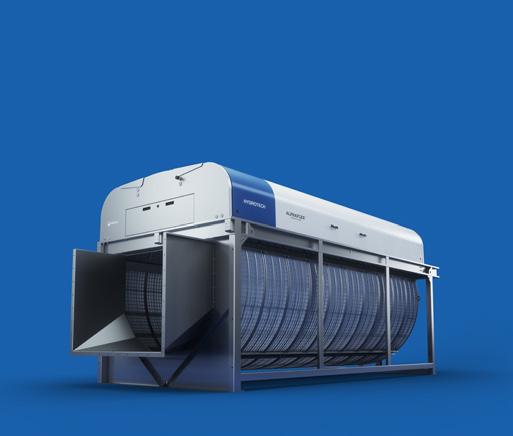
2 minute read
Using data science to optimise shrimp farm yields
How data science offers a systematic and scientific approach to detect variables associated with a high survival rate of more than 80% at a farm in central Philippines
By Neil Arvin Bretaña
Seafood is a vital source of sustenance for a growing global population and plays a significant role in food security. With wild fish stocks declining, aquaculture has become a crucial component in meeting the demand for seafood – one that is expected to increase five-fold in the next decade. However, ensuring global seafood security is a complex issue that requires a multi-disciplinary approach, including the use of data science to optimise aquaculture farm yield. Maximising yield while minimising resources, wastes, and environmental impacts requires a thorough understanding of the biological and environmental factors that affect the growth and health of aquatic species.
Traditional aquaculture methods rely on trial-and-error and intuition, leading to suboptimal results and increased costs. Data science, on the other hand, offers a systematic and scientific approach to optimising aquaculture. By collecting and analysing large amounts of data, data scientists can identify patterns and relationships that would be difficult to discern through intuition alone.
A case study in collaboration with a farm in Central Philippines
NSB-NB5 is a shrimp farm in Central Philippines that is starting to embrace data-driven innovation. In three previously recorded harvest cycles, the farm saw a loss of up to 50% in expected yield. This meant that up to half of the projected harvest failed to reach the market. This was despite attempting to maintain the farm’s standard inputs including feed, probiotics, and water quality. It was interested in identifying which of the modifiable parameters can be associated with this outcome. NSB-NB5 tapped Birkentech Solutions Pty Ltd, an Australian data science consultancy firm that focuses on supporting agriculture and aquaculture, to analyse their pond variables and identify the ones related to a high shrimp survival and yield.
Statistics-based data science approach
Birkentech recommended a statistics-based data science approach to achieve this goal. This was done in close collaboration with the farm management team and technicians. Data from three harvest cycles from nine Penaeus vannamei shrimp ponds consisting of daily recorded values for physicochemical measurements, feed and supplementary data and water management inputs were collected.
Physicochemical variables included pH, dissolved oxygen, temperature, salinity, depth, transparency, water colour and weather. Water management input included organic disinfectant, water probiotic, and minerals. Feed and supplement names were de-identified and coded to protect proprietary information.
At the end of each harvest cycle, the percent survival rate was recorded for each pond. The recorded survival rate, indicating the health outcome of the shrimp culture at the end of each harvest cycle, was utilised as the target variable for the study with a survival threshold set to 80%.
A total of 22,968 data points were made available. First, the basic summary of the data was described (Table 1).
This included the mean, median, minimum, maximum, and standard deviation of the variables. For categorical variables such as colour and weather, basic summary was described by calculating the frequency distribution. Missing values were handled by imputation using the MICE package in R.
Ponds were compared in terms of variable variation using an unsupervised clustering technique to assess whether all ponds were similar or different. K-means clustering was applied; this is one of the most basic and often used unsupervised machine learning techniques to find underlying patterns by grouping similar data points together.
The silhouette method revealed k=2 as the optimal number of cluster centroids to do the analysis. Based on a clustering analysis, the data points revealed that the clusters formed from k=2 overlap with each other. This implies that all data points could be regarded as one cluster and there was not a single pond that behaved differently as a separate cluster (Figure 1). For instance, 68 of the 119 data points in pond 2 formed with one centroid and 51 formed with the other centroid. A similar split between the two centroids were found for the data points of all other ponds. Therefore, analysis was performed on all data points collectively.