How Data Annotation is Beneficial for Artificial Intelligence and Machine Learning
The modern world is ruled by smart gadgets and equipment which are powered by Artificial Intelligence (AI) and Machine Learning (ML). Information is fed into AI machines so that they perform human-like actions. These machines work in accordance with the parameters that you establish for your data set. Data annotation is the solution that helps bridge the gap between sample data and AI/ML.
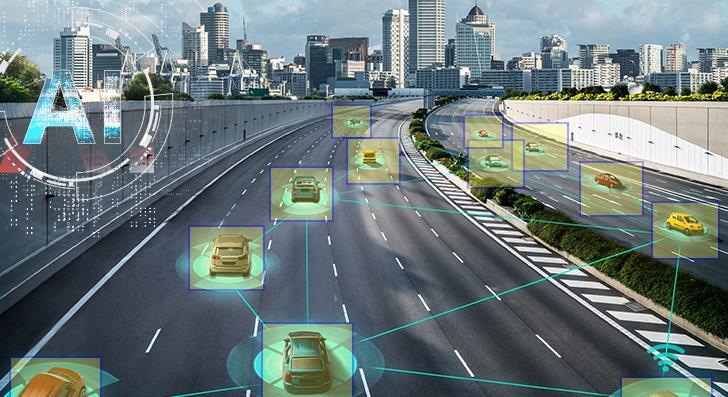
Data annotation guarantees that AI or ML projects become scalable. It is a process where a human data annotator adds labels, categories, and other contextual elements to the raw data set so machines are able to understand the information and can act upon it. Without data labeling, ML algorithms won’t be able to compute important attributes with ease.
Different Types of Data Annotation
The types of annotations to be used vary depending on what you want to accomplish from your AI and the data sources it will require. Here are the common types of data annotation services:
• Image Annotation
Image annotation labels images with keywords, metadata, and other descriptors that help comprehend the image with regard to other image descriptors. This annotation makes images accessible to users using screen readers and also websites like stock photo aggregators in recognizing and delivering photos for user queries.
• Text Annotation
Text annotation concentrates on adding instructions and labels to raw text. This helps AI to identify and understand how general human sentences and other textual data get structured to form meaning. The three main categories of text annotation that explain the different meanings with data sets are:
Sentiment: In this annotation, a human annotator gathers data for AI while ensuring they consider the emotional tone and subjective implication behind phrases and keywords. Sentiment annotation assists AI in understanding the meaning of texts beyond the dictionary meanings. This kind of annotation is beneficial for AI-powered moderation on social media platforms.
Intent: In intent annotation, the annotator concentrates on labeling the end goal of the user behind distinct statements. Intent annotation offers insight into the domain of customer service where AI-powered chatbots are needed to comprehend specific information or results that are needed to be delivered to a human user.
Semantic: Semantic annotation is driven by buyer-seller relationships and it works to offer clear labels on product listings so that AI is able to suggest in search results what customers are seeking.
• Audio Annotation
Multiple IoT (Internet of Things) and mobile devices are dependent on speech recognition and other features of comprehension. However, these devices only learn audial meanings via audio annotation. Audio annotators deal with data in the form of speech and other sound effects and the audio clips are labeled and categorized depending on factors like dialect, intonation, volume, pronunciation, and more. IoT devices rely on audio and speech recognition that comes from audio annotation.
• Video Annotation
Video annotation blends multiple features of audio and image annotation that help AI understand the meaning of visual and sound elements in a video clip. This type of annotation
has become especially useful in the development of technologies like in-home IoT devices and self-driving cars.
Features of Data Annotation
There are certain tools that make an annotation in all types of data annotation:
• Sample Sets of Smart Data
Data annotation can’t be practiced without the right set of data. As raw data comes in innumerable forms, it is important that data relevant to the training of your AI tools are chosen. The data is generally gathered from historic human interaction data that is present on the company’s file. However, open-source data can also at times meet the requirements of the data annotation project.
• Ontologies
Ontologies are blueprints that provide helpful and accurate frameworks for annotation. They include information like labeling guidelines, annotation types, and attribute and class standards.
• Dataset Management and Storage Tools
A huge amount of raw data is needed for data annotation of AI and ML projects. To organize both annotated and raw data and make it easily accessible, you have to store it in a software or file system that can handle the bandwidth.
How Does Your Business Benefit From Annotation Services?
Data annotation services improve the accuracy and quality of your data by offering you the expertise to annotate it. This makes your data more usable, shareable, and accessible. The services also help in improving your skills in data analysis. By annotating your data, you get to discover hidden insights and patterns that you might not have been able to discover before. Your business also gets access to recommendations on how it can improve its data.
Finally, data annotation services help you in improving the quality of your data analytics and warehouse tools. When you annotate data, you can guarantee that the information is easy to discover and consistent. This allows you to make informed decisions and improve the overall performance of your business.
Benefits of Using Data Annotation for AI and ML Models
Data annotation services make way for a better understanding of the meaning of the objects and help algorithms perform better. Here are some important advantages of data annotation for AI/ML models:
• Smooth End-User Experience
Annotated data provides users of AI systems with a seamless experience. An intelligent product is adept at addressing the doubts and problems of users by offering relevant help. Annotation offers the ability to act with relevance.
• Better Precision of AI/ML Models
A computer vision model operates with multiple accuracy levels over an image in which distinct objects are labeled accurately as compared to an image with poorly labeled objects. Thus, better annotation leads to a higher precision of the model.
• Easy creation of labeled datasets
Data annotation helps streamline pre processing which is a vital step in the ML dataset building process. Labeled datasets are important for ML models as they need to understand the input patterns to process them better and produce accurate results. Data annotation services result in the creation of huge labeled datasets over which AI/ML models can operate effectively. Clean labeled data is the key to foolproof AI & ML implementations.
• Ability to scale implementation
Data annotation accommodates intents, actions, and sentiments from distinct requests. With the help of annotated data, accurate training datasets are created. These datasets impart data scientists and AI engineers with the capability to scale the different mathematical models for different datasets of any volume.
Summing up
For the right application of data annotation, you need to leverage the mix of smart tools and human intelligence. This will help you create quality training data sets for machine learning. It is vital that enterprises devise strong data annotation capabilities for supporting AI & ML model building and ensuring it doesn’t fail.
Data that is accurately annotated lets you know when you have created a high-performing AI & ML model as a solution to a complex business problem. You’ll also get to know if you have wasted your resources and time on a failed experiment. Getting in touch with data annotation experts is the best move for your organization when you don’t have the required expertise and time to build a quality AI/ML model. The experts will help you rapidly scale your AI capabilities and conceptualize solutions of machine learning to meet customer expectations and market demands.
Read here inspired blog :
https://www.damcogroup.com/blogs/how-data-annotation-is-beneficial-for-artificialintelligence-and-machine-learning