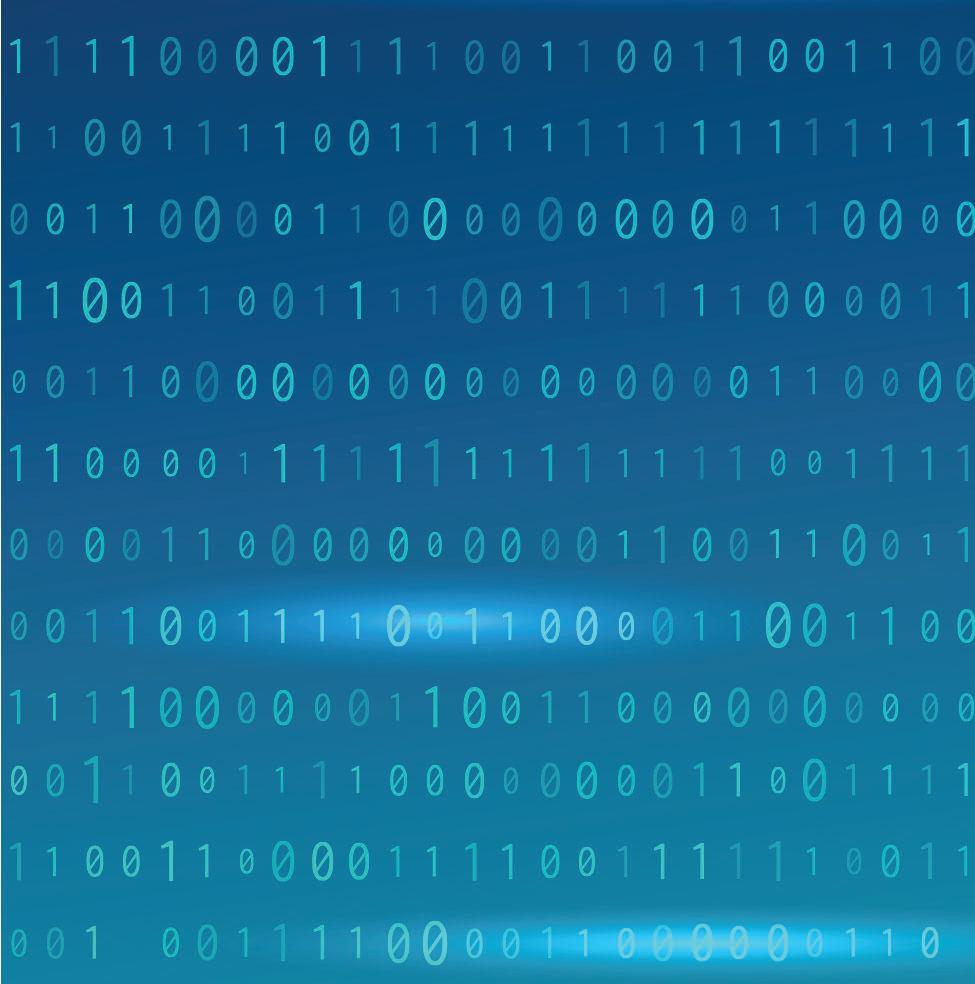
2 minute read
The Key Pros and Cons of Cloud and Edge AI
Security Considerations
One of the most significant advantages of Cloud AI over Edge AI is security. Cloud platforms, like those used in large-scale video monitoring, leverage robust infrastructure provided by cloud service providers such as AWS, Google, or Azure. These platforms benefit from extensive security protocols, including encryption, multi-factor authentication, and regular updates to protect against emerging threats. By centralizing data in the cloud, these systems can ensure that all data— whether it’s real-time video streams, archives, or event logs—is consistently protected under the highest security standards.
Advertisement
Advantages of Cloud AI:
• Minimal edge attack surface
• No physical servers to protect
• No black-box device in the on-prem network that could potentially be compromised
• With direct-to-cloud encrypted connections, there is no need to worry about port forwarding, firewalls, or devices being exposed to attacks
• Responsibility for project security shifts from local IT personnel to the cloud provider
Disadvantages of Cloud AI:
• Data stored on a cloud triggers recurring costs
• Sufficient network bandwidth is required to upload/download data
Cloud AI significantly reduces the burden on local IT teams by minimizing the on-premises attack surface to only the cameras. Modern cloud-enabled cameras can connect directly to AWS over a secure tunnel, further enhancing the security of the video infrastructure. On the other hand, Edge AI devices have a similar attack surface to regular onpremise servers. They also add an extra burden by being a black box from an IT perspective.
• Centralized cloud software updates and security patches
• Centralized monitoring and AI anomaly detection
• Centralized IAM (Identity Access Management)
• End-to-end data encryption is the standard for AWS cloud, for example
• Flexible KMS encryption allows customers to encrypt data with their own keys, ensuring privacy and data protection
• High data durability (e.g., 11 9s for AWS S3 cloud storage)
• Comprehensive compliance support data available for training, advances in model optimization algorithms, and the continuous growth of computational resources.
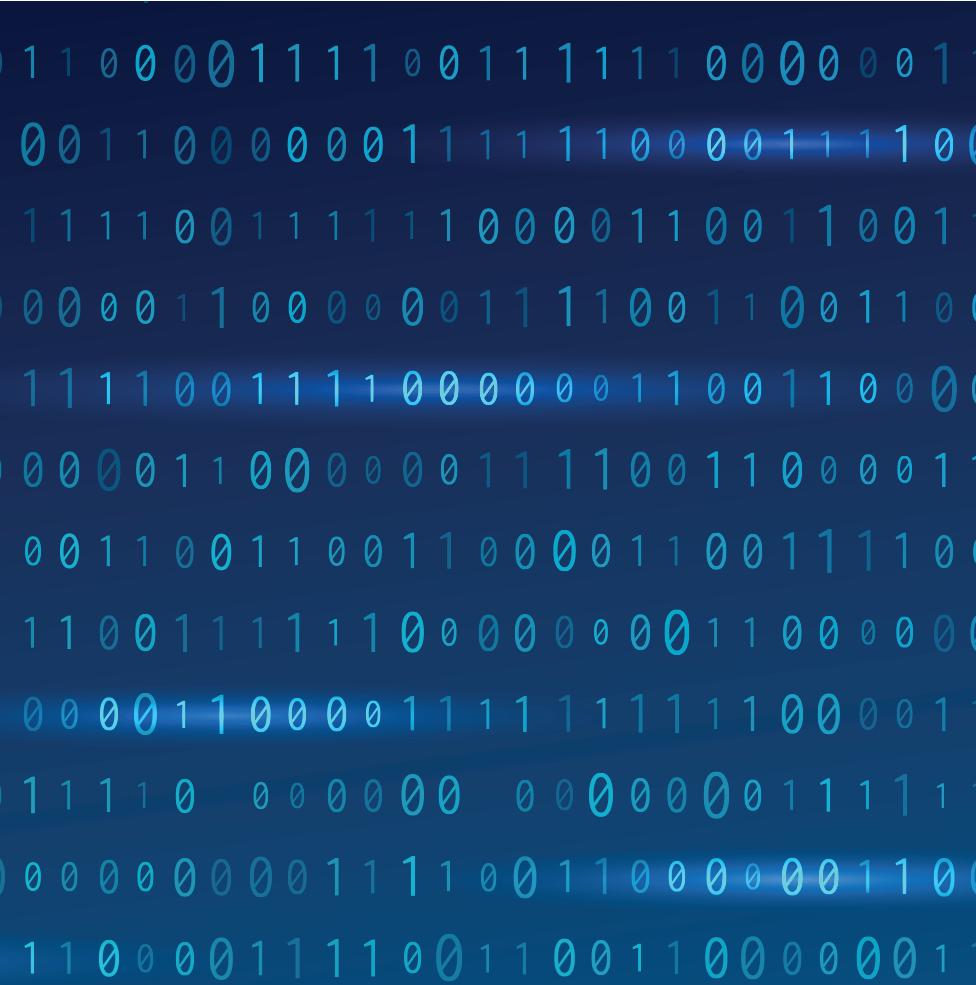
While servers are transparent to IT management, Edge AI devices are not, and if such a device is compromised, IT personnel may have no control or even a way to be alerted about the attack.
Therefore, deploying additional Edge AI devices increases the strain on IT teams, requiring them to move these devices to a separate network, protect them behind a firewall, and monitor traffic.
Multilocation installations escalate this burden further, as each location must protect and monitor its edge device in a centralized fashion, effectively pushing the IT team to recreate or adopt a thirdparty centralized solution to safeguard such multilocation installations.
Edge devices face significant constraints due to their limited computational resources, which must be divided among archiving, decoding, streaming, and AI processing tasks.
These limitations force compromises in several areas:
• AI model size and complexity
• The amount of video that can be simultaneously decoded and processed
• The number of AI modules that can run concurrently
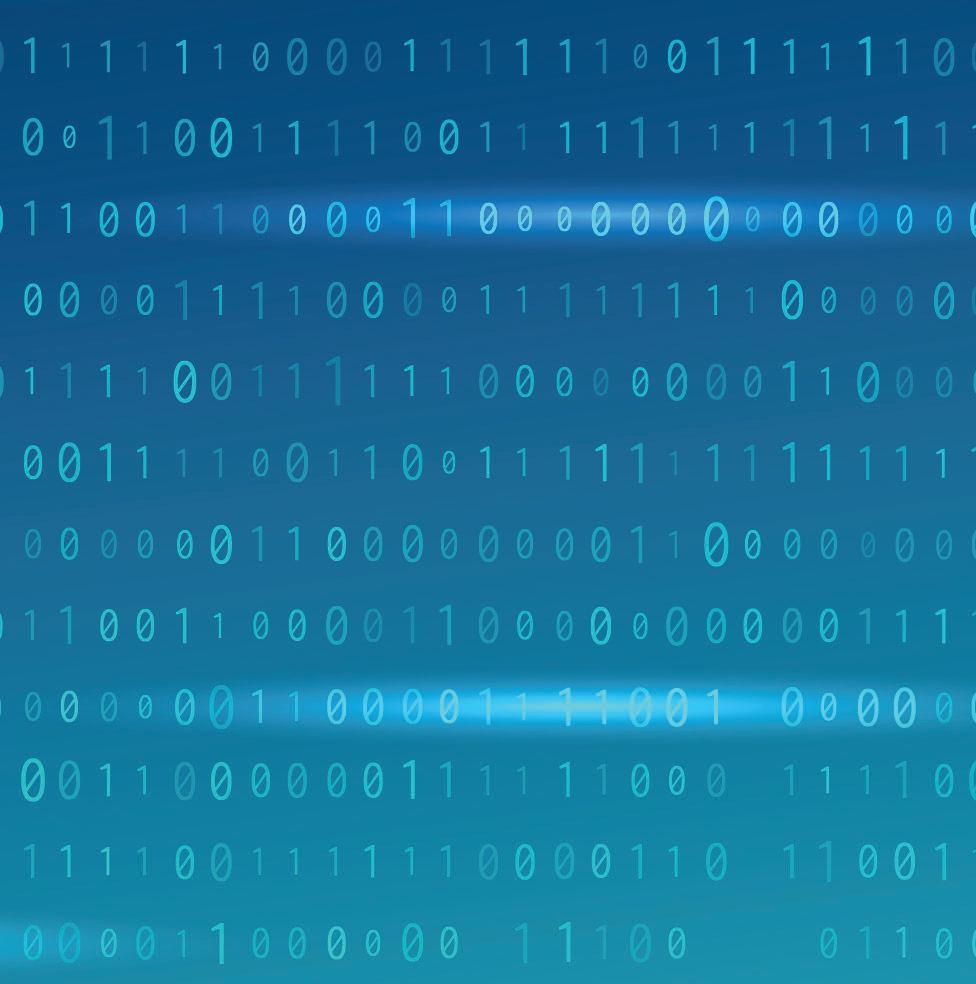
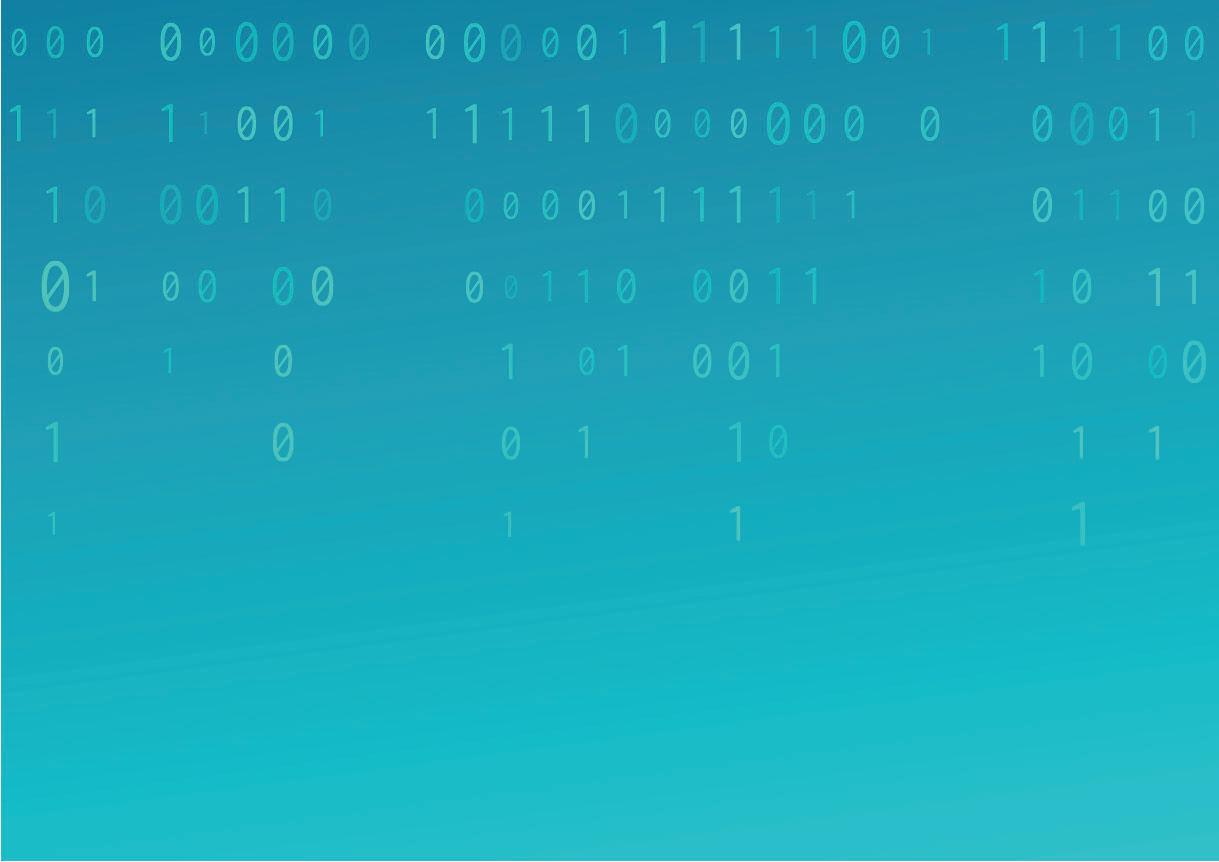
Scalability and Flexibility
Cloud AI platforms are highly scalable, making them ideal for large-scale video monitoring. As AI rapidly evolves, particularly in computer vision and large language models (LLMs), Cloud AI effortlessly manages increasingly complex scenarios. This capability is supported by the vast
As AI rapidly advances, edge devices often require frequent upgrades to accommodate newer, more computationally intensive analytics and larger, more complex AI models.
In contrast, Cloud AI solutions offer virtually unlimited scalability. As more cameras and data sources are added, cloud systems can easily expand their processing power and storage capacity to meet demand. This scalability is crucial for monitoring applications that must handle increasing data volumes without performance degradation.
We are currently witnessing the early stages of exponential growth in AI capabilities, driven by several converging factors. Cloud AI’s flexibility and scalability position it ideally to leverage these advancements without the hardware limitations inherent to edge devices.
Key Growth Factors
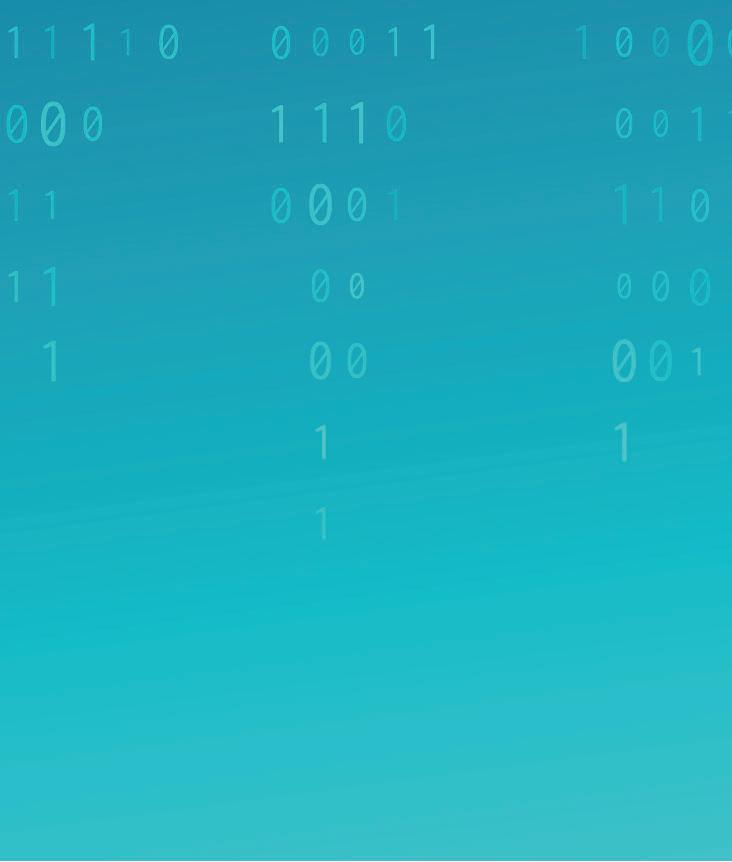
• Projected expansion of the security camera install base from 1.2 billion to 2 billion by 2030
• A shift in the $100 billion alarm monitoring market towards cloudconnected cameras
• Rapid growth of the Video Surveillance as a Service (VSaaS) market, with cloud-connected cameras seeing a 30% year-overyear increase
Cover Story continued on page 30
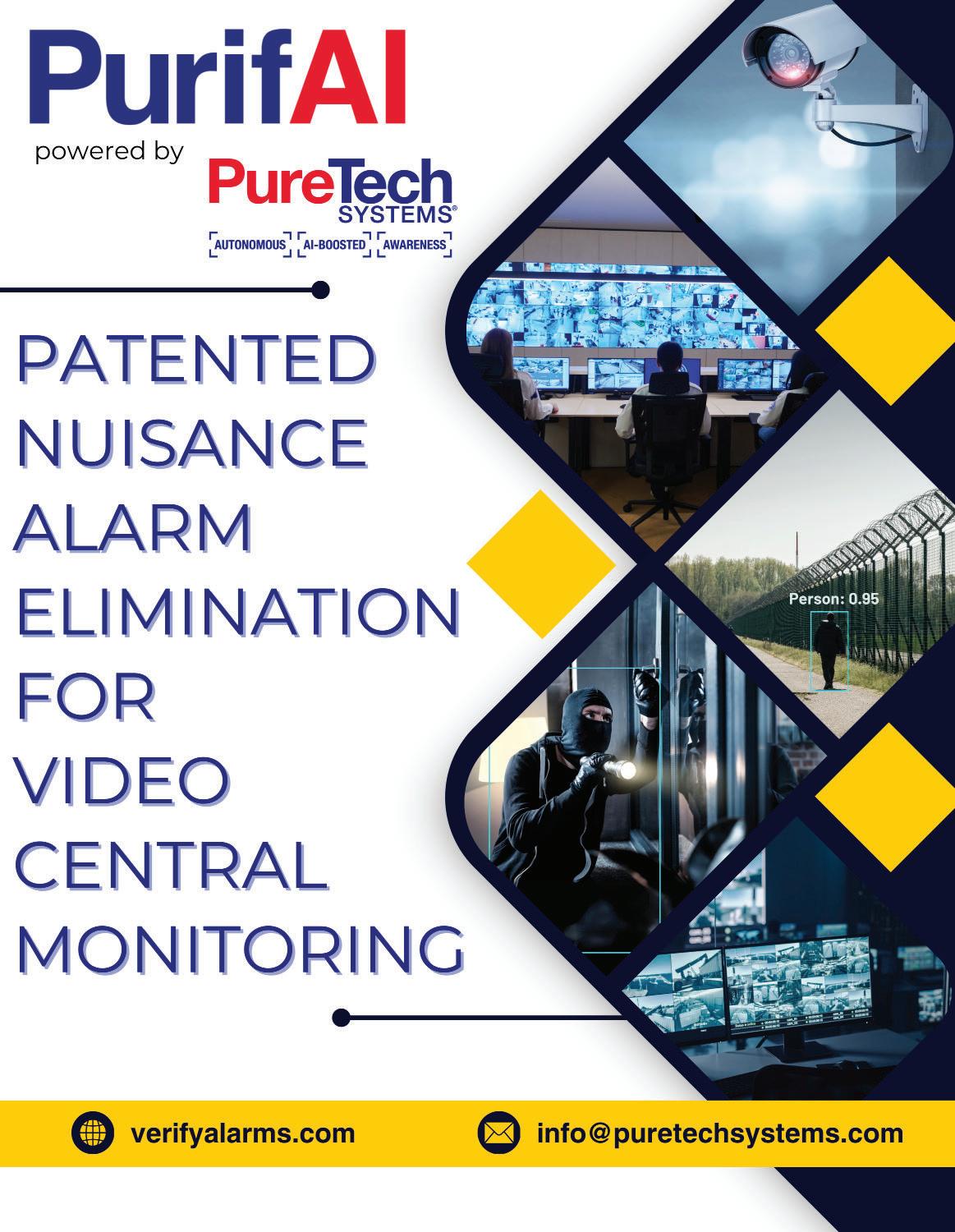