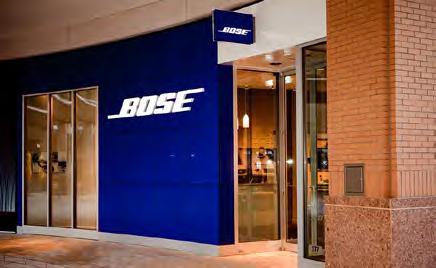
13 minute read
AI – Reshaping the Supply Chains of Tomorrow
“SCM’s interest in making data-driven decisions was consistently high, and it always will be. We are seeing the rise of AI in every supply chain segment. I feel that the challenge is not necessarily with not using AI, but it’s with what we do after finding the insights. We need a unified strategy and a cohesive approach to provide state-of-the-art experiences. The first step to achieve this is by ensuring that SCM has a seat at the table in all enterprise-wide strategic decisions,” stresses Mr. Aswini Thota, Analytics and AI leader, Bose Corporation, during an interaction.
How has the pandemic changed the landscape of such solutions for companies who are keeping a blind eye to them for many years? What are the lessons learned? Most successful companies were always innovative. They constantly try to gauge what their customers want and develop solutions that strike the right chord with them. But obviously, organizations can’t come up with groundbreaking innovations every year. The other way organizations try to attract customers is through service innovations. Offering world-class customer service, a state-ofthe-art delivery process, intuitive user experience, having the right product at the right time, and seamless returns are some ways organizations are trying to innovate.
Advertisement
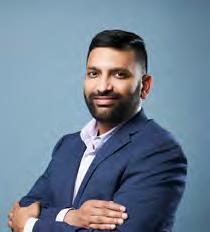
Mr. Aswini Thota is an Analytics and AI leader who solves organizational and business problems leveraging data. He always believed in the power of data and amazed what insights we can grasp from it. Over the course of his career, Aswini has developed a skill set in analyzing data and he hopes to use his experience and expertise in data science to help people discover the amazing career opportunities that lie ahead in the field of Data Science. He has effectively evolved from a machine learning researcher to an award-winning AI / Data science leader. Aswini holds two Master’s degrees in Electrical Engineering and Data Science.
For a company to innovate using service, all the components of their offering should work like a well-oiled machine, and the supply chain function is at the heart of this innovation.
Organizations realized a long while ago that innovation is key to success, and Covid-19 has created an environment where industry leaders must think on their feet and make fast decisions. The sudden shift in priorities caused by Covid-19 has exposed organizations that didn't have a foundational understanding of using modern technologies.
Perhaps a critical vulnerability that organizations realized after covid-19 was their lack of ability to make data-driven decisions. Enterprises that had a wellestablished data analytics practice were able to run what-if scenarios quickly and pivot to meet the customer needs. Organizations that realized the value of timely information made data-driven decisions their top priority to correct these shortcomings.
Why does today's business landscape underscore the need for faster and better decision-making? And how can AI help? We live in an ultra-competitive environment where a single tweet or a bad customer experience can have a devastating impact on an organization's success. Businesses must be able to anticipate the impact of their decisions and investments. Artificial Intelligence (AI), a subfield of computer science, provides practitioners with algorithms to generate predictive insights based on historical data. AI is identified as one of the transformative technologies currently available. When we think about the applications of AI in the industry, we generally come across product offerings such as self-driving cars, chatbots, etc. But there are numerous use cases of AI in solving enterprise business problems.
What role does AI play in improving planning and management across the end-to-end supply chain management? At its core, AI uses mathematical models to generate predictions and insights. These models are based on historical data. The AI algorithms offer a flexible and general-purpose framework to conduct experiments within any domain. Many SCM problems will fit into an AIbased problem-solving framework. For instance, we can: • Generate time series forecasts to predict customer demand for any given period accurately. • Predict the reason for a customer service call based on historical purchases and returns • Optimize the delivery routes based on the traffic, weather, and local events • Identify faulty machines and products
The use cases of AI to improve SCM practices are long. We can safely say that any outcome that depends on certain known variables can be a good fit for AI, and leaders should conduct a cost-benefit analysis to prioritize the use cases.
Implementing AI-driven approach in demand forecasting can work magic for companies. Can you elaborate on this with an example? Demand forecasting is probably the top use case of AI in supply chain function. Demand forecasting deals with estimating the customer demand over a specific period. Demand forecasting, on the surface, seems like distributing the correct number of units to the right store at the right time. But it has so much more to it. Having an accurate demand forecasting function helps organizations procure parts and manufacture units at the right time.
There are several proven statistical techniques to generate demand forecasts; some of them are Simple Moving Average (SMA), Exponential Smoothing (SES), Autoregressive Integration Moving Average (ARIMA), etc. In recent years, organizations have been using neural network-based approaches such as Long short-term memory (LSTM), Gated recurrent units (GRUs), and Autoencoders. When done right, demand forecasting can help supply chain leaders accurately forecast the number of units to manufacture in a specific time along with the number of units to distribute to each store.
How AI technology is likely to make real improvements in supply chain management? AI is used to augment and optimize much of the existing supply chain management (SCM) practices. From pre-season demand planning, merchandising decisions, planning and allocation, inventory availability, product assortment, inventory fulfillment, and route optimization to last-mile events, AI is reinventing SCM.
We are seeing AI being used to – extract text from supplier's documents, generate demand forecasts, optimize logistics, design the route plan, etc. The real value of AI is discovering the latent trends in the data and prescribing solutions to the decision makers to act. The approach must be comprehensive for AI to make a tangible impact on SCM. It is simply not sufficient for organizations to focus on a use case. From data gathering,
Organizations should stop thinking of AI as a one-off solution. Instead, leaders should drive using analytics and AI. Indeed, the path to implementing AI-centric SCM function is not without challenges, but none of the hardships are unique to AI. For instance, lack of quality data is one of the top impediments that often prevent firms from implementing AI. You can either fix the core data problems that plague your organization and implement advanced analytics or stay behind the AI-centered innovation.
Predicting the future using historical data is also not new to the SCM function. In fact, demand forecasting is perhaps one of the oldest machine learning use cases that used statistics and time series forecasting methods to generate temporal estimates. But the modern-day’s AI algorithms, coupled with cheap and efficient cloud processing power, have created a ripe environment for SCM function to democratize AI across all supply chain pain points.
data quality, data integration, and data storage to running experiments, all pieces of the AI puzzle should be dealt with at most importance.
How can AI technology bring transparency and visibility to supply chains by improving the security and traceability of products? Transparency and accountability have become essential for a good SCM function. Having transparency in SCM means leaders can precisely know the status of each step in the supply chain pipeline. A transparent supply chain function also assumes that the data retrieved is quality tested and is of the gold standard. A transparent and well-governed SCM function can help business leaders understand the pain points at any given period and help them provide timely solutions.
Overseeing the entire SCM function and identifying the problems based on heuristics and observations is a gigantic task. Here is precisely where AI can help. AI algorithms are very good at analyzing historical data to identify abnormal trends. Anomaly or outlier detection uses AI algorithms to identify rare and unexpected events that are different from standard patterns. Anomalies in SCM function are common. For instance, large volumes of orders coming from the same IP, distribution times that don't follow the normal curve, item prices being significantly below the previous sales price, etc., are some situations that SCM handles regularly.
How prevalent is the incorporation of AI & advanced analytics in the supply chain? Where are the user companies lagging in deploying them to the fullest? What are the challenges encountered?
The use of data and analytics to advance supply chain functions is not new. The use of analytics to monitor the supply chain started a century ago, in the late 1920s when organizations such as Ford introduced mass production along assembly lines. Enterprise Resource Planning (ERP) systems and digitization of tasks revolutionized enterprise functions such as finance, supply chain, etc. This change has led organizations to store information about all the enterprise touchpoints in transactional databases. Data warehouses were then created to generate KPIs, and business intelligence tools helped organizations view the function's performance holistically.
Predicting the future using historical data is also not new to the SCM function. In fact, demand forecasting is perhaps one of the oldest machine learning use cases that used statistics and time series forecasting methods to generate temporal estimates. But the modernday's AI algorithms, coupled with cheap and efficient cloud processing power, have created a ripe environment for SCM function to democratize AI across all supply chain pain points.
Organizations should stop thinking of AI as a one-off solution. Instead, leaders should drive using analytics and AI. Indeed, the path to implementing AI-centric SCM function is not without challenges, but none of the hardships are unique to AI. For instance, lack of quality data is one of the top impediments that often prevent firms from implementing AI. You can either fix the core data problems that plague your organization and implement advanced analytics or stay behind the AI-centered innovation.
Give us top 5 reasons if you need to convince a chief supply chain officer of any firm to deploy analytics and other advanced technology tools? State 5 major transformative landscapes to be precise. Though using advanced analytics in the supply chain has numerous benefits, below are my top five reasons to invest in data science and AI:
❖ Improve customer experience:
Providing a legendary customer experience is all about anticipating your customer's needs and exceeding their expectations. The Supply chain function must lead and manage a lot of these expectations, from procuring raw materials to putting the products on the shelves. AI can help with predicting and forecasting customer needs.
❖ Increased automation:
Organizations hire the best minds to perform meaningful tasks.
Sometimes, compliance and ethical standards demand office workers do routine and mundane tasks. AI can help automate a lot of these tasks and help your workforce focus on more value-added tasks.
❖ Preventing machines from failing: Consistency and fault tolerance are important for running an efficient production pipeline; they are also critical for ensuring workers' safety. AI can enhance anomaly detection by learning the latent trends and generating early warning signs than facilitate timely maintenance efforts.
❖ Pace over perfection: Covid-19 has proven that organizations need to think on their feet and make instant decisions. Organizations must take a proactive approach and be ready for the next disruption. Having critical
and foundational elements such as data lakes and cloud infrastructure will ensure organizations make quick data-driven decisions and be a key differentiator.
❖ Reduce attrition: One of the significant challenges most supply chain functions face is employee attrition. This is more prevalent in warehouses and distribution centers.
People analytics is a field that uses statistics and machine learning to understand the needs of employees.
The insights generated from the people analytics function can help organizations take preventative measures to ensure employee satisfaction.
Share with us steps to building a strong supply chain analytics strategy. Building a robust and comprehensive analytics strategy starts with realizing data as your number one asset. Business leaders should prioritize data over intuitions. Below are some of the building blocks that you will see in a successful SCM analytics function:
• Data storage: SCM function deals with several internal and external entities. Organizations need to digitize all the services and store the information with a mindset to retrieve them later. Big data and cloud technologies allow organizations to efficiently store and retrieve massive volumes of data.
❖ Data quality: Garbage in, garbage out. This is particularly true for the AI model. The quality of your AI models, to a large extent, depends on the quality of the data you feed into the models.
Erroneous, incomplete, partial, and non-representative data are typical data challenges organizations face. Having gaps in your data will mainly be troublesome for demand forecasting. Organizations try to fix this issue by often buying data from external vendors. Companies need to improve the data quality before rushing to modeling. ❖ Data insights: Data insights is the process of generating actionable insights from data and creating a tool or solution for your business partners to consume these insights. Business intelligence and data visualization tools offer an excellent capability for organizations to consume descriptive insights. Technologies such as AI will help organizations consume predictive and prescriptive insights from the data.
Can you share with us a use case where you have implemented advanced analytics in supply chain at Bose and garnered tangible gains? Cold-start or new product introduction forecasting is the process of understanding the sales demand for net new products. Traditionally, organizations used to perform this task based on heuristics or trivial approximation mechanisms. We developed a robust Deep Learningbased approach to understanding the new product's sell-through demand before launching the product. This helped organizations to take a proactive approach toward manufacturing and distribution. We can significantly reduce the error by combining our historic sellthrough demand data with additional data sets such as media spend, product features, etc.
Having significant experience in this dynamic field, can you share with us some of the supply chain segments that are still white spaces when it comes to adoption of new age technology tools such as AI & analytics? SCM's interest in making data-driven decisions was consistently high, and it always will be. We are seeing the rise of AI in every supply chain segments. I feel that the challenge is not necessarily with not using AI, but it's with what we do after finding the insights. Today's digital customers want organizations to provide exceptional customer experience. Part of providing an extraordinary customer experience is understanding what your customer wants and proactively providing avenues and opportunities for them to make purchases. All enterprise functions try to deliver a game-changing customer experience.
Sales and marketing try to predict the next best product, the market research team tries out cool products to impress customers, the supply chain function generates forecasts to ensure timely deliveries, etc. But to win and retain a customer, it takes more than this. We need a unified strategy and a cohesive approach to provide state-ofthe-art experiences. The first step to achieve this is by ensuring that SCM has a seat at the table in all enterprise-wide strategic decisions.
What are the upcoming disruptive technologies that we are yet to witness in supply chain? Internet of Things, Edge Computing, Artificial Intelligence, and Blockchain technologies are all set to disrupt the supply chains. We already see drone deliveries piloted in certain countries. Avoiding traffic and autonomously delivering goods can be expected to become a common phenomenon in the next few years. We might see organizations using autonomous vehicles and drone technology to first distribute goods between airports and warehouses, followed by customer deliveries.
We commonly think about Bitcoin and cryptocurrency when we hear about blockchain. At its core, a blockchain is a decentralized ledger that anonymously records transactions between involved parties. Blockchain avoids the use of intermediaries for verification and enhances security. The applications of blockchain technology in the supply chain are huge. SCM function can use blockchain technology to transact with entities with improved security, compliance, and scalability.