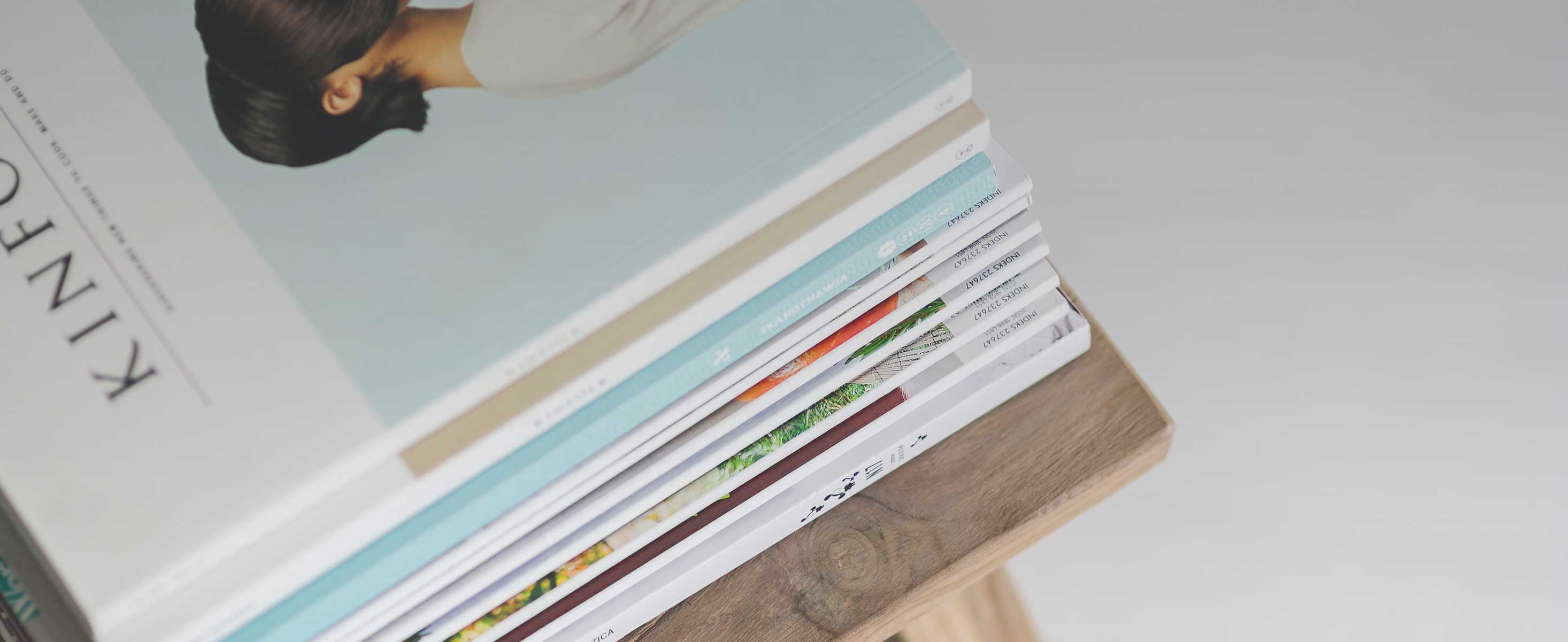
3 minute read
Analyzing the IDMS Data
Make sense of the data. Look for causal relationships. Always keep in mind that analysis is precise – each finding tells you one thing – so you need to be careful not to misuse data. In the same way that wrong diagnosis by a doctor to a patient’s illness could lead to illness, impairment, or worse, death; wrong analysis of the community’s current situation could result to plans that do not cater to the needs or the community nor address their real and most urgent concerns. Here are some of the common techniques in analysis that you can use:
Techniques in Data Analysis
Advertisement
Trends
Trends can be up and down, linear or exponential, steady or fluctuating, seasonal or random and there can be changes at a defined rate. Check out your data. Do you see apparent direction or trends when looking at the data on: • Level of knowledge about disaster? • Disability type and access to services? • Involvement in community activities by age? • Household income?
Comparison
Comparisons can be based on ranking, measurements, range, context, relationships. Do you see a difference or similarity in data such as: • Gender and access to services? • Gender and knowledge about disaster? • Family costs for disability according to types of disability?
Patterns
A pattern is a series of data that repeats in a recognizable way. Does your data show: • Clear connection or relationship between or among the indicators? You may look at the disaster knowledge level according to the level of educational attainment. • Gaps such as lack of service access and knowledge about their rights? • Outliers? There might be a specific segment of the population that has zero knowledge about disasters, for example.
Up and down vs. Flat Linear vs. Exponential Steady vs. Fluctuating Seasonal vs. Random Rate of Change vs. Steepness
Exceptions/outliers Intersections Correlations Connections Clusters Associations Gaps
Categorical comparison and Proportions
RANKING: Big, small, medium MEASUREMENTS/VALUES: Absolutes Range and Distribution CONTEXT: Targets, forecasts, averages Hierarchical Relationships
Here are additional guide questions that can help in data analysis: • Are there any similarities or differences? Do you see any pattern? • Compare your data. It can be based on time, amount, quality of data
• Find relationships in your data – is the type of housing of the household relevant to their income? • Trigger questions – and make sure you answer them.
Indeed, there are many different ways of making sense of data and the following techniques are not new. Organizations do it all the time. The most basic way of analyzing something is by using comparisons. Drawing comparisons can put your analysis into context, it brings in the wider picture and it becomes easier to understand and appreciate what the data is telling us. The comparisons you can do are: • Compare your community data to the national or regional average; • Compare your results to past results, to get a view of how the issue/topic has changed over time; • Compare your results with other communities near you; and • Compare your results with other communities with similar characteristics to your own. Look at the sample table to the right showing summary for reasons for unemployment among persons with disability. Notice that the primary reason for unemployment in the barangay is functional limitations, followed by the lack of opportunity. What other indicators do you think organizations can look into if they plan to design and implement interventions aimed at reducing number of the unemployed among the sector?
Reason for unemployment No. of persons with disability
Communication barrier 21
Difficulty in daily commute/ transport 3
Functional limitations 40 Inaccessible workplace/s 2
Lack of education 18
Lack of opportunity 24
Other reason/s 32
Personal choice 24 Refused to be allowed by 8 family to work
TOTAL 172
There are many available indicators we could zoom into. For one, examining the capacities of persons with disability which are captured in the IDMS Data can certainly provide stakeholders and duty-bearers adequate bases which livelihood projects to fund or which skills training are much needed by the sector.
Non-technical skills acquired No. of persons with disability
Auto mechanic Carpentry Computer literacy Domestic chores Driving Tailoring Electrician Plumbing
TOTAL
3 10 12 40 8 13 4 1