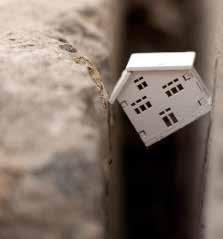
8 minute read
SOME CONSIDERATIONS WHEN MODELLING RISK AND UNCERTAINTY
By Barry Yeldham AAIQS (Ret.)
The use of risk estimating statistical packages is a common method of avoiding the many weaknesses of single value estimating. Many quantity surveyors find themselves participating in risk workshops where risks and occasional opportunities are evaluated. Workshop leaders and participants share the responsibility for creating a mathematical risk model that closely reflects the scale and extent of the anticipated risks and uncertainties. While participants share the responsibility, they are usually at a disadvantage, being unable to control the direction of the workshop and perhaps unable to interrogate the legitimacy of the values being adopted. To address this issue and “level the playing field”, I have prepared a risk estimating Excel workbook (“BasicRiskModel”) with a suite of User Defined Functions for the commonly used triangular and PERT distributions and the ability to handle multiple iterations of a risk model. This is available to download via the link at the end of this article.
Risk modelling typically uses three-point estimating and a probability profile to calculate the range of possible final outturn costs, and from this determines the risk contingency that should be applied at any probability level. My experience in reviewing risk models had lead me to the conclusion that a number of modellers have not correctly understood the nature of certain types of risk events.
One example is the failure to distinguish between the risk treatment of events such as “discovering asbestos during demolition,” and “finding rock during excavation”. At first sight they appear to be similar, but while the first is a “normal” event risk where likelihood and consequence are assessed independently, the rock risk is an example of a cost which occurs when a potential threat (the possible rock depth) crosses a particular threshold (the bottom of the excavation).
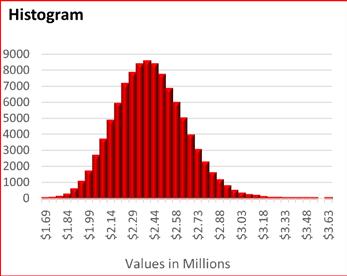
To better understand the nature of the rock and similar risks it is probably simpler to consider a more easily analysed situation, and for this I chose the risk of a critical piece of equipment not being delivered in time. Making the example concrete, suppose that the manufacturer has given a range of possible dates spanning a 10week period with the expected date somewhere in the middle of the range and the latest possible delivery date 15 days past our deadline. Since we have decided that the risk profile of the delivery time is unskewed, we may calculate the most likely arrival time as 20 days prior to the deadline. (70 days / 2) – 15 days and the earliest date is 55 days prior (15 days – 70 days). A risk profile of this uncertainty could be modelled with a minimum (Min) -55 days, most likely (ML) -20 days and maximum (Max) +15 days. If delays cost $10,000 per day, then, using a Beta PERT risk profile, this risk could take the form = RiskPert(-550,000, -200,000, +150,000). Clearly this formula would frequently return invalid negative values, but when modified to =MAX(0, RiskPert(-550,000, -200,000, +150,000)) the formula will replace all negative results with zeros.
The distinguishing feature of this risk is that it does not require a separately estimated likelihood since the frequency of occurrence will equal the frequency of positive values. It is this feature that requires “Threshold” risks to be treated differently from “normal” risks. Given the maximum cost and the skew of the threat distribution (commonly unskewed), there is a direct relationship between the most likely value, the minimum value and the likelihood. It is therefore possible to create the risk profile based on the maximum value, the skew and either the assessed percentage likelihood or the assessed positive ML value.
The rock risk is similar as the cost occurs only when the risk profile of the rock depth extends above the lowest excavation depth. Another example is the risk of flooding when a river breaches a levee. The risk occurs only when an underlying threat passes a certain threshold.
In these cases, the risk may be represented in the model, in the normal format, with a percentage likelihood and the three values, but truncated at zero. The calculation for this is based on an iterative solution which is cumbersome in the context of a risk workshop. The “BasicRiskModel” contains a worksheet which calculates the values automatically using the Max, Skew and either the assessed percentage likelihood or the positive ML value.
Estimate items are often ranged as a percentage either side of the ML value. One commonly recommended technique to assess these percentages, which avoids the need to determine the extreme values at which possibility fades into impossibility, is to allow the workshop to instead assess upper and lower limits where there is still a 10% chance that higher or lower values are possible. This can be useful for estimate items with a more or less accurately determined central ML value and where the ranges are typically less than those found with risk events. Even with estimate items, participants may not be able to assess 10% with any accuracy or understand the implications of their choices.
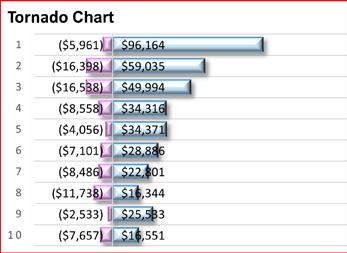
Risk software must use the estimates and the chosen risk profile to calculate the actual extremes, and although there may be agreement about the 10% values, that agreement may not survive knowledge of the real minimum and maximum values that they have unknowingly created. This method is frequently promoted as avoiding the discomfort felt by estimators and engineers in assessing real extreme values and, to avoid unsettling them, the actual limits are not revealed. I suspect that most participants are unaware that increasing the P-90 estimate will reduce the extreme minimum that their P-10 estimate produces. In the case of risk events, where ranges are typically quite wide, this method of assessment can lead to unrealistic results. I have seen numerous examples of event risks using P-10 and P-90 values which result in the possibility of clearly invalid negative results. As an example, a PERT risk distribution of Min $100,000; ML $300,000; Max $500,000, with a 10% possibility that the actual cost could be lower or higher resolves into an extreme minimum below negative $94,000. While the possibility of cost savings may be a clear sign of error, other values might also be considered unreasonable were they known. It is for this reason that I consider such techniques unsuitable for event risks. Also, since threshold event risks and many others will require zero or near zero minimums, this method can never be used in association with such risks.
It would be better to allow participants to know what values the risk software will create. The “BasicRiskModel” contains User Defined Functions that allow users to interrogate the assumptions made at the workshop. It will allow attendees to understand the true range of any costs which are assessed at the P-10 and P-90 or any other percentage values.
I have the impression that many of the event risks are being assessed by first guessing an ML value and then putting percentages either side of this value. This is generally unsuited to event risks where the extreme values may not have the same dependence on the most likely value. Participants should always be cautious with minimum values and question why they cannot be lower and still be possible. In my opinion, it is more reliable to assess these risks by first assessing the Max value, then the Min value (which is often at or close to zero), and finally deciding where, within this range, the ML value lies, and furthermore not confusing it with the average value as there is a significant difference when distributions are heavily skewed.
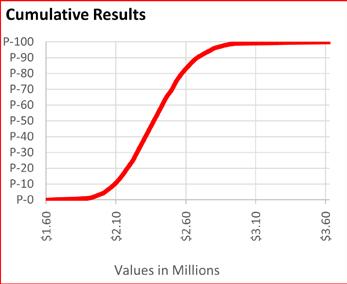
Regarding the choice between triangular or beta PERT distributions, triangular is often chosen based on its reduced emphasis on the ML value, but note that the corresponding increased emphasis on the extremes leads to slightly higher P-95 values when distributions are unskewed. This would be an unusual situation as most distributions are quite strongly positively skewed and it may well be that outputs at all P-values are substantially increased by the use of the triangular profile.
Some commercial statistical programs provide a modified beta PERT with a variable “confidence” index. This is also available in the Excel workbook “BasicRiskModel”. Lowering the index when distributions are skewed will result in generally higher P-values. It should also be noted that, when using a modified beta PERT, increasing the “confidence” index has the effect of increasing the difference between the estimated P-10 and P-90 values and the actual extremes of individual items.
To navigate Microsoft’s attempts to protect computers from harmful viruses in .xlsb workbooks created by unknown providers, the following instructions may assist.
The download is a zipped file. Copy it to a folder, right click the file, select Properties and under the General tab, tick “Unblock” then “Apply”. Open the file and click "Enable Macros". Note that your Trust Centre options should be set to “Enable all Macros” and “Allow Trusted Locations”. If you have any difficulties, please email the author on the temporary address, byc.xjk@gmail.com
This article was written by Bary Yeldham AAIQS (Ret.), regarding his developed Excel workbook which can be downloaded via Dropbox (bit.ly/3LoKfj1).