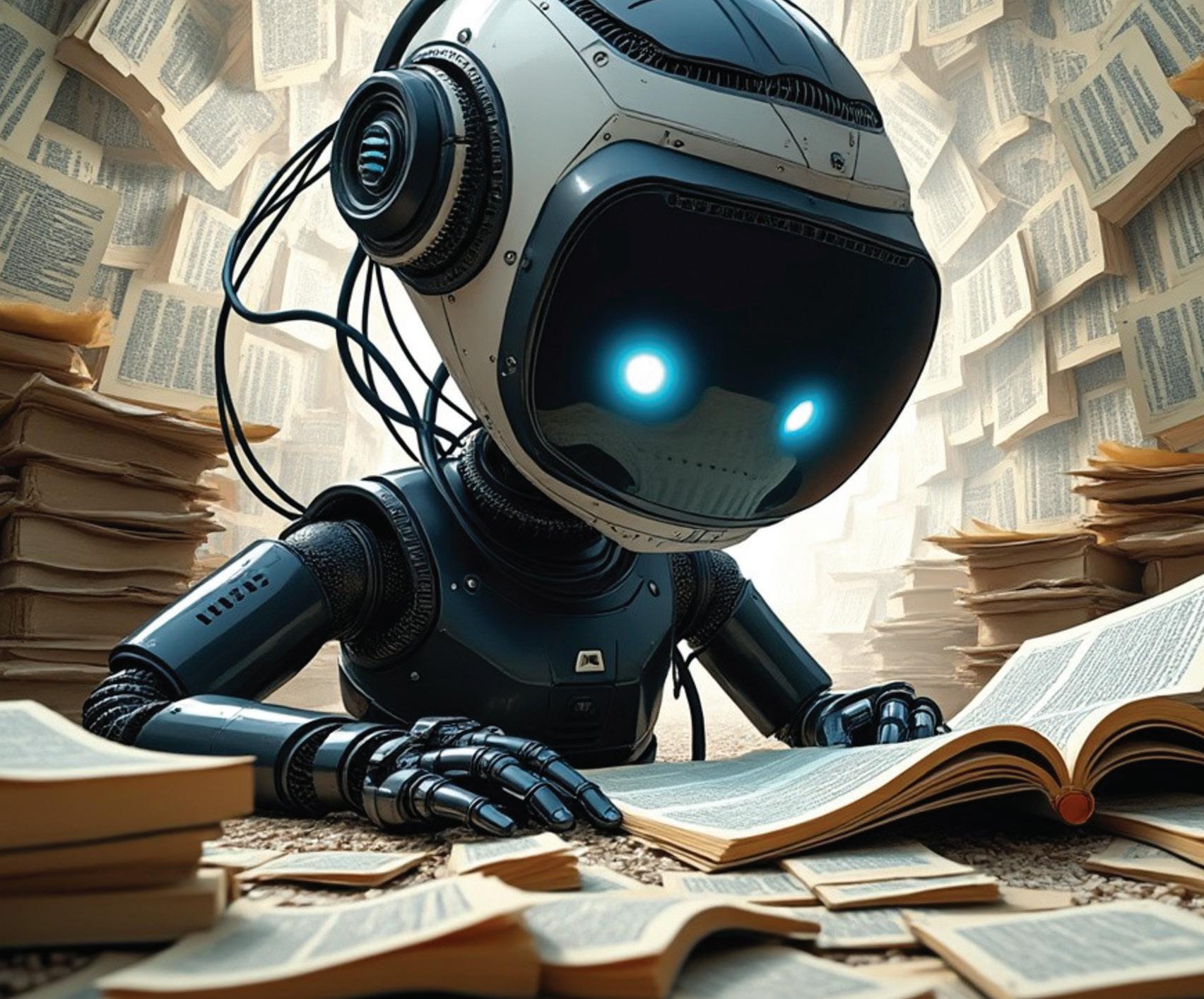
4 minute read
MAPPING THE MIND WITH AI: A JOURNEY INTO THE CHALLENGES UNSTRUCTURED DATA
/ By Tyler Donaldson, Lead Data Scientist, OLSPS /
Recent developments in large scale LLMs (large language models) are supercharging behavioural analytics in new ways. OLSPS has been at the forefront of mapping in-situ workplace mindsets and behaviours for the past 15 years. Building rich, situationally aware behavioural frameworks that explain as much as 40% of bottomline employee performance. Now, using free-text data and 15 years of insights, OLSPS is pushing the envelope on a situationally aware behavioural AI that can do everything from coaching individuals to informing company strategy, structure, and culture.
The firepower in our arsenal is the rapidly evolving space of LLMs. These LLMs are semi-intelligent in that they already understand languages and language structure, and even, to an extent, the general basis of human mindsets and behaviours. It is possible to use these LLMs as a base model which can then be ‘fine-tuned’ by training it further on very specific data and prompting it with context and limitations. They are easy to use, affordable, and readily available. The real challenge in implementing these LLMs, which isn’t limited to this use case, is the data.
The data preparation for ‘fine tuning’ one of these LLMs is not a trivial task.
Firstly, data quality and relevance are paramount. Poor data quality, or irrelevant data, can lead to suboptimal model performance, which could misrepresent customer interactions or make inaccurate predictions.
The volume and diversity of data also pose significant challenges. A model trained on too little or too narrow data might excel within those parameters but fail when encountering new scenarios or create discriminatory biases in the LLM.
Data labeling and annotation is another labor-intensive aspect. This process can be slow and expensive, requiring human expertise to ensure accuracy and consistency. Mislabeling or inconsistent annotations can degrade the model's learning process.
Cleaning and preprocessing of data involves normalizing text, handling missing data, and ensuring privacy by removing personal identifiers. This step is crucial to avoid introducing errors or biases into the model, which could have legal or ethical implications.
From a computational standpoint, there’s a balance to strike between model performance and resource use. Fine-tuning demands significant computing power, and businesses must manage this cost against the expected enhancement in model accuracy.
Ethical and legal considerations are vital, particularly around inherent bias in the data. Ensuring the model does not perpetuate or introduce discriminatory biases requires careful data curation.
Context of the data is also significant, to avoid the LLM misinterpreting the meaning of certain data. The often requires business discovery, and an understanding to the data sources, usages, and systems.
Lastly, multilingual and cultural adaptation along with continuous learning to handle data drift are ongoing concerns. As customer bases or operational environments change, models need regular updates to stay relevant, which involves revisiting the data preparation phase.
In essence, for a business, the journey from raw data to a finely tuned LLM involves navigating through a maze of technical, ethical, and operational challenges. This process is where experienced data scientists are still required to effectively utilise these revolutionary LLMs in specific contexts.
For PROPEL, the behavioural analytics tool that OLSPS has been developing with Enjol for the past 15 years, the data problem is lessened by the fact that our data collection was designed in-house by data scientists from the outset, meaning most of the above challenges can be simply solved with the right know-how.
Beyond the data, our AI is agent based, and heavily prompted. The flow of decisions and integrations needs to be designed and integrated. Ongoing training, improvement, and model lifecycles need to be considered. As well as several other issues.
At the end of the day, the LLMs available now are incredibly powerful tools. They cannot, however, be blindly applied. Applied AI requires the experience and wisdom of applied data science. Failing to prepare the data correctly, curtail the scope of the LLM, prompt it concisely, or effectively curate it, can often lead to rouge, unhelpful LLMs and even lawsuits.