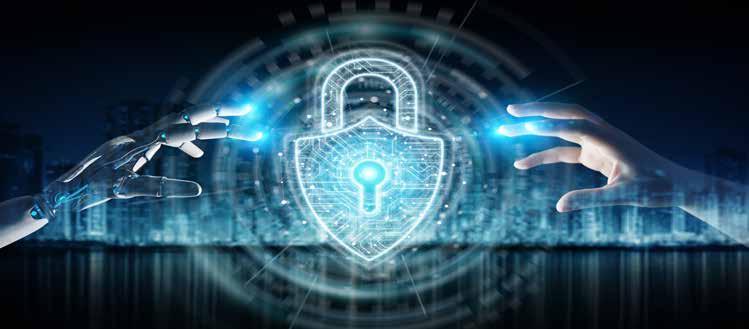
9 minute read
The future of Artificial Intelligence in Security
Deep Learning, along with huge increases in processing power and data gathering capabilities, is bringing new intelligence to the ways security is performed, from threat anticipation to robot and drone patrols.
The idea of machines that could act and think like men has been around for a long time. In Greek myth, Talos was a giant bronze man who guarded the island of Crete by throwing stones at the ships of unwanted visitors, acting as the very first security guard. The earliest computers were designed as “logical machines” that reproduced human capabilities such as basic arithmetic and memory, their engineers essentially seeking to create mechanical brains.
In AI, the goal has long been to create devices that would think like human beings, act like them, or both. As technology progressed, researchers in AI concentrated on mimicking human decision-making processes to carry out tasks in ever more human ways. To do that, they needed to incorporate one of humanity’s most fundamental characteristics—the ability to learn.
Now, 60 years after Arthur Samuel created perhaps the world’s first successful self-learning program, Deep Learning systems are at the cutting edge of AI and are beginning to have a profound effect on security systems and protocols.
Predicting the future with AI
At its core, Deep Learning relies on data. This data is fed into neural networks that mimic the way people think and understand the world. These networks also hold a number of advantages, such as speed, accuracy and lack of bias. And their capabilities have the potential to be huge. For example, researchers at MIT have created a system which can technically predict the future, albeit in a currently limited way.
For the security sector, being able to predict how people might behave is incredibly valuable. Human beings have always possessed this capacity, but historically computers have only been able to utilise data which already exists. Predictive deep-learning algorithms, such as the one being utilised at MIT, point the way to AIcreated simulations of ever greater accuracy.
Deep learning works on a system of probability. The neural network is able to make statements, decisions or predictions with a degree of certainty, based on the data fed to it. A feedback loop, which either senses or is told whether its decisions are right or wrong, modifies the approach it takes in the future. In this sense, it is able to learn. Where CCTV can only record a crime as it’s being committed, neural networks may be able to anticipate that crime before it occurs.
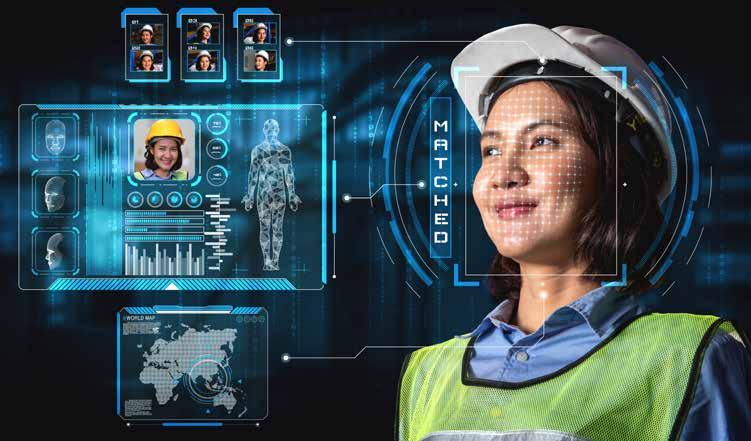
If The Face Fits
The rise of hyper-accurate facial recognition software has been at the foundation of today’s security protocols, and for many, facial recognition is the very definition of AI.
In the wake of a mass shooting that resulted in ten deaths at a Texas high school in 2018, the School District contracted with a company called AnyVision for an artificialintelligence-based application that plugs into an existing camera network. Soon the system began recognising people based on 20-yearold photos, or when they were wearing hoodies or glasses.
For the district’s director of technology, Kip Robins, the system’s capabilities were demonstrated when it was asked to search for one of his twin sons. It picked the boy out of a crowded hallway before Robins himself did. “I had to look twice and realize, ‘This is my son,’ he says. “I didn’t pick it up, but the software picked it up.”
Another company, Evolv Technology, offers portable screening machines with facial recognition software that can process between 600 and 900 people per hour, a minimum of one per second. The software connects to a database containing approved profiles of VIPs, employees, ticket holders for events and repeat patrons who should be automatically allowed entry to a venue.
The system’s algorithms can match the faces of attendees with those of persons of interest. If the visitor sets off a red light, they are blocked from entering and apprehended. If the visitor profile triggers a yellow light, indicating an unverified threat, security personnel can send the profile to central monitoring for realtime review and verification.
When facial recognition is aligned with sensors that can detect the presence of physical objects, the potential for threat detection increases dramatically. Evolv offers a separate screening device that alerts operators to concealed metallic and non-metallic explosives, firearms and other weapons. Inductive sensors are used to detect metals using an electromagnetic field, while capacitive sensors detect objects that have a dielectric constant that is different from air, such as plastic, paper and wood. Each can be used to determine whether an individual is carrying a dangerous object, even if it is not visible.
Spot the potential threat
Though sophisticated, the systems discussed above are primarily detection systems. They can see a previously identified threat—a person on a watchlist, an explosive device— and report it to security personnel. For AI to live up to its promise, it must be able to anticipate potential threats. Equally important, it must be able to distinguish these from false positives.
The MIT deep-learning algorithm uses a method called adversarial learning, wherein two neural networks — one that generates video and another that attempts to discriminate between real and generated videos — try to outsmart each other. While researchers hope to scale up this technology, currently the videos are less than two seconds long and begin with an easily predictable scenario, such as a train on a track. Meanwhile, predictive AI technology is already in use on a large scale in some countries. In Hong Kong, HD cameras, facial recognition and remote sensors gather huge amounts of data, while algorithms analyze events in real-time and indicate potential risks.
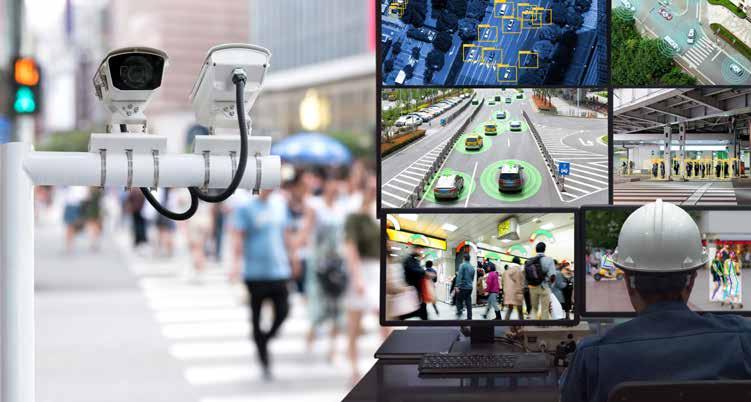
These processes may be utilised in both public and private security applications. For the home, a US company called Deep Sentinel offers a system that integrates wireless cameras, predictive AI, and human intervention to identify potential threats.
Deep Sentinel uses a combination of motion detection, human-body detection and facial recognition technologies. When motion is detected, the system begins to capture and record images. The deep learning algorithms determine if the presence is that of a human, animal or another moving object.
If the movement is from a person, facial recognition algorithms can determine whether that person is the homeowner or family member. Predictive technology identifies patterns of behaviour and can determine whether the person’s actions are suspicious. If the actions continue and the person is not recognised, the system alerts Deep Sentinel’s human surveillance team, who can then identify and act on the potential threat.
For larger commercial and infrastructure sites—electrical substations, oil and gas facilities— systems like the one offered by Digital Barriers can integrate multiple sensors, such as seismic ground sensors, wireless optical and thermal cameras, and video analytics to give users a single view of the area surveyed. The system is trained to ignore environmental effects like poor weather, camera shake, moving foliage and shadows, while differentiating between intruders and legitimate staff and visitors.
Though highly sophisticated, such systems ultimately rely on human judgement to determine whether a threat exists, and what action to take. But they can greatly reduce the man hours required to monitor premises both physically and remotely and represent huge potential for reducing labour costs.
Some companies might wish to entirely remove the human component from security processes, relying solely on artificial intelligence to identify and respond to threats. Currently, the greatest challenge in using analytics-powered surveillance alerts is the occurrence of false alarms. Over time, deep learning protocols will continuously increase accuracy of detection and greatly reduce the instances of false positives. In terms of physical surveillance and response, the use of drones and robots will become more and more frequent.
These are the drones you’re looking for
Remotely operated robots have long been used for such dangerous tasks as bomb disposal. The new generation of unmanned ground, aerial, and underwater vehicles will be capable of learning to navigate their environments while performing surveillance, reconnaissance or clearing operations.
Contracting to the US military, Shield AI offers Hivemind Nova, a quadcopter-type drone driven by Hivemind, a machine learning application that allows the robot system to learn from battlefield experiences. The Nova enables defence and security personnel to access and explore building interiors, urban areas, caves, tunnels, other high-threat environments, and GPSabsent areas to collect information about potential threats.
While still requiring a human operator, the drone learns while in operation, collecting data automatically with no risk to personnel. The machine learning also enables the system to teach and work with other robots to complete missions faster and cover a wider area, according to the company.
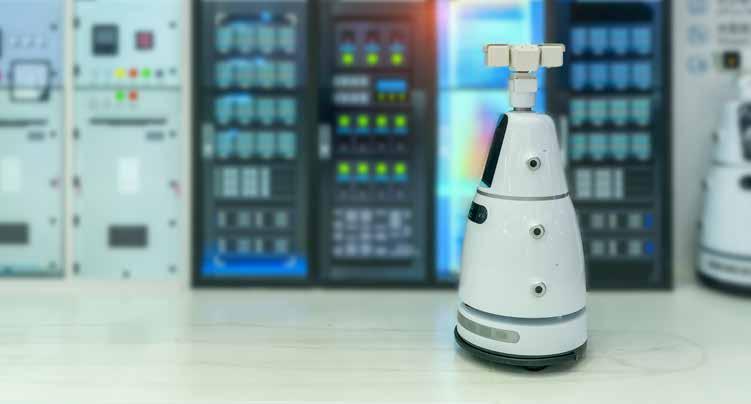
Security robots such as those built by Knightscope are used for groundbased monitoring, with the ability to provide 360º HD video streaming, detection of people, facial recognition and automatic licence-plate recognition. The units are designed to present an unthreatening appearance whilst carrying out their scheduled patrols.
While the above systems are designed for data collection and transmission, the use of autonomous or semi-autonomous machines highlights the greatest potential danger when employing AI for security applications. A security system can be “trained” to recognise possible threats, and potentially to act on them, but humans will always need to be involved in the process. Eliminating human decision-making from physical security operations has the potential to cause catastrophic incidents, for individuals, businesses and the growth of AI itself.
Questions of safety, privacy and personal data security are intrinsic to discussions about AI in security. Yet currently, there seems little in the way of pushback from the public. According to Paul Chong, chief executive of Singapore-based security company Certis Group, people trust their information is being collected for the right reason. “So long as people see that their information is not abused, they’d trade it for security, they’d trade it for convenience, they’d trade it for a lot of other things.”
The future of AI is now
Artificial intelligence has always been a future-based concept. What will it be capable of a year from now, or a decade? IBM’s Deep Blue computer beat chess grandmaster Gary Kasparov in 1997. In 2011 the same company’s question-answering system, Watson, won the quiz show “Jeopardy!”. Ten years on, AI is involved with ever-increasing aspects of our daily lives.
We have seen that for the security sector, advances in machine learning are making big changes in the way the industry operates. Advance video analytics is already in practice in many organizations. AI eliminates the need for pre-programmed algorithms, allowing sensor technology available today to capture an incredible amount of metadata in real time. Cameras can specifically identify people and licence plates – information that can be instantly cross-referenced to alert security personnel of potential threats.
Operational efficiency is being increased, as detection systems learn to discount objects and artifacts which do not represent a threat, saving human operators valuable time. Smart security solutions, such as video management systems or networked access control and visitor management can now take the data being collected and correlate it with patterns of behavior for employees and visitors to a facility.
Intelligent machines can take over dangerous or routine tasks, keeping security personnel both safer and more productive. AI-driven algorithms can help security officials make split-second decisions in the event of an incident, taking the guesswork out of answering alarms by determining which events require a call to law enforcement and which are false alarms.
For all of these advantages, however, it is clear that whatever advances occur in AI, it will never remove the need for human intuition and judgement when making security decisions. People are innately unpredictable. Suspicious people can act innocently, just as innocent people may seem suspicious. It takes human intelligence to tell the difference.