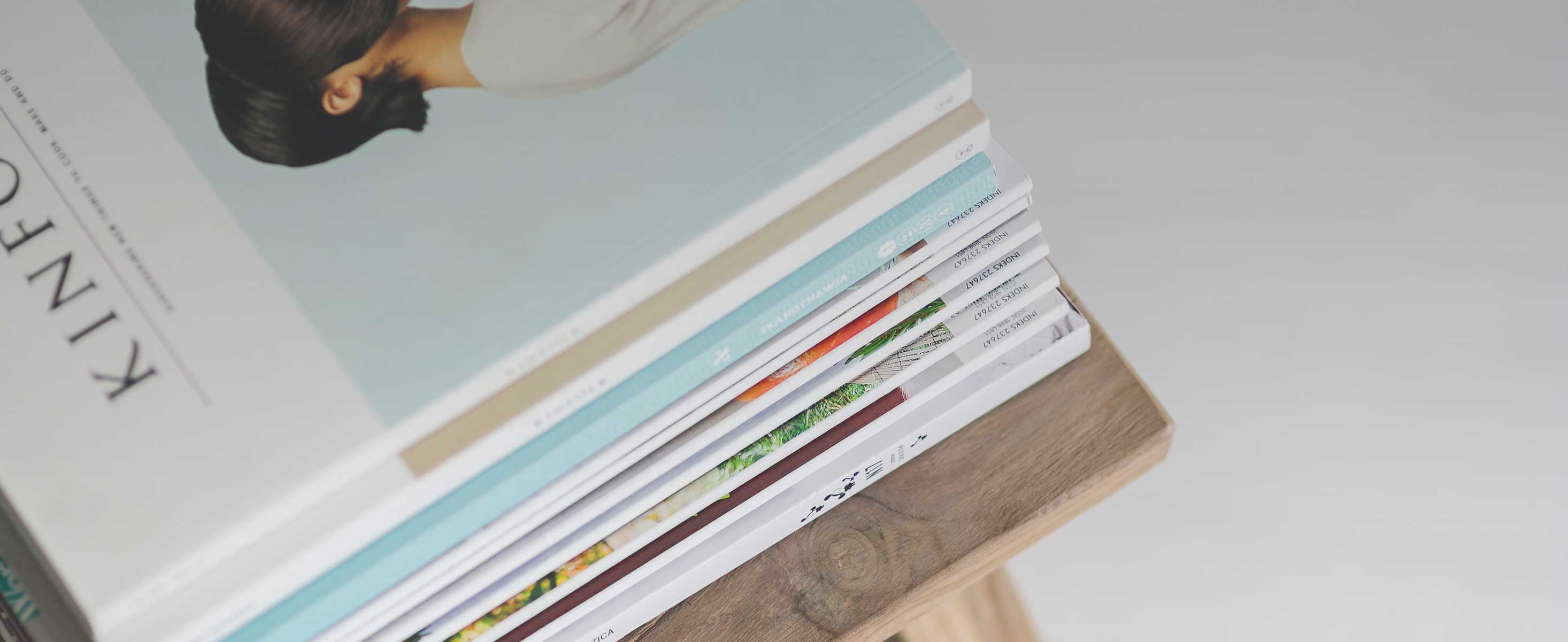
13 minute read
Managing the Mastery of Data
BY SUKETU GANDHI AND JOSHUA SWARTZ
In today’s dynamic, ever-shifting business environment, most companies realize that data is a key source of strategic advantage and an important differentiator that must be used properly.
Advertisement
Many companies, though, are struggling with managing their mastery of data because they don’t look at it in the right way and can’t react to it efficiently. Many are managing their businesses without seeing the full picture, such as by using and evaluating only backward-looking data instead of incorporating alternative datasets. However, a few companies are managing the mastery of data, and their success both lights the path for others and underscores two important reasons for mastery. One, companies using data well can generate as much as 83% more profits than those whose analytics maturity lags significantly behind that of the leaders, according to Kearney’s 2019 Analytics Impact Index. Two, the value of the data itself has become much greater. In 2018, data represented 84% of the value of most companies’ intangible assets. The overall value of these intangibles was $21 trillion in the United States, alone—more than five times greater than the value of companies’ tangible assets, up from three times greater in 2005, according to AON and Ponemon Institute’s Intangible Assets Financial Statement Impact Comparison Report. Each data leader has succeeded by taking specific steps: they have gained an understanding of the three dimensions
of digital transformation; they have clearly recognized and overcome the roadblocks to transformation that others overlook; and they have begun their transformation with targeted actions rather than by attacking all issues at once. The three interacting dimensions of digital transformation and managing the mastery of data are mindset, skillset, and toolset. They are equally important and work in tandem. Successful companies have learned that transformation is more likely to drive improvement when all three are addressed together, rather than individually—an approach that may seem counterintuitive to those who think technology investment will solve all their problems.
MINDSET Mindset is about changing what the company thinks about the data itself, how it thinks about its business, how it makes decisions, and how the business should operate. Changing the company’s mindset in the following five ways is a key step to managing the mastery of data: Shift from backward-looking data.. Throughout industries, including retail, it is common for companies to think that what
happened yesterday will happen again tomorrow; so (their thinking goes) all they have to do is look at history to predict the future. This mindset gives a limited, and incorrect, view of the future because it relies only on backward-looking data in a rapidly changing business environment. Further, analytic capabilities to enable predictive and prescriptive insights are readily available in “turnkey” offerings from large cloud providers. To manage the mastery of data, companies can no longer drive their operations with a lens on the past. Shift to a probabilistic view of data. Most companies today have a deterministic perspective of data: They believe that because data is definite, it is always 100% correct, so they tend to put too much faith in their interpretation of it. To overcome this problem, they need to change their mindset to a probabilistic perspective, which means thinking about the accuracy and likelihood of implied insights (for example, scoring). This change is a huge shift in structural thinking and more difficult to achieve than moving away from a reliance on historical data. To achieve it, companies must develop a much greater knowledge of, and deeper appreciation for, statistical methods. They must stop believing that data is always right. As successful companies have aggregated large quantities of both well-defined and alternative data (such as that from many sources), they have learned how misleading a deterministic view can be. They know that a probability perspective leads to different—and more accurate—responses than those based on a deterministic view of data alone. Shift to a closer working relationship between people and data. The third necessary mindset change is to gain an understanding of the importance of having people and large amounts of data and analytics work together. Companies are starting to use artificial intelligence (AI) and machine learning to build business models and manage the business, but they often fail to bring these two approaches together to build the best model. Instead, they let a machine run through the data and find a model they assume is correct because it came from a machine. A better approach is to start with this model but then assign someone to run the data separately to find an independent model and to check and balance the different models to arrive at the best one for their operations. Shift to multiple sources of data. Companies also must learn that the algorithm’s power increases with more data, which is delivered through multiple datasets. One dataset will provide only one answer, whether correct or not. But by running through different datasets, a company can generate dramatically different, more complete results. Goldman Sachs used limited data to determine the credit level for a husband and wife who each applied for the new Apple Card. As a result, the man received a much higher credit level on his card than the woman obtained, and a maelstrom of criticism erupted on social media. Shift from viewing data as an afterthought. Companies often view data as an add-on or afterthought—an old mindset that will slow, if not stop, their digital transformation. Instead, they need to change this mindset so they view data as a core component of the business and of each product and service—a major perspective shift that will affect everyone in the company.
SKILLSET The skills companies have depended on for years no longer meet the demands they face now. As always, they need
people who understand their business and how it operates. But, equally important, today they also need people who know how to make the business win in a digital and datarich environment, people who can work closely with those who have a different skillset: data engineers and statistical experts. Companies need these data scientists to gather, interpret, and draw insights from all the available data. Furthermore, adding these skills will change the way the company’s work is accomplished. Work will no longer be done by siloed groups that protect their turf from outsiders, but rather by agile teams from multiple functions coming together to drive decisions. Most people would agree that these business and datascience skillsets are logical requirements for companies today, but they may be surprised to learn that the following skills are important to companies as well. Psychological and anthropological skills. Retailers should employ a psychologist, or at least someone well trained in how people make decisions when confronted with large amounts of data or conflicting signals that impede decision making. The psychologist will help the company present information in a way that clears workers’ confusion and biases and nudges them into making decisions that enable them to efficiently extract value from the data, freeing them to move on to other issues quickly. Similarly, someone with anthropological skills will give the company insight into why people have a negative bias
against results generated by machines, especially if those results disagree with their own preconceived notions. This person can help others overcome this bias or at least learn to work around it and help them understand the difference between the two kinds of decisions that need to be made: those concerning problems whose factors are known and those about problems with unknown variables. Factors surrounding certain decisions—like those of math— are well understood, so prescriptive recommendations can be done by machines. Further, where these decisions have no subjectivity (for example, sales indicators), automation can reduce human work, and where subjectivity is required (for example, customer feedback), human intervention is aided by machine recommendations. On the other hand, problems that have unknown variables require decisions that come only from human thinking. When employees understand the difference between these types of decisions and know how to separate them, they will no longer waste time on transactional decisions but will focus on the subjective and difficult ones that require human interpretation. Storytelling skills. Companies that want to manage their mastery of data need to become efficient in storytelling—a skill perhaps most considered necessary for fiction writers, not businesspeople. A storyteller can provide a descriptive analysis of the data, bringing it to life for those whose eyes glaze over when looking at spreadsheets.
While visualization of data is often helpful, studies show that many people find that reading a sentence or two about the findings produces a more immediate and clearer understanding of what someone is trying to depict. A table showing a sales drop might present this information as “-35%,” leaving readers to interpret the data as they choose. But a qualitative and insightful description of the change will force readers to think more carefully about the information and what it really means.
TOOLSET At the same time that a company changes its mindset and skillset, it also will want to adjust its toolset, making sure it puts in place the underlying technology-enabled capabilities that will make it work more efficiently. Toolsets built on a belief that tomorrow’s business and customers will be much like those of yesterday are useless for the digital enterprise. Today’s forward-looking toolset will give the company abilities that only recently became possible, such as the following: • Working with hybrid clouds to reduce cost and increase data accessibility • Using AI and machine learning visualization tools to improve the consumption of data insights • Taking advantage of third-party data in real time to complement and complete the data picture • Evaluating past actions against their outcomes to inform future predictions • Running simulations about planned actions to inform future recommendations None of these abilities is possible with outdated analog toolsets. Organizations across industries are changing their mindsets, skillsets, and toolsets—and in doing so are providing examples of how companies can improve their business and better serve their customers. In the medical industry, for example, doctors are now looking not only at transactional data about patients with the flu but, more important, at an array of alternative datasets (local Google searches for “Do I have the flu” and weather patterns, among others) to understand where a flu epidemic might begin and how it might spread. Then, they use the insights from this research to stock nasal sprays, hydrating fluids, and other items in their offices and hospitals before the illness affects their communities. Also, three U.S. economists won the Nobel Prize in Economic Sciences in 2019 for their work on the concept of randomized control trials (RCT). Through this work, they developed significant insights by using deep data at an extremely granular level in studies in which randomly selected participants received either a standard treatment or a particular intervention. The results of one study point to ways of lifting people out of poverty, while those of another found that if doctors in India offered a kilo of lentils to the adult accompanying a child to their office, they could significantly improve the country’s vaccination rates.
ROADBLOCKS Why do many companies find digital transformation to be so difficult? Because the transition from being a historically analog company to a modern-day digital enterprise does not take place along an easy and smooth path. Every company attempting to manage the mastery of data must overcome a number of obstacles, including these three major ones: Acquiring data-science skills. No silver bullet exists for skipping over or going around the roadblock a company faces when trying to acquire needed data-science skills. There is a severe shortage of data-science talent in the marketplace today, and this shortage continues to increase. In addition, the available talent is costly to recruit, and the competition for these workers often leads to a short tenure at one company before they move on to better opportunities and increased salary somewhere else. To lower this barrier, companies are looking for alternate solutions. Some are sending their employees to be trained, although this is not a quick fix. Others are outsourcing at least part of their datascience requirements to third parties. Obtaining data tools. Many companies believe that obtaining the new data tools they need is a daunting and expensive effort, so they try to go around this roadblock by forcing their old tools to do new tasks they were not designed to handle. This approach never works. Updating the toolset can be less expensive and daunting than many companies envision. Furthermore, they do not have to start from scratch to organically design a one-off set of tools. Instead, they can leverage open-source software because every algorithm any company needs is available somewhere in the market today, and can best be obtained by treating this purchase like that of any piece of industrial-grade software: Know what tools are available, evaluate the responses, and choose the tools that best support the company’s efforts and address its challenges. To make certain it gets the best tools, the company will want to put in place a chief data officer (CDO) who is accountable for this purchase and for overseeing the data-science team. This team must be recognized as a strategic business function of the company and not just a technology function. And the data department should be separate from the IT department, with the CDO serving on the company’s leadership team and reporting to the COO or CFO. Changing the company’s data mindset. The biggest roadblock a company faces when trying to manage the mastery of data is changing how data is viewed throughout the business. Typically, the old view of data as an add-on or afterthought is deeply entrenched in the way companies operate, and this mindset can be changed only with a concerted, long-term effort. The effort will start with C-level leaders, all of whom must fully accept the importance of data in the company’s success and see it as a core component of each product and service. They must then integrate this new mindset throughout the company so that employees in every function—not just data science—understand data’s significance and how their roles impact the company’s strategic decisions.
GETTING STARTED—FIRST STEPS To manage the mastery of data, a company must change the three dimensions of digital transformation and overcome the roadblocks obstructing success. This effort may take many months, if not years. The good news, though, is that no company has to jump into the effort by trying to attack every aspect of it at once. In fact, it can—and should—start small, beginning with these three efforts:
• Focus areas. The company will want to focus on the highest payoff areas of the business—customer interaction, supply chain, and competitive landscape. Focusing on these areas will give the company knowledge of working with digital externally, internally, and within the competitive landscape—knowledge that will help it enhance the customer experience and improve the supply chain, while beginning to look at the future of its business and industry.
• Acquiring skills. Although the company eventually will need many new skills, it should not expect, or even want, to add large numbers of employees early in the digital transformation process. Instead, it will want to start small by hiring a few people for key positions and leveraging third-party workers to raise the skillset to the required level.
• Dual-mandate process. The company will want to run a dual-mandate process with one track being a series of small experiments and the other aiming to create the business’s long-term platform.
Companies everywhere are changing their mindsets by beginning to think differently about all the data available to them both within their operations and from multiple external sources. They are changing their skillsets by considering all the different skills now needed for success and ways to obtain those skills. And they are changing their toolsets by gaining an understanding of the kinds of tools that will work best for them in the environment in which they compete. As they make these changes and overcome the roadblocks hindering their progress, they are learning how to manage the mastery of data and are successfully transforming into digital enterprises. AQ
Suketu Gandhi is a partner with the global management consulting firm Kearney in the Digital Transformation Practice and the global product leader, Plan and Digital Supply Chain. Joshua Swartz is a principal in Kearney’s Digital Transformation Practice.