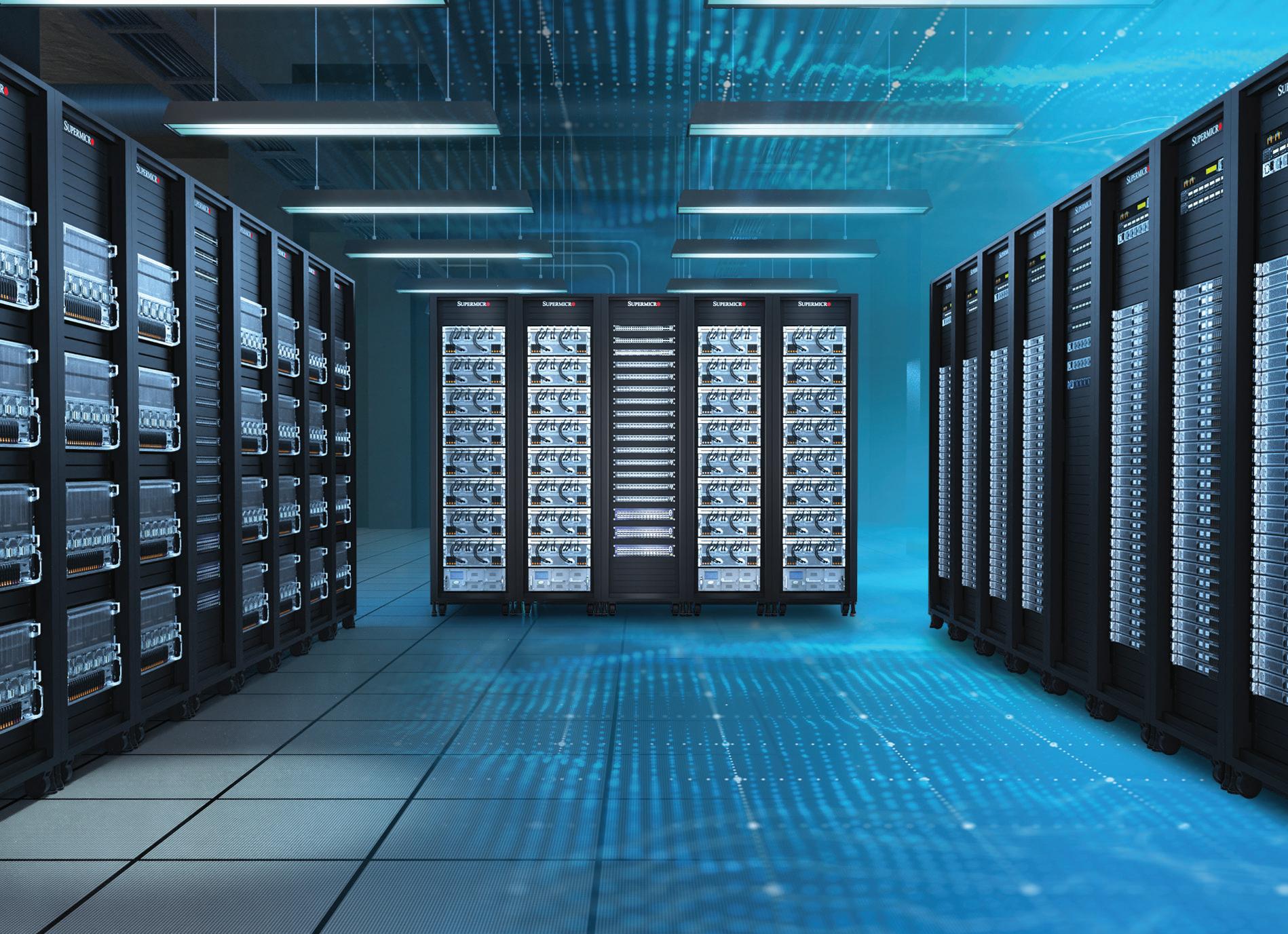
4 minute read
PREPARING DATA CENTERS FOR AN AI-DRIVEN WORLD
Today’s compute-intensive workloads require purpose-built, future-proof systems
As artificial intelligence revolutionizes the business world—allowing companies to analyze and extract insight from data at lightning speed—it’s also transforming the data center. No longer can companies simply add another rack of multipurpose servers. They must equip their centers in a whole new way.
Moreover, AI workloads are more computationally intensive than traditional ones, meaning that gaining immense computing power while increasing efficiency has become more critical. Those needs will only grow as AI use soars in coming years and workloads become more complex. According to Grand View Research, the global AI market size is expected to increase at a 37.3% compound annual growth rate between 2023 and 2030.
Building an ‘AI Factory’
Given the multifaceted nature of running AI, companies increasingly must view their data centers as “AI factories,” says Alok Srivastava, senior solutions manager at Supermicro, a leading NVIDIA partner and provider of AI-capable, high-performance servers, management software, and storage systems. The term “AI factory” was coined by NVIDIA founder and CEO Jensen Huang. It refers to a cohesive system explicitly designed to run AI workloads—or even a single AI application, such as generating human-like responses with a chatbot — with optimum efficiency.
“We traditionally think of factories as where manufacturing and mass production happens,” Srivastava says. “In an AI factory, you’re manufacturing intelligence: Raw data goes in, intelligence comes out.”
These systems blend the hardware components needed to run AI applications with the necessary software components, such as machine-learning frameworks, dataprocessing libraries, and scalable storage solutions. The value of this approach is that you’re creating an optimized system that can provide maximum performance for the tasks at hand, whether training generative AI models, deploying real-time inference at the edge, or accelerating high-performance computing applications.
“You gain efficiency and performance when you create a system around one workload instead of trying to serve multiple workloads simultaneously,” Srivastava says.
As companies create an AI factory, they must prioritize scalability—given that data volumes are only expected to grow exponentially—and flexibility to adapt to changing AI demands, he adds. This futureproofing can reduce long-term costs while allowing companies to stay ahead of the rapidly evolving AI curve.
Staying Adaptable
Supermicro creates built-to-order AI systems by taking a building-block approach. It allows customers to tailor systems for their exact AI workloads, optimizing for factors like GPU density, energy efficiency, and I/O throughput. Those customized systems include the specific hardware components and the software and applications needed to run that particular workload.
“The beauty of Supermicro is that we can provide the most advanced AI infrastructure building blocks available from NVIDIA for these modern workloads,” Srivastava says. “The entire system is plug-and-play, meaning once you receive the system, it’s ready to run your applications.”
The building-block approach is also key to future-proofing because systems can be upgraded and reconfigured as new and better components become available or AI needs change. When a supplier introduces a nextgeneration GPU with better performance and more power, Supermicro’s existing systems can be easily upgraded with that GPU. “This is a unique aspect of our systems, that with minimal changes, you can be ready for the next generation of accelerators,” Srivastava says.
Moreover, the company provides its customers with customized service and expertise, helping them configure their systems to optimize performance and efficiency.
One large enterprise customer asked Supermicro if they could help solve a problem: It had fans running to cool the system, but one of the fans was providing more cooling than needed—wasting energy. Supermicro helped reconfigure the system into two separate cooling zones, reducing energy use in the part of the system that needed less cooling.
“We ended up saving that customer a lot of energy, and we learned things from the process we’re now applying to our next generation of systems,” Srivastava says.
Supermicro’s modular and customized approach ultimately means customers benefit from a flexible AI architecture that enables seamless scalability—allowing them to quickly ramp up their capacity and compute power as their AI needs evolve and expand. The systems are also optimized to run the applications customers use to get the most out of their AI.
“This flexibility and scalability allows our customers to get the most out of their AI workloads today while being able to expand and grow fast in the future,” he adds.