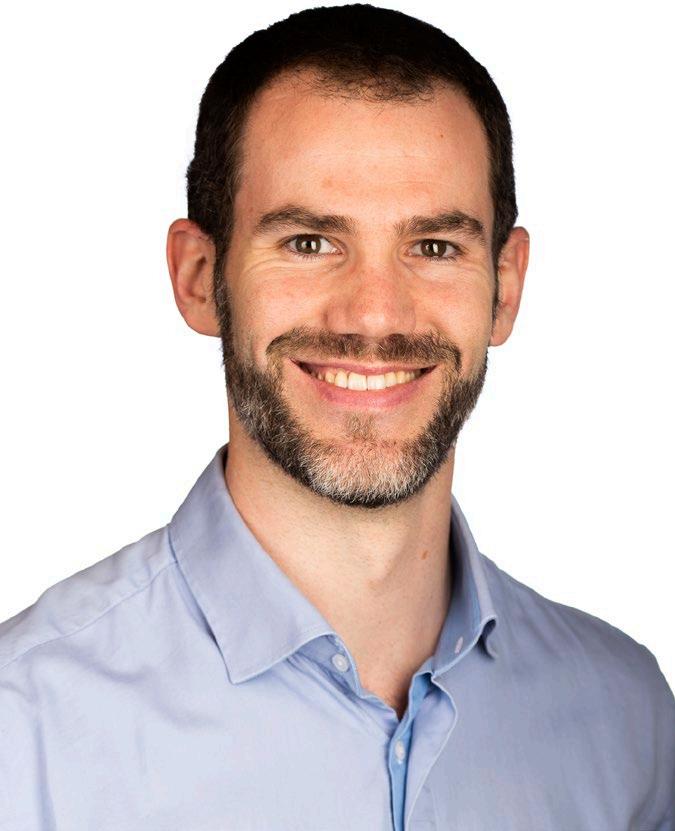
2 minute read
New score accelerates rollout of open banking
New score accelerates risk-based management
By Barrett Hasseldine*
Advertisement
Our team of data scientists at illion have unlocked new insights into consumers’ credit risk behaviour, based on key trends from income and expense transactions that have traditionally been hidden.
In a post-COVID-19 world, this is the game changer that the market has been looking for – a more sharply focused lens which gives decision makers a clearer picture than they have had before.
There are upsides for consumers as well as credit providers, with the key insights needed to unlock more tailored lending terms and conditions and a broader range of products through enhanced credit underwriting and more suitable loan pricing.
This is good for financial institutions who can price more efficiently, lend more responsibly and do so at lower cost, and it is good for consumers who will have an expanded choice and get the products that are right for them, quickly. It’s one of those rare innovations where everybody wins.
Our modelling also shows that for personal loans, lenders can increase their portfolio profits by 20%1 , considerably reduce risk, and have happier customers.
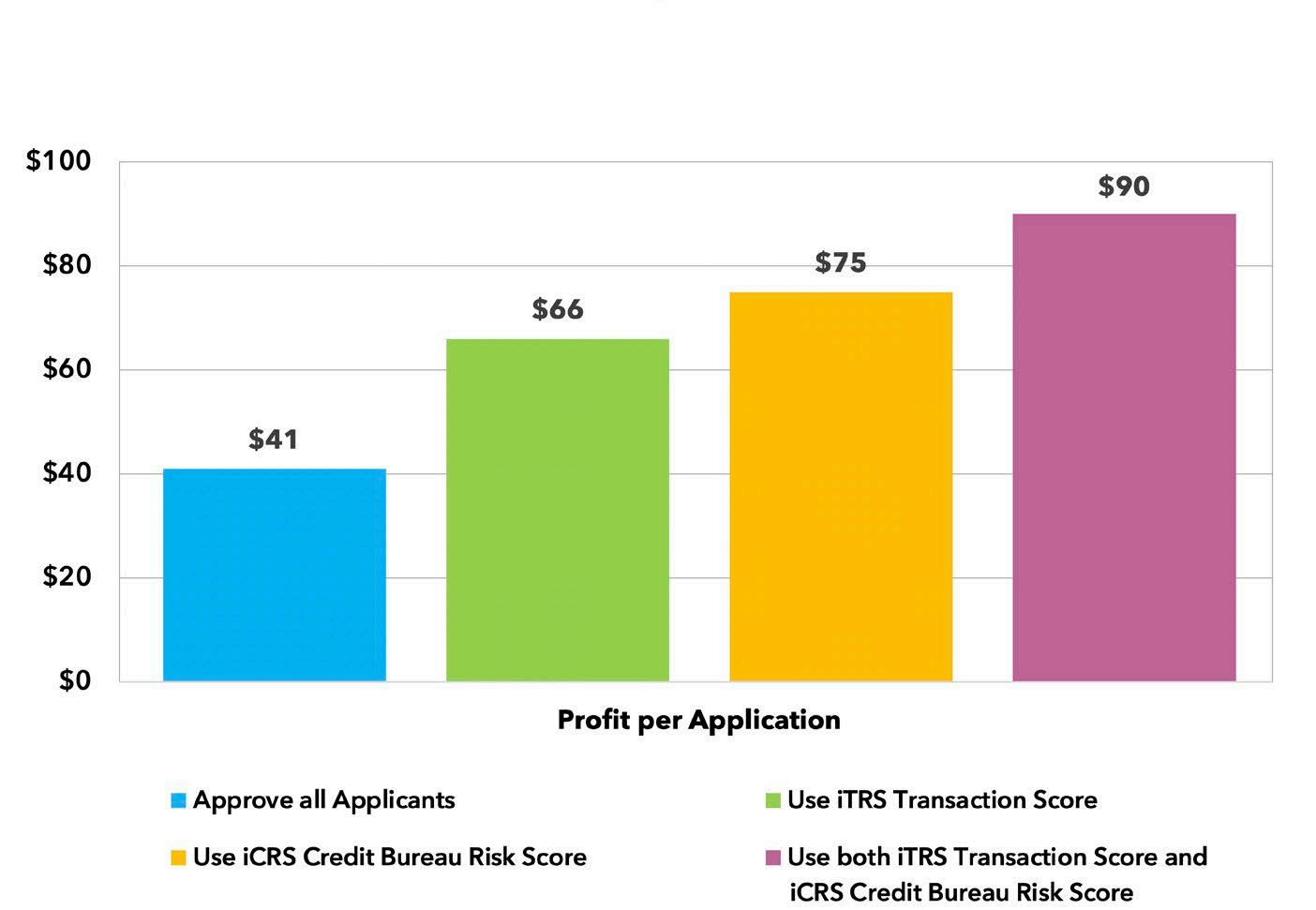
Profit per Credit Application from each Risk Assessment Scenario
Profit per Application
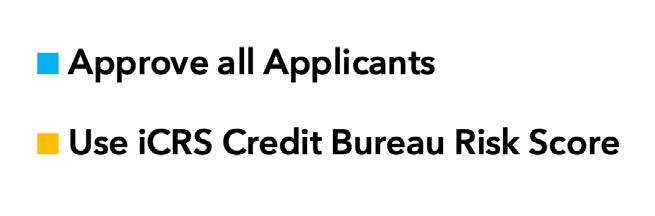
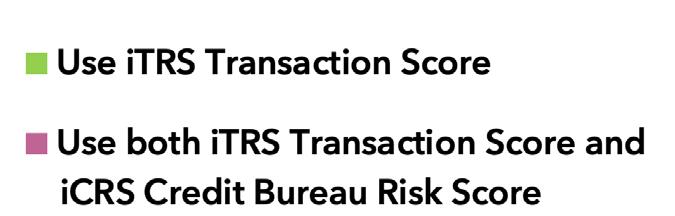
Banks and credit providers hold a raft of data that shows both the financial state and spending behaviour of their customers. What the score does is mine this data and make sense out of it in a straightforward manner.
While this data offers the capability to understand spending behaviour, most credit providers have made limited use of it for this purpose. For example, it has rarely been used to understand a consumer’s propensity to modify consumption habits in-line with their economic wellbeing.
Furthermore, by their nature, smaller banks have traditionally had limited access to transaction information, as they have generally held a secondary relationship with the consumer. This has disadvantaged them when competing with Tier 1 banking institutions for prime borrowers.
Developing Transaction Scores requires both a technical understanding of banking data structures as well as a deep knowledge of spending and broader consumption behaviour.
*Barrett Hasseldine Head of Modelling illion E: barrett.hasseldine@illion.com.au
FOOTNOTES: 1 Over the use of traditional credit risk data alone.
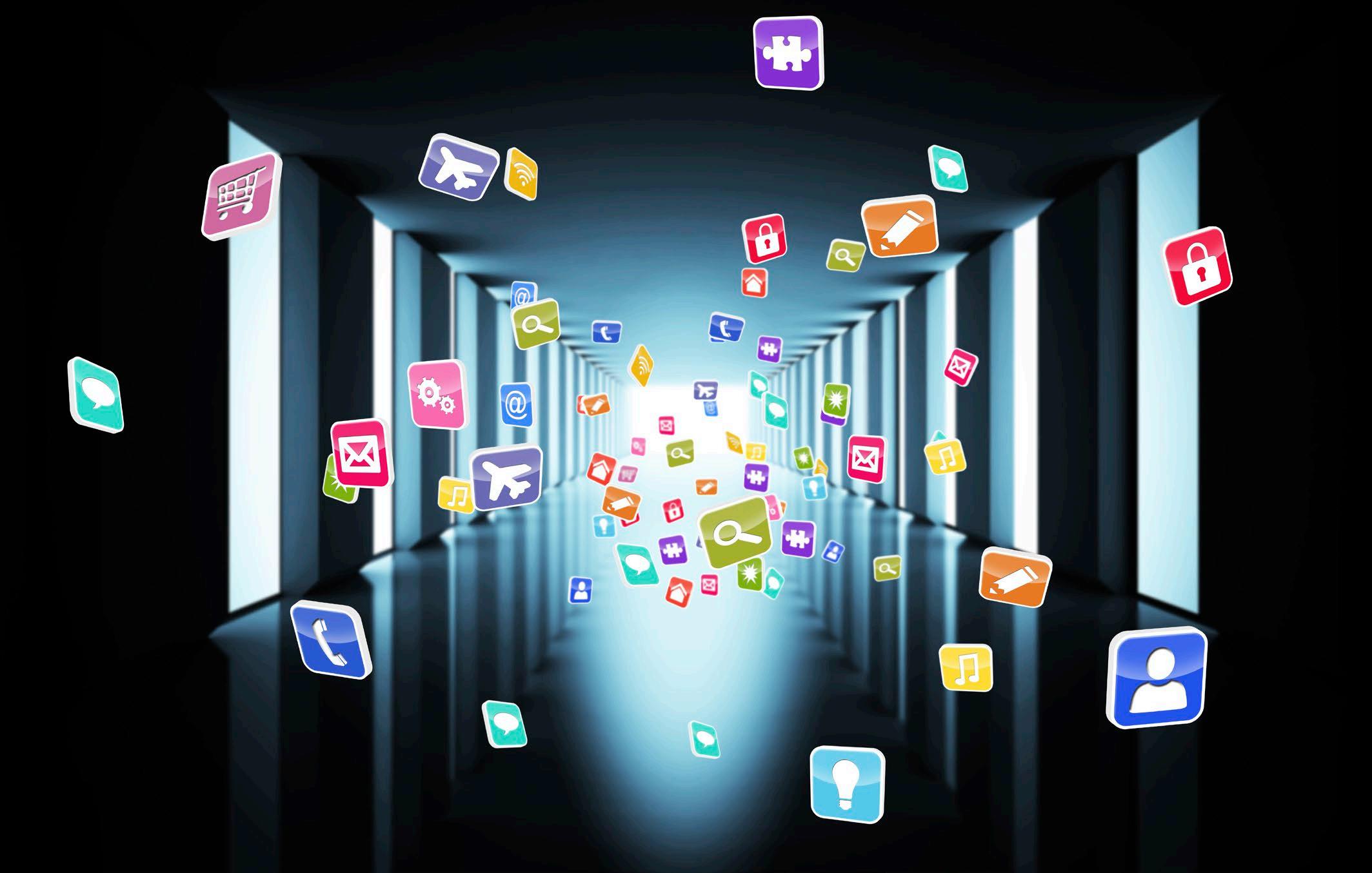