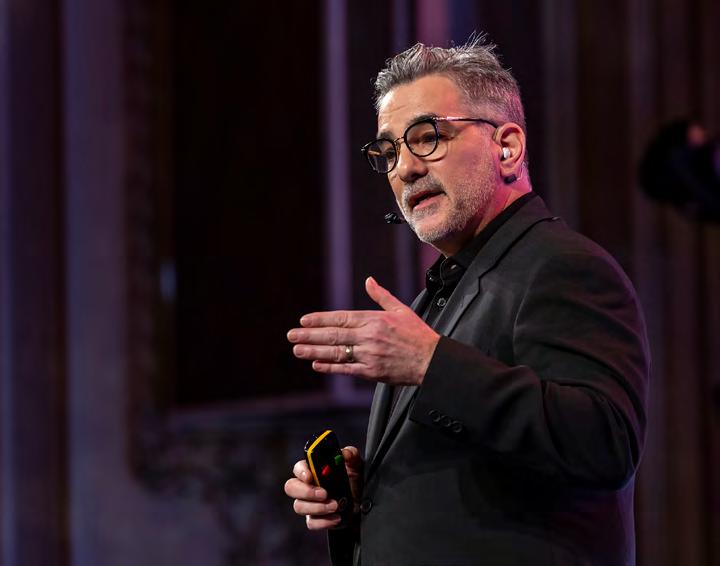
6 minute read
Artificial Intelligence is not magic
from BR/03/2023
He is the CEO of Trustwise, President of the Responsible AI Institute, and Founder of Qantm AI. He has ideated some of the most innovative AI strategies for a variety of Fortune 500 companies and designed and led cutting edge technology strategy initiatives with IBM. Trained as a human geneticist, he launched his career in tech as he was preoccupied with the rigor of the scientific method applied to business combined with his interest in human nature being described through data. At IAA’s Creativity4Better conference in Bucharest, BR had the chance to sit down with global AI expert Dr Seth Dobrin, to do a deep dive into the technology that’s making huge waves in the business world.
By Romanita Oprea
Advertisement
You say that AI is centred around humans—or at least that it should be—and we should acknowledge the fact that you have a lot of insight into the way humans themselves work.
I’ve always had this concept that everything I do should have a positive impact on humans and society. I don't know if that's just me as a person, as a human being, or if that somehow comes out of my educational training. But even in graduate school, I was in the field of psychiatric genetics. We would join physicians as they visited patients or performed surgery, in order to understand how what we were doing would or could impact humans and, more importantly, what it could mean for physicians and nurses. In my talks, I say that we need to consider the human that's going to be impacted by the AI and the human that's going to be using it—and this is a really good example. Patients are the ones that can be impacted by the AI, and the medical staff are the ones who are going to be using it.
What were the main challenges at the beginning, but also throughout your career in AI?
I think the biggest challenge that companies have been facing is that they’ve started these AI or data science programmes without a real strategy that's tied to actual business value. As technologists we often think, how many AI models have we built? How good are they? How well is my model performing on all these technical metrics or how many companies even measure AI? Because we should really be measuring it. How is it improving my revenue? How is it generating or saving me money and how is it making my company’s interactions with consumers better, cheaper or faster? The number one problem is we don't have it tied to real business and real metrics that matter for organisations.
The number two issue is that they don't consider the humans. Humans are an afterthought. How is this AI model going to interact with us as human beings? How are we going to present it? How is it going to be used? What's the real problem we're trying to solve for that human?
The third problem is the fact that trust is also often an afterthought, as we tend not to think about how we're going to implement this in a trustworthy and responsible manner and explain it to humans until it’s too late.
And finally, safety, robustness, accountability, and consumer protections are often not examined upfront. Doing that early on starts with having an understanding of the human and having this human-centred approach that I talk about to building AI, where you actually sit down with people and understand the problems they need to solve, how you can solve those problems, and how you are going to implement that. So, what’s going to be the interface we’re going to use to make someone’s life better using AI?
So, it comes down to educating the client and talking to them prior to starting a project.
Yeah. I mean, oftentimes, leaders hear buzzwords and they want to start using them, right? Five years ago, it was deep learning. Every customer I talked to wanted to use deep learning. That’s great technology to solve certain problems. But most problems are easier to solve. Let's keep it simple! The simplest solution is always the best solution. It's important to not just pursue the shiny objects, but to use the right tool to solve the right problem. And so, I think that’s something that we can do as leaders to get better outcomes.
Do you think that AI is somehow being seen as a sexy version of science right now, and maybe that’s why so many are attracted to it without actually knowing too much about it?
I don't think so. I think companies that have been successful at implementing it have generated a lot of value for themselves and their shareholders and made the lives of the humans they interact with better. And lots of other companies are seeing that. But they don't take a methodical approach to developing these AI systems. In fact, Monsanto, where I started really solving business problems, is held up as an example of a company that did this right. I stepped out of a very scientific role into a business role. And I didn’t understand the problems that were important to the supply chain, to my marketing team, my sales team or my customer success teams. I had to actually sit down with the business leaders and understand their problems, then map out the decisions we were going to tackle with AI. Then I had to translate those into things that technical folks—the data, data science, machine learning, and software development people—could use to solve their problems. Since I didn't know the answers and I acknowledged that, I had to take this human approach. And that proved really valuable.
And by having those conversations, I also found out that all the business leaders cared about was how this was going to help them hit their business targets. How is it going to make money, save money or increase their NPS score? That's all they cared about. Therefore, we started measuring our programme in those terms and we were able to actually assign a dollar value: this is how much money we've made as a company. This is how much money we've saved, and this is how much our NPS has increased, which you can also correlate to how much money you're making or
People think AI is magic. You just create these teams, you tell them to go and make or save you money, and it just happens. But it's not magic. It needs to be directly tied to your business strategy. It needs to be directly tied to the KPIs, the outcomes that the business has promised to its shareholders.
Another related thing is the fact that we often think AI is biased. It’s just math and computer science. Math itself is not inherently biased. What's happening is the math is learning the biases from the bad decisions humans have made in the past. And so, if the math itself is not biased, it's merely picking up on the biases we have propagated around the world. We have a choice. We can allow that to happen, allow AI to accelerate and propagate those biases and bad decisions we’ve made as humans, or we can use AI to help us make better, fairer, and more inclusive decisions, because we can monitor and control for those things and help humans present other humans with a slate of decisions that are all less biased than the ones they would have made on their own.
And how do you do that?
saving because it impacts customer retention and satisfaction.
What would you say are the top myths around AI and how would you debunk them?
I think the main one, specifically in terms of responsible AI, is that we need to eliminate all biases, period. We're never going to eliminate all biases, nor should we. What we should do is much like the approach the EU is taking to regulation: understanding the outcome. We're trying to understand humans, along with the biases or inequities we need to control for. AI will never eliminate all biases; the ones that should be eliminated are those that are relevant to the decision the AI is helping to make.
Over the last 3 to 5 years, we've developed algorithms that monitor data for specific biases that are called protected classes. They monitor the data—the output of the algorithms— for those protected classes. And you create thresholds, and you say, for example, for this mortgage decision, the balance between men and women getting a mortgage needs to be between 45 percent women, 55 percent men or 55 percent women, 45 percent men. If it deviates from that, the model cannot go into production. It cannot be used for decision making.
And you tune the model. There are these things called hyper parameters—think of them as knobs. You're tuning the equaliser on your radio to get the sound you want. You're tuning the performance of the model so that you get the best performance, the best business outcome, while minimising the biases that you don't want to see. That's how it is done. We use AI to monitor our models.