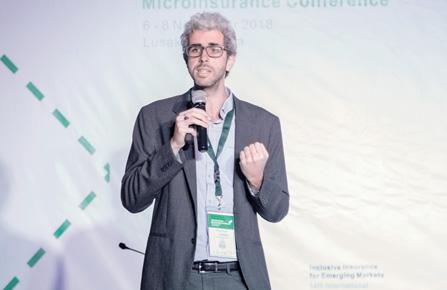
6 minute read
Parallel session 9 Alternative client data for inclusive insurance
Hosted by insight2impact
By Laura Montenbruck In this session, hosted by insight2impact, practitioners provided examples of the application of alternative data in insurance provision as well as opportunities and challenges. Alternative data can range from social media profiles, online platforms, call records and shopping data, to data and images from drones and satellites as well as smartphones. Used in the right way, such information can help improve the understanding of clients and the design of more efficient insurance products. Several such examples were introduced in this session. Using credit bureau data to drive inclusive insurance
Advertisement
At Hollard, credit bureau and shopping data is used to better target customers. Potential customers in the South African market have a need for the products Hollard offers but often can’t afford them and culturally there is also a phenomenon of difficulty in saying no to an offer provided. This results in more than desired product volumes which are not taken up. To address this, a predictive propensity-to-pay model using bureau data was developed. When customers call the hotline, their phone numbers are matched to credit bureau data providing information on their ability to pay, and subsequent customer USSD engagements and dial strategies are used to optimise conversion. Through the propensity-to-pay model, Hollard is able to better target customers and not overburden those who cannot afford them, while optimising operational efficiencies. Decreasing operational cost enables product pricing optimisation which results in updated products that are able to reach more customers. In another project, shopping data is being used to better understand the customer and the correlation between retail and insurance behaviour.
Smartphone use in crop insurance Because of basis risk, index-based insurance may in some cases not compensate a farmer’s actual damage. Sending an expert to the field for damage assessment is too costly. Is there a more cost-effective way?
64 — Francisco Ceballos, Senior Research Analyst, International Food Policy Research Institute (IFPRI), United States
65 — Belhassen Tonat, General Manager / Non-Life, Munich Reinsurance Company of Africa, South Africa
66 — Mia Thom, Technical Director, Cenfri, South Africa
67 — Megan Lawrence, Managing Director of Customer, Strategy and Data Analytics, Hollard, South Africa
68 — Herman Smit, Technical Director, Cenfri & i2i, South Africa, facilitator of the session 64
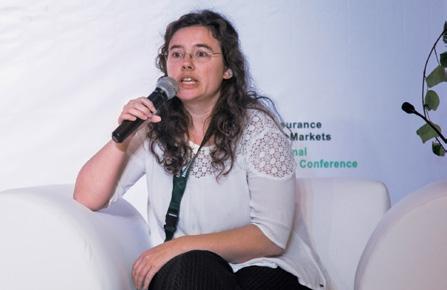
66 65
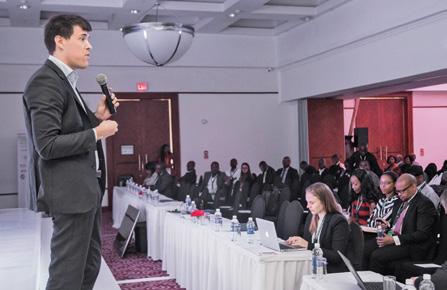
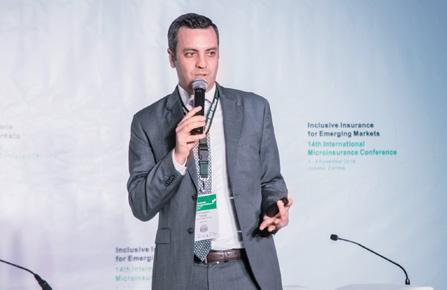
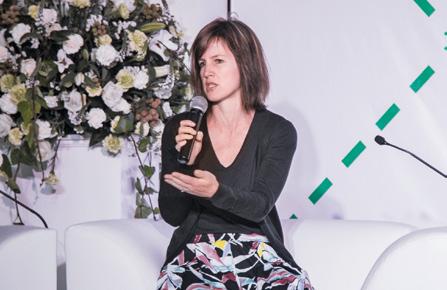
67
In a project in India, researchers from the International Food Policy Research Institute (IFPRI) implemented picturebased insurance (PBI) for farmers. Farmers download an application, take an initial picture of their field and repeat this process weekly from planting to harvest. A “ghost picture” in the background thereby helps them to take the picture from the same position and angle every time. GPS-tracking serves as confirmation. Experts evaluate the pictures and can thus adequately judge whether a loss has occurred and how large the loss is.
These pictures not only lower the cost of the claims process. They also speed up claims payment. Moreover, relating these pictures to secondary data allows giving farmers additional, personalised advice that can be sent directly to their phones (see Figure 31). As index insurance is still cheaper in various contexts, picture-based insurance could complement indexbased insurance. Remote-sensing devices for improved claims service Munich Re Africa is working with remote-sensing devices to improve insurance products. In particular, drones are used to increase efficiency in claims assessment. When a claim is made, a drone flies out and takes pictures of the damage, which are then evaluated by an algorithm. This not only reduces management costs, as sending an expert to the site becomes redundant, but allows for immediate payment after loss assessment. In cases where the site is not directly accessible, for example after a fire, it also enables the insurer to assess the situation earlier and pay the loss sum right when it’s needed. This is a huge success, especially in a low-income context, where immediate payouts can make an enormous difference for the lives of the poor. A second project uses remote sensing in pipes to prevent or raise alerts about losses before or just as they are happening. These sensors measure water temperature and are able to tell whether a fire is about to break out in the next few hours or not. This might sound like an expensive prevention mechanism but, according to Munich Re, amortisation over time makes it affordable even in a low or middleincome context.
Figure 31 Added value of picture-based insurance
Smartphone pictures from farmers Crop profiles Soil data Weather data Satellite images
Personalised advice
Alternative data and the insurance value chain
A study carried out by Cenfri investigated the current applications of alternative data in insurance. Based on client interviews and an analysis of the value chain, it revealed that such data is mostly used in the areas of sales and product development. However, applications also emerge in other parts of the value chain: tailoring the premium collection, reducing risk behaviour, and decreasing costs in claims processing, etc. Insurers can thereby fall back on internal as well external data, such as credit history and social media profiles (see Figure 32). Combining the available information increases the understanding of customers and helps ameliorate their insurance experience. Currently, many insurers are focusing on improving and understanding their own data before engaging in secondary data collection. Important aspects here are the standardisation of customer data capturing and storage, as well as linking data systems to create singlecustomer views.
Alternative data can benefit both insurers and clients. Client-specific information allows more personalised risk pricing. As credit, savings and insurance are often used interchangeably, data can help insurers provide the most appropriate product. And it can decrease their administrative costs. Customers benefit from these improvements as well. The use of insurance experience and data as proxy collateral for credit scoring can decrease the price of insurance. And efficiency gains in processing claims can expedite payment. Two cautions however: data quality is heterogeneous, which can make the matching process complicated and inaccurate; use of alternative data can invade the privacy of customers and they should be informed of how their data is used. Lessons learnt
• Alternative data can help identify the needs and wants of the excluded, in Africa and elsewhere. • The value of alternative data in designing products depends on, amongst other factors, the cost of getting the data. • Alternative data, used in the right context, can help reduce (management) costs of insurers, thereby making insurance more affordable and accessible to the poorer population. • Alternative data must be used without violating the customers’ right to privacy.
Figure 32 Data use in the insurance value chain
Insurance value chain
Product development
Sales
Premium collection
Servicing and risk management
Claims processing Internal External
Information provided on sign-up Transactional (financial)Biometric identifiersPsychometric dataComplaints and call centre Customer interviews Survey dataGovernment (open) data Credit bureau dataSatellite/aerial imageryGeographic information systems (GIS) data Call detail records (CDR) and airtime Credit historyMobile money dataSocial media dataSmartphone dataSensor (IoT) dataImage (meta)dataData types
Fewer More