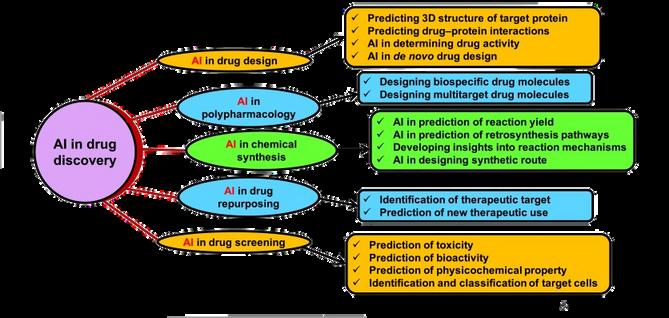
2 minute read
AI Mediated Drug Discovery And Development
article by-
Sweta Sahu
Advertisement
The pharmaceutical business has undergone a transformation that is nothing short of revolutionary as a direct result of the integration of artificial intelligence into drug discovery and development. This has resulted in an acceleration of the sector's overall growth In this article, we will explore areas of integration, tools and approaches used in the enforcement of AI, current obstacles, and potential solutions to those challenges. In the pharmaceutical industry, data digitization has increased dramatically in recent years However, the difficulty of gathering, evaluating, and utilising knowledge to solve complicated healthcare problems arises with digitalization. This encourages the adoption of AI, which can manage massive amounts of data with greater automation AI is a technology-based system that use a variety of advanced tools and networks to simulate human intellect. At the same time, it does not threaten to totally replace human physical presence. AI employs systems and software that can read and learn from input data in order to make independent judgments for achieving certain goals.
Drug discovery and development are two of the most important translational scientific activities that contribute to human health and well-being. However, developing a novel medicine is a hard, costly, and time-consuming procedure. The combination of artificial intelligence (AI) with new experimental technologies is intended to improve the search for novel drugs faster, cheaper, and more effective.
In spite of its many benefits, artificial intelligence (AI) is currently confronted with a number of significant data challenges, including the scale, growth, diversity, and uncertainty of the data. The data sets that pharmaceutical companies have available for drug development can involve millions of compounds, and the traditional ML tools that are used may not be able to handle the complexity of this type of data. A computational model that is based on the quantitative structure-activity relationship (QSAR) can quickly predict large numbers of compounds or simple physicochemical parameters like log P or log D On the other hand, these models are a long way from being able to predict more complicated biological properties, such as the efficacy and adverse effects of compounds In addition, models that are based on QSAR are plagued by issues such as having insufficiently large training sets, having experimental data in training sets that is inaccurate, and not having sufficient experimental validations. The recently developed AI approaches, such as deep learning (DL) and relevant modelling studies, which can be implemented for safety and efficacy evaluations of drug molecules based on modelling and analysis of big data, can help overcome the challenges that have been presented here. Various AI tools are being used to aid in all four stages of the drug development process (basic research for drug discovery; pre-clinical phase; clinical phase; and postmarketing).
Identifying molecular targets, searching for hit and lead compounds, synthesising drug-like compounds, and predicting ADME-Tox are some of the main tasks where AI has proven useful. On the one hand, this review brings a mathematical vision of some of the key AI methods used in drug development closer to medicinal chemists, while on the other hand, it brings the drug development process and the use of various models closer to mathematicians The emphasis is on two aspects that are not covered in other surveys: Bayesian approaches and their applications to molecular modelling, and the eventual final use of the methods to support decisions.
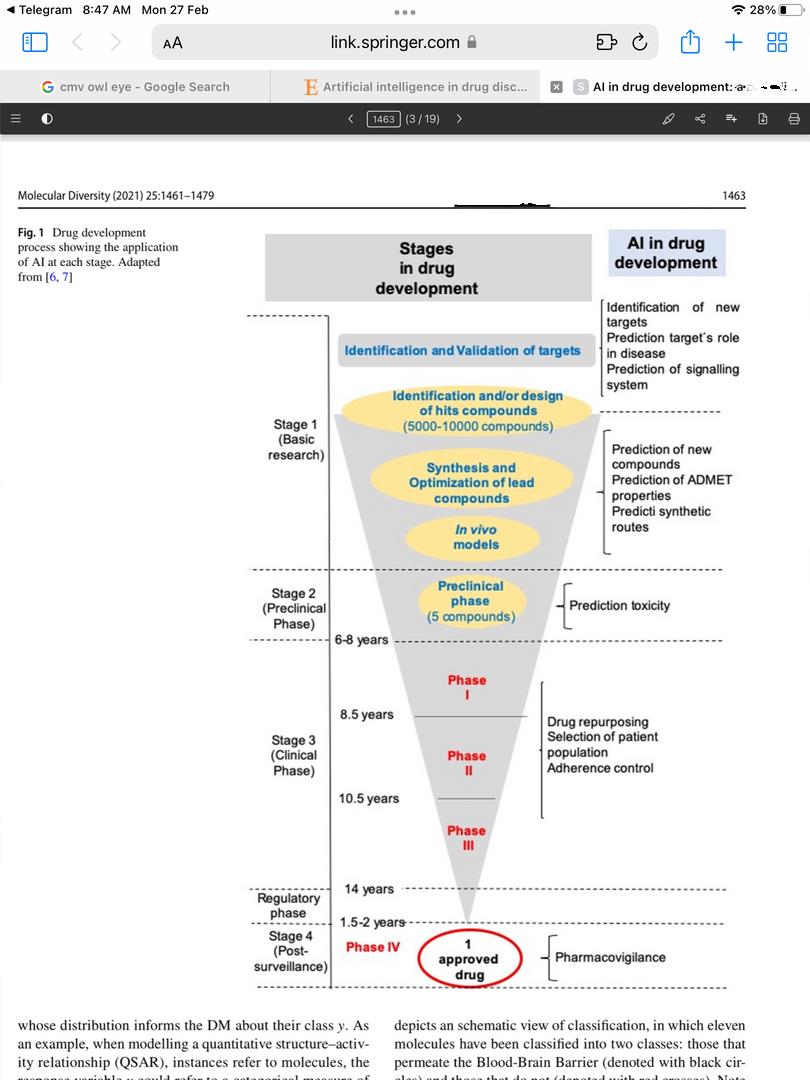