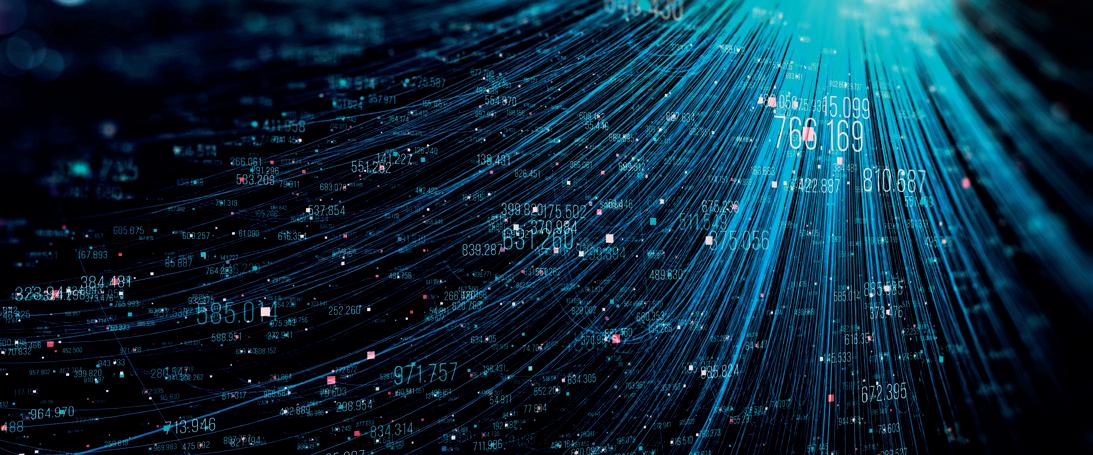
2 minute read
6 Future Perspectives on Scenarios
Scenario development is a constantly developing field of research. Scenario methodologies and applications have advanced substantially in the past decades, with further changes and growth to come. Two of the greatest changes anticipated within the insurance modelling community are the increased availability of data, and the potential for customised modelling supported by machine learning.
Traditionally, insurance scenario modelling has been dependent on the availability of structured internal data, produced on a pre-determined frequency (monthly, annually, quarterly etc.),100 and desktop research conducted by underwriters and staff. Alternatively, external catastrophe modelling approaches aim to understand potential future losses due to catastrophic, tail risk events, with synthetic loss events in the future. The ‘Fourth Industrial Revolution’ has spawned a new generation of data collection tools that presents an opportunity for the insurance industry’s use. Advanced data mining techniques have generated a substantial increase in the availability and frequency of large and unstructured data sets that can be used for real-time scenario analytics. Much of the data is third-party mined from digital platforms such as social media and is therefore used mainly as a rich source of data for insights into consumer behaviour.101
With an estimated 2.5 quintillion bytes of data being created daily, the insurance industry is increasingly using big data and machine learning techniques to analyse large and unstructured data sets which may aid the scenario development process.102 Opensourced data management tools such as Apache and Hadoop are increasingly being used by insurers to disseminate structured and unstructured data sets in short time horizons. This has aided in the development of real-time analytics in the insurance industry.103 Real-time behavioural data opens new opportunities in understanding behavioural risks that have been previously difficult to forecast. Consumer specific data also heralds the possibility for personalised scenario modelling, based on real-time monitoring and visualisation of consumer behaviour. Examples of customised data collection have been increasingly seen in auto and health insurances, which have been introducing personal monitoring devices to provide more accurate premiums to consumers.104
The insurance industry is lagging behind many other sectors in investment into AI and machine learning, with only 1.33% of insurance companies investing in AI in 2017 compared with 32% of internet and software companies.105 However, a survey by Deloitte in 2017 estimated that 95% of insurance executives intend to start or continue to invest in AI capabilities. Indeed, investment in AI in the insurance industry increased by 68% between 2011 and 2014.106 As investment in machine learning and AI tools continues to grow, scenario development will increasingly involve these tools to create more complex and comprehensive scenarios.