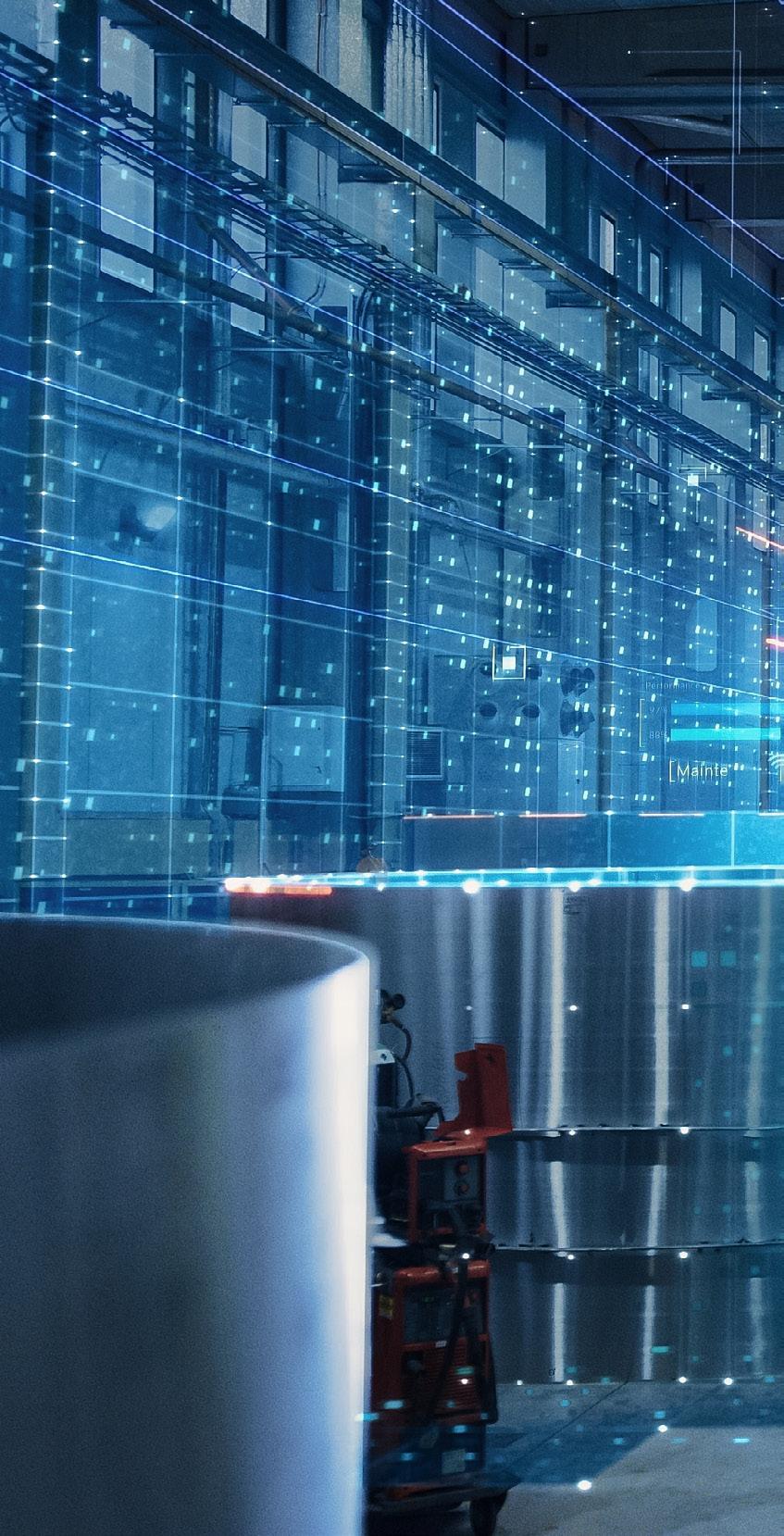
5 minute read
De-mystifying digital twins for oil and gas
In understanding our customers’ interpretations of digital twins, we found a range of concepts including engineering analysis of a physical component, predictive maintenance, performance optimization of processes, 3D virtual walkthroughs of a factory with automated operations, and everything in between.
What these ideas all have in common is that a digital twin consists of a digital representation of something in the physical world, is updated with live data, and drives business outcomes. With this backdrop, we define Digital Twin as follows: Adigitaltwin(DT)isalivingdigitalrepresentation ofanindividualphysicalsystemthatisdynamically updatedwithdatatomimicthetruestructure, state,andbehaviorofthephysicalsystem,todrive business outcomes
Per the above view, a digital twin has four key elements. The first is the physical system – meaning there has to be something real, something tangible. The second is the digital twin itself which is a model. In this case we do not just mean a computer science data model, but rather a behavior model such as a simulation or other response model to which you provide an input, and it returns back a response output. The third is the data connectivity between the two and this is the key to the definition of digital twin. The real-world operational data from the physical system is used to update the model on a regular basis as the physical system evolves. The data could be sensor data, time-series data, inspection data or any other data. Lastly, there must be a purpose for the digital twin. We always work backwards from the desired outcome because that determines which part of the physical system should be modeled, at what fidelity, and how often we need to update the digital twin model. These all depend on the specific use case. For example, if we have a digital twin for crack growth in a part, then updating on a weekly or monthly basis is usually enough to capture the dynamics of the crack growth. However, if we have a digital twin predicting near real-time performance of a chemical processing plant, then we will likely need data feeds every second or more.
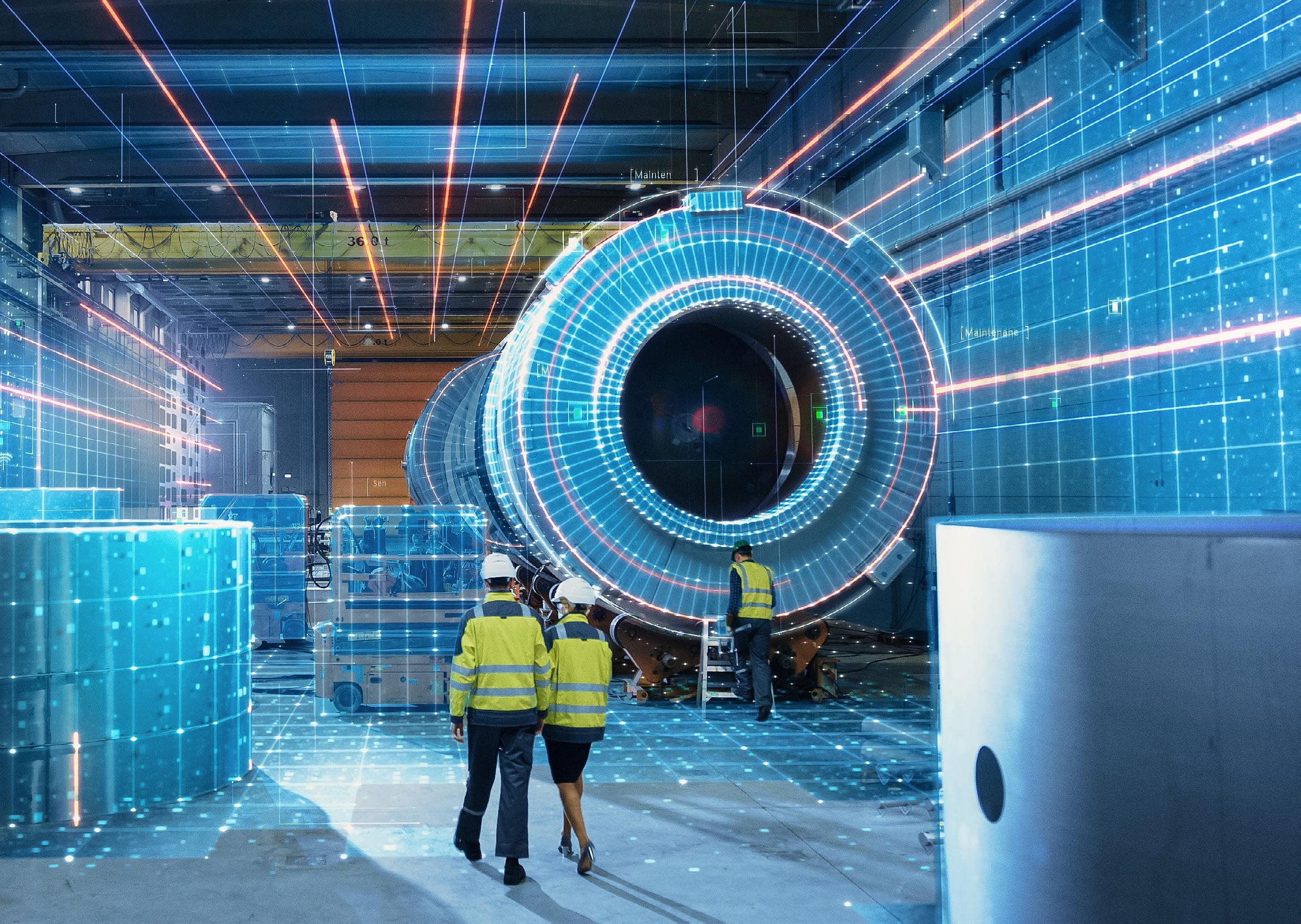
Now, in discussing with customers, we found that we had to expand our interpretation to include other use cases that our customers considered digital twins.
The digital twin framework
In our L1-L4 framework, Level 4 is the definition of digital twins described earlier. Levels 1 through 3 are other categories of use cases that have business value and our customers are asking for. The L1-L4 framework helps operators understand the data, models and business operations needed by first helping them categorize their use cases and identifying similar use cases solved by others. In our leveling framework, we define the 4 levels as L1 Descriptive, L2 Informative, L3 Predictive, and L4 Living.
L1 Descriptive is about describing the structure of the system. This includes all the engineering and scientific simulations such as 1D analysis, systems dynamics, computational fluid dynamics, and structural mechanics. It also includes visual representations such as 2D engineering diagrams or complex high-fidelity 3D/AR/VR models used for virtual walkthroughs or immersive training.
L2 Informative is about understanding the state of the system. This is through connectivity where the IoT sensor data and maintenance data from the physical system is aggregated and displayed in context relevant manner. It enables the end user to understand the present condition of the physical system and can include simple analytics to trigger alarms. This is the domain of IoT and asset management integrated with enterprise asset management (EAM) or enterprise resource planning (ERP) systems to show asset configuration, maintenance history, and upcoming work orders on a single pane of glass.
L3 Predictive is about understanding behavior using a pre-trained model with the implicit assumption that future behavior is the same as the past. The model can be a physics-based (first principles) model, an ML model, or increasingly, we are seeing hybrid models. The predictions can also be tied to recommended prescriptive actions that the user can take to remedy identified or predicted anomalous operations.
L4 Living is also about understanding behavior but for situations where the future behavior is not the same as the past. This is the norm for industrial equipment whose performance evolves over time due to degradation. Here we need a living model where the data from the physical system is used to update and individualize the model parameters itself to make probabilistic predictions and what-if scenario analysis.
The benefits of digital twins in oil and gas
Let’s now use the L1-L4 framework to understand how digital twins can help address some of the key challenges facing the oil and gas industry, specifically the challenges of retiring workforce, lowering production costs, and optimizing production processes. We will discuss some of these applications below.
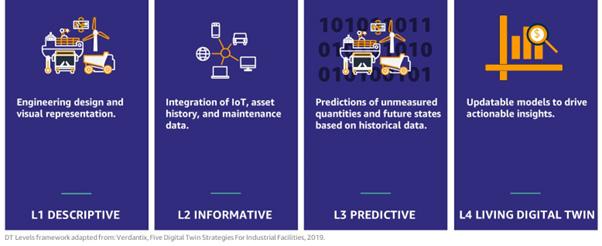
In our discussions with oil and gas customers, the concern of knowledge loss due to a wave of retirements of the most experienced operators was top of mind. Periodic economic shocks over several decades has resulted in low hiring rates of younger, next generation employees. Now, there is an urgent need to capture the knowledge of the most experienced personnel and to transform that knowledge into engaging training content and operational decision support for the next generation.
In this context, L1 DTs of virtual immersive environments of offshore oil rig platforms or refinery control room environments can be used to provide realistic scenario training for operators. Similarly, L2 DTs can support the augmented worker to have most relevant information available at their fingertips. The use of augmented reality (AR) can help new technicians by providing context aware prompts for service interventions, in effect offering a virtual apprenticeship, as well connecting to a centralized pool of experts for remote assist.
Building maintenance plans with digital twins
As we look to managing production costs, we see digital twins used across the board particularly in predicting the remaining useful life (RUL) of equipment and then building predictive maintenance plans to insure continuity of operations. One challenge is that it has been common to use L3 DTs (pre-trained models) to predict equipment failure. This approach, however, tends to lead to large number of false positives as the L3 DTs are based on pre-trained models based on average fleet behavior. An improved approach is to use L4 DTs where the models are individualized using sensor data from the specific equipment, in effect creating a “model of one”.
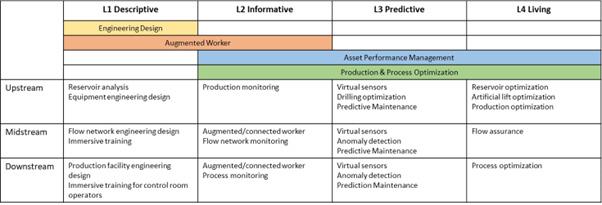
These L4 DTs are created using a combination of physics-based models, augmented with ML, and using probabilistic Bayesian estimation methods to provide predictions with uncertainty estimates. For example, knowing your compressor is predicted to fail in 6 months +/- 5 months drives a very different operational business action versus a prediction to fail in 6 months +/- 1 week.
For optimizing production processes, L3 DTs for anomaly detection go beyond simple threshold and pattern-based alarms, but rather use models of the process itself to identify deviations from expected operations. L4 DTs, however, are the most relevant as the “model-of-one” approach with uncertainty quantification enables more accurate up to date models to conduct what-if scenario analysis for their facility. For example, refineries already use model-based control paradigms, and extending the concept to using probabilistic Bayesian calibrated plant models to conduct scenario analysis enables operators to identify the optimal operating parameters.
Additionally, unconventional oil production is common for an oil well to start with thousands of barrels per day of flow, and then drop to hundreds or even tens of barrels of oil over a few months. Determining the optimal artificial lift throughout the life cycle of the well is a complex decision based on techno-economic factors such as reservoir conditions, operating conditions, well-to-well flow interactions, and the market price for crude. These decisions are historically based on operator experience and rules-of-thumb developed over years. We’re seeing customers build L4 DT “artificial lift optimizer” models to better support the field operators in deciding when and how to replace the artificial lift.
The oil and gas industry has been at the forefront of developing and deploying Digital Twins to solve industry business challenges across upstream, midstream, and downstream domains. Much progress has been made to date and efforts continue to apply Digital Twins from L1 through L4 in a range of applications across the industry.