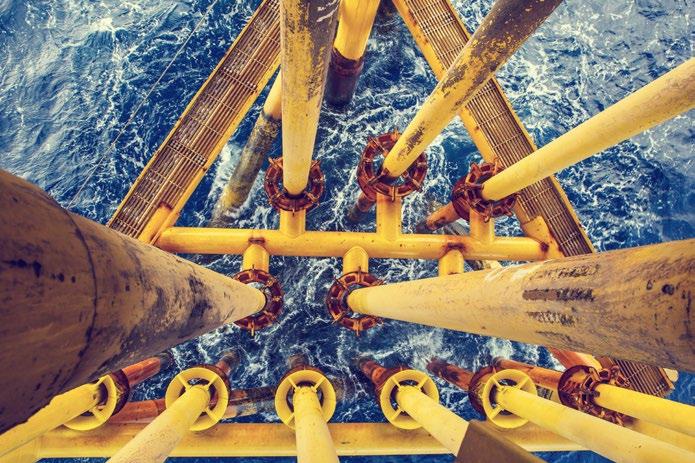
8 minute read
Delivering operational excellence in a datadriven world
Oil and Gas Technology spoke to Brendan Sullivan, Technology Business Leader at Viasat, about how the company is helping oil and gas operators meet the myriad of challenges they face in today’s volatile business landscape.
As the world’s leading network and technology provider for connecting and securing complex, distributed sites and operational assets, Viasat provides optimised solutions that deliver competitive advantages for oil and gas operators. With over 12000 onshore and offshore sites deployed around the globe, Viasat is the world’s leading provider of secure communications networking solutions for remote exploration and drilling. Viasat provides system integration services that can be customised to deploy communications systems for global connectivity. The monitoring tools and support and designed to protect the remote network and site data.
One of its essential offerings to the industry is Intelie by Viasat. This machine learning platform uses automated realtime processes to manage operational risks that threaten safety, compliance, integrity and performance. Viasat delivers powerful AI solutions that help organisations transform data into results.
What knowledge will you impart to the delegates at the upcoming Future Oil and Gas events?
I use this event to focus on operational AI for oil and gas operators to increase profitability. It all comes back to data, an industry awash with data, especially at the operational level.
Intelie by ViaSat is what we call operational AI, and the whole point is to eliminate or reduce what they call NPTnon-productive time - or hidden loss time. We are using data and machine learning on top of data to achieve these results, but where the rubber meets the road is on a decent size fleet for big operators. If you can get a one per cent improvement in efficiency, that is around a billion dollars of actual savings.
Data is not new to the oil and gas industry; it is very mature in digital data, so how can you help them get business value from that data?
They are good at data because of all the seismic data they collect. There is just a new way to take advantage of that data and let them change their operating procedures while the process is running, rather than waiting on the analysis of a one-year campaign to say we could have been more efficient. We are increasing the cycle time of operational improvements using machine learning and AI on top of the data in real time.
Can you give me an example of what that means for operators?
Almost all operators and contractors now have collectors on rigs, mostly deepwater rigs. They are collecting 10,000 to 50,000 data points a second of downhole and topside data for a drilling campaign. That is a tonne of data, and a lot of that data goes dark, but it is sampling almost every single second.
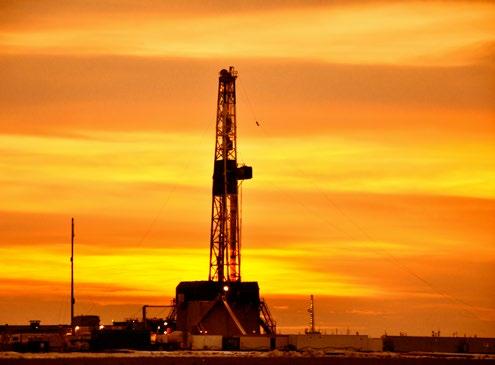
Intelie focuses on real-time operating centres where we take this data in real-time. We have experts sitting on shore rather than being on each rig, and can we have them look over the shoulder, suggest improvements in the operational process, and make decisions faster? Suppose you can remove a couple of people off the rig. In that case, that is millions and millions of dollars of savings because you can have a 25-year expert helping five or seven rigs at a time instead of sending them out individually to each rig.
Suppose you can do that real-time advisory system on top of these drilling campaigns. In that case, you can save hundreds of millions of dollars in cost because you speed up decision-making, and at the same time, you significantly improve safety, which oil and gas care more about than just making a profit. The drill room itself is the most dangerous place on the rig, so getting some of these people out of the red zone and even just putting them somewhere else on the rig also significantly improves safety.
Is this data there already, or do you need to add extra sensors to gain complete results?
You have the sensors on the rig and the connection in between; even deepwater rigs are all connected by satellite. Then you have all the work happening in the cloud to conduct data analytics in real-time. We do nothing with sensors; we let the experts handle that.
Is this drilling as well as its completion and flow control? What benefits do you deliver?
We concentrate on drilling and completions. We do offer some production solutions, but we focus much more on upstream and support both the drilling contractor and the operator. If it is a drilling contractor, they get most of the value out of predictive maintenance; they just want the assets to be up more often, and some deepwater drill rigs are up to a million dollars daily. If they have unplanned downtime of several days, they lose millions of dollars in deferred or lost revenue. Anything you can do to increase uptime benefits the drilling contractor.
If we are dealing with the operator, they care much more about staying to plan, getting to first oil faster, and shortening the drilling campaign from six to five months. The quicker they finish with the upstream, the faster they reach profitability. We try to work on the penetration rate, feet per hour or days drilled. Then, avoid things that make you redrill, such as washouts.
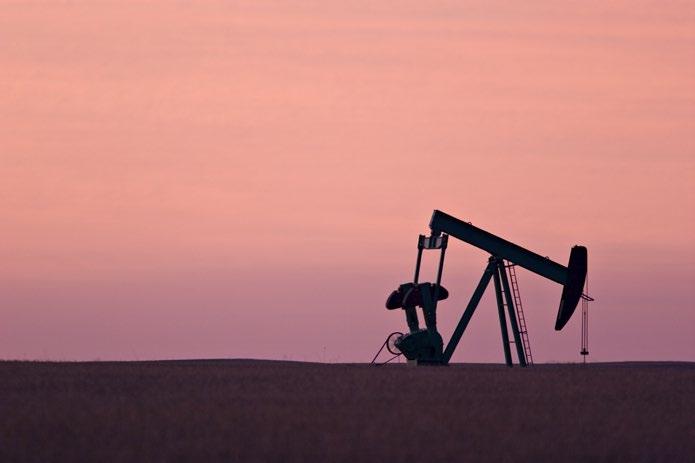
How much of a problem is it getting access to real-time data from all the operators and OEMs involved in the programme?
It is their data, and for the last ten years, operators have been writing into their contracts with the drilling contractors that they have to have access to this data. What it is really about is that there are better ways to use the data than ten years ago. There have been significant advancements in machine learning, which keeps improving each year. Maths has been around since the 50s, but we did not have the computational power to do as many maths models in real time as we do today, with edge computing and the power of the cloud.
How difficult is it to develop accurate algorithms?
Many people can do real-time visibility, and many people are competing in lab-built machine learning models. The big problem with that dream of taking data and putting it in a lab scenario is that it takes six months to develop a new predictive algorithm. Ninety per cent of those never make it out of the lab and into production. Most of those projects have high aspirations, but they fail, and it is because they are very tricky when there is that much data and even in the same oilfield, there can be a lot of nuances. There is a lot of noise in that data, either the quality of the data or missing data, that when you are building a lot of algorithms and using a lot of sampling, you have to tune them in production. This takes a while, people lose patience, and the operations guys lose trust. Where we are different is we try to do three things at once, we try to build this absolute real-time state model to give you visibility into exactly where you are on the ground and precisely what is going right or wrong. We have over 100 different dashboards running over 200 models simultaneously in the cloud. We have a real-time state model that we create, and then we conduct physics models, so nothing predictive, but there are complicated maths models on calculated events. We have a state model and a series of physics models, and then we will apply predictive models on top of that. It predicts where you should be against what the other models say, which is where it gets interesting. If we change some assumptions about speeding up, slowing down and taking a bit more risk, can we get back to plan?
Are these the ‘what if’ simulations you run to create the best scenarios?
Yes, you hear these terms thrown out, which I think is just jargon for jargon sakes from data scientists. It is all about heuristic models or stochastic models. Suppose you are running an extensive simulation with a stochastic model. In that case, you are taking every variable and changing the conditions, and you might run 20 million simulations to see which is better. Assuming the following parameters is the best possible plan with the best probability of the outcome you want.
When you run a heuristic model, it is like solving Sudoku. You have to guess and see and then work backwards to see if it worked. The same happens in very complicated scenarios, and with maths, there are too many unknown variables. So you must lock a few in, make an educated guess, and then simulate it. Doing heuristic models in real time is tricky, but that is where we are going; we are trying to present all those things together.
How do you deliver it, and how can someone monitor the 100s of dashboards you provide?
We go into year one of the new engagement, and we will throw out 100 or 200 dashboards, and then it will get rationalised down to around ten that are used. We try to use Intelie to remove all the subsystems from OEMs and be the integration platform so that you can go from different dashboards to one set.
The end customers for Intelie are not data scientists; our platform is designed for senior engineers, like the 20-year-old veteran who knows the oil and gas process backwards and forwards. It is for them to look through the data and make a decision faster. We have to throw a lot of different looks out there because, depending on your speciality, they are used to seeing information in a particular format, and they still want to see that.
Are your real-time insights advisory only and not control?
No, that is not true. We started as read-only, and then we went to advisory, which is much more of a legal thing than a technology thing because people would not hand over decision-making to the machines. You did not want the operator doing advanced maths. In my keynote, I will talk about our joint venture of ViaSat and Transocean, the biggest drilling contractor in the world, and a robotics company out in Norway called Intellilift, and we created a JV for autonomous well construction. We are remotely piloting and running the drill room from transition and shore using AI.
It seems like Intelie is at the cutting edge of AI in the oil and gas sector.
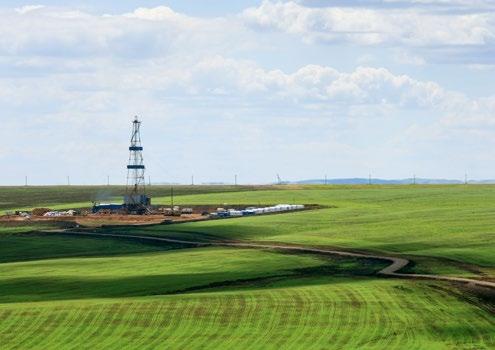
Intelie is not a new solution; it has been around since 2009 and has worked in oil and gas since 2012. There have been many startups in the last couple of years and a lot of unproven technology; a lot of snake oil is being sold. We have patents in history, meaning we have been through all this pain before and learned many lessons.
Secondly is what we already talked about: we try to use multiple forms of visibility and context through physical models and then apply them on top of it. We go from basic to enhanced to advanced.
Thirdly we try to create an innovative human-machine interface and try to dumb information down for super advanced users and allow them to modify our code directly if they have the skills to do that. We will enable them to reorganise all their views to get it the way they need to see the data, and with that human-machine interface, we took a step further by putting robotic control into it. It is not just an advisory system, it is now a fully round trip system, and by doing that, you take it to a whole new level. This is one of the most advanced AI platforms in the world.