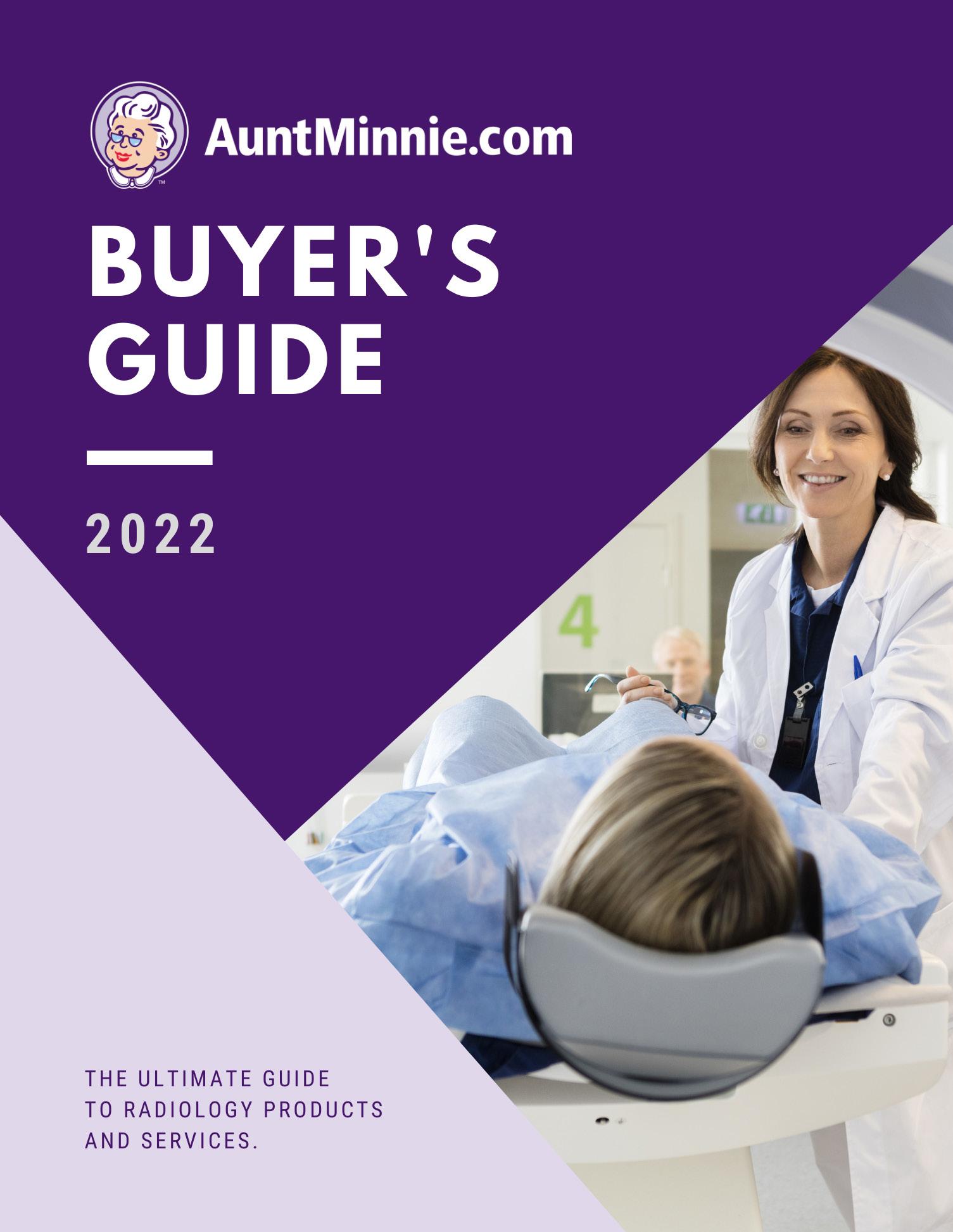

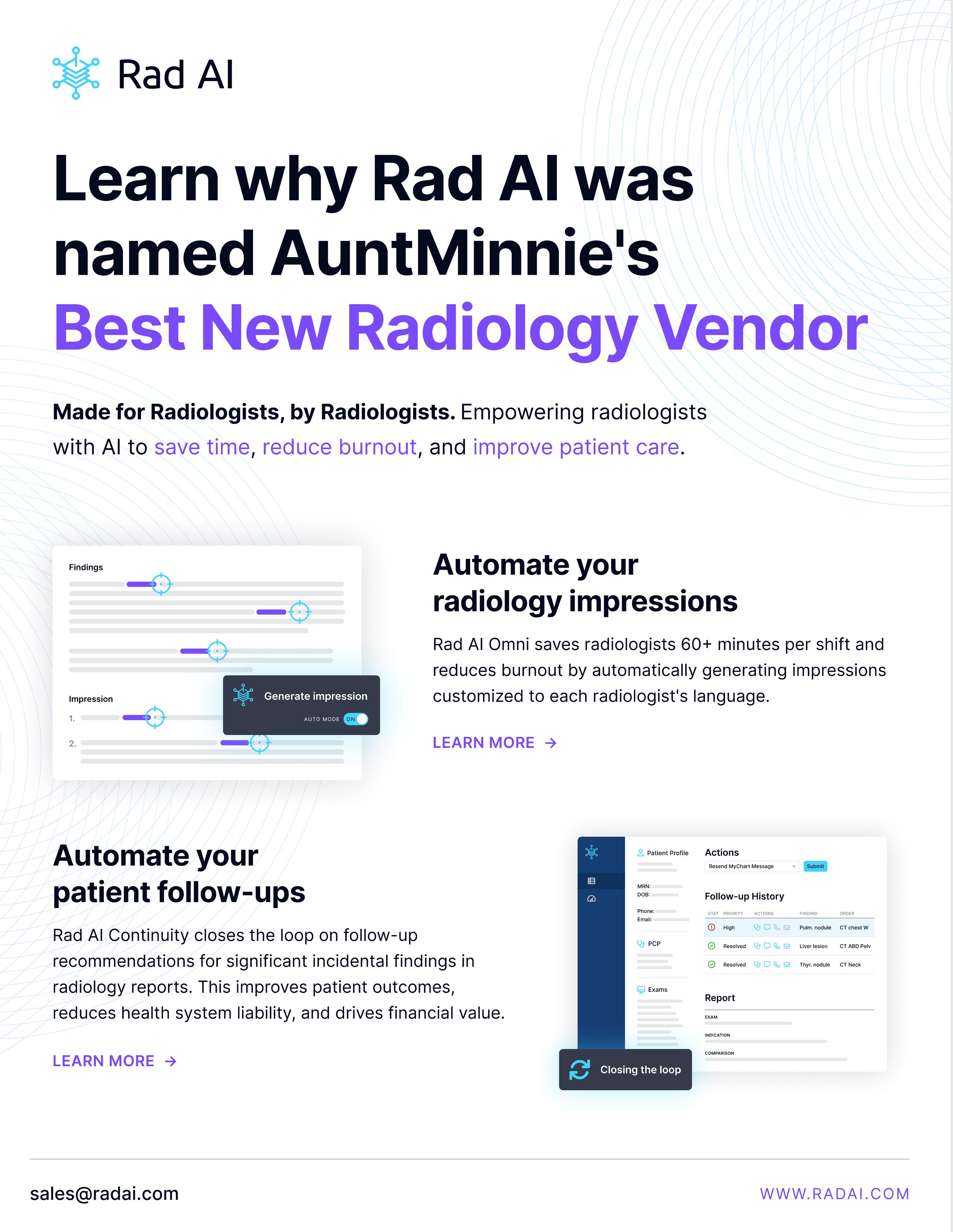
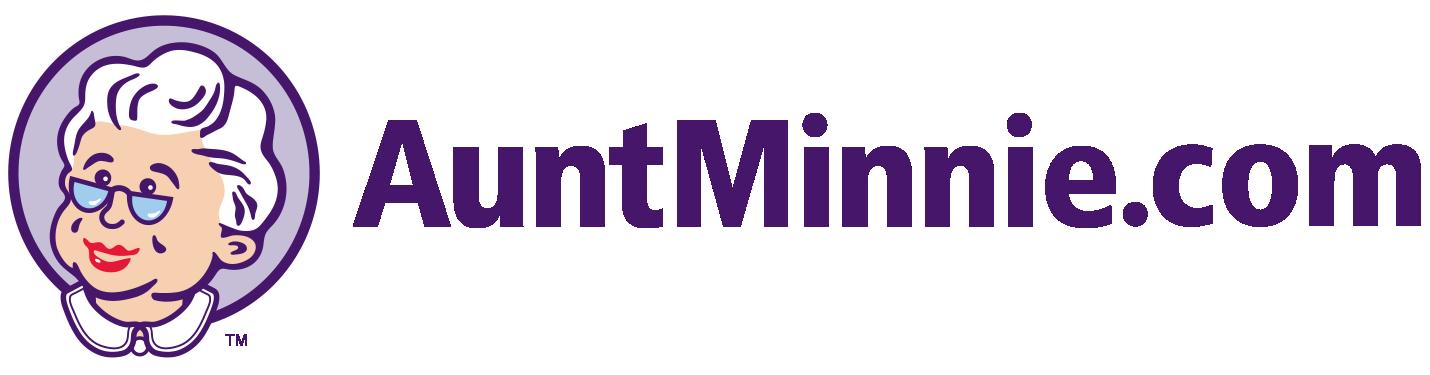
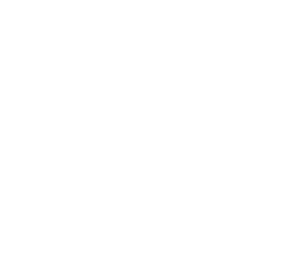
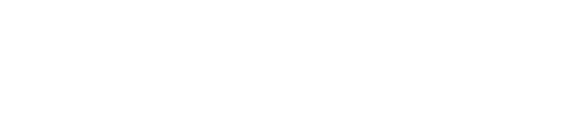
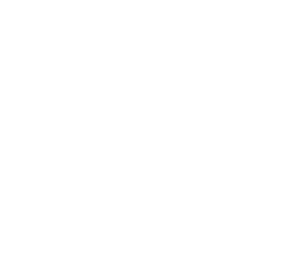
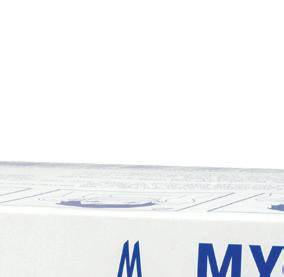
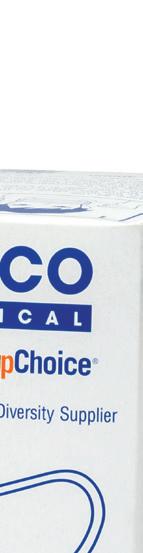
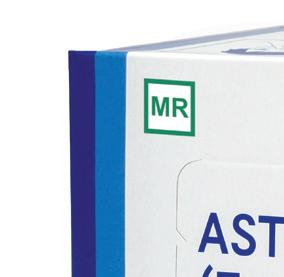
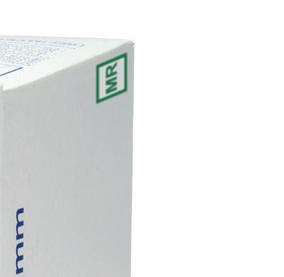
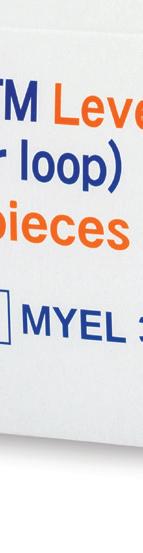
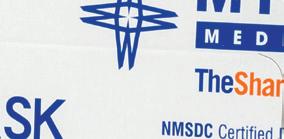
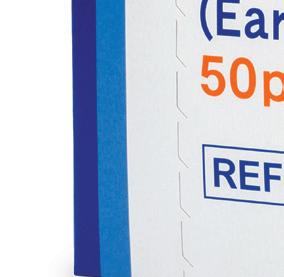
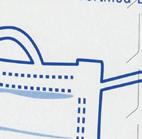
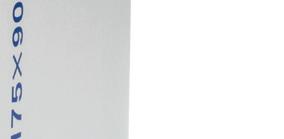
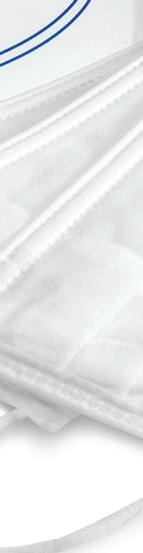
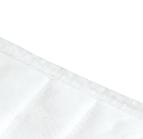
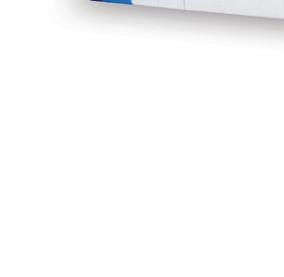
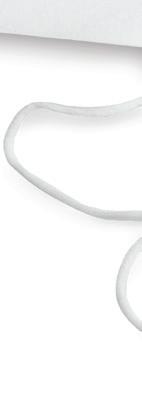
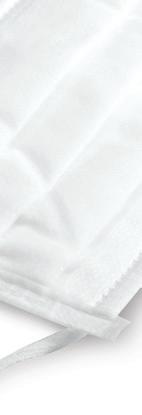
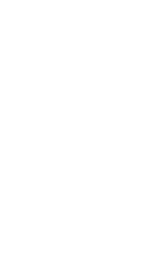
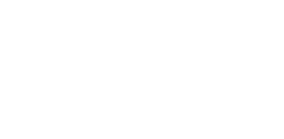
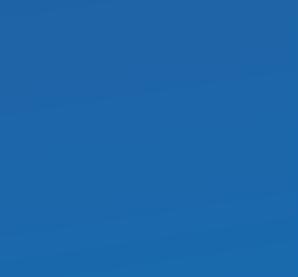
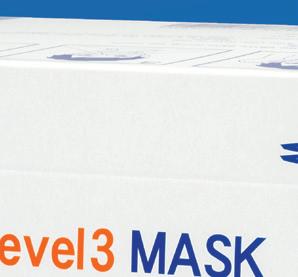
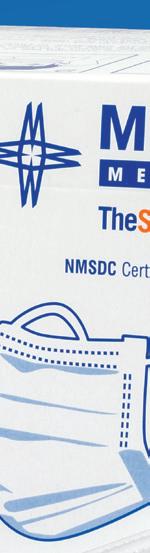
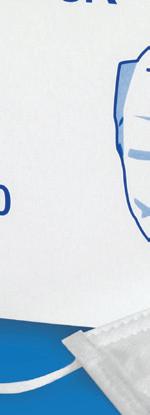
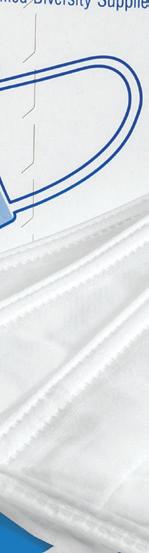
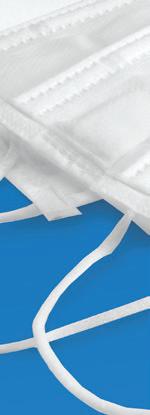
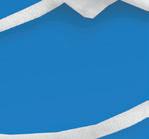
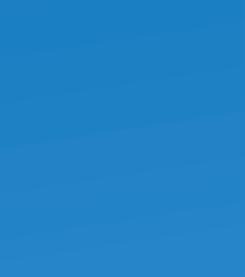
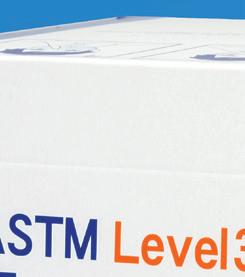

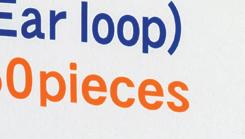
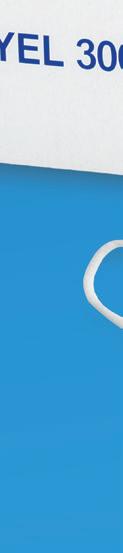
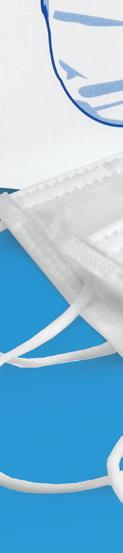
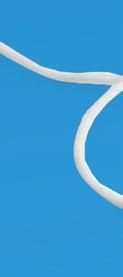
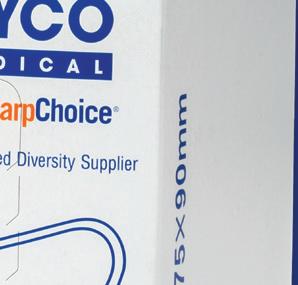
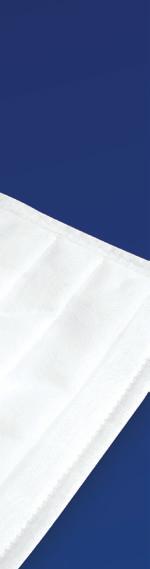
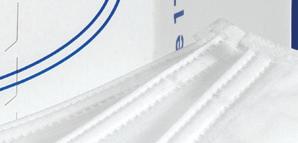
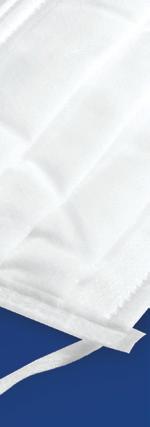
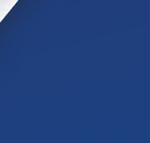
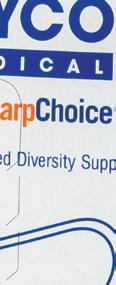



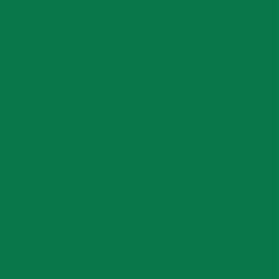
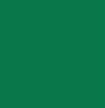
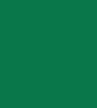
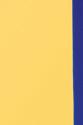
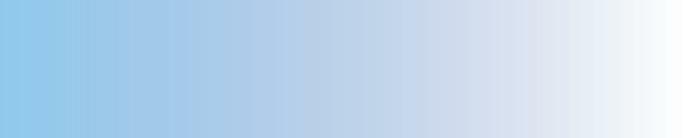
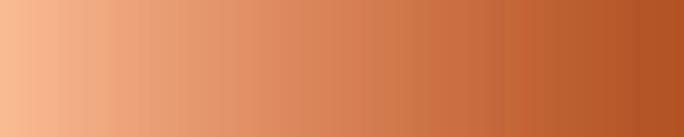
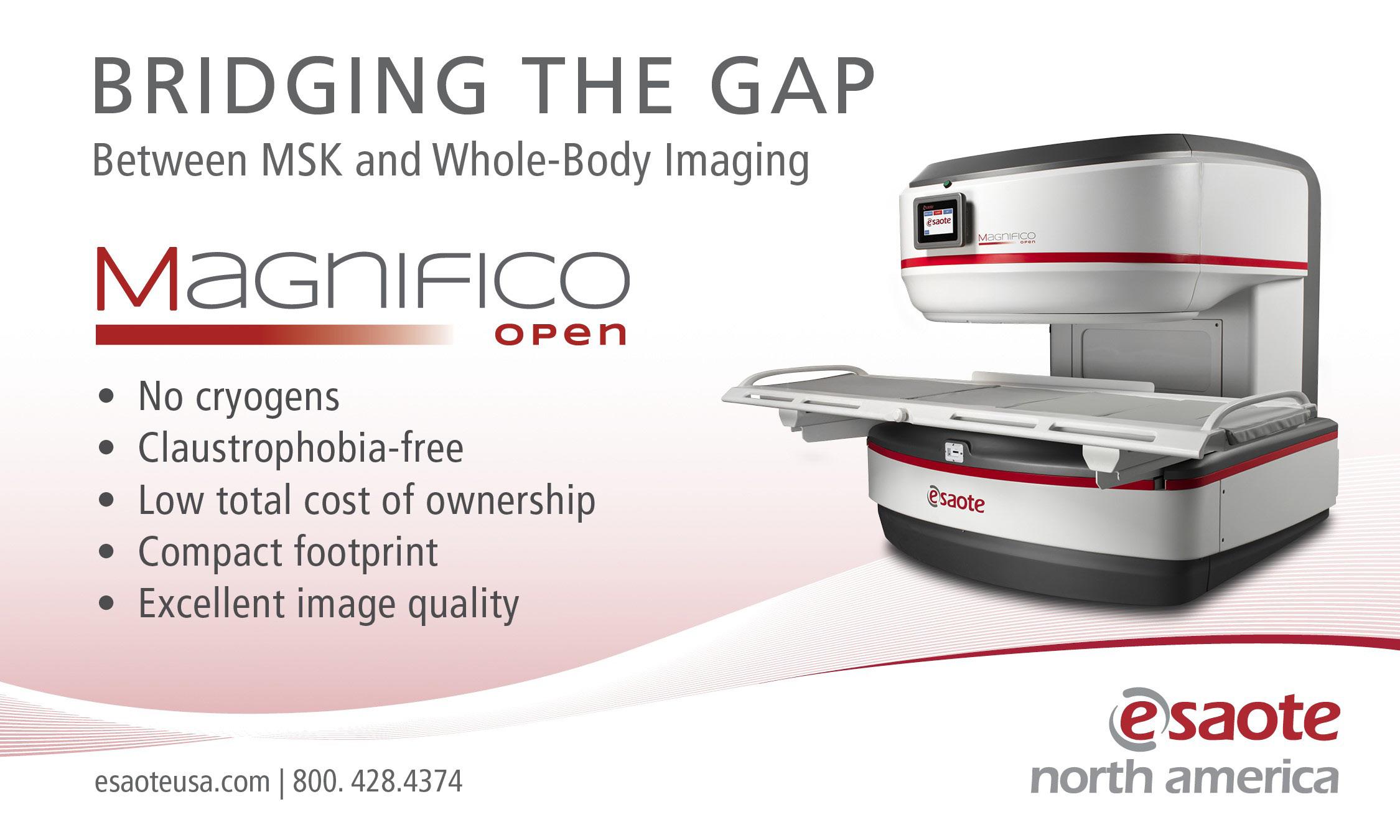
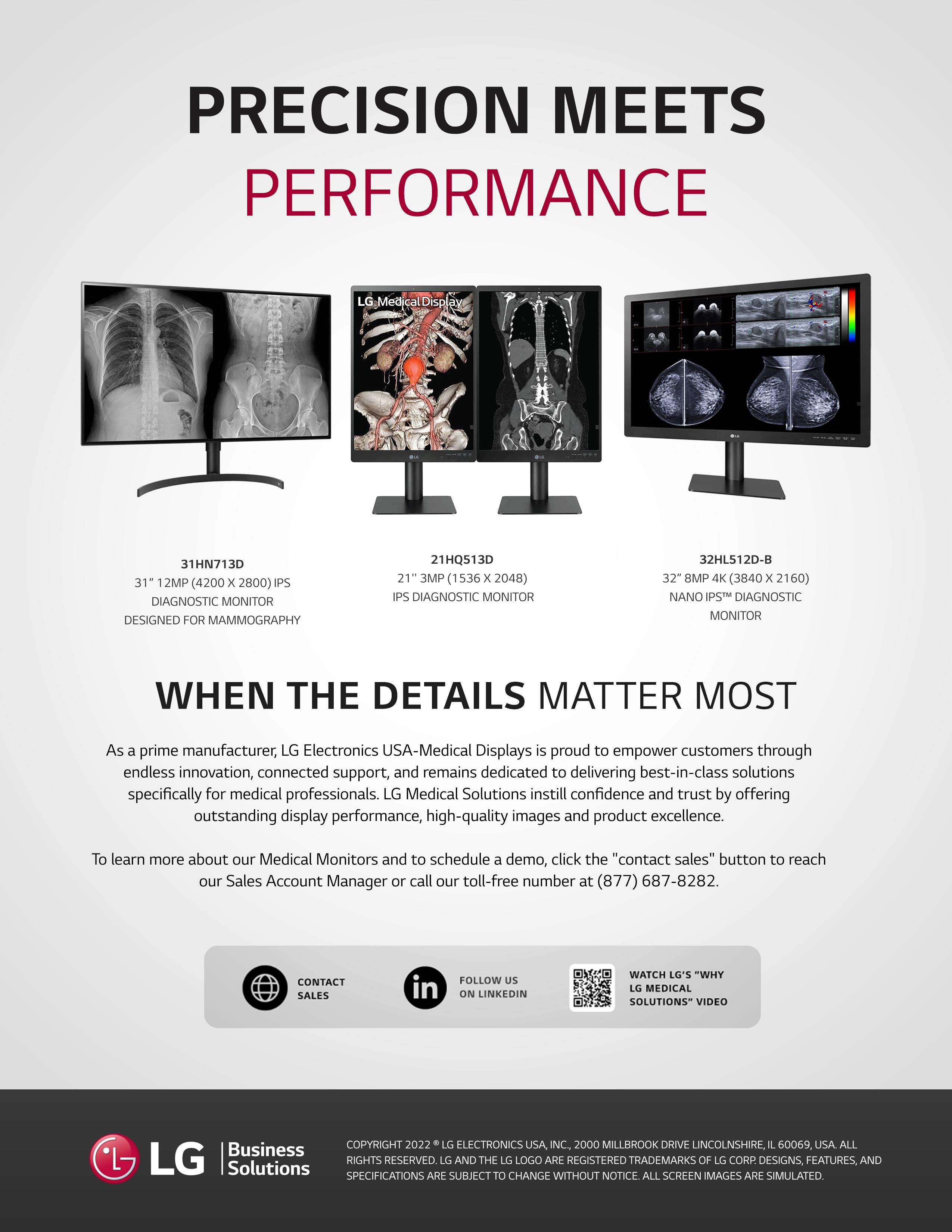
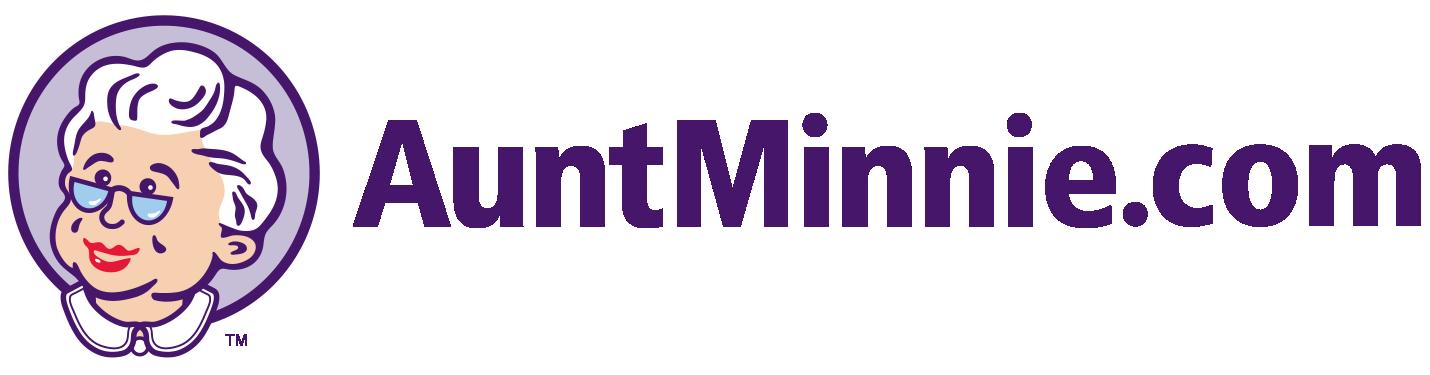
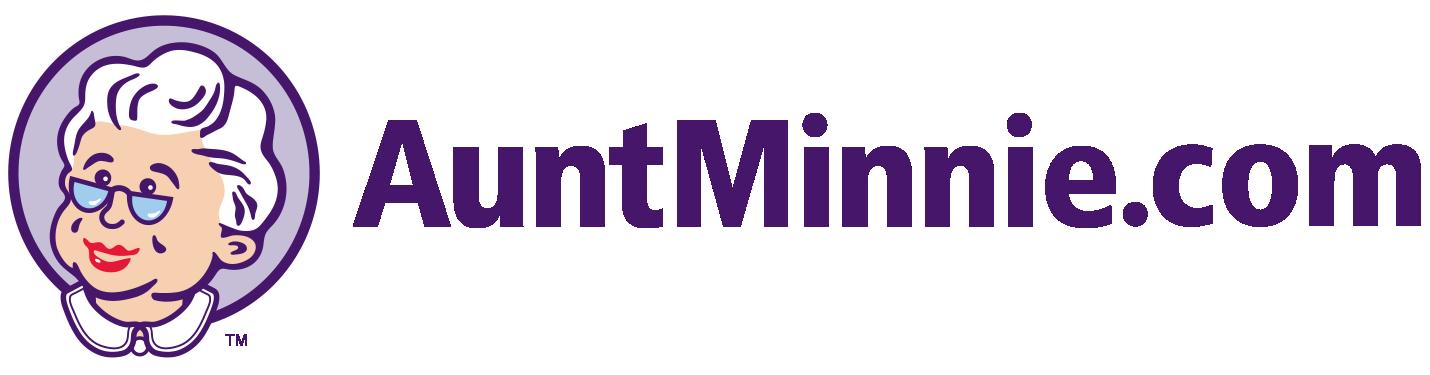
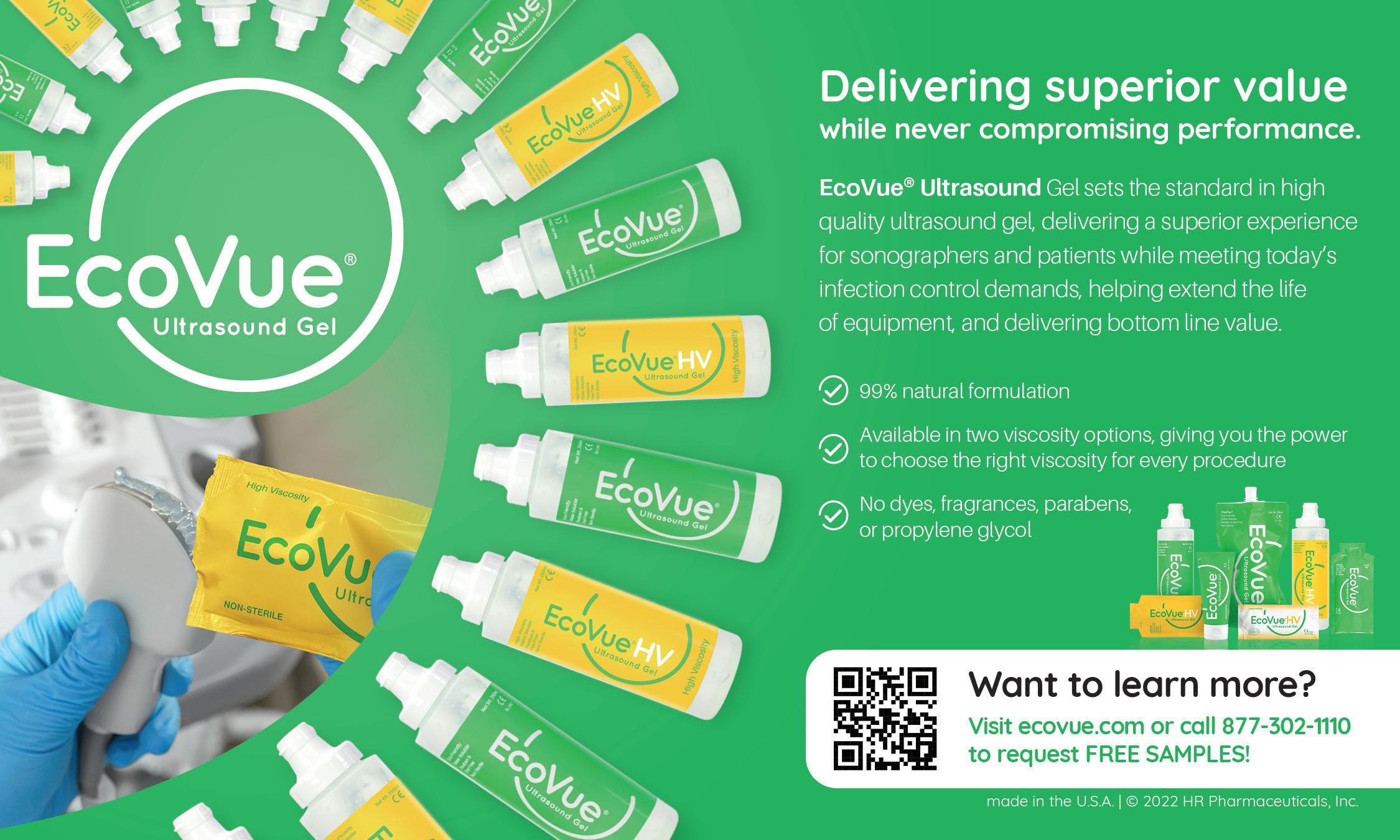
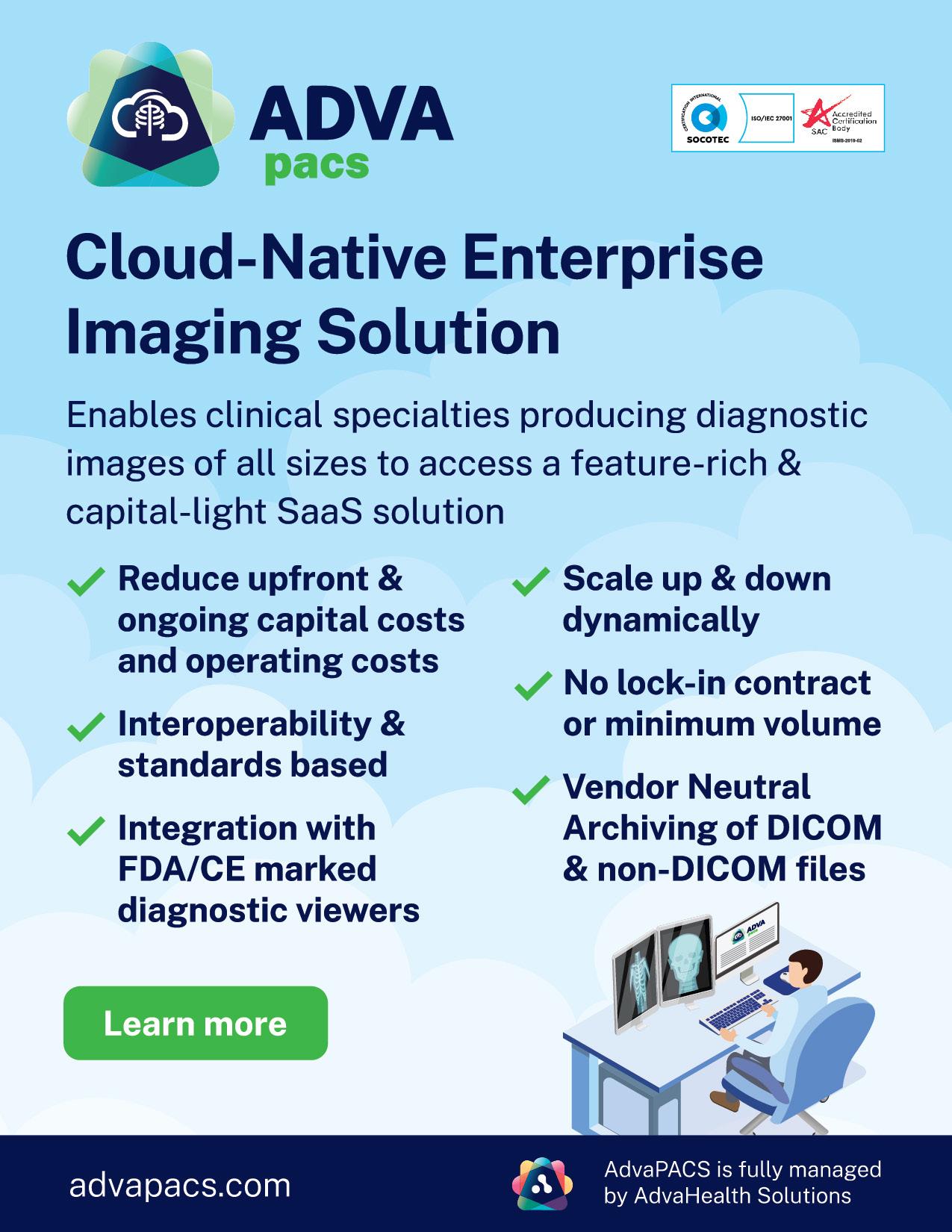
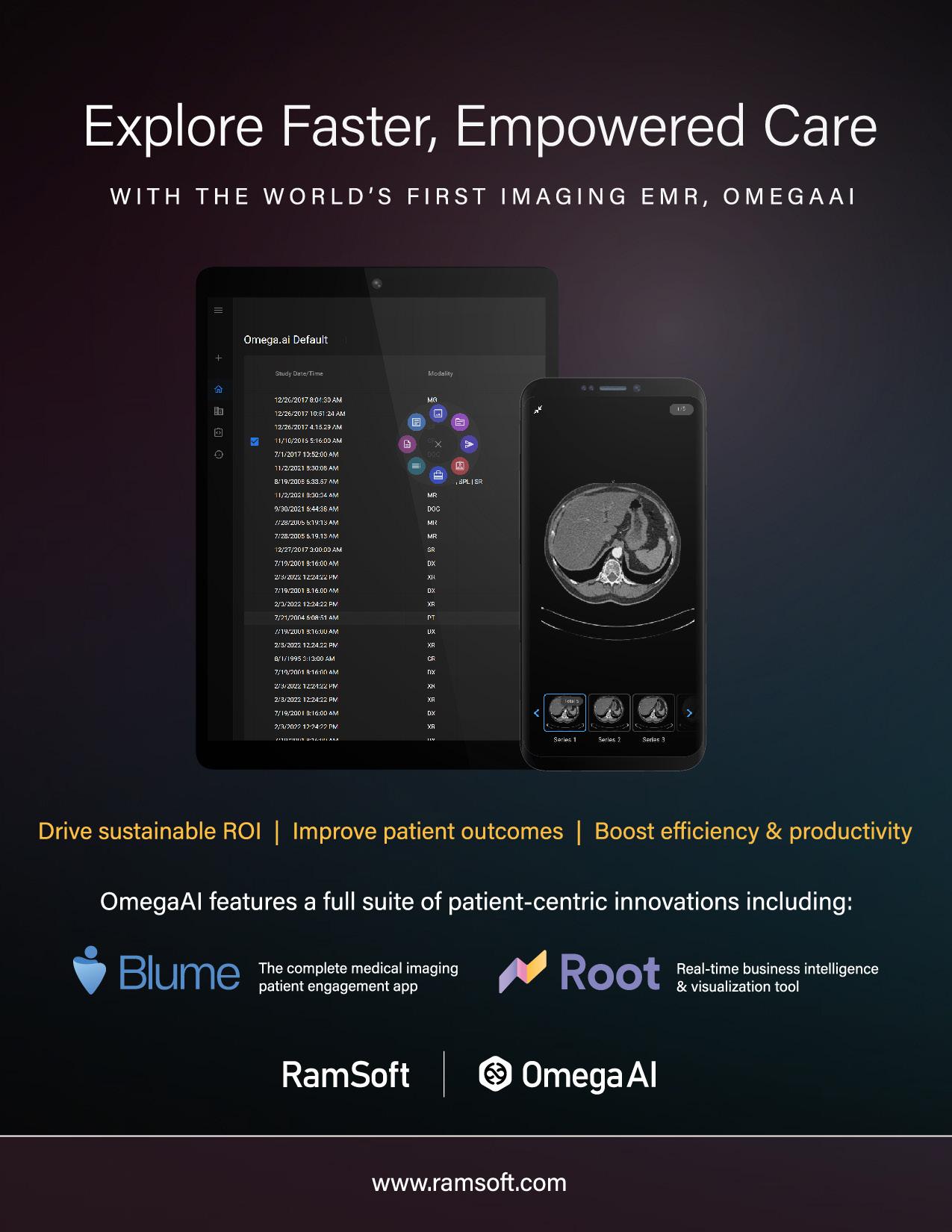
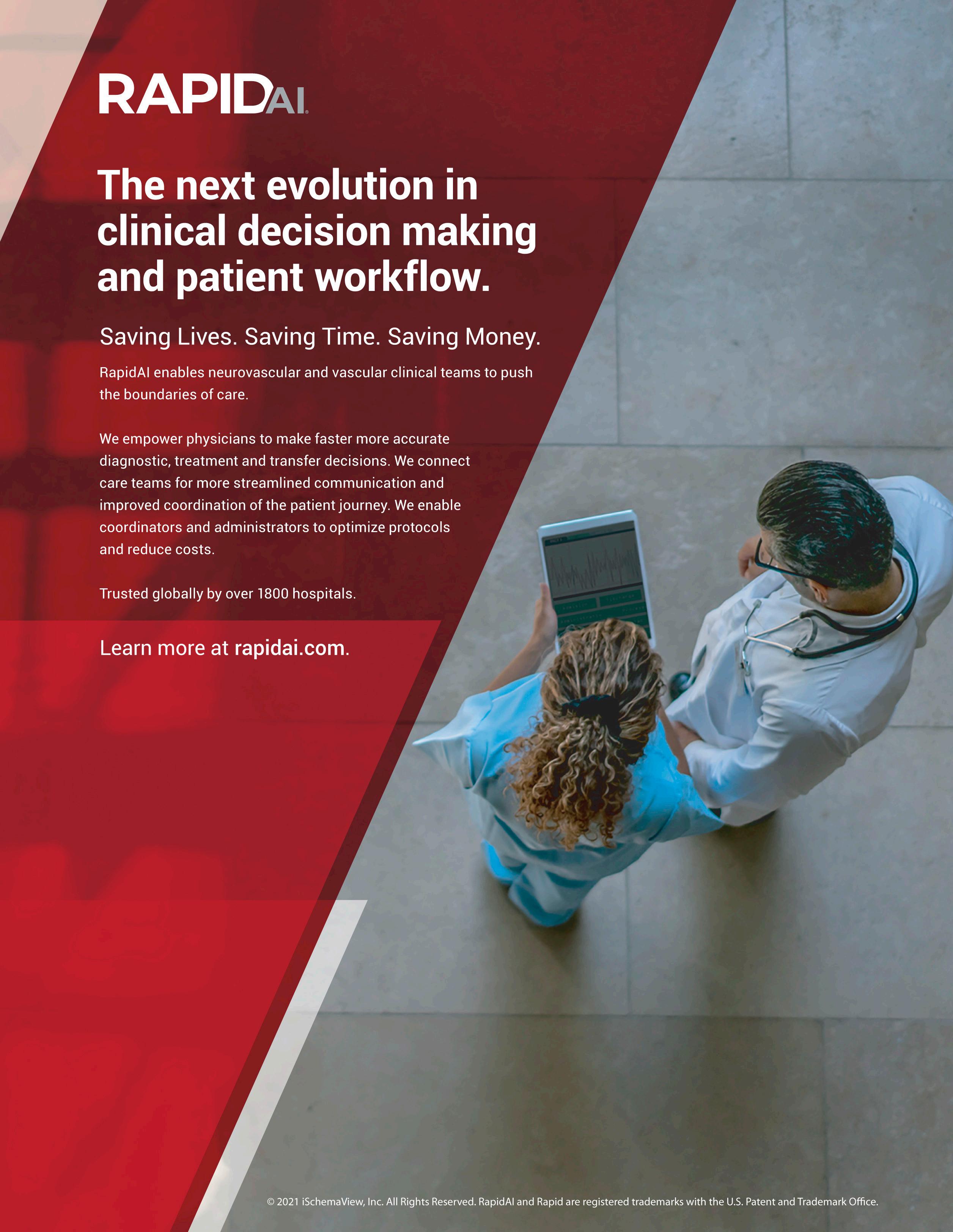
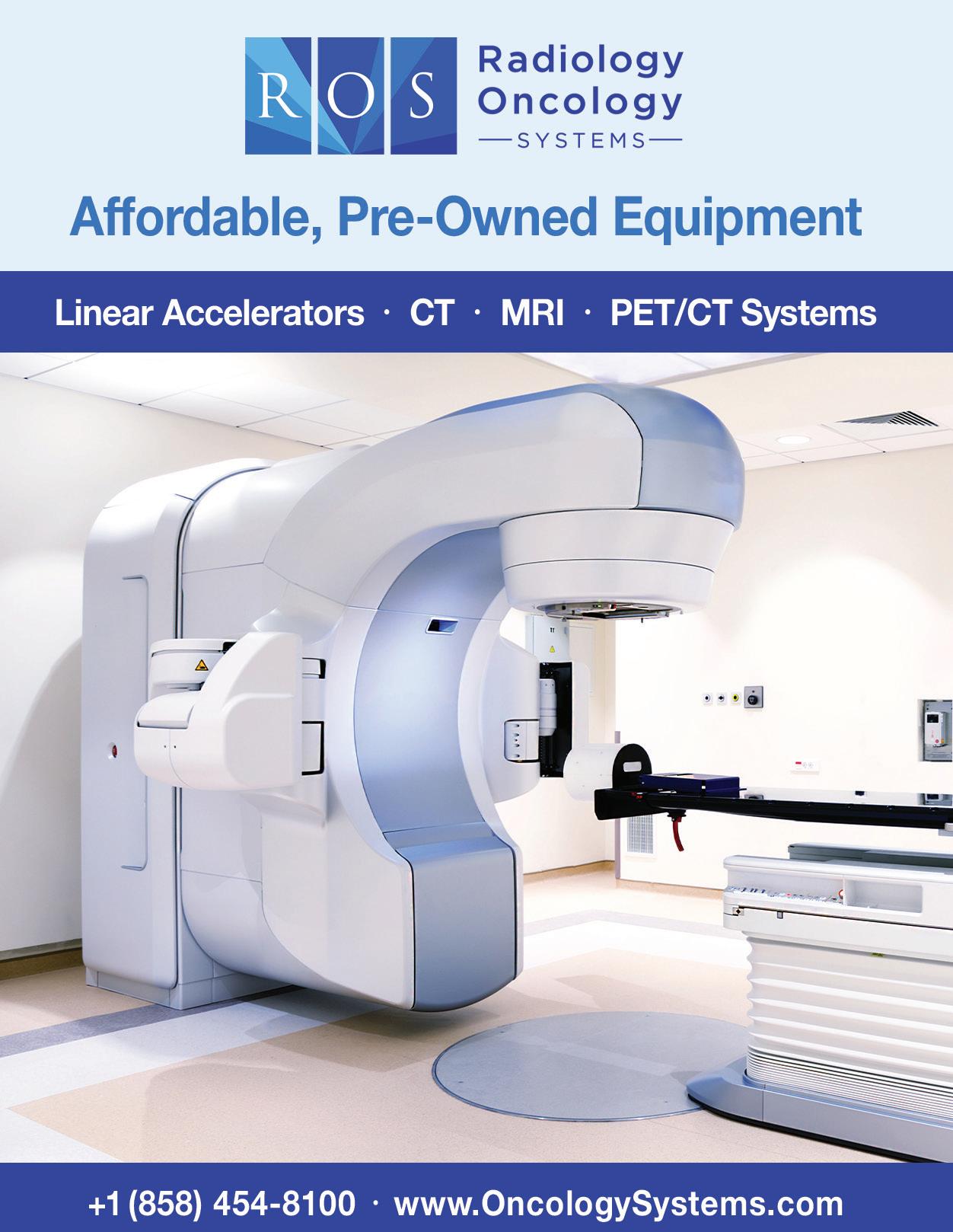
CHICAGO - A deep-learning model that predicts the 10-year risk of death from heart attack or stroke by using a single chest x-ray will be highlighted November 29 at the RSNA annual meeting in Chicago.
A team led by Dr. Jakob Weiss from Massachusetts General Hospital found significant associations between the risk predicted by the model and observed major cardiac events in their study of over 147,000 chest x-rays from more than 40,000 participants.
“As chest radiograph images are commonly available, our approach may help identify individuals at high risk for cardiovascular disease, prompting risk factor assessment and targeted prevention,” Weiss and colleagues wrote.
Current guidelines recommend estimating 10-year risk of major adverse cardiovascular disease events to find out who should get a statin to lower cholesterol for disease prevention. Risk scores used for atherosclerotic cardiovascular disease assessment take into account patient age, sex, race, systolic blood pressure measures, hypertension treatment history, smoking status, type 2 diabetes status, and a lipid panel. But these variables may not be available in a patient’s electronic record.
Weiss and colleagues wanted to test the potential of artificial intelligence (AI) in estimating
10-year cardiovascular risk from routine chest radiographs. They developed a deep learning model that was trained using 147,497 chest radiographs from 40,643 cancer screening trial participants and conducted independent testing in a separate cohort of 11,430 outpatients who were potentially eligible for intervention.
In the study, major adverse cardiovascular events occurred in 1,096 (9.6%) cases over a median follow-up of 10.3 years. The team found significant ties of risk predicted by the model and major adverse cardiovascular events in patients eligible for statin (hazard ratio [HR], 2.03; 1 is reference). This finding remained significant after adjustment for cardiovascular risk factors (adjusted HR, 1.63).
The researchers also found that in the subgroup where all variables necessary were available to calculate atherosclerotic cardiovascular disease risk, the model showed similar performance and added to the overall risk score (adjusted HR, 1.58).
The team concluded that deep learning could estimate cardiovascular risk from a routine x-ray image similar to the current clinical standard.
“This enables opportunistic screening to identify high-risk patients who would benefit from prevention with statins,” Weiss et al wrote in their abstract.
A new study from Philadelphia has proved that using an artificial intelligence (AI) system to send scheduling reminders to patients needing imaging follow-up significantly reduces compliance failure rate and potentially improves patient care and outcomes, delegates heard on November 29 at RSNA 2022 in Chicago.
The research group aimed to improve patient compliance rates for follow-up imaging recommendations by implementing a natural language processing (NLP) algorithm and tracking and reminder system that identifies patients requiring follow-up imaging based on radiology reports, organizes follow-up recommendations by due date, and reminds patients of due or overdue recommendations.
Historically, Philadelphia patients were notified of further imaging recommendations only via a letter mailed to their home, in line with Philadelphia legislation. This meant there were unknowns: Were patients actually aware of imaging recommendations and were the exams completed?
“Failure of the patient to comply results in delayed treatment, poor patient outcomes, additional unnecessary testing, lost revenue, and legal liability,” noted Dr. Jung H Yun, from the department of radiology, Einstein Medical Center, Jefferson Health, Philadelphia.
With this in mind, the American College of Radiology (ACR) launched the “Closing the recommendations follow-up loop” initiative, through systemic tracking and care coordination.
Yun’s group worked with Within Health to implement an NLP algorithm that automatically extracted radiology reports containing both the recommended radiologic examination and the recommended due date. The algorithm extracted patients’ numbers from the patients’ electronic record and sent SMS text messages. The software also included an automated tracking and reminder system that checked if patients scheduled and completed follow-ups. Patients were compliant with recommendations if exams were scheduled and completed within a period spanning 30 days before and 60 days after the recommended due date, a compliance range defined by the American College of Radiology.
Prospective analysis was performed on all outpatient diagnostic radiology reports, excluding mammograms, generated at the institution from July 1, 2021, through April 30, 2022. Inclusion criteria for outpatient radiology reports included the mention of imaging modality, body part, and due date for followup. Patients who unsubscribed to SMS, or who scheduled but did not complete follow-up, or whose follow-ups were not clinically indicated, were excluded.
Patients were divided into two groups, control, and intervention. In the control group there were no SMS reminder notifications until compliance date expiration date had passed. In the intervention group, the algorithm sent an initial SMS reminder three weeks after the original
examination with the recommended due date for follow-up examination, then reminders every two weeks at two weeks, four weeks and six weeks after the recommended date, unless the exam had already been scheduled or completed, until the period of ACR compliance had elapsed. A final notification was sent at compliance expiry date.
The researchers then measured adherence rate in both groups and compared the two. Statistical significance was set at p = 0.05.
There were a total of 275 radiology reports, 116 in the control arm and 159 in the intervention group. In the control group, 63 (54%) patients complied with follow-up, while 53 (46%) didn’t.
In the intervention group 111 (70%) patients complied with follow-up while 48 (30%) didn’t. The intervention group compliance was higher than control group compliance at 70% versus 54%. Compliance rate comparison was statistically significant at p = 0.08.
The study had several limitations including small sample size at a single institution, no verification of active or inactive phones, and no further reminders if appointments were scheduled. “As we continue to work with this AI software in the future, there are some considerations... we should communicate with patients a little earlier and send them reminders even before the start of the ACR compliance range. We also want to send more reminders even if the exams are scheduled until they are actually completed, and incorporate other modes of communication such as email and integration with the patient portal. Finally, we want to involve more patients in our study which could include inpatients and ED patients,” Yun said.
November 11, 2022 -- A deep-learning model can generate “super-resolution” synthetic brain MR images from portable MRI scanners, enabling automated quantitative morphometry on these low-field-strength systems, according to a study published November 8 in Radiology.
In a proof-of-concept study, a group led by Juan Eugenio Iglesias, PhD, of Massachusetts General Hospital in Boston trained a convolutional neural network (CNN) to synthesize images with 1-mm isotropic spatial resolution images from lower-resolution T1weighted and T2-weighted brain images generated on a 0.064-tesla portable MRI scanner. In testing, the automated morphometry measurements on the synthetic images correlated highly with measurements on actual 1-mm isotopic images from high-field strength 1.5- to 3-tesla scanners.
Portable, low-field-strength MRI has the potential to transform neuroimaging in a point-of-care setting. For example, depicting intracerebral hemorrhage and multiple sclerosis lesions, according to the authors. However, it’s limited by low spatial resolution and low signalto-noise ratio compared with high-field-strength MRI.
Interest has grown in artificial intelligence (AI) approaches that might help augment image
quality to extract more information from scans while maintaining accuracy. Morphometry of low-field strength MRI would substantially extend its use for research and clinical neuroimaging, scientists say.
In response, Iglesias et al developed LF-SynthS, a technique for training a CNN to generate synthetic images from low-field-strength image data. To test the model, they utilized automated segmentation tools on both the synthetic images and actual images acquired on high-field-strength systems in 24 patients who had presented with neurologic symptoms.
When the researchers compared the quantitative morphometric assessments on each type of image, they found high correlations between the synthetic images generated by the algorithm and actual high-field-strength images. Volumes correlated between synthetic image datasets based on low-field-strength MRI at 0.064 T and high-field-strength T1-weighted MPRAGE datasets for multiple brain regions, even for smaller regions that are difficult to segment (e.g., the hippocampus: r = 0.85; p < 0.001).
In an accompanying editorial, Dr. Birgit ErtlWagner, PhD, and Dr. Matthias Wagner of the Hospital for Sick Children in Toronto noted that the algorithm is an exciting technical development.
“Future studies with larger sample sizes and a variety of brain pathologic findings will be needed to demonstrate the clinical value of the method and to ascertain those pathologic findings in the brain will not be filtered away or artificially created,” wrote Ertl-Wagner and Wagner. “Nevertheless, this technical development study demonstrates the potential to go low on field strength and aim high for spatial and contrast resolution using artificial intelligence.”
At Canon Medical, we believe in providing innovative solutions that enable you to enhance your business and patient outcomes. We do this by investing solely in research and product development that directly addresses your specific patient and business needs to help improve life for everyone. In addition, we strive to improve accessibility, and cross platform availability to address your specific patient, business, and cyber security needs.
See how Canon Medical is striving to enhance clinical confidence, create true patient-centric designs and endeavor to be your trusted partner. Visit Canon’s Virtual Gallery