
3 minute read
DETECTING PNEUMONIA IN CHEST X-RAYS USING AI INFERENCING AT THE EDGE
Subh Bhattacharya Lead, Healthcare, Medical Devices & Sciences at Xilinx
Figure 1
Advertisement
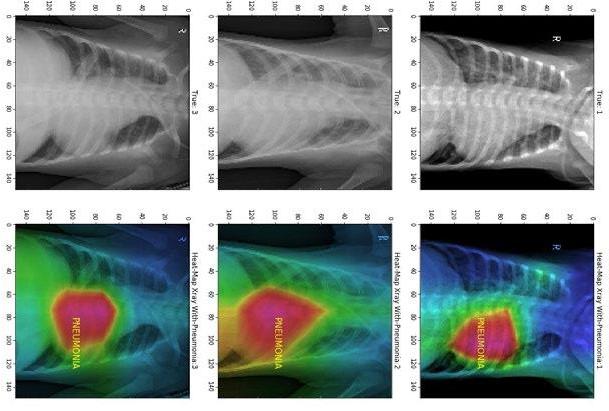
The use of artificial intelligence (AI), specifically machine learning (ML), is fast becoming a transformational force in healthcare. Using AI and various image processing techniques within radiological modalities like X-rays, ultrasound and CT scans can lead to better diagnosis and better patient outcomes. Additionally, use of AI can lead to increased operational efficiencies, and significant cost reduction in healthcare procedures.
Chest X-rays used to detect respiratory diseases, like pneumonia, are the most used radiological procedure with over two billion scans performed worldwide every year, that’s 548,000 scans everyday. Such a huge quantity of scans imposes a heavy load on radiologists and taxes the efficiency of the workflow. Some studies show ML, Deep Neural Network (DNN) and Convolutional Neural Networks (CNN) methods can outperform radiologists in speed and accuracy, particularly under stressful conditions during a fast decision-making process where the human error rate could be quite high. Aiding the decision-making process with ML methods can improve the quality of the results, providing the radiologists and specialists an additional tool.
Healthcare companies are also now looking for effective point-of-care solutions to provide cost-effective and faster diagnosis and treatment in the field or in locations away from large hospitals. As a result, there’s rising demand to perform accurate image inferences to efficiently detect respiratory diseases like pneumonia in the field and provide clinical care using small, portable and point-ofcare devices at the edge. Spline.ai (a partner of Xilinx) has developed a model using curated, labeled images for X-ray classification and disease detection (Figure 1). The model - trained using datasets from the National Institute of Health (NIH), Kaggle and from the likes of Stanford and MIT - can detect pneumonia with greater than 94% accuracy today. The model is then deployed and optimized on Xilinx’s
Zynq® UltraScale+™ MPSoC running on the ZCU104 platform acting as an edge device. Xilinx’s Deep Learning Processing Unit (DPU), a soft-IP tensor accelerator that enables low inference latency of less than 10 microseconds. The model will be retrained periodically to improve the accuracy of its results.
Xilinx technology offers a heterogeneous and a highly distributed architecture, delivering more compute for image processing, enabling healthcare companies to offer effective and faster diagnosis. The highly integrated Xilinx Zynq UltraScale+ MPSoC with its adaptable Field Programmable Gate Arrays (FPGA), integrated accelerators for deep learning (DPU), integrated digital signal processor (DSP) and its ARM® multi-processor systems perform accurate image classification and detection with AI inferencing in close to real-time with low latency and low power consumption.
PYNQ™, the open-source Python programming platform for Zynq architecture with Xilinx’s latest AI Toolkit Vitis AI™ version 1.1, is used to compile the deep learning models for running accelerated inference making this solution cost-effective. Xilinx unified software platforms, Vitis for application development and Vitis AI for optimizing and deploying accelerated ML inference, mean that data scientists and algorithm developers can use advanced devices like the MPSoC easily in their projects.
New healthcare workflows need to deliver more compute for image processing, data privacy, security, patient safe ty and accuracy in much smaller edge devices. Heterogeneous and adaptable distributed systems, which can be small and portable, are key for solving this problem. Xilinx devices like the Zynq UltraScale+ MPSoC and the Vitis software platform are ideal for delivering the optimized clinical device enabled for AI inferencing at the edge.
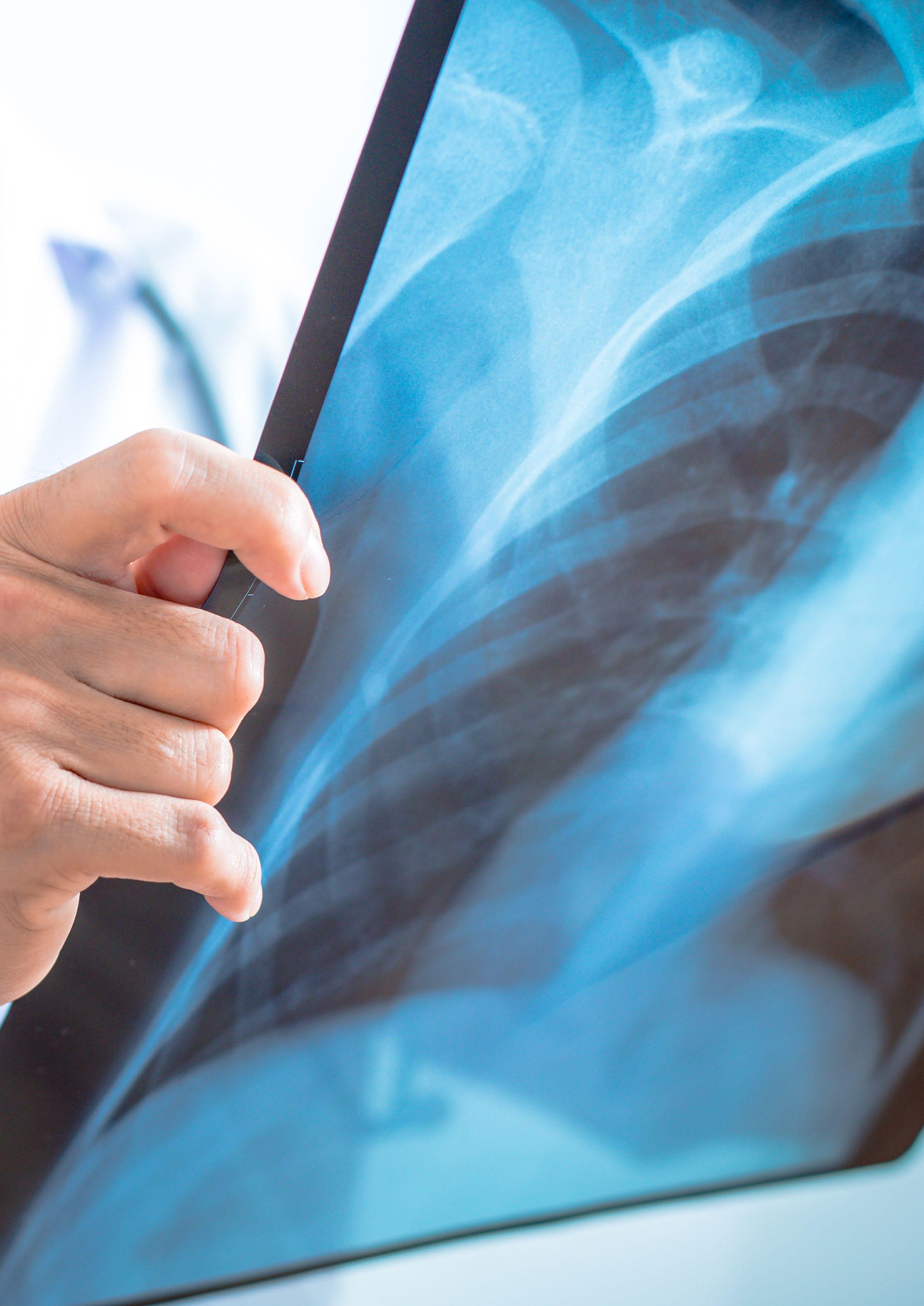
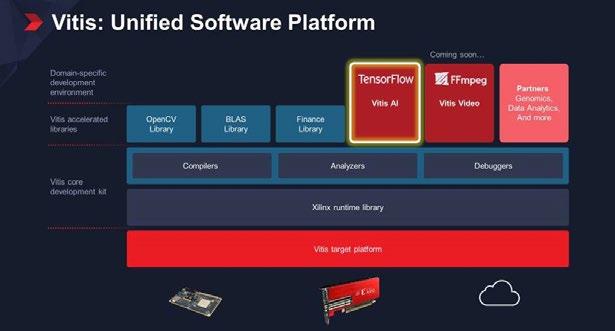
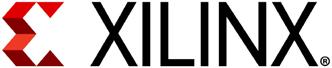
MV