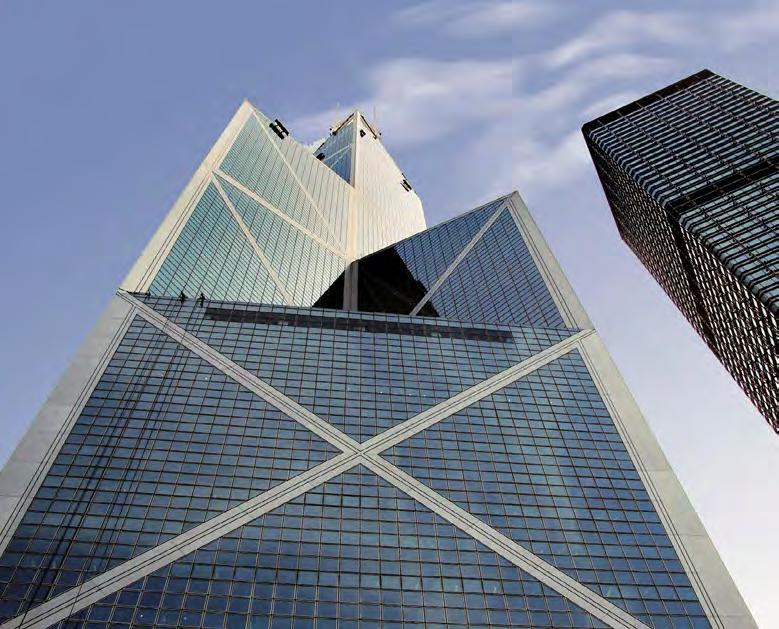
5 minute read
Artificial intelligence and advanced analytics are key to open banking
RETAIL BANKING: THE BANK OF THE FUTURE Artificial intelligence and advanced analytics are key to open banking
These accelerated innovations are essential for banks to move towards global transformation.
To foster continuous improvement beyond the first deployment, banks must augment homegrown AI models and talents
Open banking in markets such as Australia, Hong Kong, the Philippines, and Malaysia still has a kind of anxiety around it. However, with markets, such as Singapore, whose regulators show strong support as well as supportive players, it may as well be a matter of time, Irene Xu, director of banking practice for SAS Asia Pacific Global Industry Practice, observed.
“In this region (Asia Pacific), we can expect open banking as the next biggest disruptive force in the financial services industry not only due to the fast rise of the extensive digital economies but also that we have observed very strong support from many local regulators in this region, with the intention to bring more competitive and better financial products and services to the community,” Xu said.
Before the move to open
Open banking is the next biggest disruptive force in the financial services industry
banking, Xu stressed the importance of leveraging data and the use not only of artificial intelligence (AI) and machine learning (ML) but also advanced analytics (AA).
McKinsey partners, Renny Thomas and Violet Chung, believe that lenders will need to move towards an enterprise-wide road map for deploying AA and ML models that would also include plans to embed AI in business processes.
They stressed that to establish a robust AI-powered decision layer, banks will need to shift from attempting to develop specific use cases and point solutions to an enterprise-wide road map for deploying AA and ML models across entire business domains.
“In addition to strong collaboration between business teams and analytics talent, this requires robust tools for model development, efficient processes (e.g., for re-using code across projects), and diffusion of knowledge (e.g., repositories) across teams. Beyond the at-scale development of decision models across domains, the road map should also include plans to embed AI in business-as-usual processes. Often underestimated, this effort requires rewiring the business processes in which these AA/AI models will be embedded; making AI decisions ‘explainable’ to endusers, and a change-management plan that addresses employee mindset shifts and skills gaps,” they added.
Thomas and Chung said that to foster continuous improvement beyond the first deployment, banks also need to establish infrastructure (e.g., data measurement) and processes (e.g., periodic reviews of performance, risk management of AI models) for feedback loops to flourish. Additionally, banks will need to augment homegrown AI models and talents.
Four layers of AI banking
To become AI-first, banks must invest in transforming capabilities across all four layers of the integrated capability stack: the engagement layer, the AIpowered decisioning layer, the core technology and data layer, and the operating model, Thomas and Chung explained.
The first layer will entail reimagining customer engagement as customers expect banks to be always present in their end-user journeys as well as know their context and needs no matter where they interact with the bank, and to enable a frictionless experience.
Second, building the AI-powered decision-making layer will enable delivering personalised messages and decisions to millions of users
Platforms Crucial for A Bank’s Success
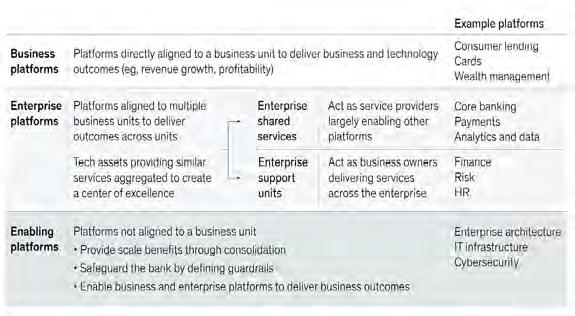
Irene Xu
Violet Chung
Source: McKinsey & Co.
and thousands of employees, in near real-time across all platforms.
The third and fourth layers will strengthen the core technology and data infrastructure and transition to the platform operating model.
“Whilst most banks are transitioning their technology platforms and assets to become more modular and flexible, working teams within the bank continue to operate in functional silos under suboptimal collaboration models, which often lack alignment in terms of goals and priorities,” Thomas and Chung explained.
Innovation for SMEs
Xu said that AI, ML, and deep learning help harness data for business decisions, address gaps, and have a deeper understanding of their customers. A particularly interesting area for her is in the small and medium enterprise (SME) businesses.
“Traditionally, I will say that small and medium enterprises see a lot more difficulties in terms of accessing finance, getting additional capital, and funding because they are often associated with higher risks, insufficient information, or lack of collateral. Based on what we have seen in the past, it can be as high as 50% of SMEs getting rejected when applying for loans. It’s a very unpleasant experience and the whole process can be lengthy and laborious,” Xu said.
Banks in the early stages of adopting AI and automation have undergone a significant change and have now optimised the customer experience. Xu shared an example of the Mizuho Bank in Japan which launched a new service called Mizuho Smart Business Loans in 2019.
“Under this service, the bank has internal data, as well as external information to understand customer transactions, particularly on deposit information. From there, it helps them understand the company’s ability to repay the debt,” Xu explained.
Additionally, the bank also adopted application programming interfaces or API and automation processes so that SMEs are no longer required to submit paper documents or financial statements, leading to a significantly reduced turnaround time of two to three working days.

Banks’ global transformation
Xu pointed out that API will certainly play a very critical element in the development of open banking.
“From that perspective, regulatory requirements are very much driven by the local regulators and vary from market to market. One of the key things that we are seeing is probably, very soon we will see much more standardised API regulations at the global level which will help players have much better access at a significantly lower cost,” Xu said.
Banks should leverage the adoption of data analytics, cloud computing as well as other real-time capabilities to provide services in real-time.
“Lastly, in the areas of security, privacy, and fraud detection, the main concerns are how data can be governed, how data privacy is secured whilst information is being shared amongst banks, and how third-party providers can guarantee protection just in case attackers try to take advantage. It’s certainly important for banks to look at the right infrastructure and capabilities to ensure they can identify thirdparty API model vulnerabilities, as well as using AI and machine learning to prevent and detect fraud quicker,” Xu concluded.