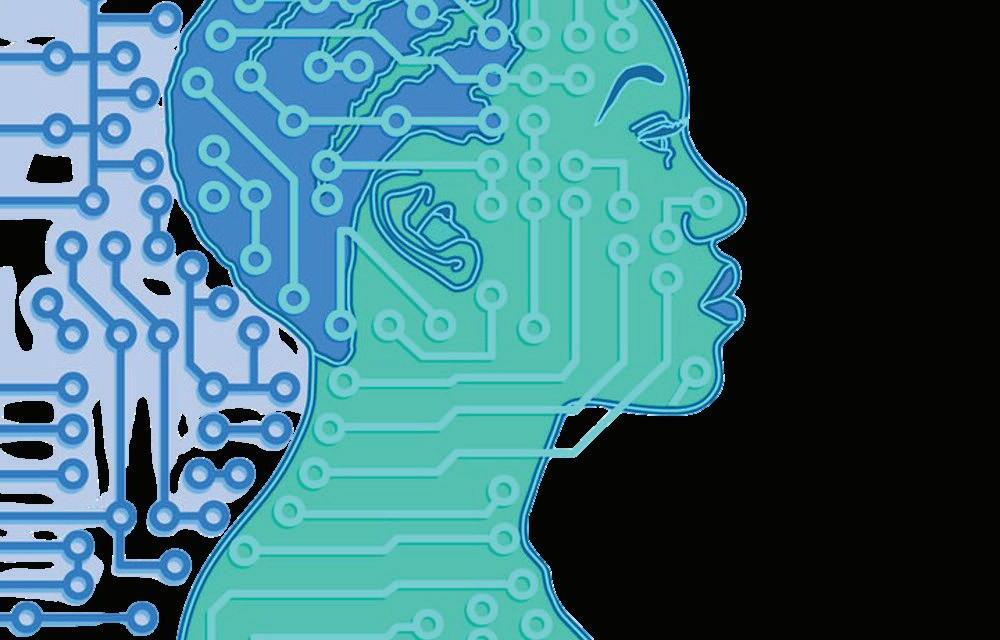
6 minute read
MAKE MONEY IN REAL-TIME – Using behavioral social science and machine learning
“63% of companies plan to increase or maintain AI and machine learning spending in 2023”
When asked to cite the top three drivers behind these budget changes, leading factors included changes in business strategy, cost pressures, and inflation.
But have the costs been alleviated yet?
According to Harvard Business Review, most ML projects fail to deliver value. There are many reasons for this, but we believe that there are 3 fundamental issues that can lead to high failure rates:
Deployment Complexity can skyrocket costs.
Putting accurate and successful machine learning projects into production is complex. The tools to create predictions, build recommenders and configure experiments are costly, complicated and require expertise. Moving to a low-code, specialised deployment environment reduces these costs immediately, and gives business users more involvement throughout the lifecycle of the project.
Current predictions don’t account for everchanging human behaviour.
Although machine processes are largely designed to target, speak to, and engage with humans; most of the patterns and correlations it identifies don’t tell you much about behavior. Ai needs to advance and factor in human behaviour and context. The extra layer of meaning this adds to the data allows companies to communicate more effectively, to offer more relevant recommendations, and understand what customers truly want.
Reliance on historical data doesn’t provide real-time insight.
58% of successful machine learning projects take more than several months to deploy. Delays happen, which means that at the point of deployment, the data being analysed and predicted on is from the past. As stated, this can’t account for a customers changing behaviour, and crucially, it doesn’t factor in their most recent transactions. Can a deployment based on historical data really predict what customers are going to do in the future? Consider the data from the last 3 years when the Covid pandemic influenced an un-referenceable series of data points. Can we reliably use this data to predict what customers want this year, or the next?
Our low-code platform increases your speed to market by reducing complexity costs
The platform has a no-code environment Workbench, as well as low-code environment Notebooks. Designed to be accessible to everyone in a business – from the Businessperson who needs to drive success. To the Data Scientists who require uncomplicated tools to untangle machine learning complexities. As well as Technologists who require flexibility in the form of code-based customizations that are easy to implement and integrate. ecosystem.Ai’s multi-faceted technology provides a single platform that works within your own environment, or in the cloud. It facilitates open collaboration for all team members to work on the same project, and keep track of all deployments.
Engage with people more authentically using behavioural analysis
Generic data science answers the “how” through analysis of data points and trends. But ecosystem.Ai use Computational Social Science to take this a step further by asking the “why” and “what next”. Uncovering this deeper level of understanding reveals a multitude of untapped data patterns to identify real human behavioral nuances. Every digital interaction leaves a transactional trail that gives insight into the behaviours of a human. Analysing this trail as it plays out in real-time, helps you make better predictions about that individual. Companies can structure offers and engagements based on the rhythm and actions of a customer, while continuously testing and experimenting with novel offers to spark interest.
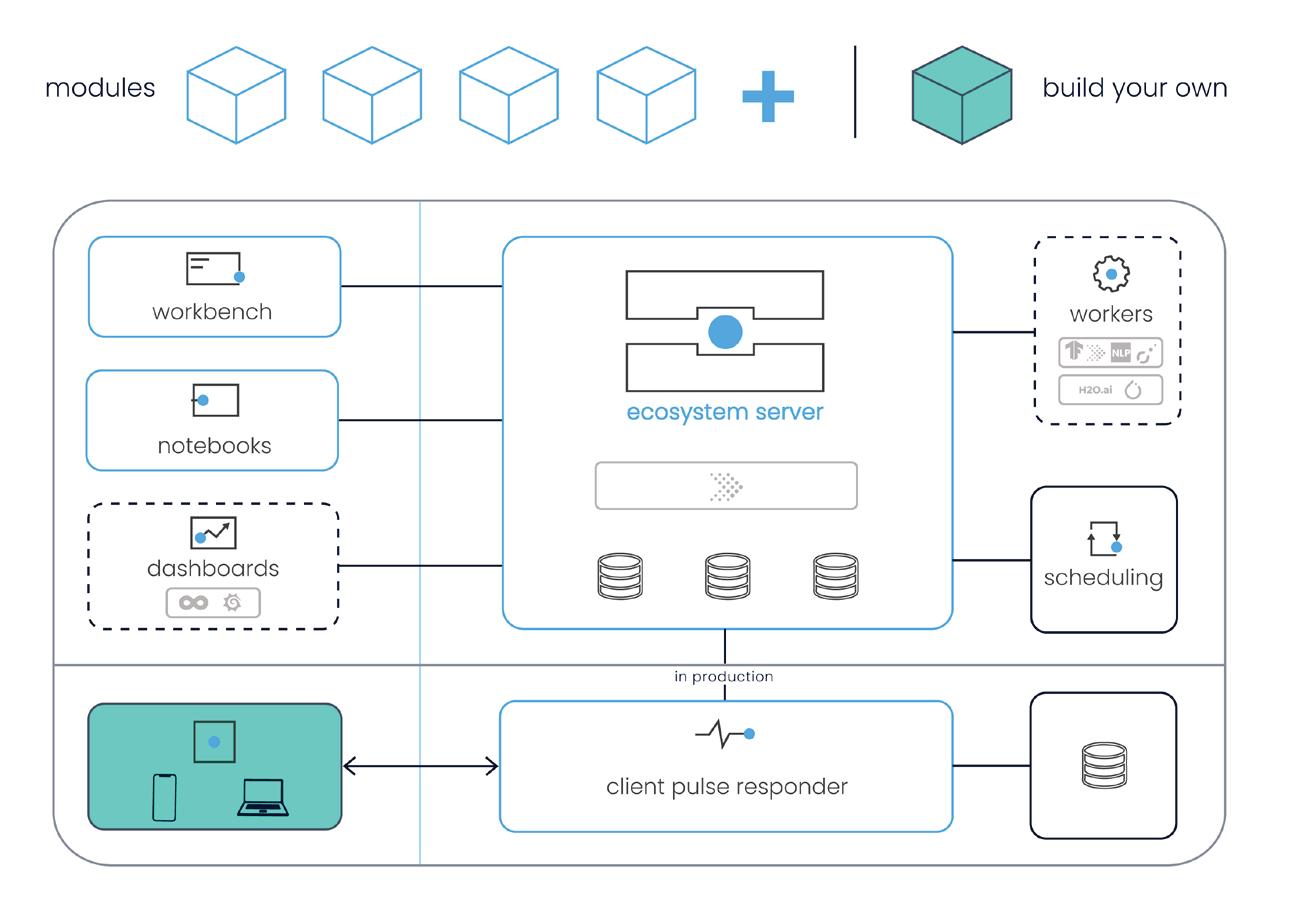
This is ecosystem.Ai’s area of expertise. Our revolutionary platform integrates Computational Social Science to bridge the gap between machine and human intelligence. Providing analytics from a human and behavioural context, we help companies build behavioral models in their own environment and get their real-time predictions into market faster. Offering the tools to make money by learning while in production and save money by avoiding complicated implementation costs.
Capitalize on emerging trends and ritualisticpatterns with real-time analysis
Companies need to evolve. There must be frequent continuous cycles of experimentation to keep pace with changing behaviors. Our real-time engine makes this possible by giving your machine-learning models more accuracy and relevance, right now. Allowing you to change configurations, tweak engagements, and tailor offers and messages as customers interact with you. Real-time means no more waiting for batch processes to analyze performance.You can let your customers reactions guide decisions as you view their real-time interactions. This is a game-changer as it takes the element of guesswork out. With predictions there is benefit to using historical data. But in realtime you don’t necessarily need data to get started. Our experimentation process means you can start building relevant predictions with no data.
Incorporating generative ai to help you engage like never before
Generative Ai has taken this year by storm, becoming available for everyone to use. Gen AI has a myriad of applications and 70% of Gen Z report using the technology, 52% of them trust the technology to help them make informed decisions. But very often, results generated can be misleading or downright inaccurate. This is what we call the hallucination.
Our Fact Injection functionality helps to reduce the hallucination by allowing you point to a source. The problem of minimising the hallucination common in generative AI models is tackled excellently by ecosystem. Ai, ensuring the process is seamless and uncomplicated.
With Fact Injection and generative AI, you can write up-to-date engagement messages that change as your customers change. In the ecosystem.Ai Workbench, you can save the messages to a workspace then add directly to your experimentation pipeline. Identify tangible evidence of offer takeup in a personalized customer basis, and write according to your findings. Learn from each customer which option suits them best, and which message style they are most likely to engage with.
Using generative AI in the workbench
Continuous will catapult your business to success. Then add your messages to the experimentation’s powerful real-time feedback system, and continuously learn from your customers to enhance both system knowledge and actionable outputs.
Recommend the right things at the right time to the right people
ecosystem.Ai are advocates for appealing to the human as a changing being. We have structured our recommenders to always remember the human, by offering fresh, new options to customers to introduce an element of novelty. Our recommenders offer further enrichment to businesses by providing accurate and timely view of customer takeup. Real-time information combined with human behavioural analysis, allows for more personalized, targeted offers at pivotal times in the customer journey.
Engagements are enhanced, as the customers behavior determines the design, structure, amount of information, and wording that can be used. The recommender can then offer the best options depending on feedback from the customer in real-time.
Multiple recommenders and experiments can be run at the same time, all easily managed from one central location - the ecosystem.Ai Platform. Companies should always be experimenting. When you increase the frequency of experiments and deployments, you stay more current and more competitive.