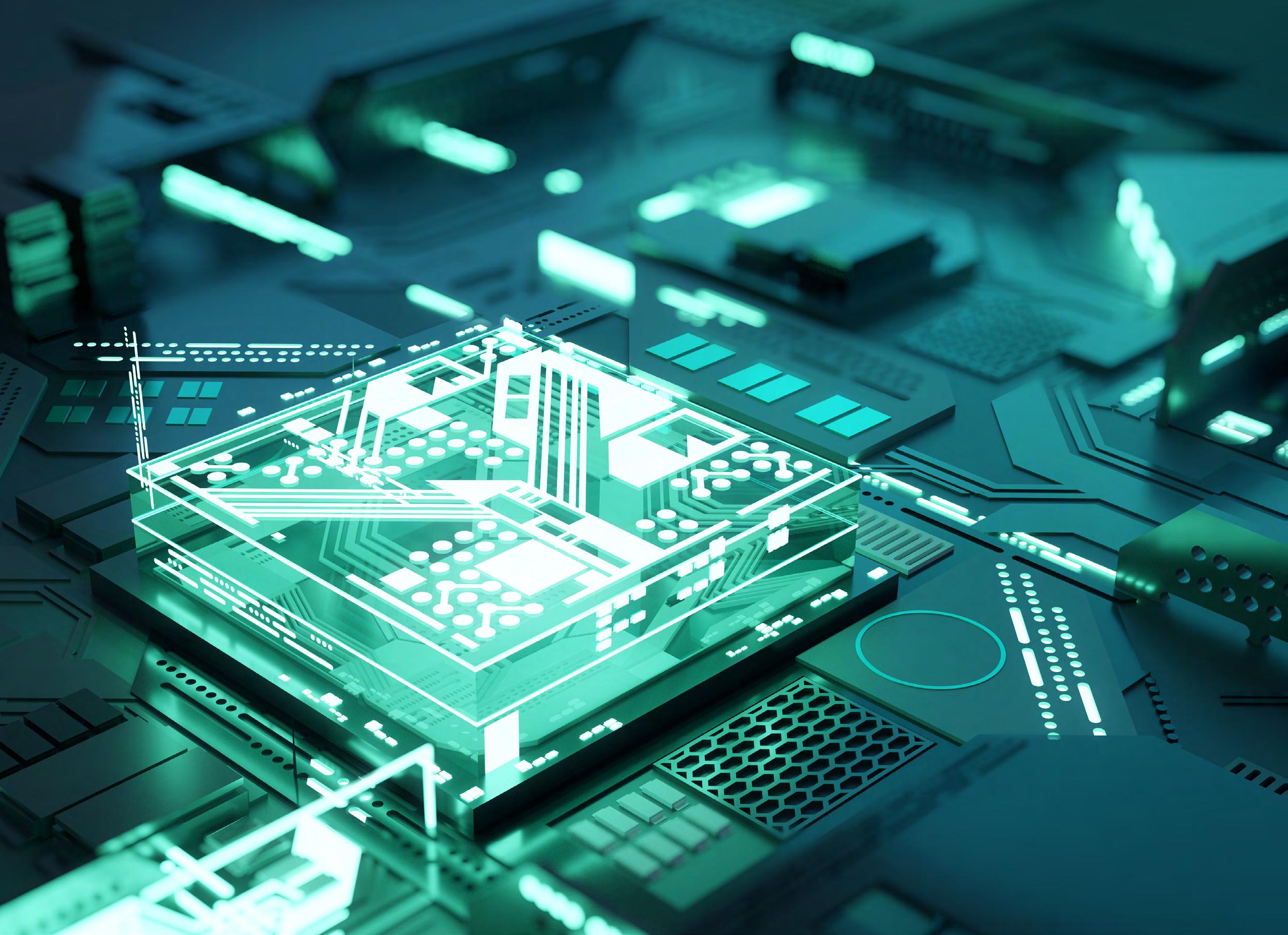
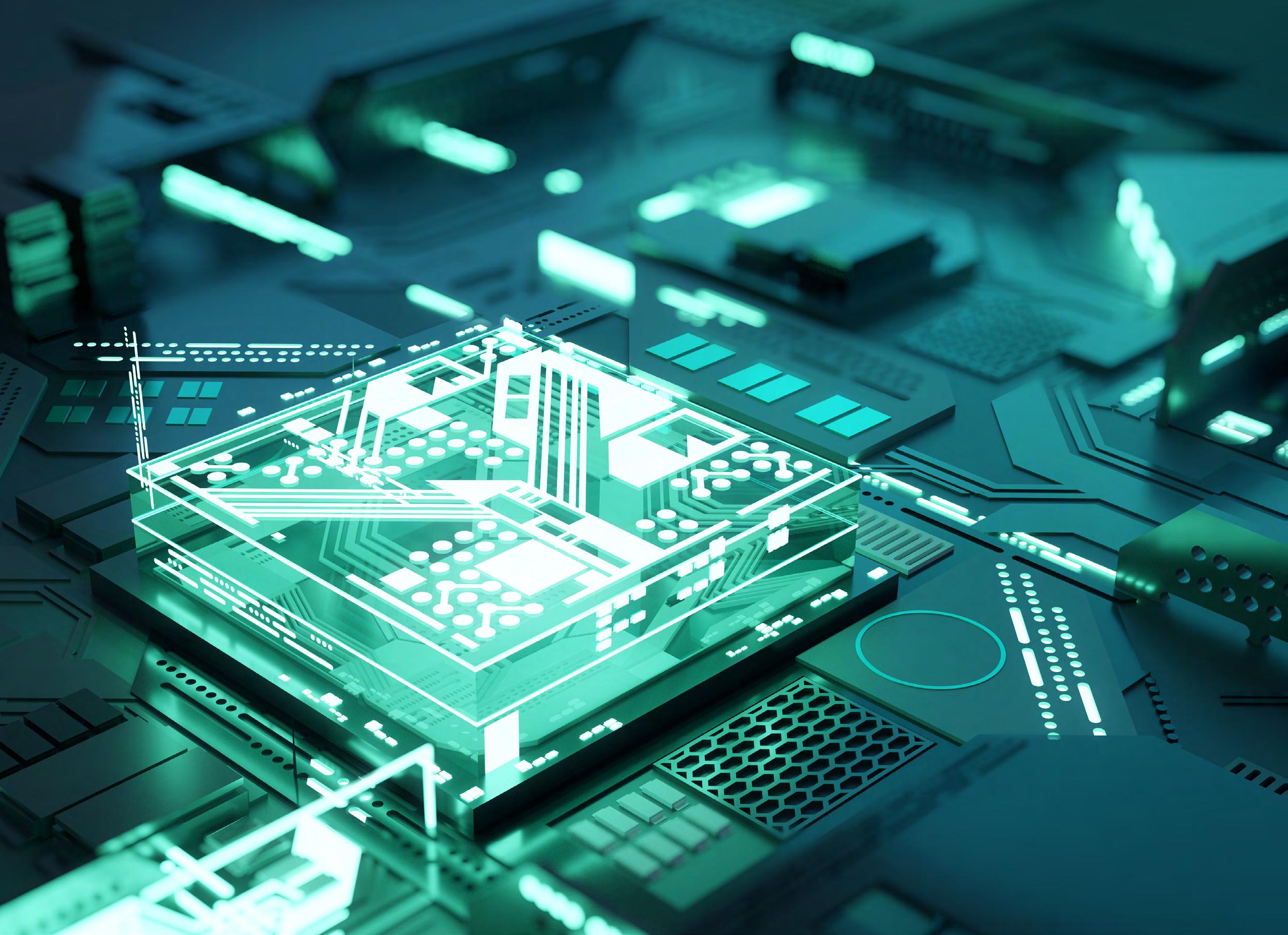
MOVING BEYOND BUZZWORDS
A new normal – models and risk management changes
Andreas Simou, CeFPro
Navigating the AI/ML risk landscape
Gustavo Ortega, Voya Financial,
Seyhun Hepdogan, Fifth Third Bank
Beyond risks and controls: The role of governance in advancing AI/ML
Shawn Tumanov, BMO Financial Group
Quantification of model risk according to the principle of relative
Michael Jacobs Jr., PNC

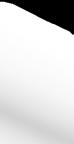
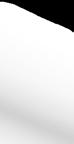
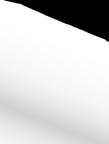
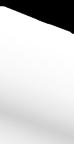
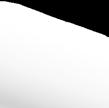

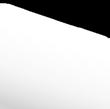

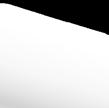

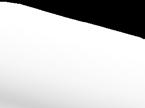

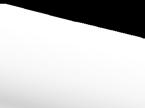

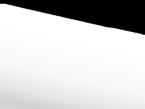
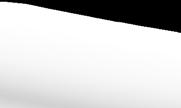
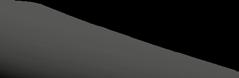

Using model risk management principles to manage AI risk
Chris Smigielski, Arvest Bank
Global third party risk management report out now!
The power of generative AI within financial organizations
Roderick Powell, Ameris Bank
The rise of non-validation model risk teams
Andrew Mackay, RBS and Deutsche Bank
Risk EMEA: The importance of stress testing in an uncertain world
HEADS
What role will AI and machine learning play in the future of financial services?
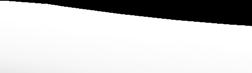

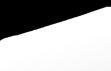
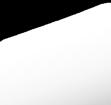
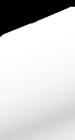
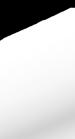
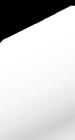

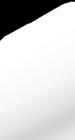

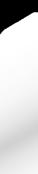
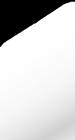

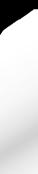
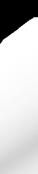

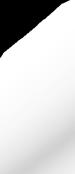

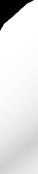
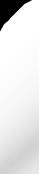

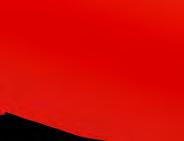

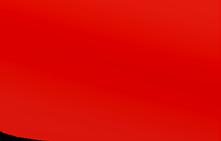

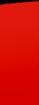
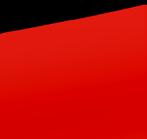
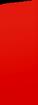
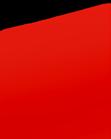
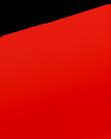
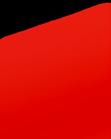

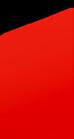
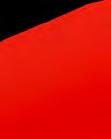

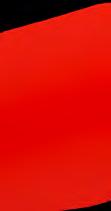
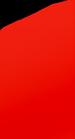
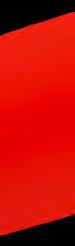
A new normal – models and risk management changes
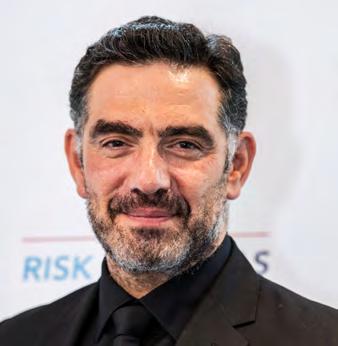
CeFPro is delighted to launch this new edition of iNFRont Magazine, covering all things Advanced Model Risk. After the success of our Advanced Model Risk event series in New York City, appetite to hear and learn more was higher than ever. The team at CeFPro compiled insights, conducted interviews, and heard from some of the leading industry experts to develop this exclusive edition.
As technology continues to further advance in all aspects of life, it is rarely more prominent than the evolution within financial services and the opportunities for risk teams in particular. We had the chance to hear from industry experts from Voya Financial and Fifth Third Bank on how they are navigating the complexity of the AI and machine learning risk landscape, with a focus on regulatory expectations, risks across the supply chain, and resource requirements to manage complexities.
AI and machine learning are buzzwords we have heard across the industry for some time. Though they now are becoming more and more embedded in BAU activities and reducing the need for human intervention on laborious, manual tasks. BMO Financial Group’s Director for Data and Analytics Governance shared his personal views on the role of governance in advancing AI and machine learning. He demonstrated the role of governance as an enabler in advancing transitions to more advanced approaches to modeling. Looking beyond simply capabilities and opportunities, but the successful implementation and embedding into BAU.
As the pace of industry change slows for the summer months, we hope this issue provides vital reading, sparking some ideas for considerations in your advanced modeling journey.
As always, CeFPro would like to thank the authors for their contributions to this issue who give their time and efforts to share insight and advance the industry. We look forward to the return of events after the summer when the next issue of iNFRont will launch, with a broader focus on non-financial risks.
Wishing our readers a great summer.
Andreas Simou, MD, CeFPro
OUR MAGAZINE TEAM...
We welcome contributions. If you or your organization are interested in featuring in our next issue, please contact infront@cefpro.com
ADVERTISING & BUSINESS DEVELOPMENT
If you are interested in sponsorship and advertising opportunities, please contact: sales@cefpro.com
PUBLISHER
Alice Kelly alice.kelly@cefpro.com
EDITORIAL ASSISTANT AND OUTREACH MANAGER
Ellie Dowsett ellie.dowsett@cefpro.com
MANAGING EDITOR
Kate O’Reilly infront@cefpro.com
HEAD OF DESIGN
Natasha Marino
www.cefpro.com
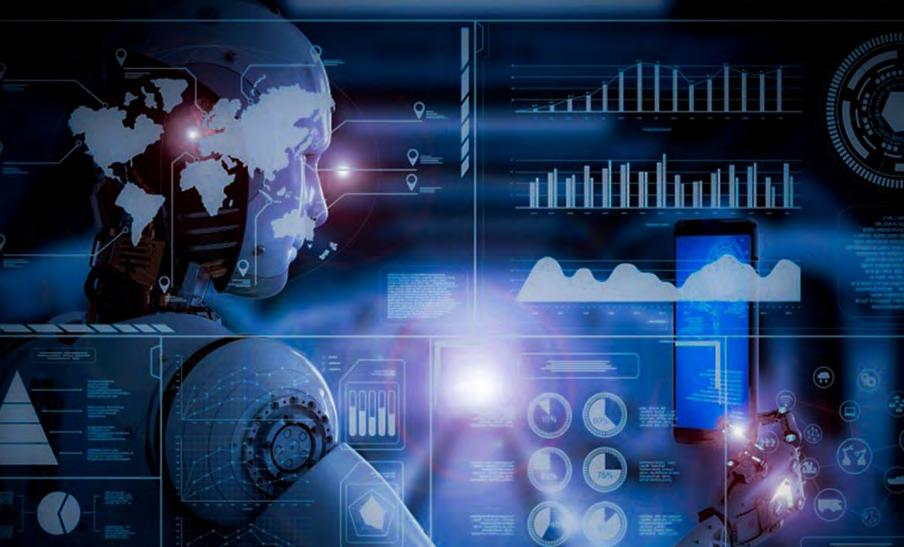
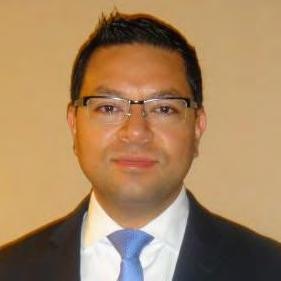
Artificial intelligence (AI) has gained increased momentum within businesses, including financial services, with more organizations exploring its potential. AI and machine learning (ML) tools are being used by financial institutions to increase productivity, create efficient workloads, and begin to reduce, if not eliminate human errors. However, alongside the advancement in this technology comes an increasing number of risks to explore, understand, and mitigate.
CeFPro’s Risk Americas Convention 2023 featured numerous sessions on AI, further demonstrating its importance within financial services and its place at the forefront of risk management. A session that drew particular attention was the Advanced Model & Risk Trends panel discussion, which focused on managing risks associated with AI & ML validation and oversight. Here, we summarize the most compelling aspects from this session, featuring insights from the panelists…
What is the regulatory expectation of AI models?
Senior Exec, Financial Institution: Artificial intelligence models can be broken down into the categories of credit risk models, fraud models, marketing models, and underwriting models. If you were to present underwriting models to a regulator, they would ask challenging questions to ensure organizations have an in-depth understanding of them and that they are not restricting credit. Although there are many questions around the restriction of credit and the explainability of adverse action, I believe standards are high when it comes to underwriting and regulatory models, as there are severe implications on capital and stress testing. However, in my view, standards are lower with fraud and
NAVIGATING THE AI/ML RISK LANDSCAPE
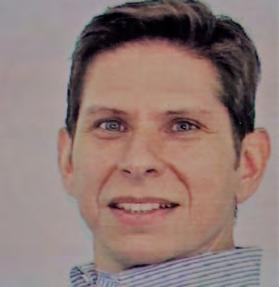
Seyhun Hepdogan
SVP Director Analytics
Fifth Third Bank
marketing. This could be due to the vast amount of data available on fraud and marketing, meaning that AI/ML is a more acceptable route to develop those models.
Which risk area should you most watch out for if you use vendors: third-party vendors or machine-learning models?
Seyhun Hepdogan: If you are using credit models, the most important thing to watch out for are vendor models and their changes. An AI/ML model has masses of data that could change unexpectedly. Throughout my career, I have seen vendor models providing an output with model drift. Issues such as data and/or model drift may not be noticed right away. Having a strong partnership with vendors, along with strong front-end and back-end model monitoring metrics are all high-level practices, we employ.
Gus Ortega: What worries me the most are the unknowns. I believe working very closely with data scientist groups, modelers, and assurance functions like legal and compliance is critical in this area. However, what I’m most interested in are the unintended consequences. The regulatory bodies are still trying to learn as much as we are about how they govern and supervise the emerging risk of Generative AI. From a model risk perspective as it relates to Generative AI and embedded algorithms in software packages, there are effectively two things to consider: 1) the discovery of platforms that have AI capability in them, and subsequently documenting these algorithms as models; and 2) understanding the data inputs, transformation, and validation, and how the output is subsequently used for management decisions.
How would you look at AI from a multi-risk management perspective, and how do you manage and monitor that?
Gus Ortega: AI is not a new thing – large language models have existed for decades and the use of machine learning and AI capability in our industry has been in place for many years. However, the popularity of platforms such as ChatGPT, Bard, and others, has generated significant amounts of interest among many risk professionals. The use of these tools without proper risk, compliance, and security controls can have damaging effects to an organization. There is inherently privacy, data, legal, and operational risks that must be understood and managed when using some of the new capabilities like ChatGPT and others in your dayto-day work environment. I also recognize the commercial benefits this technology would have in our industry and the potential to be a real disruptor that as risk managers, we must lean in, learn, and pivot to a new risk landscape enabling creativity and business innovation while deploying secured and well-governed IT controlled environments for this type of technology.
Regarding the question of regulatory implications, I think there is going to be an increased need for supervision. As of now, it is a bit of an unknown in how regulators would come together to supervise this new technology risk. However, my best advice is leveraging the tools and processes that are already in place from good governance to robust risk assessment and enhanced monitoring – the tools within our risk toolkit can help ensure risk and controls are being considered.
What are the critical things to watch out for when it comes to vendor ML models used in account origination, such as underwriting?
Senior Exec, Financial Institution: It’s not just vendor models but developing in-house custom models, too. The focus of the development team will change with AI/ML models, away from allocating resources and towards assigning more resources to test robustness. It’s imperative to spend more time testing perturbation analysis robustness when discussing the underwriting and explainability of the models, especially the adverse action. You must be able to tell your client why you’re declining them – if you don’t understand your models, how can you articulate the adverse action?
Where is the bar in terms of fraud relative to a consumer?
Seyhun Hepdogan: Compliance and legal teams set the high-level bar on what is and isn’t allowed. But when we look at vendor models or even internal models, fraud and marketing explainability/intuitiveness requirements used to be less strict compared to credit underwriting, collections, and other credit models. An emerging trend is regulatory
requests on explainability for marketing and fraud models. On top of a simple profile review of fraudulent customer transactions, we as an organization, a model owner, or a model user need to have an understanding what model(s) do.
Explaining a model in isolation does not necessarily help. It can be preferable to show how the model is assisting in regard to the goal you are trying to achieve. If you can explain this clearly, you will be on your way to trying to solve the problem. I believe this is the approach regulators are using; it’s about knowing the model’s limitations and being able to explain them. When it comes to underwriting or credit models, you need a stricter level of review.
How can we ensure the stability, robustness, and reproducibility of AI models in general?
Senior Exec, Financial Institution: Firstly, the validation cycle will be different. If applying these models in a fraud space, there won’t be a 3-6 month validation cycle, as fraud vectors are ever-changing; speed becomes of the essence. Once you start implementing these models, how do you ensure that the data going in is robust, whether the data controls are in place, and who’s checking the data quality? This process could be automated, but the system must be faster than traditional credit risk models. In the machine learning space, there is no silver bullet; rather, there is a variety of tests to triangulate your level of comfort around the models. There’s a lot more emphasis on testing and robustness, but even if machines are going to build the models, they must still be tested.
What is the current status regarding resources, talent acquisition, and ensuring in-house skills exist to deliver on any new AI/ML requirements?
Gus Ortega: There is a slight talent shortage in certain areas, including this one. How do you incentivize and ensure your institution is able to attract the right talent? It’s a challenge for sure, especially as we’re all after the same talent.
Senior Exec, Financial Institution: The key is to hire people who truly understand how the machinery is built. Strength and statistics are vital to comprehend, test, and showcase the models to regulators and there will be a race for this type of talent. The nature of work is going to change, but AI cannot synthesize analysis, which is where the competitive edge will come from.
Seyhun Hepdogan: For me, it’s all about partnerships; between model risk management, model development, legal, and compliance. Now more than ever, models are changing at a much faster rate. Wherever modelers can cut the amount of work that needs to be conducted, it needs to be done in a partnered manner, and learnings need to come from all the partners in the game.
To hear more on how AI and machine learning can advance data analytics and advance the evolution of customer experience view the agenda for Customer Experience Europe, taking place in London on November 21-22. Visit the website at www.cefpro.com/customer-experience
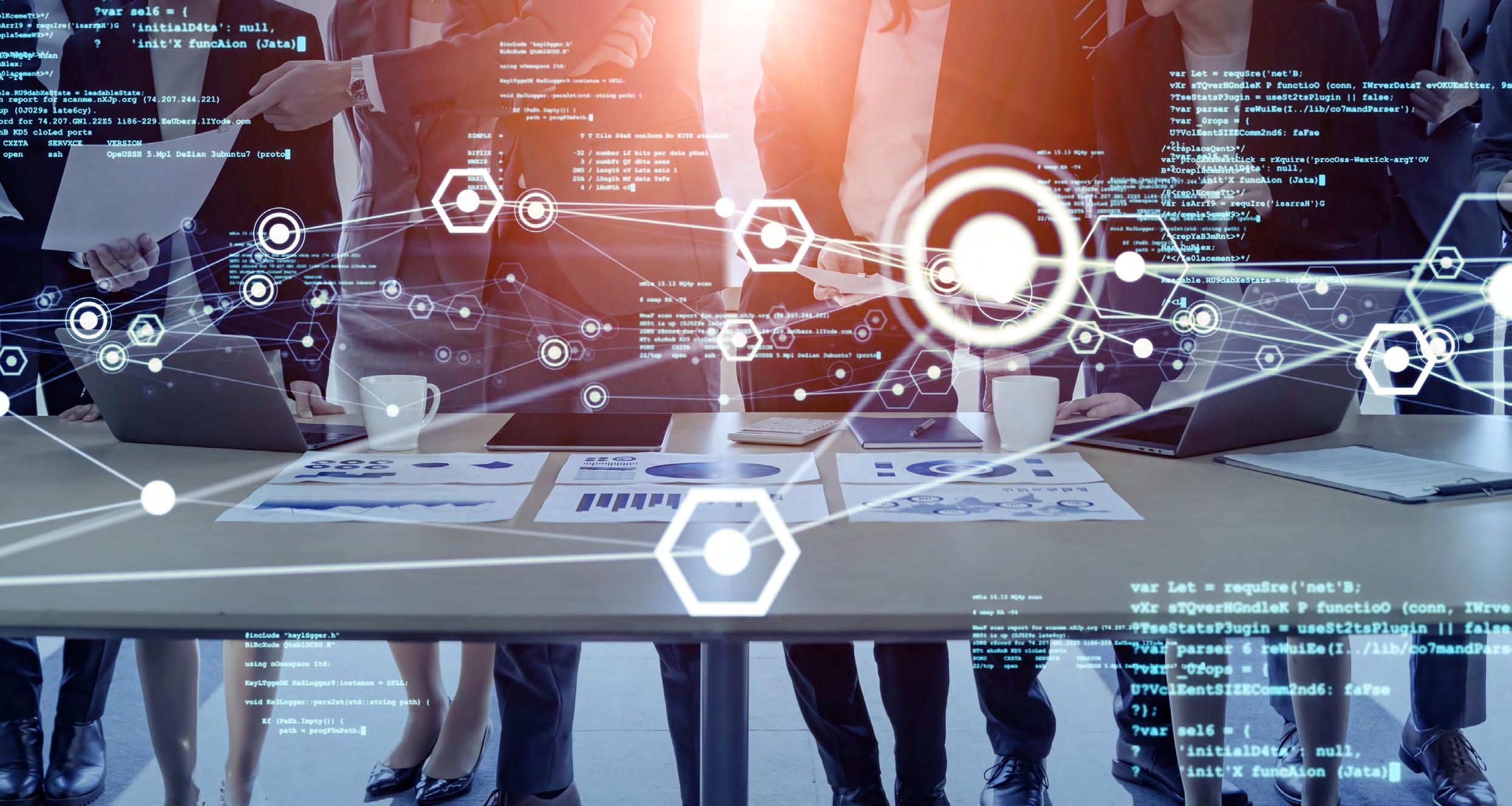
BEYOND RISKS AND CONTROLS: THE ROLE OF GOVERNANCE IN ADVANCING AI/ML
When we think about governance, words like compliance, audit, controls, regulations, and checklists come to mind. But governance can also play a vital role in enabling and advancing an organization’s transition to advanced analytics such as artificial intelligence (AI) and machine learning (ML).
Governance professionals should of course help an organization to navigate complex regulatory expectations, such as SR11-7, EU AIA, OSFI E-23, etc. But at the same time, they should also support the advancement of AI/ML, providing paths of least resistance, not roadblocks.
Auditability and explainability activities may not be required for all AI. These activities are best reserved for high- or limited-risk AI. Some AI proponents have suggested that it is not necessary to fully understand how each technology works in order
to reap its benefits, especially if the overall efficacy of the AI system can be demonstrated using alternative mechanisms. This approach is further supported by US regulatory expectations that evaluation activities should be commensurate with the bank’s risk exposure.
So, how can governance enable and support AI/ML?
• Use existing governance frameworks – AI has been around for over 50 years; it is not as new or emerging as some people claim.
• Help fill the gaps – Privacy, bias, and discrimination are currently receiving a lot of attention; help the AI/ML practitioners fill in the gaps.
• Become an advocate for AI/ ML usage – A data-driven
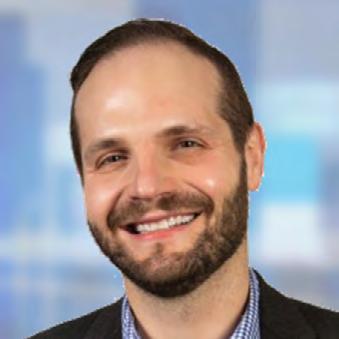
BMO
Financial Grouporganization should challenge legacy thinking; governance should be an advocate for change.
Use existing governance frameworks
The majority of financial institutions have existing risk governance functions. According to the Canadian Banker’s Association (CBA), these risks are already adequately addressed within existing operational risk frameworks. Therefore, it may not be necessary to create a brandnew AI/ML governance framework. Governance professionals should instead consider leveraging existing frameworks where possible and appropriate.
Model risk management (MRM) may be able to evaluate AI/ML in many organizations. In fact, MRM has been evaluating regression models (a type of machine learning model) for years. What should be in scope for MRM? It
is best to think of a ‘model universe’. The Federal Housing Finance Agency defines this as ‘models, model-based application, model processes, and significant end-user computing tools’. This expands the scope of MRM to include other types of models, sometimes referred to as non-risk, and can help ensure that they are developed and implemented in a way that minimizes risk and maximizes their effectiveness.
Validation of these models should be commensurate with the bank’s risk exposure; validation processes for AI should be tailored to specific risks, rather than a one-size-fits all approach. For example:
• The risk of a natural language processing (NLP) non-risk model turning words into text is likely not high enough to warrant a back-testing review or evaluation of modeling choices. Validation should still consider the choice of selecting a particular NLP model, implementation process, and annual confirmation that the usage has not changed.
• Another NLP model may be used to predict customer sentiment to drive customer attrition risk. Such a model would likely be higher. Validation may choose to validate this model like a ‘risk model’, potentially focusing on performance outcome analysis.
Help fill the gaps
In May 2022, the Comptroller of the Currency outlined the following incremental AI risks: explainability, data management, privacy & security, and third-party providers. Ethical risk is another area which comes to mind. Governance professionals can advance and enable advanced analytics by filling in the gaps between existing bank frameworks (such as model and technology) and incremental advanced analytics risks. This will enable data scientists to develop useful solutions while maintaining appropriate guardrails. According to Accenture, ‘Building sufficient data and AI ethics capacity can serve a key role in maintaining trust between organizations and the people they serve, whether clients, partners, employees, or the general public.’
One way to fill the gap would be to introduce privacy, legal, compliance, and ethics professionals to model developer and model validator teams. Working with a variety of stakeholder governance professionals can help
the development of an advanced analytics risk assessment, ensuring organizations consider the following questions:
• Ethics – Does the solution impact an individual’s access to products, services, or employment? Is it possible to explain the outputs of the solution?
• Privacy & legal – Does the use or collection of data comply with privacy legislation? Is personal identifiable information used?
• Fairness – Which variables are used in the model? What steps are being taken to avoid them being used as proxies for protected characteristics?
Whether something is considered high, moderate, or low risk will depend on the individual institution and its risk appetite level. In general, advanced analytics risk will likely be high if a solution is being used to automate access to products, services, or employment and there is no way to explain the decision. Higher-risk solutions should be reviewed by a committee to provide a recommendation to the solution owner about reducing and mitigating incremental risks. The committee should have the authority to stop projects which are deemed outside the risk appetite. One recommendation from the committee may be to introduce a human in the loop to review all or some outputs. Note that it is vital to have customer/client advocates as committee members.
Become an advocate for AI/ML usage Governance must become an advocate for AI/ML while bearing in mind this advice: ‘The goal is to ensure that humanity is not negatively impacted by technology that has so much potential to do good’ (ISACA Journal, Volume 4, 2022: Guy Pearce, Focal Points for Auditable and Explainable AI).
Governance professionals tend to think of risk, compliance, and controls. While risk mitigation is still at the core, in a data-driven world, organizations must challenge legacy thinking and not put up roadblocks unless necessary. Some tips for becoming an AI/ML advocate include:
• Focus on collaboration – All lines of defense must work together collaboratively. Historically, second line has been in the business of only providing effective challenge. In a
data-driven world, second line should become trusted partners willing to provide input into the process, while remaining independent.
• Education, education, education – Learn as much as you can about AI/ML. You do not have to be a quantitative statistician to harness its power, simply build a general understanding of AI and its use cases. The ISACA’s AI Fundamentals Certificate is a good target to aim for.
• Focus on use cases – Start with a small proof-of-concept that demonstrates the potential benefits of AI/ML.
• Keep up with regulations –Regulators and standards boards are constantly releasing new AI guidance or expectations (e.g., NIST AI framework). While it can be challenging to stay up to date with every latest development, awareness will help your organization to remain aligned with the wider industry.
• Engage stakeholders – Consider advocating for AI/ML with senior executives, business unit leads, IT teams, etc. Educate them about the benefits of AI and seek to address any concerns. Identify other AI/ML advocates in your organization to amplify your message and promote responsible and ethical use.
Conclusion
Protecting the bank is the professional primary role of governance. In a data-driven world, those banks that embrace AI/ML will be the ones that excel. Governance professionals should help data scientists navigate complex regulatory requirements while finding the path of least resistance by using existing frameworks when possible, filling in the gaps as appropriate, and becoming advocates for the everyday usage of AI and ML. However, it is important to note that the use of AI and machine learning raises concerns about risks and controls, as well as ethical implications. Therefore, any implementation of these technologies should be done thoughtfully and with consideration for how it will impact the workforce and the wider society.
The views and opinions expressed in this article are those of the thought leader as an individual, and are not attributed to CeFPro or any particular organization.
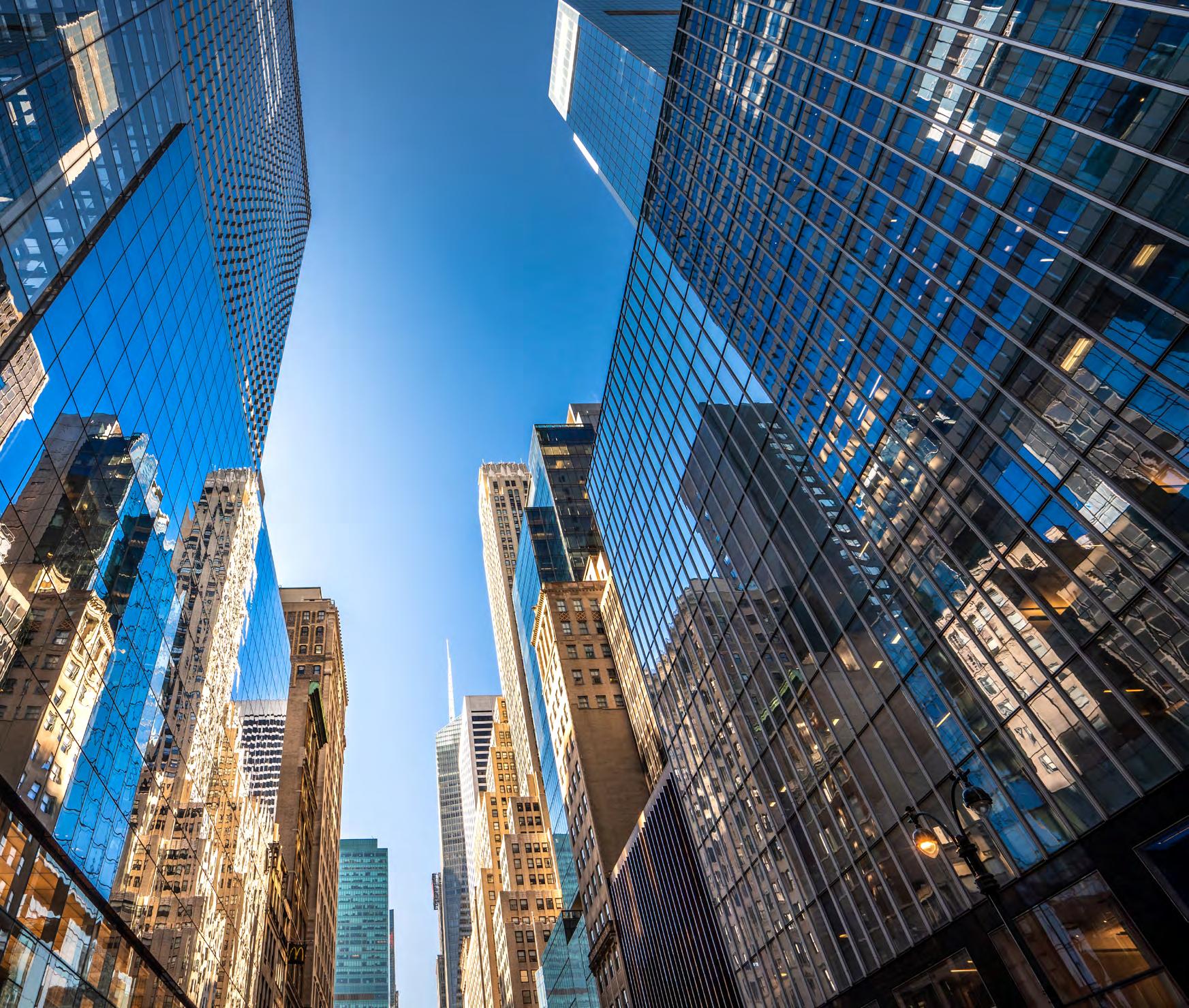
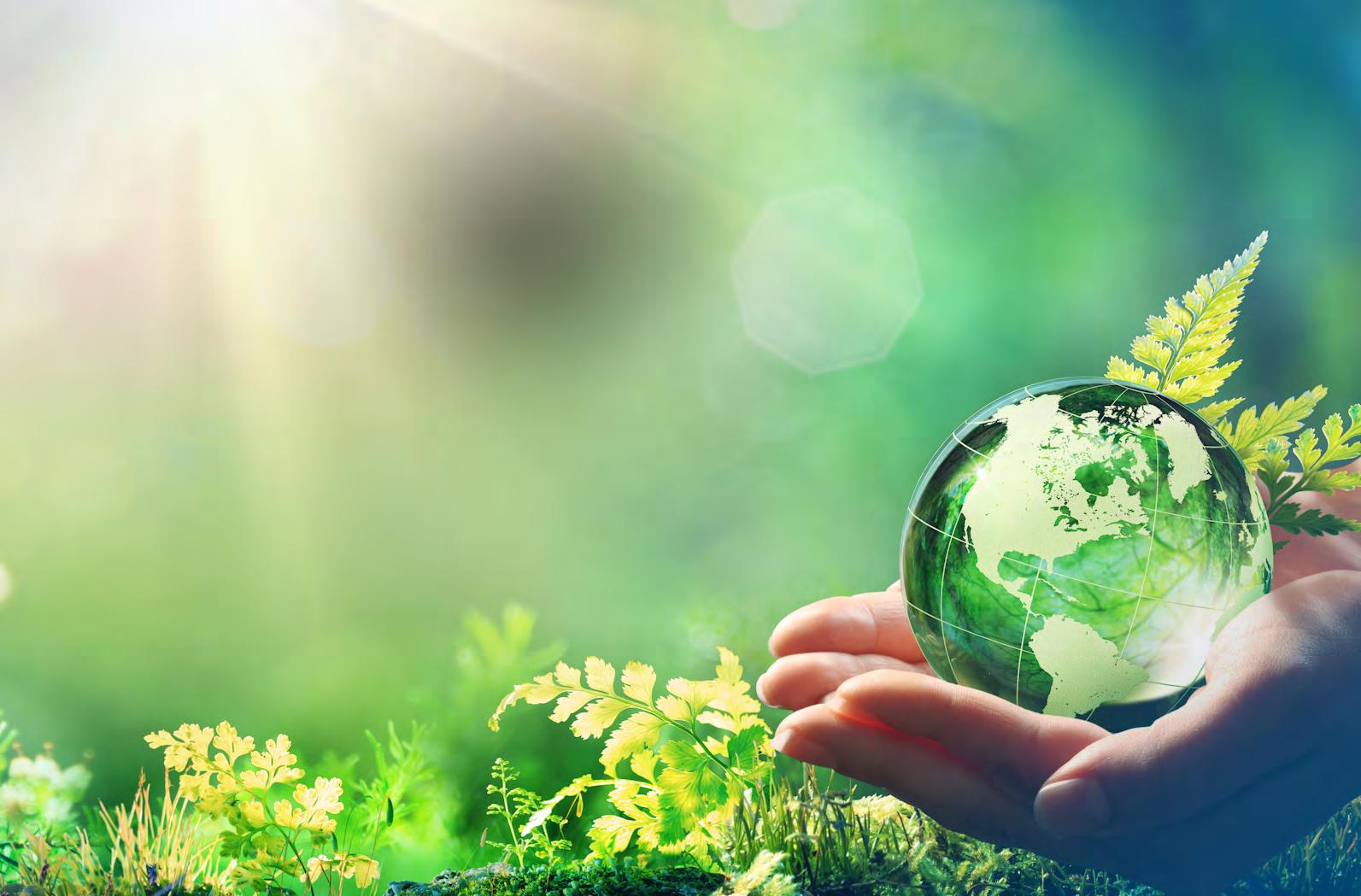
A 2-in-1 conference experience
NAVIGATING COMPLEXITIES ACROSS NON-FINANCIAL, OPERATIONAL, CYBER AND CLIMATE RISK
NFR AND OPERATIONAL RISK USA
CeFPro has once again undertaken an extensive research study in order to generate industry consensus on the key challenges and trends across non-financial and operational risk. As certain subsets of operational risk continue to evolve into their own risk teams and departments, it remains important to bring teams back together to view operational and non-financial risk holistically. The agenda for Non-Financial and Operational Risk USA does just that, providing a holistic overview of non-financial risk, whilst providing a deeper dive into specific niches to educate teams.
As part of the generation for any event agenda, the CeFPro research team conducted in excess of 50 one-on-one research calls with industry thought leaders, many of which are contributing as speakers to the event. Below are some of the key trends and challenges highlighted throughout the research process.
The research, and subsequently the event, opened with risk culture highlighted as a key focus as we navigate a new normal and operate under changing environments. It is more important than ever that organizations acknowledge
the importance of embedding a risk culture across departments and seniority. Risk culture can cascade through the organization and impact how teams function, both within risk and externally, having consistent messaging remains key. Addressing the risk culture of an organization remains a challenging feat, with many financial institutions being built on complex corporate and governance structures, implementing a consistent approach across the board is both vital and challenging. Organizations must develop methods and approaches that both support and drive a strong risk culture, allowing teams to feel empowered to raise issues and encouraged by solutions.
As part of this, knowing the internal team structures and points of contact are important. Ensuring that individuals know reporting lines and where to go to raise anything. Risk culture is a complex and evolving challenge, maintaining a strong culture, embedding it, and evolving it are key to success.
Unsurprisingly, a key area highlighted was the diverse nature of cyber risks and threats on the horizon. Cyber threats were raised so highly, the agenda was formulated
to carve out a separate stream dedicated to cyber risk and advancing knowledge across a range of areas. As would be expected, cyber defenses were top of mind and staying ahead of the continued escalation of techniques used. It was repeatedly highlighted that financial institutions stave off hundreds, if not thousands of attacks, so need to be highly successful; cybercriminals only need to be successful once. The ecosystem continues to be extremely complex and the threat vectors expanding with the ongoing race between attacker and defender. As is the case with many organizations, and in fact industries, securing budget to prioritize cyber security remains challenging. Many organizations have not necessarily felt the impact of a cyber breach, and therefore take a more reactive approach instead of getting ahead of the risk. Network security development and penetration testing should receive prioritization for all information security teams. Alongside the continued evolution and advancement of tactics, comes the increased risk of geopolitical tensions
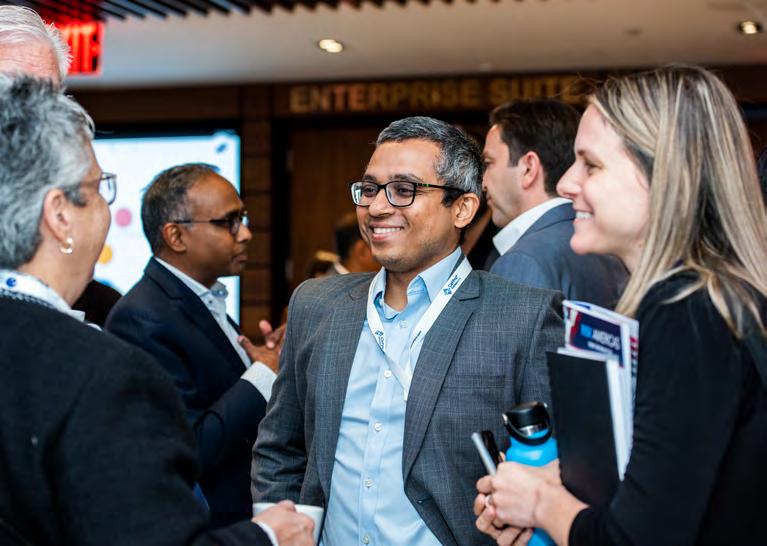
and the reality of cyber warfare. The risk of attack on vital infrastructure remains ever present, demonstrated by attacks on UK NHS systems, and US colonial pipeline over the last few years. The potential for further attacks on critical infrastructure remains a key concern with the weaponization of cyber warfare and state actors. The above risks are all applicable directly to financial institutions, however an added complexity is the risk of vulnerabilities across supply chains increasingly being exploited. Risks of a cyber incident to a vendor or third-party of an organization holds a high level of risk with the same potential reputation fallout and access to data. Organizations need a level of transparency across their third parties, and outsourced services, with contractual agreements detailing maintenance and controls over information security. Regulators also acknowledge the risk and continue to detail further guidance and requirements to ensure security across the ecosystem.
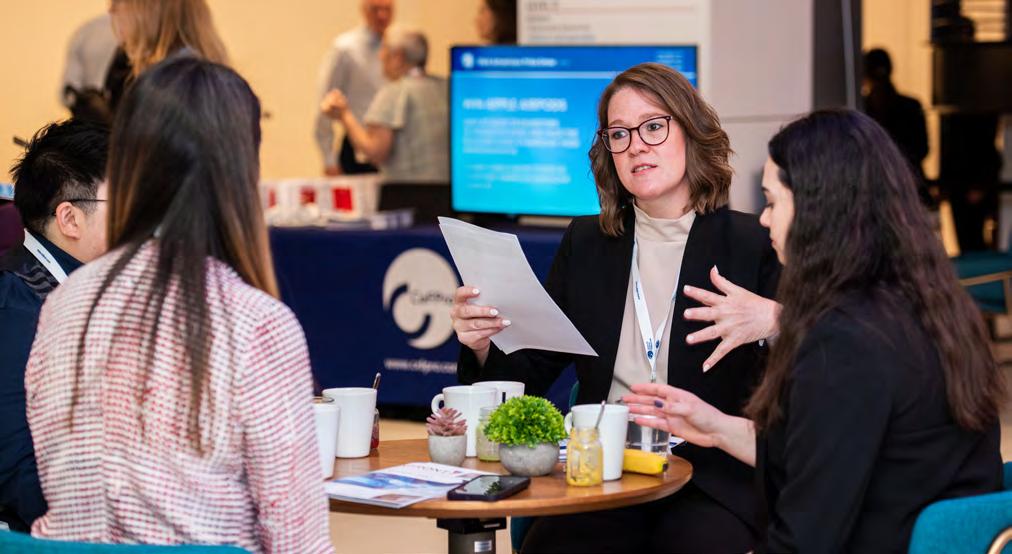
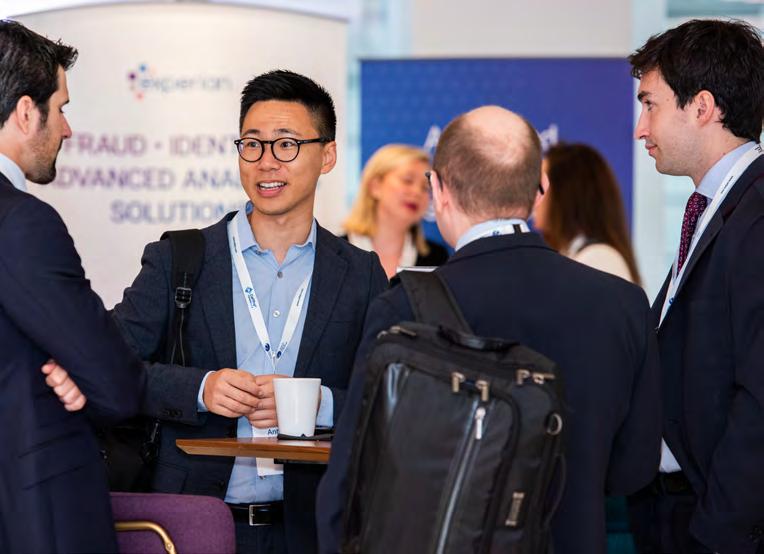
CLIMATE RISK USA
Taking place at the same time and same venue is Climate Risk USA, highlighted as part of the non-financial risk research as a key emerging area of concern and interest for many organizations. The research for this event focused purely on climate risk as opposed to ESG more broadly, though not to diminish the importance and complexity of ESG, the immediate focus was highlighted to be focused more directly on climate risk.
One of the key areas of focus was that of measuring, managing, and quantifying the impacts of climate related risk. In order to develop effective programs and mitigate risks to organizations, understanding the impacts and management techniques is vital. As a key, emerging trend, organizations are looking to integrate climate risk measurement practices into ongoing strategy, viewing no longer as emerging, but towards embedding into programs. Understanding the impact across portfolios from a climate perspective is an ongoing undertaking, understanding the complexities and variation of impact across products further develops an understanding of quantification metrics Climate risk also interacts with other risk silos, having an understanding of where it fits within a risk organization and its interaction with other areas of the business continues to cast uncertainty.
Climate risk has received substantial focus from global regulators, with some jurisdictions more advanced than
others, the impact on global organizations is substantial. Regulations to date have been fairly prescriptive in nature, with complexities for those operating across jurisdictions. Therefore, adapting strategies to incorporate these changes and ensure successful adoption is a focus for many. When navigating the climate regulatory landscape, organizations have several key considerations across the current and upcoming regulations and policies. Assessing the impact of already implemented changes, and interaction of future expectations remains a challenge, with uncertainty as to the focus and direction of global regulators. Inconsistency and lack of convergence of global regulators make the implementation of changes complex, with expectations interacting and sometimes conflicting. Adding to the complexity is not just global regulations, but state regulations within the complex US regulatory system. For organizations operating across state lines, requirements can differ from state to state, adding further intricacy to the work required.
The research for both event agendas was extensive, and driven by industry thought leaders to ensure its relevance, accuracy, and topical nature. Both feature detailed agendas, providing in-depth knowledge on a range of subject matters across non-financial, operational, cyber and climate risks. The events offer a one-stop shop to gain an overview as to the key non-financial risks, alongside deep dives into topical risk domains.
For more information on either event, visit www.cefpro.com/climate-risk-usa and www.cefpro.com/oprisk-usa.
AI adoption in financial services AI adoption in financial services
AI and machine learning: Is it hype or the future of financial services risk management functions?
AI and machine learning continue to gain momentum within financial services as more organizations explore its potential across business functions. Risk management functions continue to leverage the power of AI in reducing workload for manual tasks and mitigate some risk of human error. AI continues along its course to become a mainstream tool within the financial services sector. As the sector targets growth through leveraging technologies including AI, tensions continue to rise as to the future of risk managers. CeFPro’s Fintech Leaders report highlighted that in order for AI to reach its potential; training, recruitment and, technical knowledge are key considerations for the future in order to implement the technology and upskill team members.
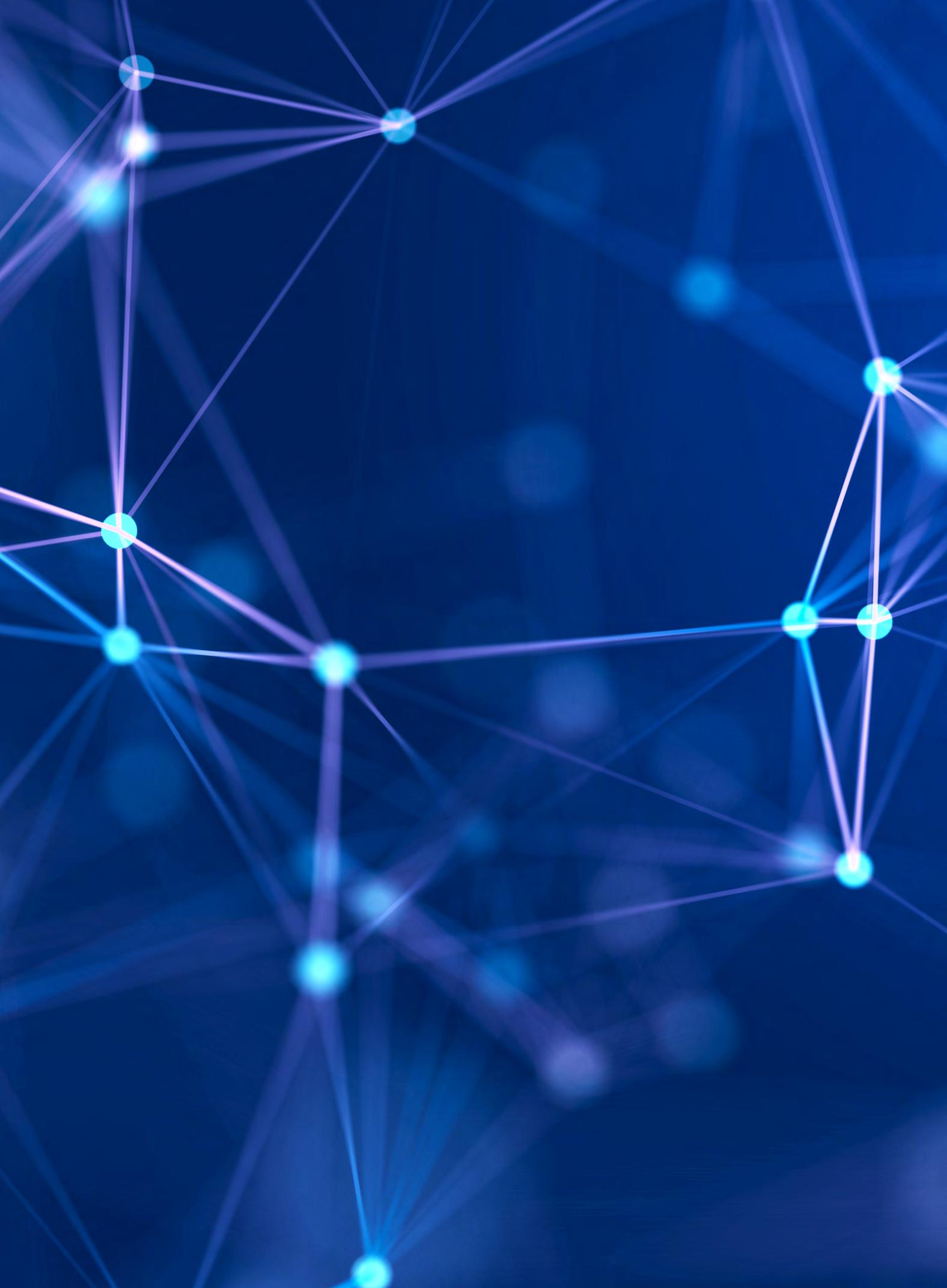
Have a
products and services
Source: The Economist: Banking on a Game Changer
AREAS CONSIDERED MOST IMPORTANT FIELDS WITHIN THE APPLICATION OF AI:
Source: PwC Study - How mature is AI adoption in
WHAT BENEFITS ARE YOU SEEING FROM YOUR AI INVESTMENTS
Source: Nvidia: State of AI in
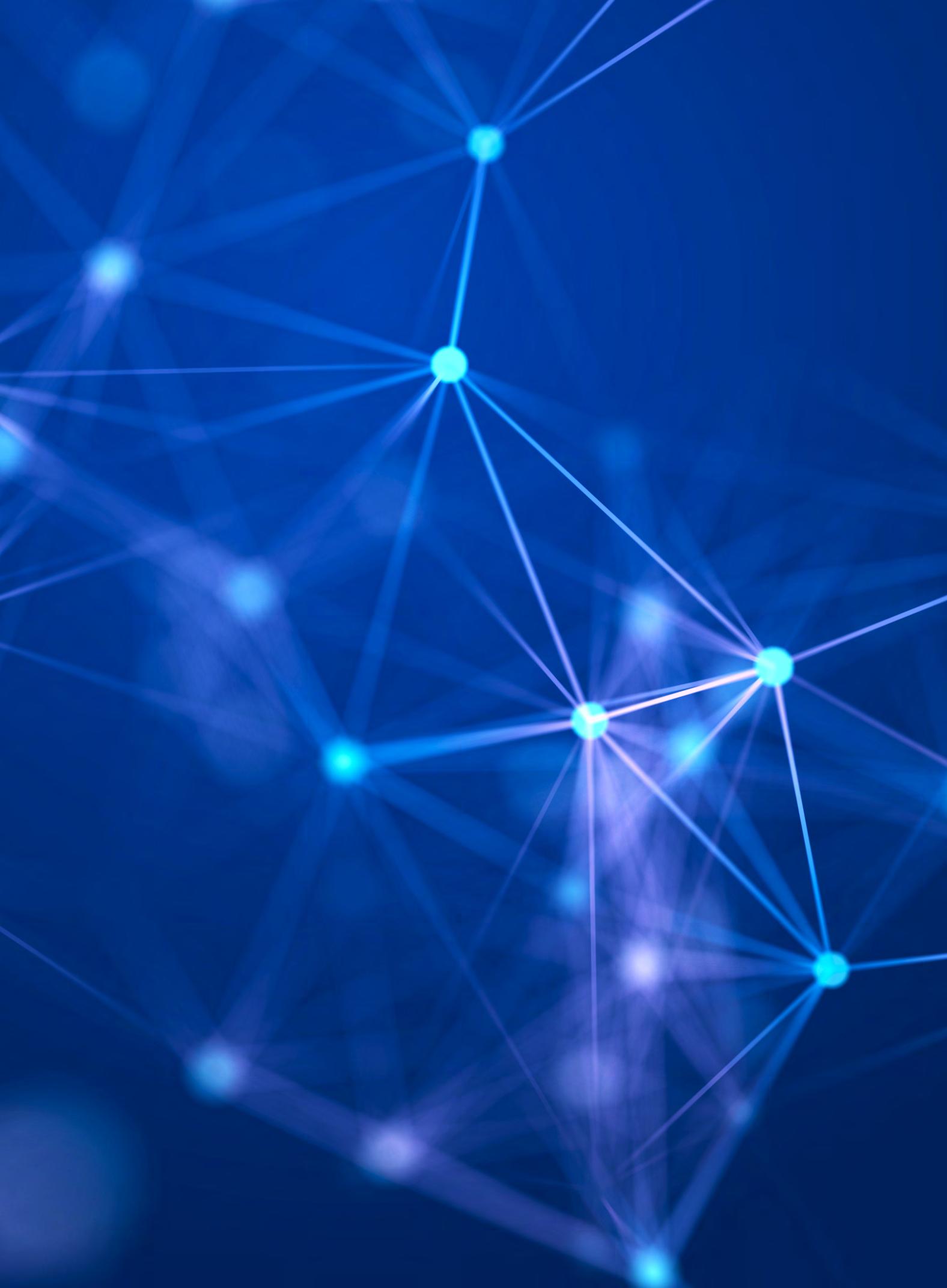
THE TOP 7 MOST IMPORTANT FINTECH OPPORTUNITIES FOR FINANCIAL SERVICES FIRMS IN THE NEXT 5 YEARS AS RATED IN CEFPRO’S FINTECH LEADERS REPORT
Source: CeFPro Fintech Leaders
QUANTIFICATION OF MODEL RISK ACCORDING TO THE PRINCIPLE OF RELATIVE ENTROPY
Risk measurement relies on modeling assumptions, the errors in which expose such models to model risk. This article introduces and applies a tool for quantifying model risk and making risk measurement robust to modeling errors. As simplifying assumptions are inherent to all modeling frameworks, the prime directive of model risk management is to assess vulnerabilities to and consequences of model errors. This article presents a study summary that is consistent with this objective in model risk measurement, focusing on calculating bounds on measures of loss, which can result in a range of model errors within a certain distance of a nominal model for a range of alternative models. To this end, it is proposed to quantify such changes according to the principle of relative entropy. Illustrating the application of this principle the measure for corporate probability-of-default (PD) is the Aikakie Information Criterion (AIC).
QUANTIFYING MODEL RISK ERRORS
In the building of risk models, professionals are subject to errors from model risk; one source being the violation of modeling assumptions. This can be addressed by applying a methodology for the quantification of model risk by using a tool in building models robust to such errors. A key objective of model risk management is to assess the likelihood, exposure, and severity of a model error in that all models rely upon simplifying assumptions. For this reason, a critical component of an effective model risk
framework is the development of bounds upon a model error resulting from the violation of modeling assumptions. This measurement is based upon a reference nominal risk model and is capable of rank ordering the various model risks, as well as indicating which perturbation of the model has a maximal effect upon some risk measure.
In line with the objective of managing model risk in the context of measuring and managing risk in various contexts (e.g., credit or market risk, portfolio management), confidence bounds are calculated around some measure of risk (or loss) spanning model errors in a vicinity of a nominal or reference model defined by a set of alternative models. These bounds can be likened to confidence intervals that quantify sampling error in parameter estimation. However, these bounds are a measure of model robustness that instead measures model error due to the violation of modeling assumptions. In contrast, a standard error estimate conventionally employed in risk modeling does not achieve this objective. The latter construct relies upon an underlying probability generating distribution of errors. It should be noted that in applying relative entropy to model risk measurement, this assumption is not needed but rather a test of whether this assumption is valid.
Meeting the previously stated objective in the context of modeling is achieved by bounding a measure of loss that can, within reason, reflect a level of model error. It is observed that while amongst practitioners, one alternative means of measuring model risk is to consider challenger models; an assessment of estimation error or sensitivity in perturbing
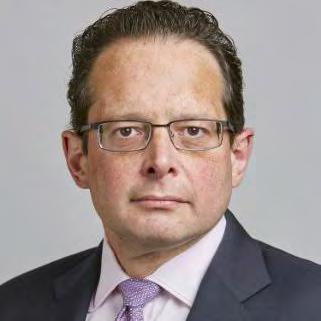
parameters is a more prevalent means of accomplishing this objective, which captures only a very narrow dimension of model risk. In contrast, our methodology transcends the latter aspect to quantify potential model errors, such as incorrect specification of the probability law governing the model without assuming that it is correct.
APPLYING THE PRINCIPLE OF RELATIVE ENTROPY
As these types of model errors under consideration all relate to the likelihood of such error, which is connected to the perturbation of probability laws governing the entire modeling construct, the principle of relative entropy is applied. In Bayesian statistical inference, relative entropy between a posterior and a prior distribution is a measure of information gain when incorporating incremental data. In the context of quantifying model error, relative entropy has the interpretation of a measure of the additional information required for a perturbed model to be considered superior to a champion or null model. Said differently, relative entropy may be interpreted as measuring the credibility of a challenger model. Another valuable feature of this construct is that within a relative entropy constraint, the so-called worst-case alternative (e.g., in this case, a divergence in the distributions of a loss estimate between the models due to ignoring some feature of the alternative model) can be expressed as an exponential change of measure.
Illustrating this application to alternative types of corporate PD models, a comparison of loss distributions is performed of
1- and 3-dear default horizon through-the-cycle (TTC) models suitable for credit underwriting, vs. point-in-time (PIT) models used in early warning systems. These models are built from a long history of borrower-level data sources from Moody’s, COMPUSTAT, and CRSP. The quantification of model risk is performed with respect to the following modeling assumptions:
• Omitted variable bias – leaving out the Merton distance-todefault (DTD) risk factor.
• Misspecification according to neglected interaction effects.
• Misspecification according to an incorrect link function – the Cumulative Log-Log (CLL) as opposed to the Logit.
Distributions of the relative proportional deviation in AIC (RPDAIC) from the base specifications through a simulation exercise is developed. It is observed that omitted variable bias in relation to DTD results in the highest model risk, an incorrectly specified link function has the lowest measured model risk, and neglected interaction effects are intermediate in the quantity of model risk.
CONCLUSIONS
Consistent with this objective in model risk measurement, we calculated bounds on measures of loss that can result over a range of model errors within a certain distance of a nominal model for a range of alternative models. This was accomplished by quantifying such changes according to the principle of relative entropy. The application of this principle was illustrated through a case study where the measure of loss in models for corporate PD considering alternative use cases is the AIC.
There are various implications for model development and validation practice, as well as supervisory policy which can be gleaned from this analysis. First and foremost, this exercise of simulating a loss measure shows we should exercise caution in over-reliance on measures of model fit derived from a single historical dataset. Even if out-of-sample performance is favorable, there could be an unpleasant surprise when adding to the reference datasets when reestimating the models.
Second, from a fitness-for-purpose perspective, it is a better practice to consider the use case for any credit model in establishing the model design. The proposal for measuring model risk better supports this objective in contrast with other approaches. Considering the observations and contributions to the literature, applying the principle of relative entropy provides valuable guidance to model development, model validation, and supervisory practitioners.
Additionally, the discourse has contributed to resolving the debates around which class of credit model is best fit for purpose in large corporate PD modeling applications. This better performance is manifested broadly as both an improved fit to the data and lower measured model risk due to model misspecification.
This piece is a summary of a larger research report, to view the full piece, sign up to CeFPro Connect for free at www.cefpro.com/connect.
CeFPro Connect offers a diverse range of content from iNFRont Magazine, Fintech Leaders, NFR Leaders, videos, industry insights and much more…
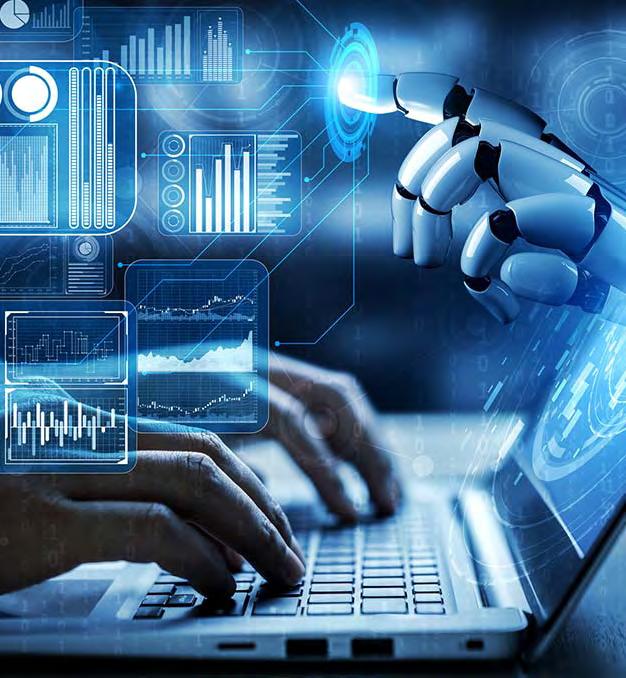
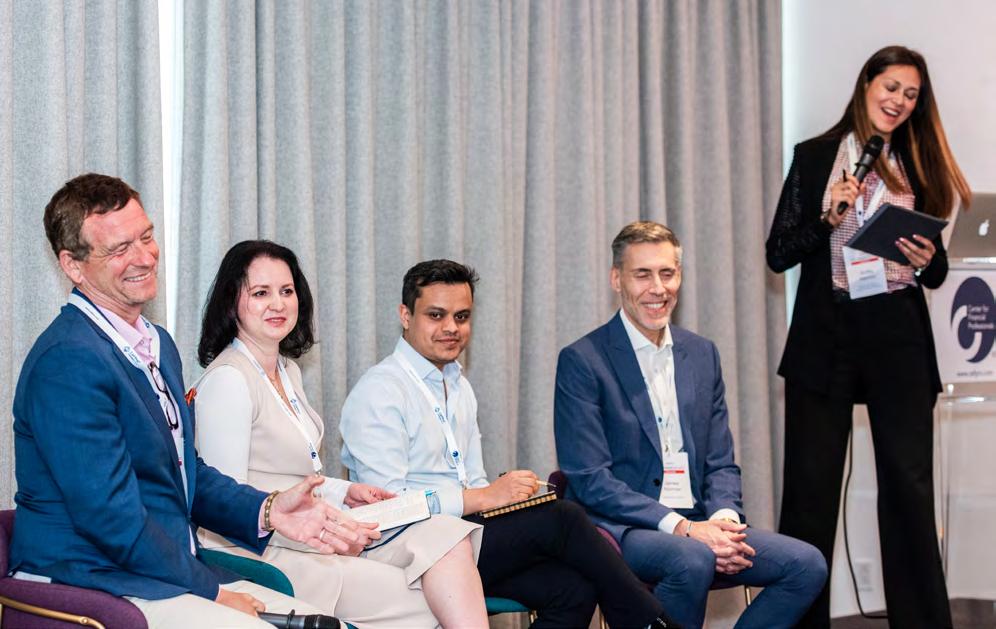
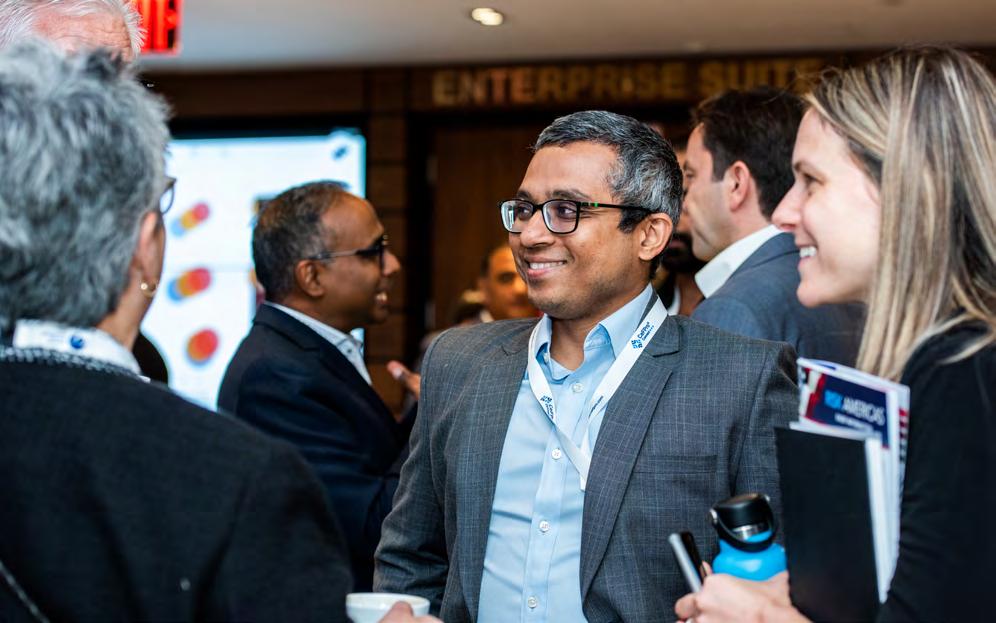



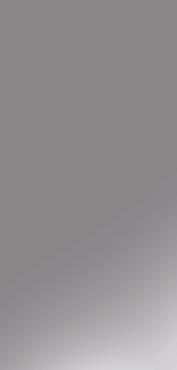
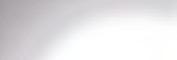




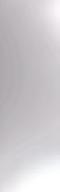
AMERICAS
SHAPING THE RISK LANDSCAPE
At CeFPro’s 12th Annual Risk Americas congress, learning, networking, and discussion opportunities were in high demand. Throughout the two-day event, recent closures of multiple US banks were front of mind for both speakers and attendees, making the chance to share a deeper understanding of risk topics among financial professionals – with a view to preventing and preparing for future events – especially timely.
The four overarching themes for each stream were: Advanced Model and Risk Trends; ESG (Environmental, Social, and Governance) and Climate Risk; Non-Financial Risk; and Market and Financial Risk Trends. Delegates were able to move freely between the different streams following the keynote session from Charles A. Richard III, Senior Vice President of Marketing and Co-Owner at QRM, who issued an important reminder: risk management is the key and must be a part of your culture.
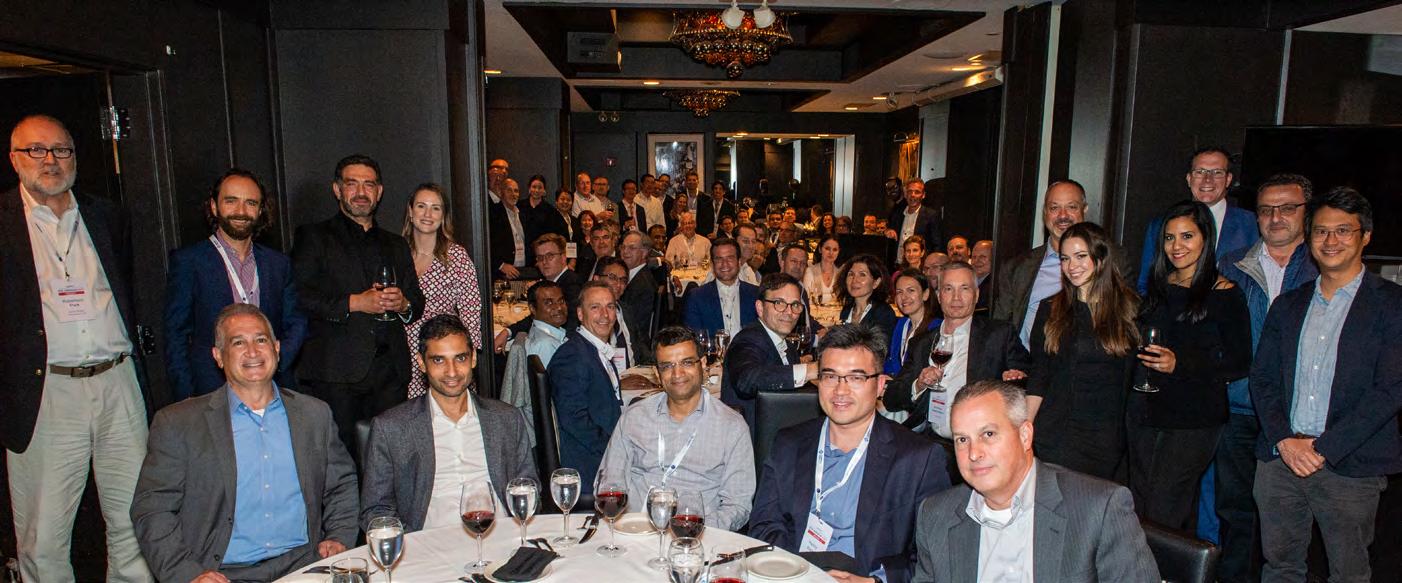
DAY 1: NAVIGATING WFH RISKS AND PREPARING FOR RECESSION
With the ripples of the pandemic lingering within business functions, risk professionals were keen to explore its effects. The ability of businesses to continue operating from a home-based environment is impressive, yet as we learn more about this change to our working culture, there is a realization that it might have come at a cost. The pros and cons of hybrid working were therefore one of the first topics addressed. Discussions centered on various risk factors, such as the difficulty of managing teams from a distance, challenges in safeguarding, lack of misconduct monitoring, and being unaware of colleague welfare issues. And although working from home has benefited personalsocial relationships, it is clear that work-social relationships have been drastically affected.
Risk management discussions continued to flow at the luncheon roundtables, providing an opportunity for attendees to network and discuss topics on a deeper level in a more intimate setting with smaller groups. With seven roundtables covering different topics quickly filling up, and people grabbing chairs to sit nearby and listen in, this format proved a resounding success.
As the second half of the day started, attendees were eager to delve further into the financial risk landscape. One stream explored
how to monitor risk and prepare for a future recessionary environment. In answer to this question, the first suggestion provided by the speaker was around the importance of looking at current information and questioning everything. Patterns change over time, making it even more relevant to think outside the box. Another key point made was the value of inter-management communication with the board to form essential pathways between those challenging the problem and those actioning it.
Day one closed with a networking drinks reception, allowing attendees, speakers and event partners to end the day in a relaxed environment while reflecting on lessons learnt.
DAY 2: MANAGING EMERGING RISK THREATS – ESG AND TECHNOLOGY
Tom Wipf, Vice Chairman at Morgan Stanley, opened the second day’s sessions by sharing lessons learned across the industry using real-life events. By gathering data and conducting post-mortems of catalyst events, firms can better understand how certain events happened and take steps to ensure they do not occur again.
Discussions then moved onto how risk management needs to be at the center of every conversation. As the advancement of technology continues at full speed ahead, strategic risk management needs to run adjacent. A holistic overview of risk was explored in the keynote panel discussion, which delved into the increased level of technology risk. With the introduction of mobile banking, what used to take weeks can now be done in a few hours, leaving less time for errors to be rectified. The speed of information is a genuine benefit, yet, with the way social media is currently functioning, a tweet could crash a bank.
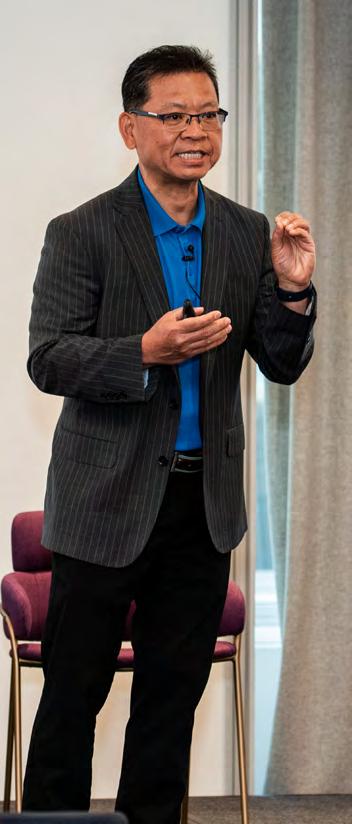
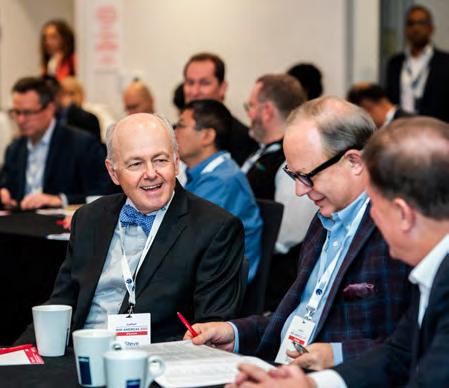
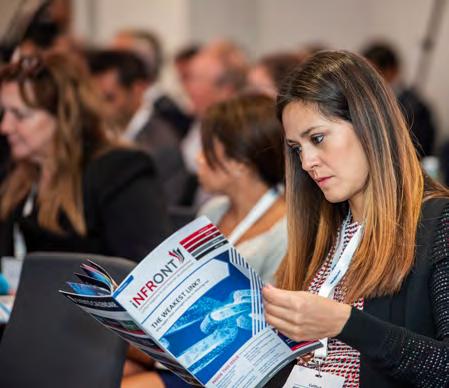
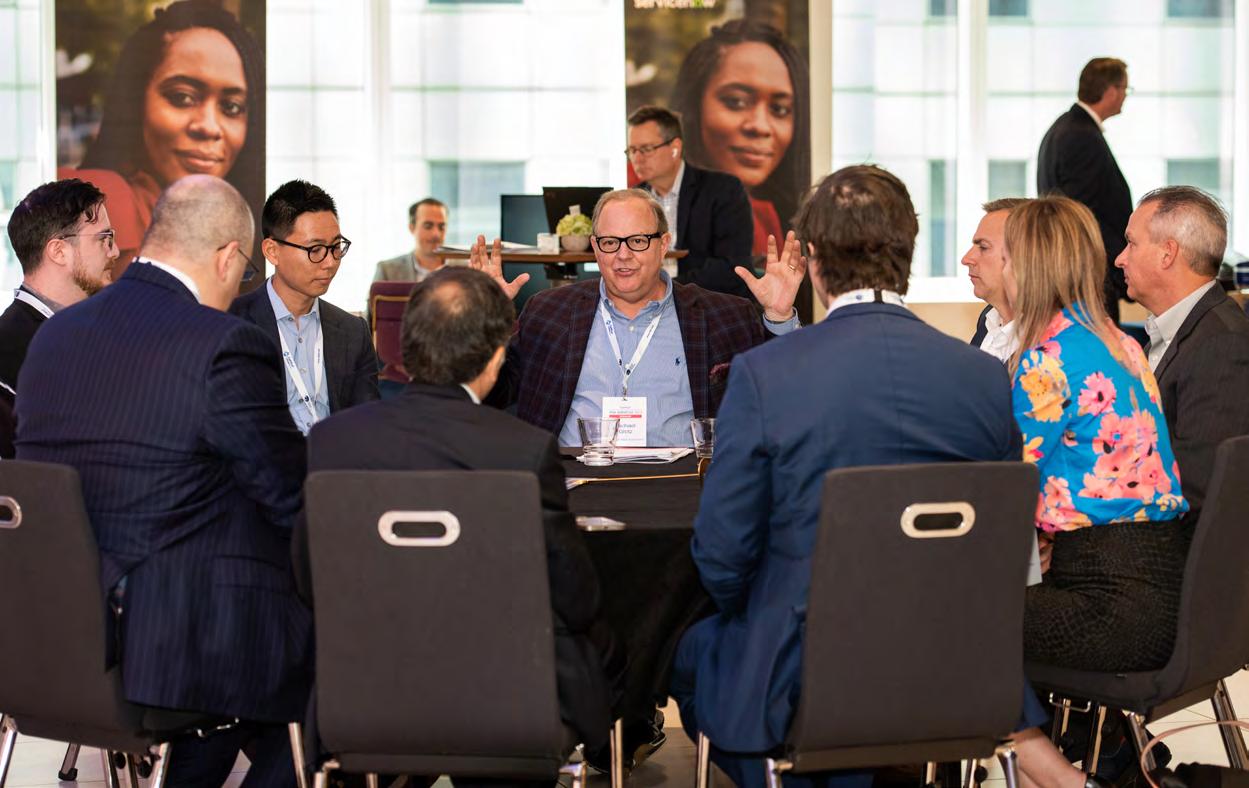
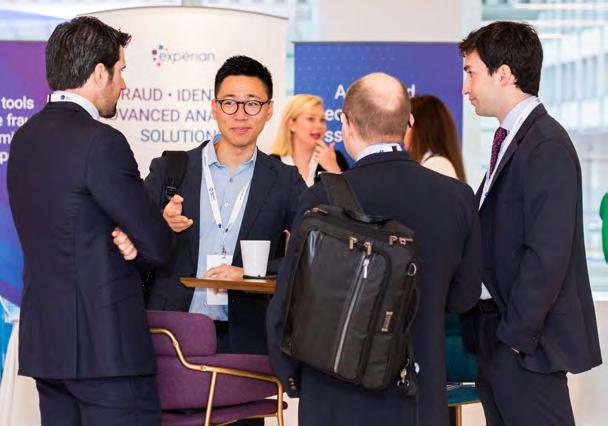
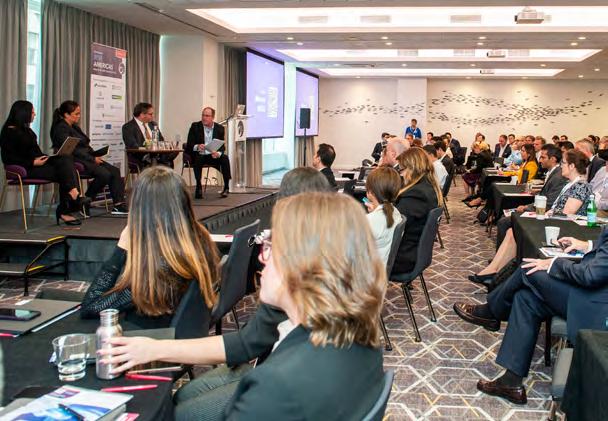
Another significant focus that businesses, especially in the US, are facing is ESG risk. US businesses have started to act on climate standards but are still doing less than other countries, such as the UK. The speaker explained how the EU and UK are more advanced and more invasive in their push for environmental change. It was interesting to note that those US banks that have a presence in the UK must adapt to the guidance provided by the UK government. Therefore, much of the groundwork has already been done. Communication across jurisdictions is clearly needed to enhance development within the climate sector; regardless of US regulators’ demands, it is ideal to have higher standards company-wide.
As the day ended and vendors started to pack away, attendees continued to network. Small but crucial conversations like these are vital in assisting the development of the financial services industry, with effective communication being fundamental for growth. Over the two days of Risk Americas, it was clear that the opportunity provided by the event for much-needed knowledge sharing and communication is of real benefit to those in risk management.
If you missed out on Risk Americas 2023, register early to join us for 2024!
Risk Americas is researched with over 100 industry experts, guaranteeing a topical and insightful agenda. Set to take place in May in New York City, join us early and secure your seat at Risk Americas 2024!
Call for research and speakers is also open - if you are interested in helping shape the agenda, or being considered for a speaking slot, please email production@cefpro.com.
Visit the website for more key highlights at www.risk-americas.com
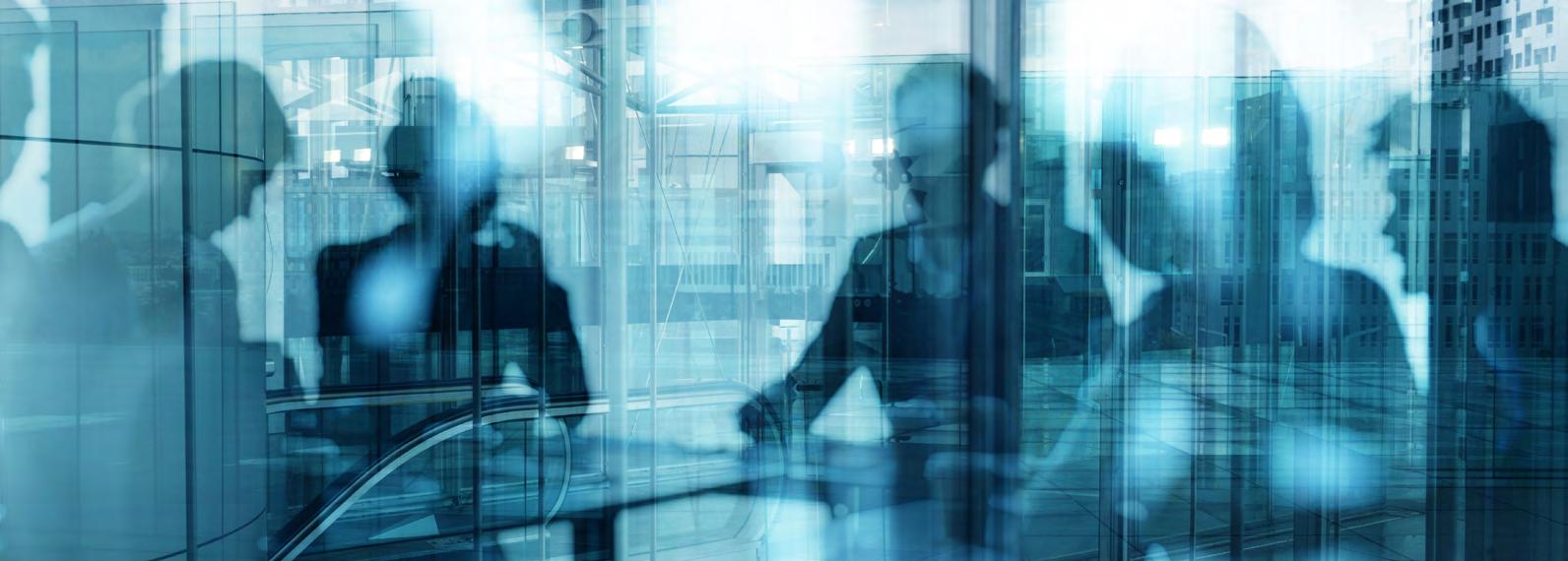
USING MODEL RISK MANAGEMENT PRINCIPLES TO MANAGE AI RISK
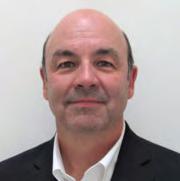
The rise of artificial intelligence (AI) and automation has transformed various aspects of the financial services industry, including the deployment of chatbots, AI tools, and other automations in banking. While these technologies offer numerous benefits, they also pose risks, particularly concerning model risk management (MRM). US regulatory agencies, such as the Consumer Financial Protection Bureau (CFPB), have expressed concerns about potential harm and bias resulting from poorly constructed or biased AI models.
As financial institutions increasingly deploy chatbots and other AI technologies, it becomes crucial to establish governance control frameworks specific to these applications. While MRM may not have sole responsibility for overseeing chatbots and AI systems, it possesses the necessary skillset and expertise to collaborate with other risk groups in identifying, inventorying, and monitoring these technologies.
Managing chatbox risk
The inclusion of chatbots, other AI tools, and applications in a model inventory is essential for effective MRM, aligning with a useful concept called the model universe, as outlined in the
FHFA’S AB 2013-07. While chatbots and AI systems may not fit the traditional definition of models, they possess characteristics that make them susceptible to risks and require adequate oversight.
Chatbots and AI systems introduce unique risks that require dedicated governance control frameworks. These technologies rely on algorithms, machine learning, and natural language processing, which can introduce biases, privacy concerns, and the potential for errors or malicious manipulation. Implementing governance control frameworks ensures proper risk identification, assessment, and mitigation specific to these technologies.
While MRM may not have direct ownership over chatbots and AI systems, it possesses valuable expertise in risk identification, evaluation, and management. By collaborating with other risk groups, such as operational risk, technology risk, and compliance, MRM can contribute its knowledge and experience in developing governance control frameworks that align with existing risk frameworks and address the unique risks posed by chatbots and AI.
Ensuring consistency
Including chatbots and AI within the purview of governance control frameworks ensures consistency and standardization across the institution. By leveraging the established processes and practices of MRM, financial institutions can ensure that chatbots and AI systems are subjected to a consistent risk assessment methodology, testing, and ongoing monitoring. This helps in maintaining transparency, accountability, and regulatory compliance. It may also be the case that unique policies and programs will not need to be written to address every instance of AI technology, e.g., chatbots, robotic process automation (RPA), low-code/ no-code AI tools, etc.
Addressing regulatory concerns
Regulatory agencies are increasingly focusing on the risks associated with AI technologies, emphasizing the need for effective governance and control frameworks. By proactively implementing these frameworks, financial institutions can demonstrate their commitment to addressing regulatory expectations and protecting the interests of customers and stakeholders.
Including chatbots and AI in governance control frameworks provides a comprehensive approach to risk management. While other risk groups may focus on specific aspects, such as technology risk or operational risk, MRM can contribute its expertise to ensure that the unique characteristics and risks associated with chatbots and AI are adequately addressed.
Implementing governance control frameworks for chatbots and other AI technologies is essential to address their unique risks. While MRM may not bear sole responsibility for these technologies, it can collaborate with other risk groups to leverage its expertise and contribute to the development and implementation of comprehensive governance frameworks. By doing so, financial institutions can ensure transparency, consistency, and effective risk management in the deployment of chatbots and AI systems, safeguarding against potential biases, errors, and other risks associated with these technologies.
Hear appraoches to AI and machine learning, alongside model risk management practices at Non-Financial and Operational Risk USA. Taking place in NYC on October 4-5, this event will also run concurrently with Climate Risk USA in the same venue. Visit www.cefpro.com/oprisk-usa
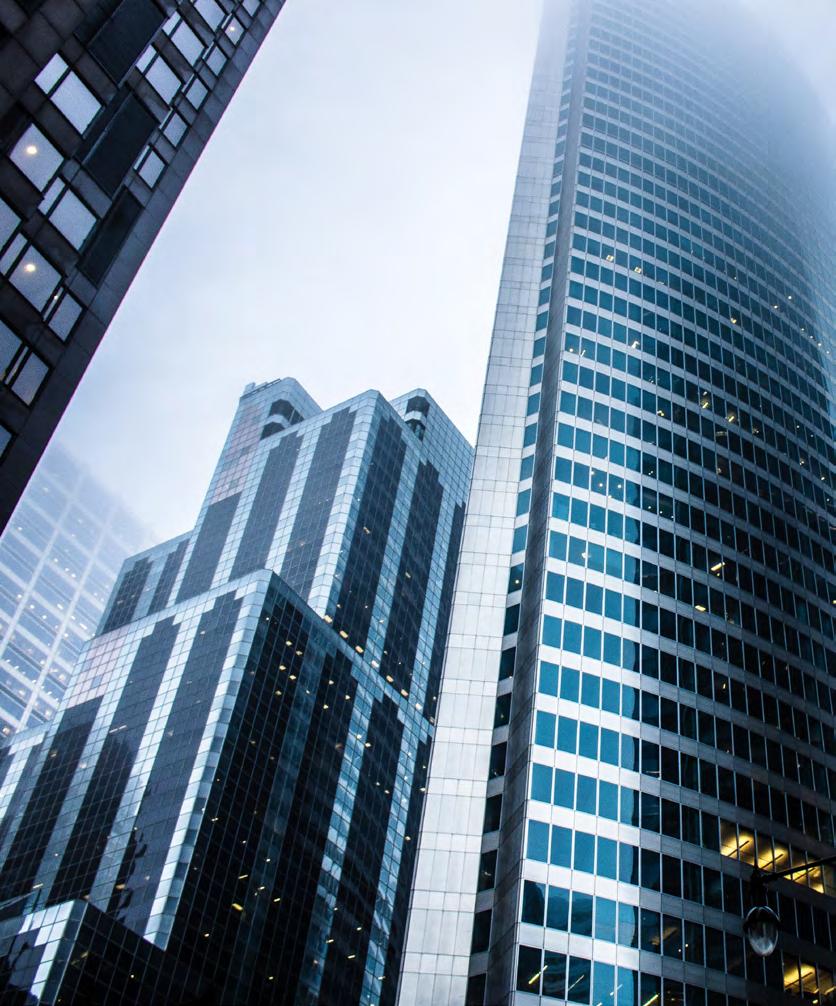
GLOBAL THIRD PARTY RISK MANAGEMENT REPORT OUT NOW!
Join risk professionals around the world as they utilize CeFPro Research’s valuable resource to analyze their institution's TPRM team, benchmark against peers, and gain a comprehensive understanding of the relatively immature third-party risk sector.
Informed by the responses of 200+ knowledgeable third party risk professionals, our report highlighted disparities and divergent approaches throughout the industry. As third-party risk management continues to gain traction as organizations across industries remain increasingly reliant on outsourced activities and services, now more than ever, effective oversight and understanding of supply chains are critical.
Regulators globally appear to acknowledge the risks and are imposing more stringent requirements, with particular focus in some geographies on critical services and looking beyond third parties to understand the risks further than the direct relationship.
This research is a must-read for professionals looking to obtain the expertise needed to shape institutional strategies and excel their career.
Key findings
(Figure 4)
45.6% of respondents have a TPRM team of only 1-5 members 45.6%
(Figure 5)
71.5%
There is no separate oversight committee for intragroup arrangements for 71.5% of survey respondents.
(Figure 6)
Regulatory pressure was viewed as the most important or significant obstacle in managing third-party risk for 2023. 78%
(Figure 7)
50/50 votes towards whether technology developments like ChatGPT and Web3 were important when managing third-party risk in 2023. 50%
Immerse yourself in our global research study and discover:
Is the way your institutions TPRM team in alignment with your peers? Are there any structural differences that you can learn from?
The top opportunities, challenges, and obstacles with managing third party risk.
With cloud becoming a critical third party to most institutions, how is the industry managing associated risks and remediating incidents?
Are other institutions categorizing TPRM the same as yours? Are there any benefits to be obtained from the way they are doing it?
Is there an alignment across institutions on how they conduct assessments and due diligence practices?
What impacts have global events had over TPRM? The future is not certain in such a turbulent environment of today, and lessons can be learned going forward
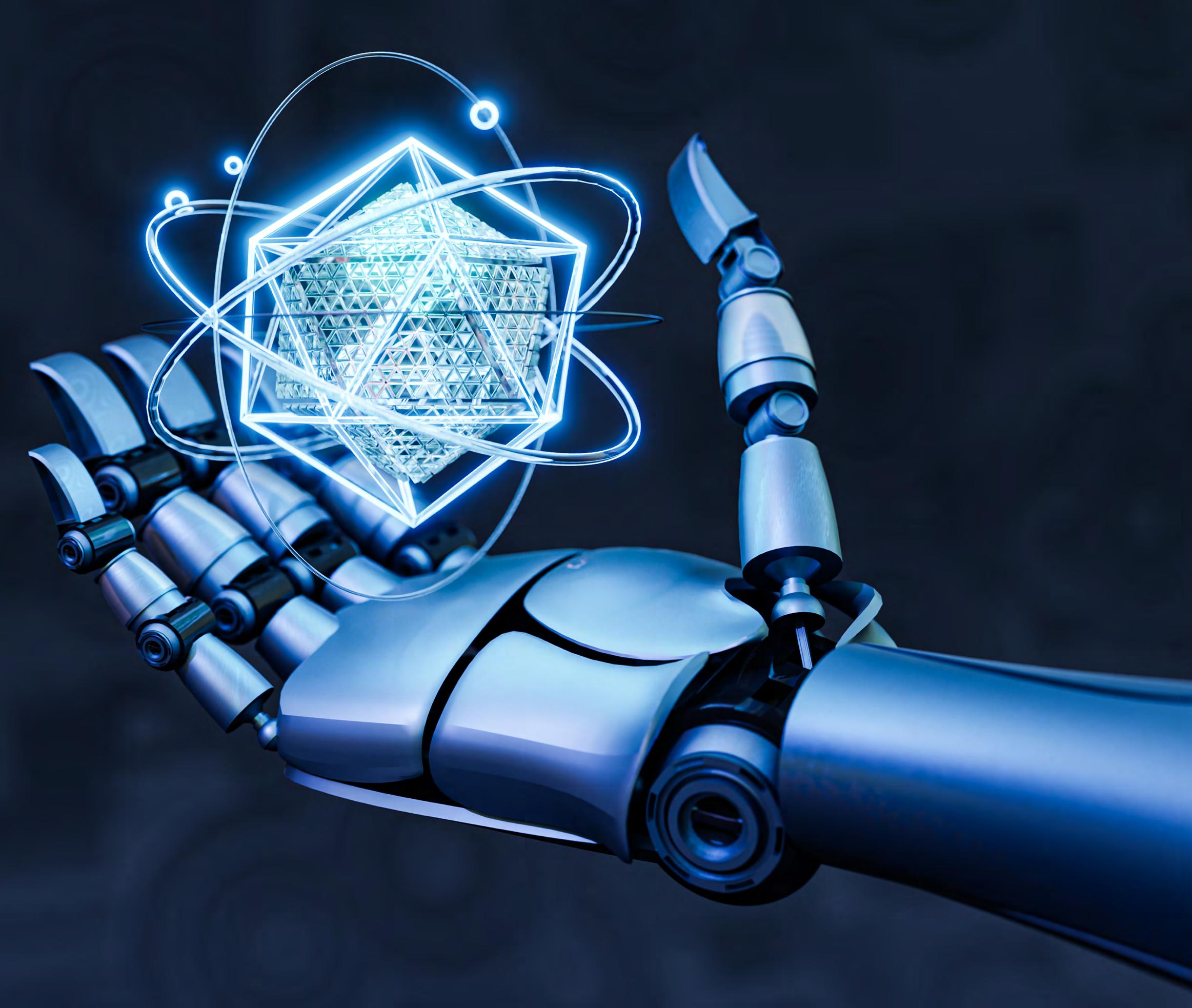
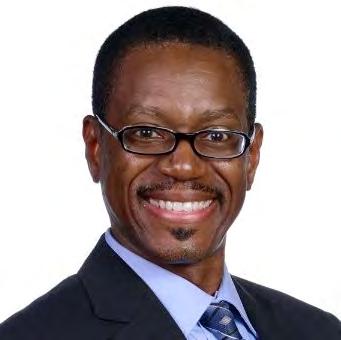
THE POWER OF GENERATIVE AI WITHIN FINANCIAL ORGANIZATIONS
What are some of the key benefits of using generative AI (GenAI) in financial institutions?
Model risk managers write numerous policies, procedures, and reports but don’t always have an aptitude for writing, as they typically prefer quantitative analysis. GenAI and by extension large language models (LLM) can assist model validators with the writing aspects of their role. Once an analysis model is complete, data can be put into an LLM to help craft the model validation report. Analysts will still be involved in the process, but as
humans, we are prone to spelling and grammatical errors. LLM typically does not make these types of mistakes, improving the final output. Additionally, AI and LLM platforms are built on the collective wisdom of the whole world via the internet, which has more knowledge than any one analyst.
At my institution, we’ve run a few tests to see how much of a benefit AI can provide. We asked one analyst to write about a model risk management topic without using AI, and then asked another analyst to do the same thing using AI. When comparing the amount of written work produced within a defined timeframe, the analyst who used AI wrote at least three times more
than the analyst who didn’t. This shows the major difference and importance of using AI and where some of those efficiencies can be leveraged.
What are the potential pitfalls of GenAI?
Although I anticipate GenAI affecting quite a few jobs in banking, finance, and across society in general, humans still need to be involved with this technology. At times, generative AI, particularly LLM, can produce nonsensical results. Another big issue with LLM is that it is prone to hallucinations; this is when LLM produces text which looks and sounds
authoritative but is in fact inaccurate information. This is due to the LLM being trained on information from the internet, which is not always factually correct. If there are problems with the training data, then its output will be inaccurate, as LLM can only produce data on what it has learned. Therefore, LLM writings must be double-checked to make sure the results are accurate, factual, and make logical sense. AI eases the writing task, but a human must still be involved in the process to review the function and check that the AI is generating the required information.
In terms of the risks associated with this, I don’t think they are many. Whether an AI or a human made the mistake, it is good practice to have someone review a report before it’s widely disseminated. At present, the benefits of using the technology far outweigh the risks when it comes to writing.
On the other hand, there are some concerns about data privacy. When a host organization allows you to tap into their models, they have the ability to see what you’re doing. Some organizations train their LLM based on what they see you doing with their models. For example, if you ask an LLM to help write a paragraph about asset liability management models, the owner of the LLM can see which prompts were put into their system and use that data in any way they want to. Therefore, there are some data privacy concerns that have yet to be addressed.
How can GenAI be used for model risk management (MRM)?
Across the whole financial services industry, including my institution, teams have experimented with generative AI in pilot projects. It is as yet unclear if GenAI has been deployed widely within organizations as experiments are ongoing. However, generative AI goes beyond MRM; banks can use sophisticated GenAI, like chatbots, to interact with customer demands 24 hours a day. As far as MRM is concerned, then assisting with personnel write-ups and the creation of model validation reports and policies and procedures for model areas are some of the tasks in which it could be used.
Where do you see some key areas for consideration in regard to validating GenAI models?
Quantitative techniques can be used to validate the results of generative AI models and to check their accuracy, as well measure errors. If organizations are using GenAI, then model inventory inclusion is a must, as it may be subject to being validated, invalidated, and risk rating assigned. The models are complicated and advanced, so having in-depth knowledge about them is vital. Ask yourself: do you really understand what the model is doing? Can you explain it to an outsider? Can you assess the transparency of the model? Is the model outputting biased or discriminatory information? Have you checked the data? Is the AI hallucinating or producing inaccurate or misleading information? Many areas need to be checked when validating generative AI models.
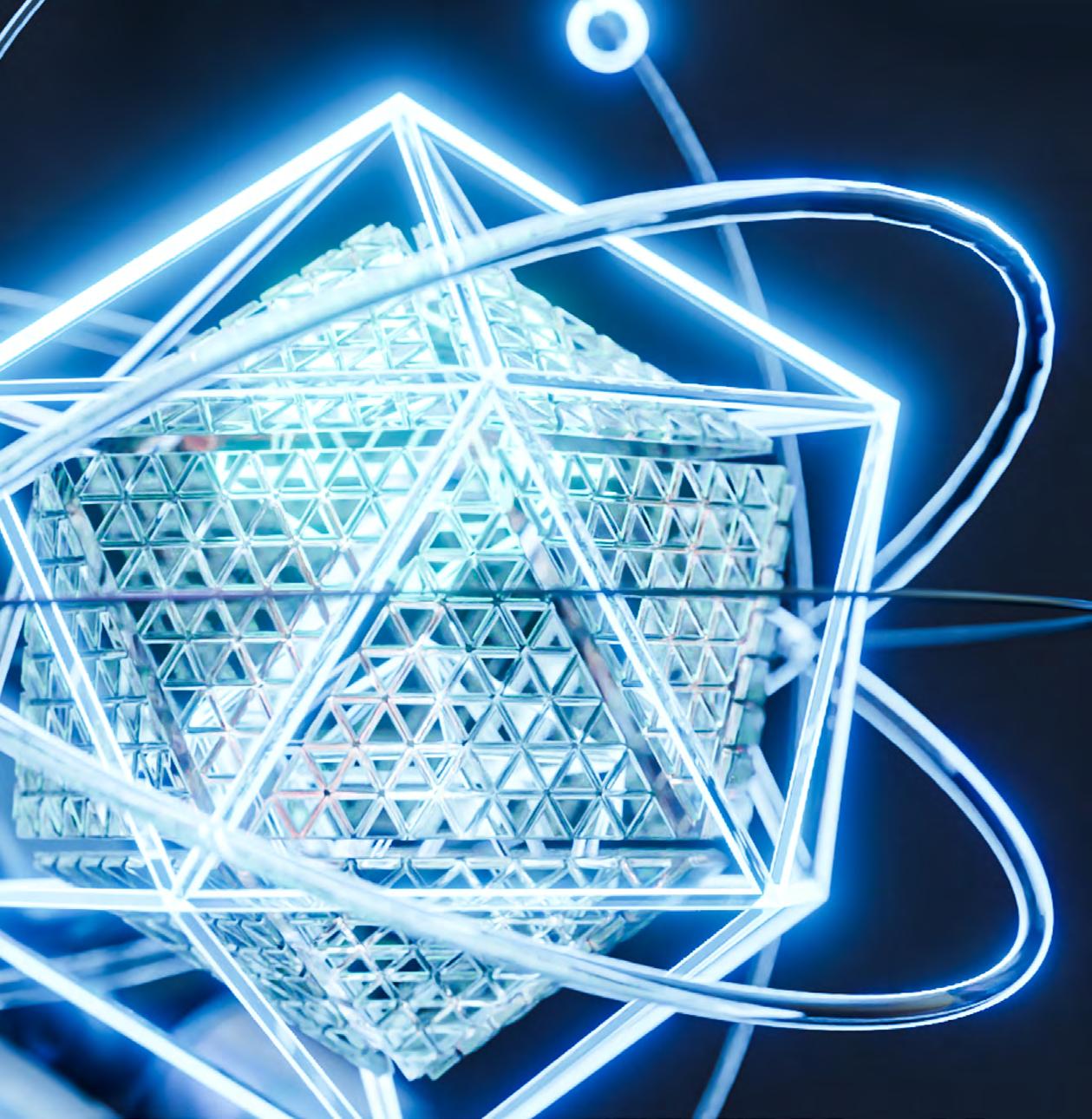
Are financial institutions developing their own generative AI?
I am unaware of any organizations building their own LLM, as it requires a lot of computing power, resources, and money. Typically, companies are using technology developed by vendors. For instance, one popular vendor is OpenAI, which created ChatGPT and an underlying LLM which they refer to as 3.5 or 3, or 4, indicating the progression of advancement in the model. As open source, anyone with an API can gain access to the model, so there is currently no need for institutions to build their own language model. This means they must decide which vendor they trust to use for their organization.
What do you see as the future of AI and LLM within financial services? How broad is the scope for its usage and how could this impact banks’ operations?
As generative AI develops and advances, the industry will continue to embrace it. GenAI has the potential to improve the quality of work produced and increase productivity, making a dramatic difference to the operations of financial institutions. It is therefore a good idea for all institutions to explore how they can leverage this new technology. We are still in the trial phase of experimenting where to find its best use, but one thing GenAI can do is help people to do their job better. The automation of AI can be used for mundane and repetitive work, leaving humans to carry out more high-level, impactful tasks. To achieve this, institutions must call for training to ensure that their employees are comfortable using generative AI.
Generative AI and Large Language models are being used across industries, hear how other industries are leveraging technology across their supply chain management at Supply Chain & TPRM USA: Cross Sector.
A unique insight into how a range of industries leverage technology and apply to a range of third-party and supply chain issues.
For full information visit: www.cefpro.com/supplychain-cross
THE RISE OF NON-VALIDATION MODEL RISK TEAMS
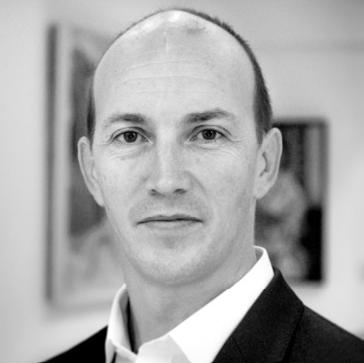
As banks continue to work towards full compliance with new and upcoming regulations – whether outsourced to consultants or kept in-house – the role of non-validation model risk teams is becoming increasingly important. The average model risk function, at least in global banks, has gone from a few teams that validate models, to being more akin to areas overseeing other primary risk stripes like credit, market, and operational risk.
In particular, the role of non-validation model risk teams is increasingly important to a firm’s ability to manage model risk efficiently and effectively. Governance teams are responsible for designing, implementing, and embedding model risk frameworks, and a lot more besides. These are no longer tasks that can be carried out quickly by validators, even in smaller firms. Instead, highly specialized teams are required, with close ties to validators and the ability to communicate and collaborate with the rest of the bank. Failures in this space can have expensive consequences.
GLOBAL VARIATIONS
Prior to 2011, when the US regulators (OCC, FRB, and FDIC) published SR11-07, model risk teams in even the largest global banks were typically two distinct entities: one focused on credit models and the other on endof-day valuation (pricing) models. These teams were often siloed, making it difficult to get a holistic view of model risk across the bank. Since then, several regulations have shaped and steered banks in different ways. While there is a consistent direction of travel, the specific requirements vary depending on the bank’s primary location:
• US – Beyond SR11-07, the OCC Handbook on Model Risk Management provides additional guidance, including on new types of models such as AI/ML.
• EU – There is no overarching model risk guidance, but the report summarizing the findings of the Targeted Review of Internal Models (April 2021, where 55 institutions were included in the horizontal analysis of general topics) flagged that:
‘Few institutions have a comprehensive framework for model risk management in place, and in cases where there is one, it often requires improvement.’
• UK – The regulator has laid out a clear trajectory towards principlebased model risk oversight, first through SS3/18 (April 2018 – ‘Model risk management principles for stress testing’) and more recently in SS1/23 (May 2023 – ‘Model risk management principles for banks’).
• Rest of the world – While there is variation in the rest of the regulatory landscape for model risk management, the direction of travel is consistent. Canada’s OSFI (Office of the Superintendent of Financial Institutions) is a noteworthy example, with its Guideline-E23 and plans to update it at the end of 2023. OSFI is also arguably leading the way with its excellent collaborative paper on AI models: ‘Financial Industry
Forum on Artificial Intelligence: A Canadian Perspective on Responsible AI’.
BEYOND RIGHT AND WRONG
As required by SR11-07, validation is just one tool to help banks manage model risk. It makes it very clear that model risk is not the risk of a model being ‘wrong’, but the risk of users not fully understanding how wrong it is (the uncertainty in the output), as well as the reasons why (the drivers of that uncertainty). It is also important to consider risk not just at the level of model usage but in aggregate and examine how model risk interacts with, and can influence and amplify, other risk categories.
As such, the OCC, FRB, and FDIC collaborated to note the need for a model risk framework that goes beyond validation. They emphasized that a comprehensive framework should also cover development, implementation, and use, as well as governance, stating the importance that governance
‘…sets an effective framework with defined roles and responsibilities for clear communication of model limitations and assumptions, as well as the authority to restrict model usage.’
To meet these requirements, banks are increasingly promoting model risk to a Level 1 risk, alongside credit, market, liquidity, and operational risks. They are also creating central model risk functions, bringing all validators under a single head. These functions often include a governance team/s to manage all non-validation aspects, such as risk appetite, reporting, and training.
THE ROLE OF GOVERNANCE
The scope of model risk governance teams varies depending on the requirements of all risk stripes (typically defined by the Enterprise Risk Management function), but they should aim to follow three simple objectives:
1. Minimize model risk across the bank.
2. Optimize the length of time validators spend validating models.
3. Proactively lead collaboration with other risk stripes.
To achieve these objectives, some key tasks need to be carried out. These tasks have historically been completed by validators. However, many banks are now seeing the benefits of using a highly-skilled, collaborative governance team, often supported by specialist consultants. This is because governance teams (and consultants) can bring a broader perspective to the table and can help to ensure that model risk is considered in all decision-making processes.
The key tasks include, but are not limited to:
• Helping the bank’s senior management set the risk appetite.
• Authoring the bank’s policy and procedures.
• Managing (and often overseeing the development of) the model inventory, including processes to ensure its completeness.
• Executing and/or supporting risk assessments (including tiering) of the models.
• Model approval governance.
•
• Defining and delivering model risk reporting.
• Consequence management.
• Team administration, including resource management (supporting hiring, validation planning, etc.).
• Audit interactions (internal and external).
• Regulatory understanding, including gap compliance.
• Strategy (forward-looking).
• Training, including for senior management and boards.
MATCHING TALENT TO TASKS
The key to managing all risks effectively is having the right people in the right roles with the appropriate authority to fulfill their responsibilities, and non-validation roles in model risk teams are no exception. Ideally, these roles should be filled by ex-developers or validators, but they do not have to be. However, they do need to have a good understanding of the demands and expectations of developers and validators.
Each of these tasks, if done poorly, has the potential to generate audit and/ or regulatory findings. Less obvious consequences include inefficiencies and decreased validator retention rates. Model risk teams have always needed some level of administrative support, and this has not changed. It makes sense that if you hire someone with a PhD in mathematics or physics, you will want to maximize the time they spend applying their knowledge and skills. Initially, this support may have been for relatively simple tasks like planning. However, as model risk teams evolve, so do the non-validation requirements, and so does the risk of not optimizing teams and resources. In a job market where the best quantitative analysts are in high
demand, banks that optimize their resources stand a better chance of retaining their staff and gaining a competitive edge.
COLLABORATION IS THE ROUTE TO SUCCESS
As well as implementing the latest regulations, model risk teams are facing increased demands, both from areas of the bank that are new to model risk (such as anti-money laundering, anti-fraud, and trader surveillance) and new risk areas (such as ESG and AI/ML). These heterogeneous areas require a multidisciplinary approach to find creative and innovative solutions. The common challenges faced by these teams generate a greater need for collaboration to build consensus and support for solutions, both within the bank and with other risk stripes.
As banks continue to work towards full compliance with new and upcoming regulations, the role of non-validation model risk teams is therefore becoming increasingly important. These teams are responsible for a wide range of activities that are essential for managing model risk. As the complexity and scope of models – and the number of regulations governing them – continue to grow, the importance of having the right people and/or the right consultants supporting non-validation model risk teams will only increase.
See where Model Risk ranked in the top non-financial risk rankings as part of NFR Leaders 2023. View the full report and findings for free with your CeFPro Connect account. Sign up and view the report at www.cefpro.com/connect
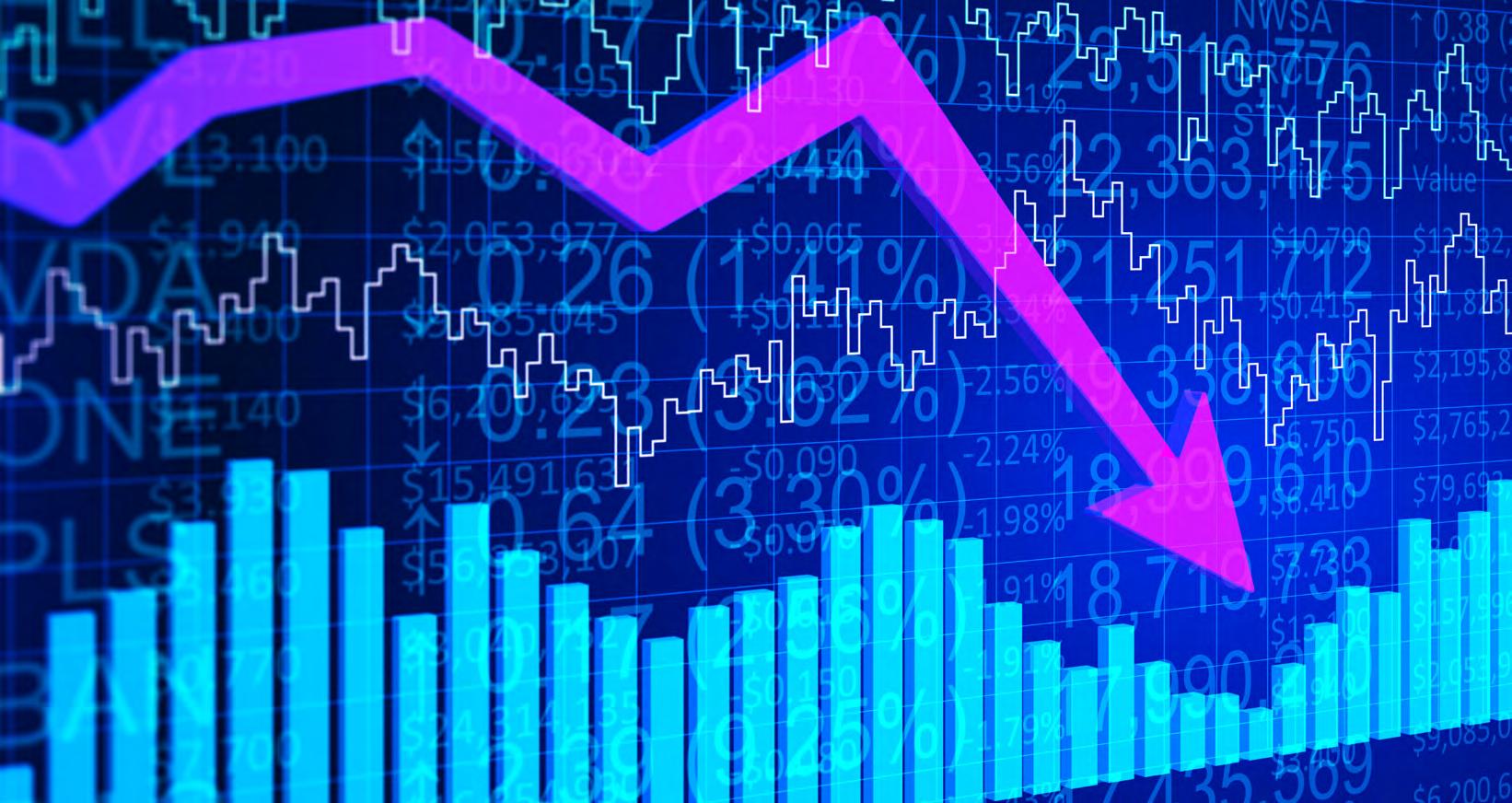
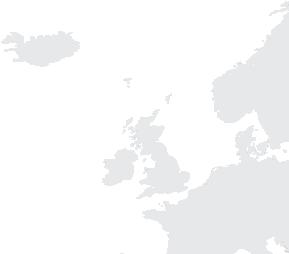
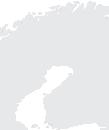
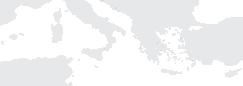
RISK
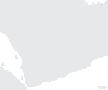
EMEA 2023
THE IMPORTANCE OF STRESS TESTING IN AN UNCERTAIN WORLD
THE IMPORTANCE OF REMAINING AGILE
Taking place in London in June, Risk EMEA 2023 provided a well-rounded mix of education, networking, and the chance to meet industry-leading vendors across multiple siloes of risk management. The two-day event featured insight from over 70 thought leaders across multiple sessions, as well as a networking drinks reception, lunchtime briefing, and interactive Q&As with speakers and event partners.
The day one keynote session featured two industry experts from MUFG and Starling Bank, who discussed the management of economic risks and the long-term impacts of global crises. The discussion proved very timely and audience members listened intently, engaging interactively with pertinent questions to the speakers.
Scenario analysis and stress testing were front and center, described as being ‘the key tools for modern risk management’. The panel explained that they do not need to be complex blackbox models and can be as simple as outlining an approach and assessments, and then testing assumptions and sensitivities. They can help firms understand sensitives and determine whether recalibrations are required, or whether liquidity profiles need to be raised, for example.
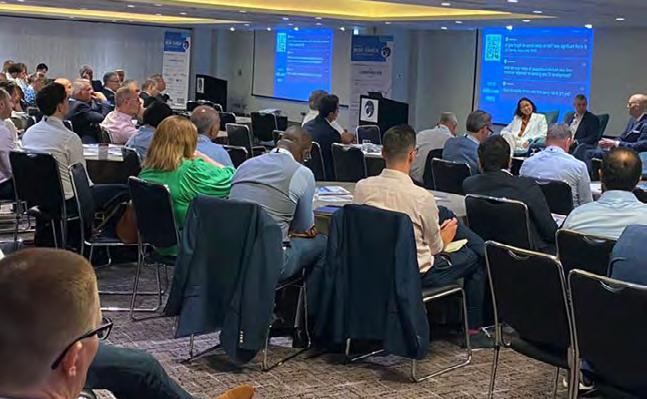
The panel also outlined that the scenarios and process need to remain agile in order to be effective; they will need to change and adapt over time to provide timely and accurate data. It is therefore important to determine whether key processes are still relevant given the changing landscape, acting as a tool to identify potential emerging risks.
Stress testing was seen as key to understanding the impacts and consequences of events. Given the regulatory mandate as a result of the financial crisis, a culture of stress testing has been embedded within banks, with management taking the results very seriously. The culture of stress testing was described as being ‘in our fabric’. The panel stated that organizations should leverage this to develop unique scenarios to develop definitive action plans, developing a playbook of sorts to guide quick decision-making in stress events.
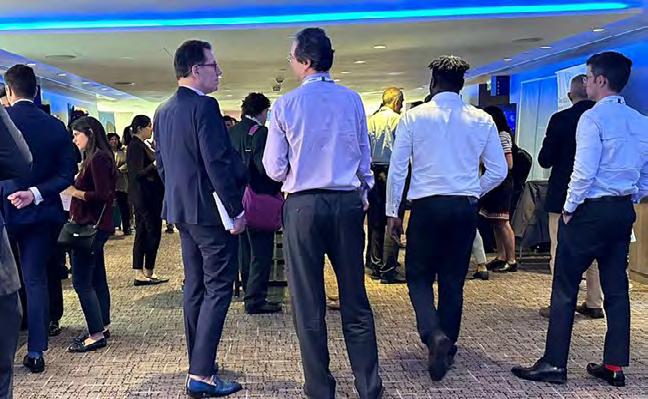
SHAPING FUTURE PLANS
The panel moved on to the future of risk management and whether stress testing holds a place or if more can be done to build on the current stress testing practices. One panelist described the risk management role as being an ‘essential advisor to management and the challenges of the business, to identify future risks and ensure management has plans to address that... risk management is all about stress testing’. Organizations should be leveraging stress testing to help with future planning; it should no longer be a reporting function but an advisory tool.
Stress testing also should go beyond the understood risk register to the assessment and management of unidentified risks. The expansion of the function should look towards developing capabilities to create an emerging risk management function with a truly forward-looking view.
The panel concluded with their views that stress testing and scenario analysis need to become further developed as a BAU tool. No longer just a regulatory exercise, they are an essential risk management tool, embedded in risk culture. Results do not always have to result in increases in capital or liquidity requirements; instead, they can be used as a risk identifier, helping firms become more forward-looking and better aware of emerging risks.
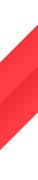



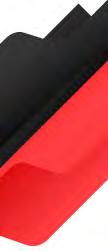



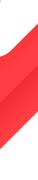
WHAT ROLE WILL AI AND MACHINE LEARNING PLAY IN THE FUTURE OF FINANCIAL SERVICES?
As the industry continues to advance its journey towards increased digitalization and reliance on new technologies, AI and machine learning gain more and more traction. What was once a ‘buzzword’ is now an integrated technology in many organizations and risk functions. Below we explore where some organizations are seeing or utilizing the potential
Irfan Kazi Director, Advanced Analytics, Internal AuditCIBC
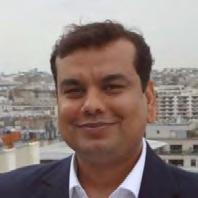
“Artificial intelligence and machine learning have and will continue to drastically change the way we work, and how we serve our clients. For the next couple of years, automation of redundant processes will continue to be key. Financial institutions (FIs) will be able to offer clientcentric products (not pre-packed deals) and automated changes in product characteristics based on changing client profiles e.g., personalized financial/investment plans, cards, mortgages, and insurance underwriting. The sooner FIs open their doors to AI and ML and embrace it, the quicker they will fail, the quicker they will learn, and the quicker they will ultimately succeed.”
Catherine Havasi Chief of Innovation & TechnologyStrategy
Babel
Street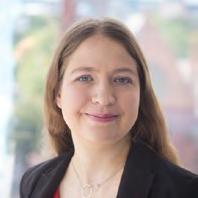
“Beyond customer experience, AI can be useful in compliance and in combating fraud. The most powerful use cases will be where AI acts as a force multiplier for an analyst. If AI can find the right person, determine if information points to a risk that matters, and make sure that the individual plays a role of interest in the scenario, then AI can cut down the false positives and let the human analyst focus on the inputs and decisions that truly matter. This will become increasingly important where computer-generated content messes with our signal-to-noise ratio.”
Dr. Peter Quell Head of Portfolio Analytics for Market andCredit Risk
DZ BANK AG
“AI and machine learning have permeated a lot of areas where banks draw inferences from data. Nevertheless, the big role played by noise in financial markets data seems to be problematic. On the one hand, there will be hopelessly overfitted machine learning models with deteriorating prediction quality. On the upside, an explicit focus on the separation of signals from noise could lead to better time series analysis and financial market risk estimation. Even though this separation will never be perfect, tests on real portfolios and real market data show that with slight modifications, we could get large improvements compared with current standards.”
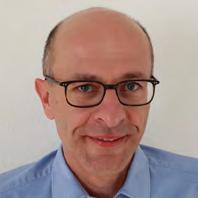
SVP
Director AnalyticsFifth Third Bank
“AI and machine learning (ML) will be transformational for the financial services industry. Given the regulatory requirements and risk management practices in commercial/retail banking, the biggest use cases are:
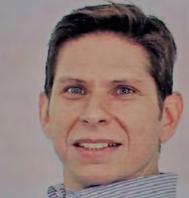
• Credit risk management: Assessing credit risk in lending by allowing financial institutions to make more informed decisions about how to manage risks and profitability.
• Personalization and marketing: Tailoring financial products, automated 24/7 customer service, and providing solutions for marketing. This can help financial institutions to attract and retain customers and increase brand loyalty.
• Fraud risk management: Identifying fraud patterns by efficiently utilizing large amounts of data. With effective fraud prevention, fraud losses can be reduced and customers can be protected with less friction.”
Liming Brotcke Senior Director, Head of Model Validation Ally“The financial sector has long been using machine learning algorithms to assist key decision making, with the best known use cases being decision trees and logistic regressions. Classifying and identifying content and pattern based on pre-existing data are discriminative AI uses. In contrast, generative AI is primarily designed for content generation, leveraging large language models (LLMs) and other subcategories of AI for language generation, image creation, music composition, video synthesis etc. Aside from continued use in fraud detection, banks can leverage generative AI to significantly enhance customer service including improved voice and text customer support, advanced sentiment analysis, and personalized financial advice.”




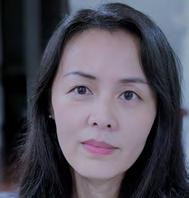

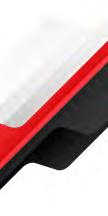
EVENTS CALENDAR 2023
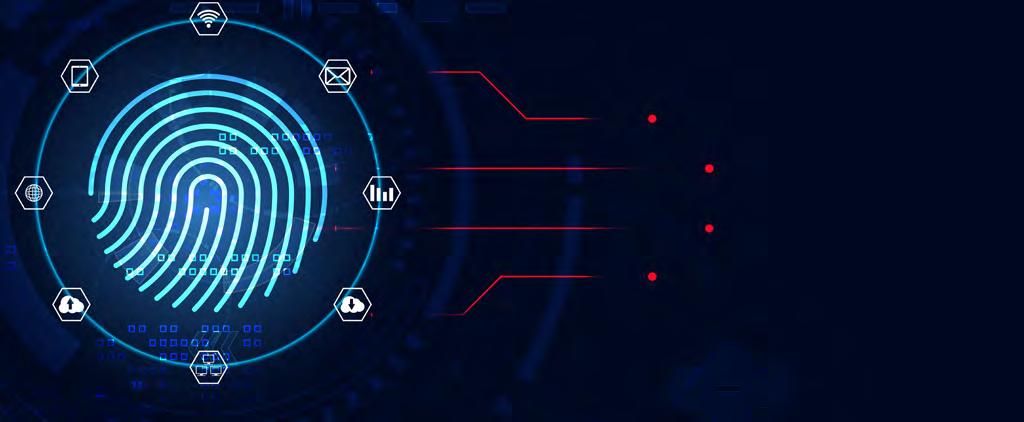
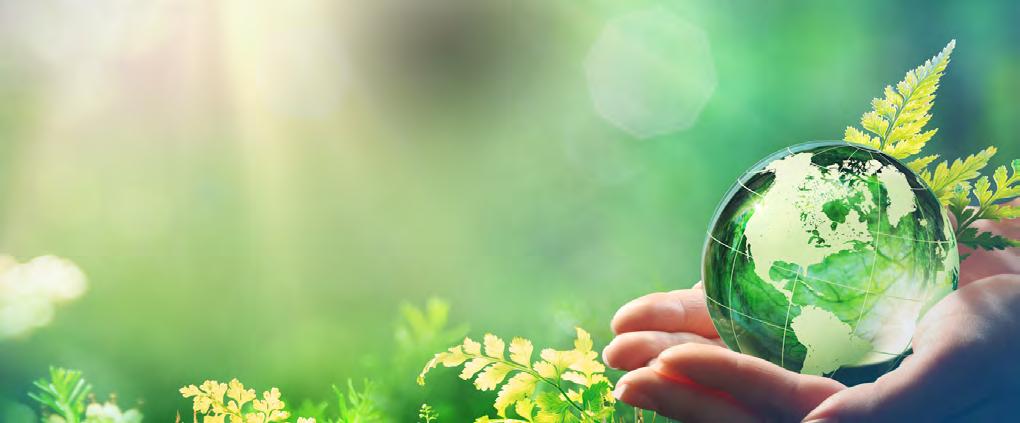
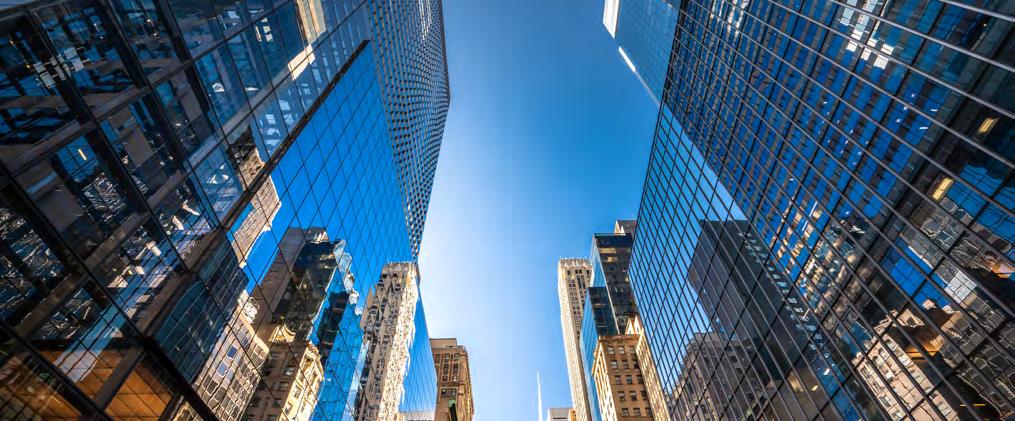
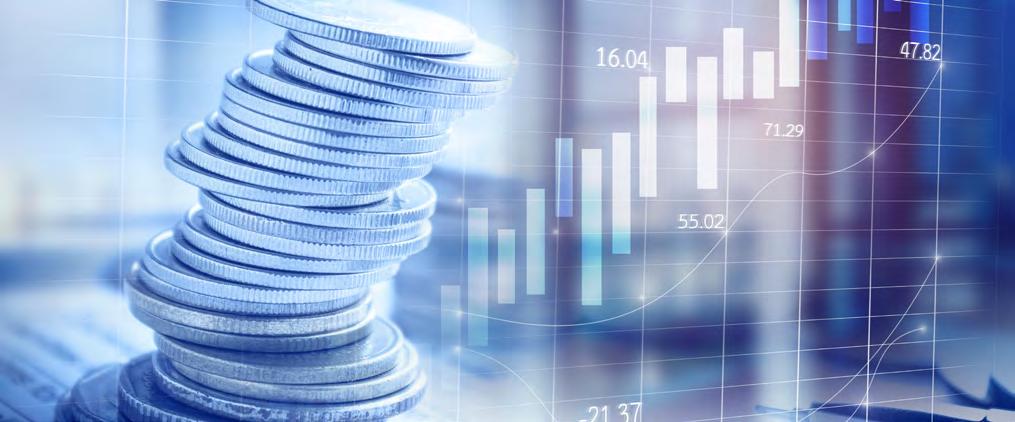
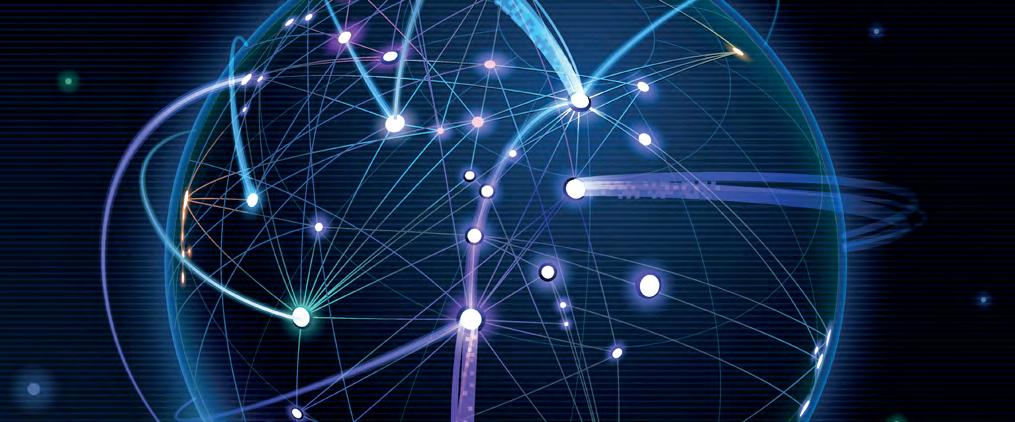
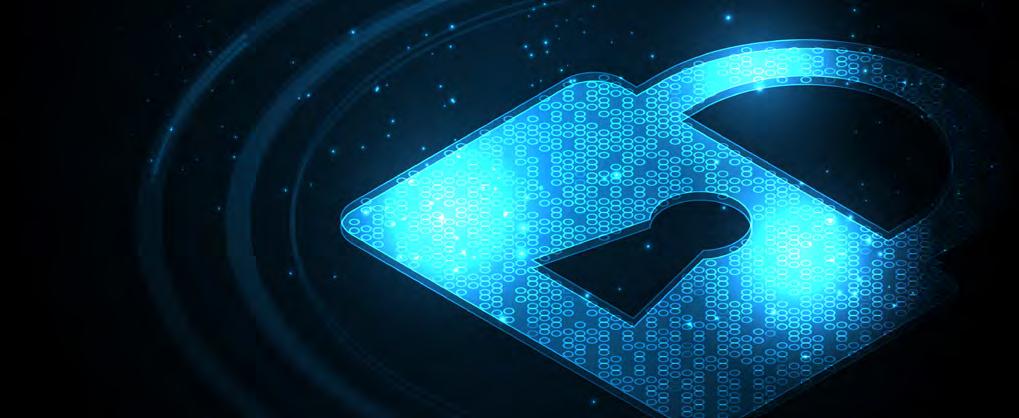
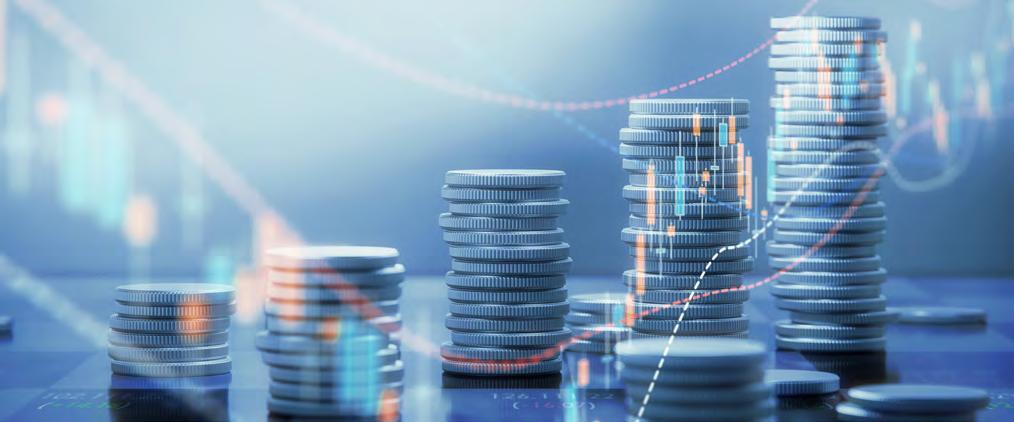
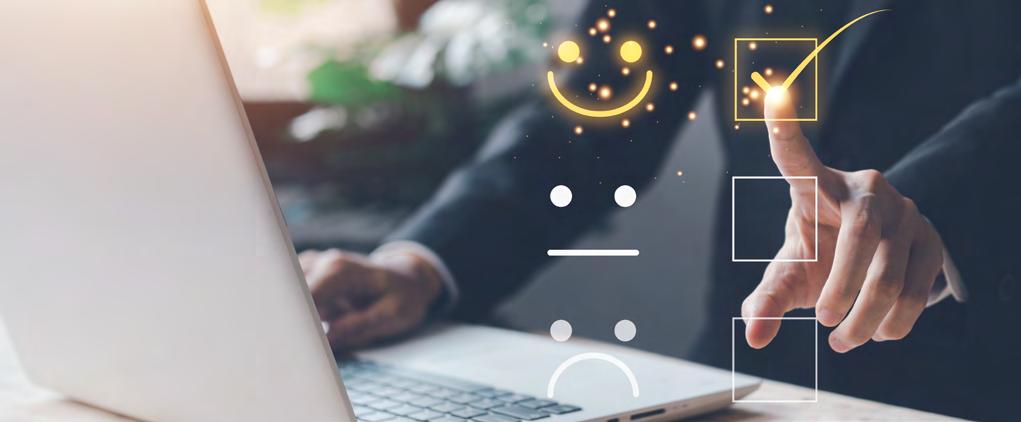