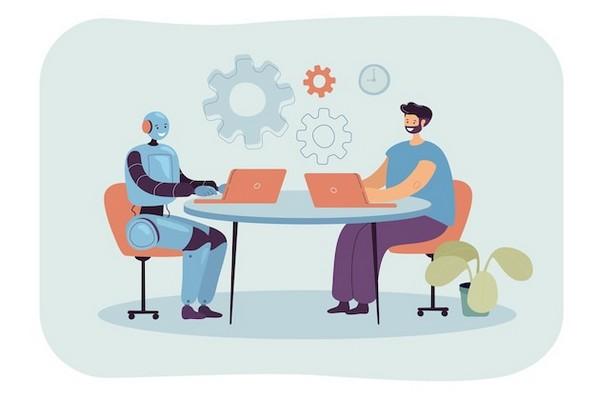
1 minute read
Challenges in Machine Learning Data Annotation
Applications of Artificial Intelligence and Machine Learning platforms are becoming commonplace for businesses. Yet, a thick layer of overhyped and fuzzy jargon shadows the challenges faced by companies looking to implement AI and ML-based models. Some of these are listed here:
• High-Quality Training Datasets
Advertisement
• AI/ML Projects are Data Hungry
• Cost of Project Completion
Key Advantages of Annotation in Machine Learning
For the Machine Learning algorithms to perform better, data annotation is the key as it provides a context and a deeper understanding of the objects. Collaborating with professional data annotation companies helps businesses to enjoy a gamut of advantages as listed here:
• Improved Precision
• Streamlined End-User Experience

• Progressive AI Engine Reliability
• Imparts ability to Scale Implementation
Conclusion
The right application of data annotation is only possible when businesses leverage the strategic combination of human intelligence and the latest technologies to create high-quality training data sets for Machine Learning algorithms. Companies must build strong data annotation capabilities to support their AI/ML project building and prevent it from failing miserably.
Accurately labeled data determines whether you created a high-performing AI/ML-based model as a solution to a certain business challenge, or wasted time and efforts on a failed experiment. So, when lacking resources and time to build such capabilities, collaborating with experienced data annotation companies is a smart move. Apart from time and cost optimization, professional providers allow you to rapidly scale your Artificial Intelligence capabilities and conceptualize Machine Learning solutions to meet customer expectations and match the market requirements.