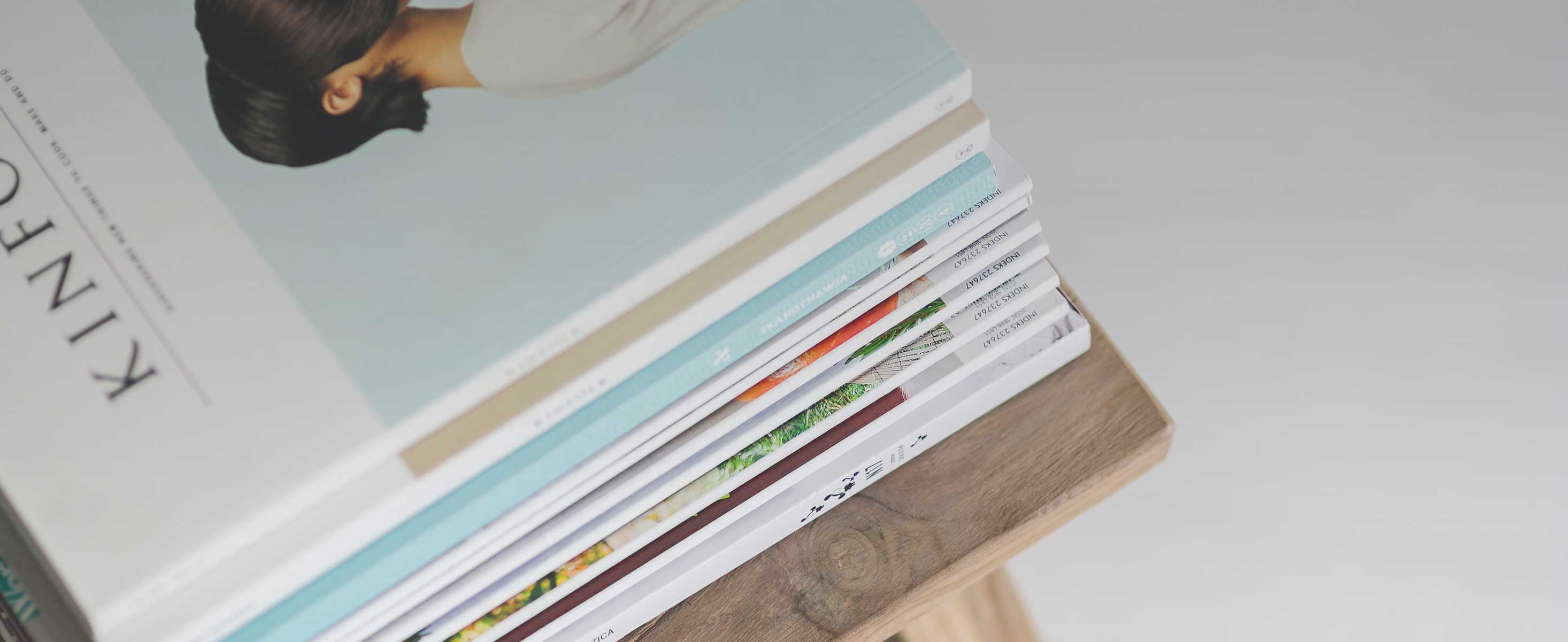
1 minute read
Future Trends in Machine Learning for Data Science
Deep Learning
Deep learning, a branch of machine learning, is concerned with neural networks having several layers It enables machines to learn hierarchical representations of data and has shown remarkable success in areas such as image recognition, natural language processing, and speech recognition. As computational power increases, deep learning is expected to drive significant advancements in data science
Advertisement
Explainable AI
Explainable AI aims to make machine learning models more interpretable and transparent As the adoption of machine learning algorithms grows, so does the need to understand their decision-making process Explainable AI techniques provide insights into the factors influencing a model's predictions, helping data scientists and stakeholders gain trust and confidence in the models.
Automated Machine Learning
Automated Machine Learning (AutoML) focuses on automating the process of model selection, hyperparameter tuning, and feature engineering It empowers non-experts to leverage machine learning without in-depth knowledge of algorithms or programming. AutoML tools streamline the machine learning workflow, enabling faster model development and deployment
Conclusion
Machine learning plays a vital role in data science, enabling organizations to unlock valuable insights and make data-driven decisions Its applications span predictive analytics, natural language processing, image and video analysis, fraud detection, and recommender systems However, challenges such as data quality, interpretability, and bias must be addressed to ensure the responsible and ethical use of machine learning in data science.