roles and responsibilities, you should provide regular training and education. This approach includes training on best practices for model development, validation and monitoring, and the business context in which you use the models.
7. Stay up to date on regulatory requirements. Finally, it would be best to stay current on regulatory requirements for model risk management, including data privacy, security, and ethical considerations. This includes staying informed of changes in regulations and industry standards and participating in industry working groups and forums.
Actions you can take next
Move towards trustworthy AI by consulting with our specialists or attending our public or private workshops.
Determine how AI impacts your organisation by asking us for an AI risk assessment.
Collect and acquire big data you use to train AI lawfully by asking us to draft your big data contracts.
Protect your commercial interests by asking us to draft your AI contracts.
Stay updated with the latest AI law news by subscribing to our newsletter
Discover more about AI by reading our AI law page