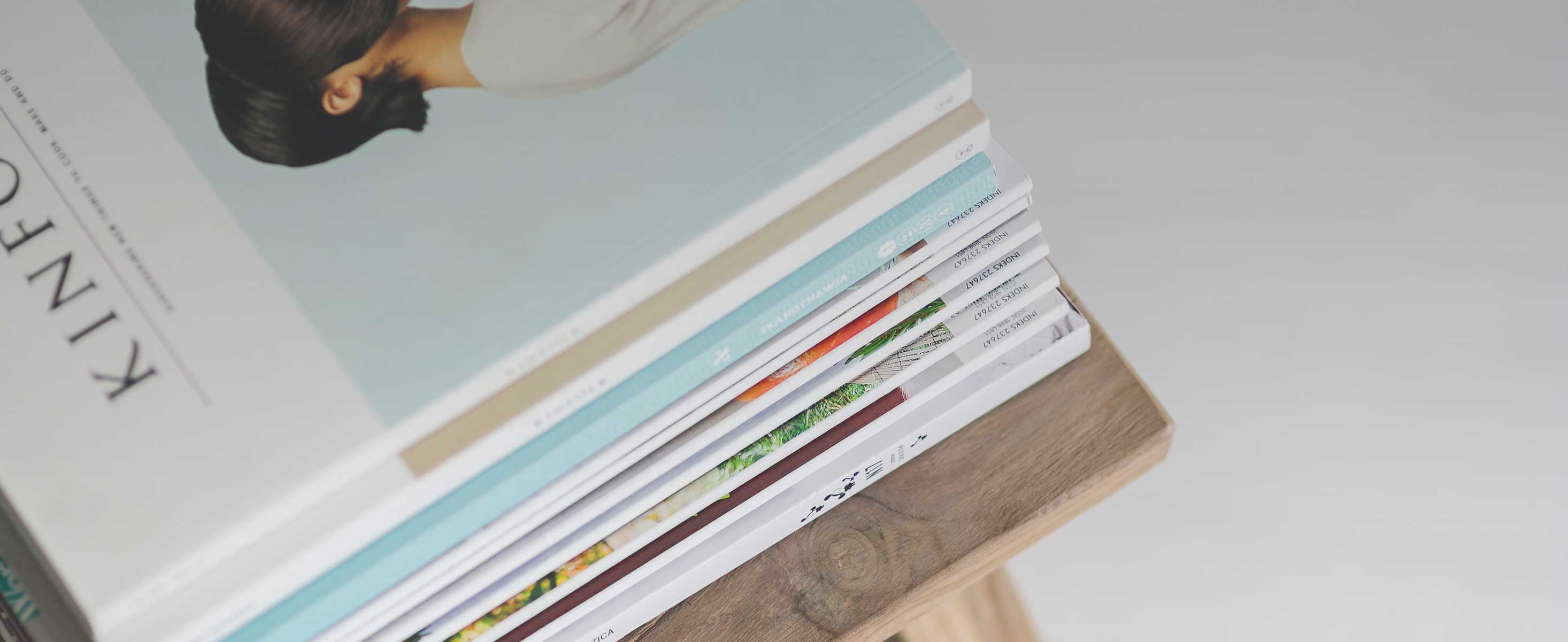
1 minute read
Processing and Visualization of EEG Data Using EEGLAB
Creid Johnson
Faculty Sponsor: Dr. Iordanka Panayotova, Department of Mathematics
Abstract
The purpose of this research project was to demonstrate the advanced signal processing of capabilities of EEGLAB and its applicability to electrical signal processing. EEGLAB is able to collect and process signal data, develop 2D and 3D simulations of electrical signals in the brain, and separate signals into independent components. By demonstrating how EEGLAB can be used in advanced signal processing, signal processing researchers are able to visualize and understand how EEGLAB would benefit them in processing signal data. EEGLAB provides researchers with several options in modeling, simulating, and presenting their signal data. EEGLAB has an Independent Component Analysis (ICA decomposition) algorithm feature for separating signals into independent components. Before EEGLAB was implemented, researchers were only able to use the averages of the ERP waveforms to calculate signal properties. Event-related dynamics aren’t always accounted for in these plots. Also, ongoing EEG processes that may be time or phase-locked are also ignored in averaging ERP waveforms. So, ICA decomposition must be applied to ensure that signal plots and data interpretation are correct. For the research project, an experiment was conducted in order to better understand how social categorization affects face processing. In this experiment, participants had electrodes placed on their scalps to monitor signal activity. Individuals were given faces to memorize while completing an age or sex categorization task. A fixation cross was presented in the middle of the screen between face stimuli. The signal and eye movement data were recorded and processed using EEGLAB. Signal data was collected from 64 scalp channels. An ICA algorithm was then used to separate the signals into independent components. After applying the ICA algorithm, the component ERP image and spectra were able to be plotted. The independent components were used to show concentrated regions of brain activity. Signal concentrations were used to show the greatest source of signal activity and main signal type in specific brain regions. This would be very beneficial in monitoring for epileptic seizures, mental disorders, and other brain irregularities.
Creid Johnson is a student, at Christopher Newport University, pursuing a B.S. in Electrical Engineering. He plans to earn his master’s degree, in Electrical Engineering, and pursue a career in developing advanced satellite communication systems. On campus, he is a part of CNU’s Unmanned Aerial Systems Team that is dedicated to develop durable aircraft systems and drones. Besides designing wireless communication systems for drones, he is also pursuing research in developing programs capable of sensing turbulent wind patterns around airborne drones. These turbulent patterns would then be used in developing an autopilot system capable of rotating the drones to continue flying through unexpected wind conditions. Through CNU’s Signals and Systems course, he was introduced to basic signal properties, characteristics and its importance in neuroscience, psychology, and electrical engineering.