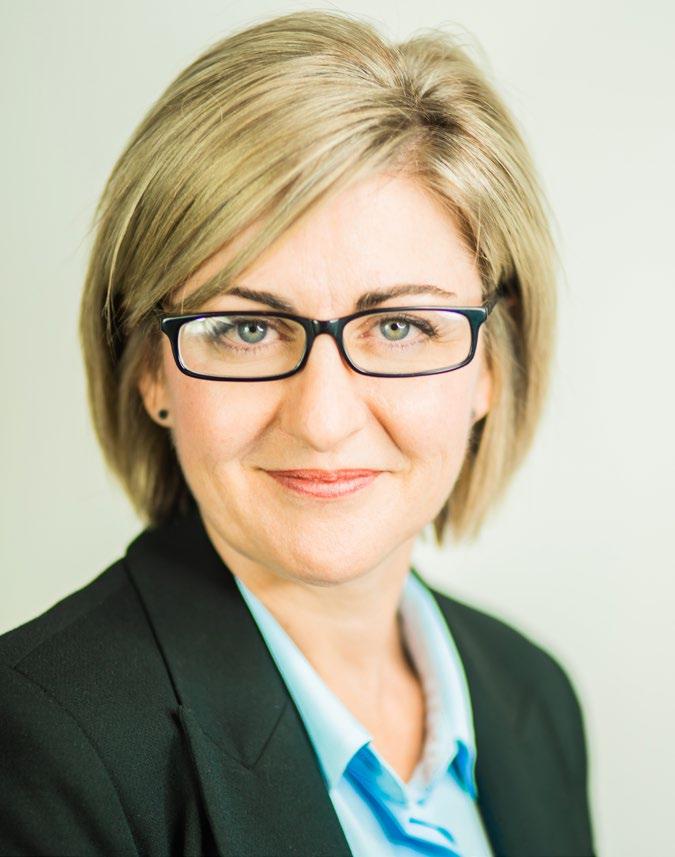
7 minute read
The rise of advanced, automated credit decisioning systems in 2022
Change is the only constant – are you adapting your credit decisioning models to maximise accuracy?
By Lynne Walton MICM*
Lynne Walton MICM
Winston Churchill once said “To improve is to change; to be perfect is to change often”. It seems he may have been talking about credit decisioning models at the time.
Of course, predicting outcomes can never be perfect but, to maintain a high level of accuracy in this unstable, fast moving world, change is really the only constant and incorporating that change into credit decisioning models in real time is the key to optimising business performance and avoiding failing customers and those least likely to pay within terms.
Implementing the right solution – one that delivers the required insight with speed, accuracy and confidence – can dramatically improve business results and lower operating costs but it’s important to remember that even the best models need to be constantly maintained.
This increasingly volatile economic environment is a catalyst to ask the question, when did we last re-visit our models? Are they still relevant today in this post covid world? Do we know how our scores are derived? Are
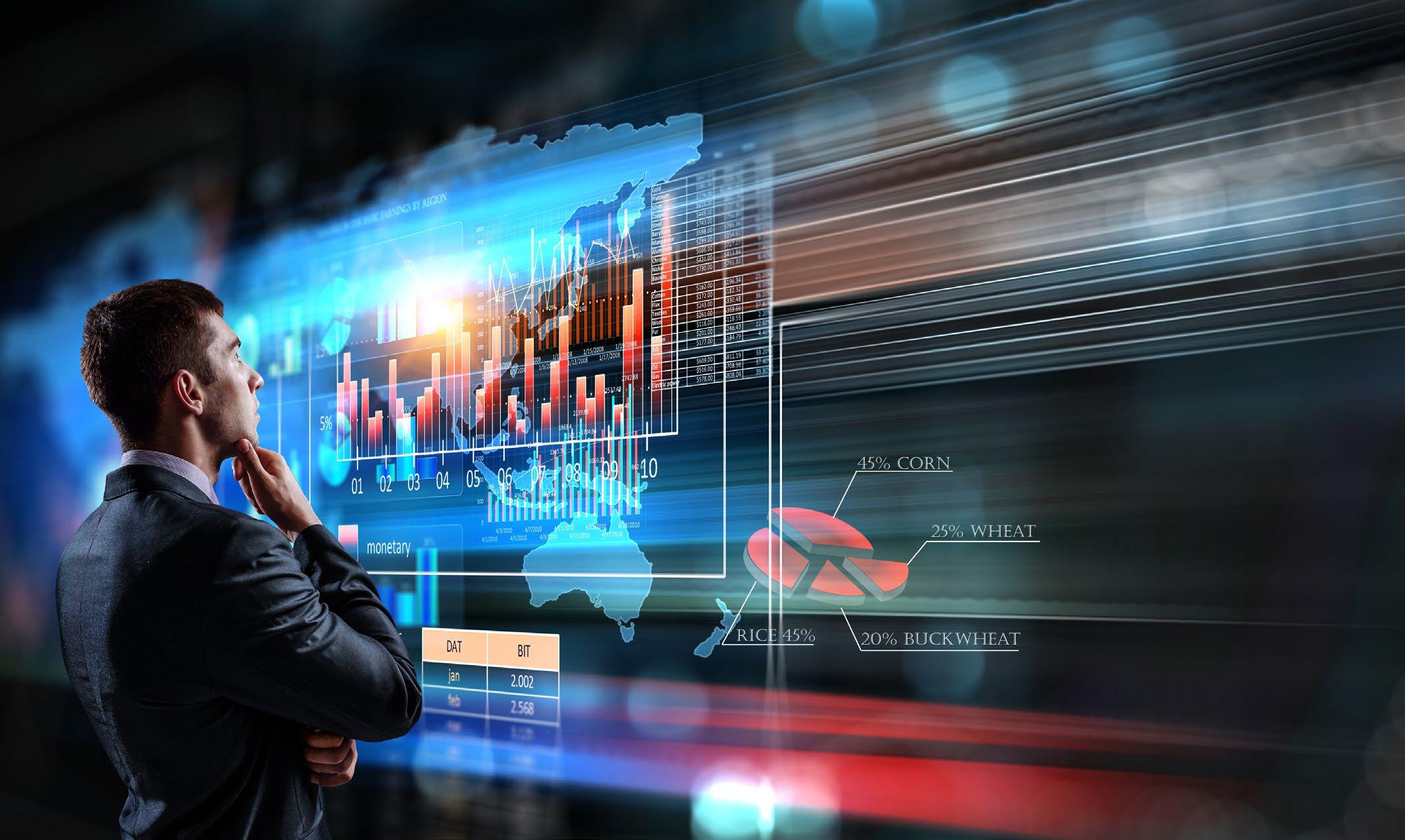
we rejecting profitable business because our ‘yes’ bar is too high?
What is a credit decisioning model?
A decisioning model takes a specific question and formulates an answer for the decision maker using predefined parameters. The model usually aggregates large amounts of information, applies multiple ‘rules’ and produces a result or set of results.
When it comes to trade credit decisioning the question might be ‘do we wish to approve this customer for a trade credit account?’. The model output may be ‘yes – open the account’, ‘no – do not open the account’ or ‘refer for further investigation’. The model may also be set to recommend a credit limit, approve an increase or set a review date.
Traditional methods of assessing the suitability of a prospective customer, largely based on distant historical performance, are now considered outdated and unreliable. Modern practices focus efforts on looking ahead at the potential of the prospect and use multiple, disparate data sources to predict the probability of an outcome.
Benefits of decisioning models
Credit decisioning models have multiple benefits. Practically they are much quicker than traditional methods. They are cost and labour resource efficient and enable a business to standardise decision making and uniformly and objectively apply a risk strategy whilst optimising control over the new customer approval and onboarding function.
For organisations with a high volume of account openings, processing can be systemised with automated approval and account opening following required criteria being reached. This tends to suit lower value exposures which lend themselves to automation with time and cost savings considerable.
Innovation in the credit management space has meant that a large number of businesses now share customer aging data in return for receiving credit risk scores. Recent payment history is prediction gold. For example, ➤
if no-one is getting paid, or a business is showing a dramatic downward trajectory in payment performance, the writing is on the wall. More often, however, the deterioration indicators are much more subtle and subjective. It’s therefore useful to have a solution which alerts users to a gradual decline over an extended period of time which can often indicate problems that may eventually be terminal.
Provided they consult the most appropriate data and are maintained correctly, even at the lower, more basic end of the sophistication scale, credit decisioning systems save time and money whilst improving performance and control over the entire customer onboarding process. As the higher end, the more advanced decisioning solutions have the potential to completely transform the fortunes of an organisation.
Maintaining decisioning models
One of the challenges in implementing a decisioning system is maintaining an organisational understanding of the mechanics of the model. There is a tendency to ‘set and forget’ risk parameters assuming that the status quo will remain which is, of course, never the case. It is therefore vital to have visibility and control over the settings. Even better is to use more advanced decisioning systems capable of instantly and automatically reflecting external influences both in terms of what they learn as they go (artificial intelligence) but also in terms of the foundation components – both the structured and unstructured data indicators which give rise to the risk output.
An example of where basic foundation components can cause a model to fail in the accuracy stakes is in reacting to change. We all accept that certain industries are more risky than others and hence industry specifics built into models. We know that covid has adversely affected the food service and construction industries in recent times and that aged care is relatively stable. But what happens if, as is contemplated, the government throws the struggling construction industry a lifeline? What does it mean if health care reforms adversely affect support measures for retirement or assisted living facilities? How quickly is this type of change reflected in our models? Ideally, changes like these would be implemented immediately and automatically. But are they?
Systems that clearly display risk model settings and an investment in educating and enabling authorised users (senior management) to adapt risk strategy variables to meet the ever changing landscapes we navigate will pay dividends in the long term.
Accessing the detail
We value ‘hard facts’ – the streams of aggregated data based on actuals because we understand them. Things like how long a business has been trading, their industry, their location, the volume and type of court actions they are involved in. These ‘hard facts’ have substance – they make sense to left brain logic and traditional decisioning systems consult this type of structured data to formulate results.
But what of the softer more intuitive indicators? Are these to be ignored? Of course not. Experience and instincts count and that’s why having access to the detail that produces a result can sometimes be more predictive than a score. One highly regarded credit manager we know with 40+ years experience regards businesses with long names a high risk. This, and many other, well-honed instincts of seasoned credit managers to sniff out the favourably scored inconsistencies are vital not only for higher exposures but for all accounts as we know how easily limits creep up over time. Experienced credit managers and those that have a natural tendency to dig deeper will always look beyond a score to understand how the model works behind the ‘yes’ or ‘no’ output. That’s why it’s important that users can gain access to the detail – the multiple components that create the ‘yes’, ‘no’ or ‘refer’ result.
Measuring performance
There is a tendency to enjoy dealing with a high revenue generating, promptly paying customer without requesting ‘more of the same’ from sales teams. Similarly, businesses tend to record an insolvency and move on to focus on customers that will pay without learning from it across the organisation.
The best credit decisioning models measure themselves and evolve over time. They use artificial intelligence to improve on past performance and increase prediction accuracy. The best credit teams reflect on outcomes and lessons learned. Applying outcomes, both positive and negative, will enhance the overall effectiveness of decisioning models and lead to improved profitability across the organisation.
Conclusion
Credit risk decisioning models applied through new customer onboarding and monitoring systems are becoming commonplace among forward thinking businesses in 2022. They have the potential to completely transform the fortunes of a business provided they are set up carefully with key influencing factors understood by management as well as being maintained well enough to reflect both macro and micro economic changes. Access to decision detail is advantageous both to protect against credit loss and to ensure the businesses is taking full advantage of all sale opportunities.
*Lynne Walton MICM CEO Access Intel www.accessintel.com.au https://www.linkedin.com/in/lynne-walton0241b861/
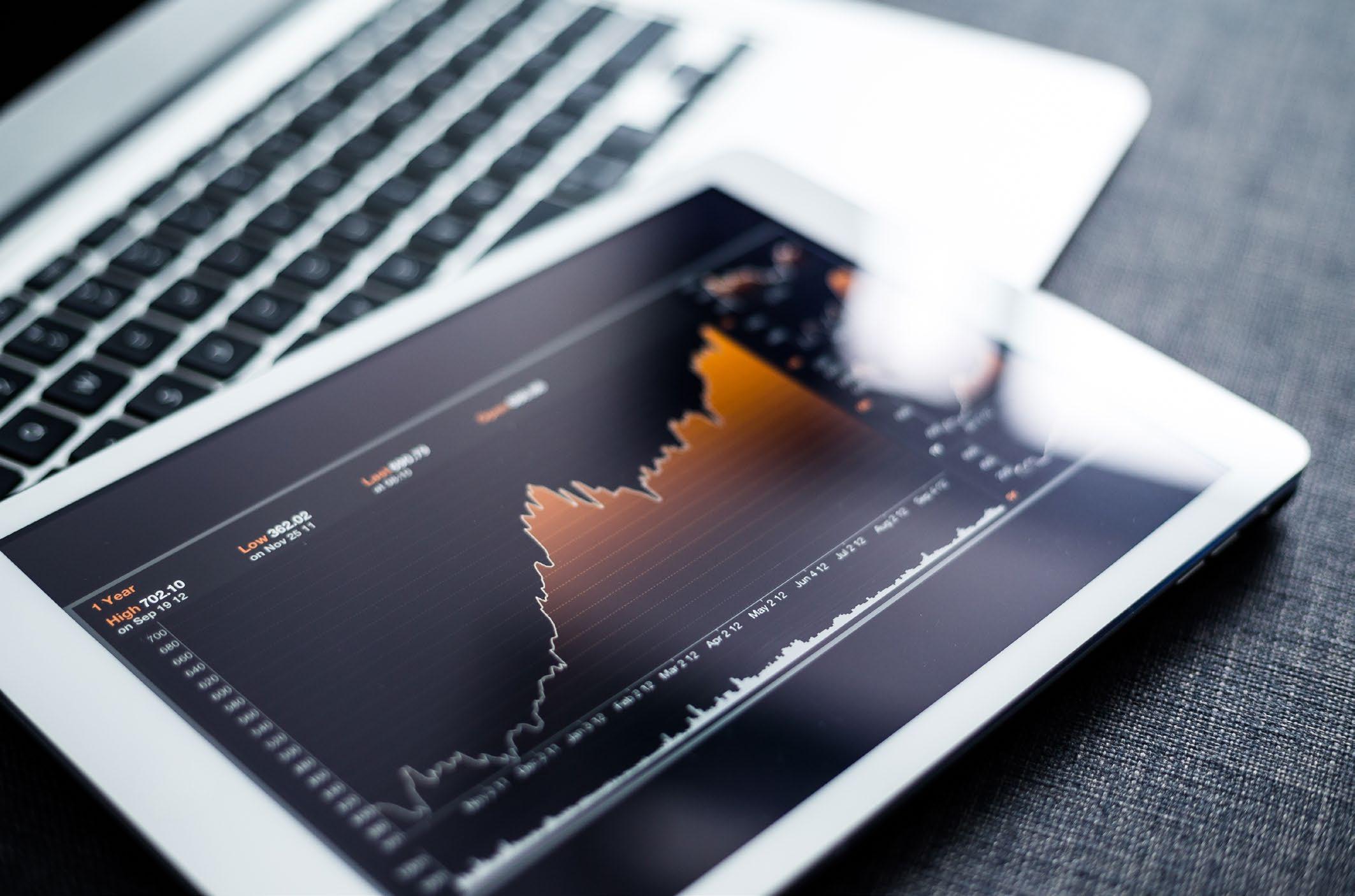