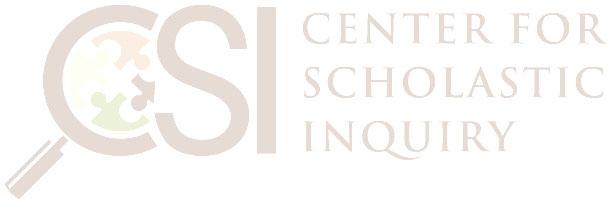
27 minute read
Sources of Academic Self-efficacy & Academic Performance in Online Learning Jennifer Miller, Tougaloo College Jillian Skelton
Sources of Academic Self-efficacy & Academic Performance in Online Learning
Jennifer Miller Tougaloo College
Advertisement
Jillian Skelton
Abstract
The purpose of the correlational study was to examine to what extent relationships exist between the four sources of academic self-efficacy in math and academic achievement for online undergraduate math education students. Data were collected from 93 undergraduate math education students enrolled fully and exclusively in an online program at a major university in Arizona, using an online survey comprised of self-reported cumulative grade-point average and Zientek, Fong, and Phelps’s modified version of the sources of self-efficacy in mathematics scale. The multiple linear regression results indicated mastery experiences significantly and positively predicted academic achievement, which aligned with past research from traditional learning environments and the original theory. However, the results also indicated verbal persuasion was a significant negative predictor of academic achievement, but neither vicarious experiences nor physiological state were statistically significant, results that differ from both past research conducted in traditional learning environments and the original theory.
Keywords: Academic self-efficacy, academic performance, online learning
Introduction
Online learning is emerging as a key technology for undergraduate education, with over 5 million students taking at least one course online in the United States in 2016 (Allen, Seaman, Poulin, & Straut, 2016). However, as this platform expands in use, so too are the dropout rates expanding, with online programs yielding attrition rates 3% to 5% higher than traditional programs (Cochran, Campbell, Baker, & Leeds, 2014; Lee, Choi, & Kim, 2013). Among those
college students dropping out of their programs, education majors have the highest attrition rates (Chen & Soldner, 2013), which is particularly concerning, due to the current shortage of math teachers in the U.S. (Diekman & Benson-Greenwald, 2018). Research into student attrition has identified academic performance as a key factor in online student drop out (Lee & Choi, 2011; Park, Luo, & Kim, 2015). Current studies continue to confirm a positive correlation between academic self-efficacy and its sources with academic performance in traditional college environments (e.g., Alyami et al., 2017; Bartimote-Aufflick, Bridgeman, Walker, Sharma, & Smith, 2015; De Clercq, Galand, Dupont, & Frenay, 2013; Doménech-Betoret, Abellán-Roselló, & Gómez-Artiga, 2017; Farchi, Cohen, & Mosek, 2014; Fong & Krause, 2014; Høigaard, Kovač, Øverby, & Haugen, 2015; Macaskill & Denovan, 2013; Trigwell, Ashwin, & Millan, 2013; Zientek, Fong, & Phelps, 2017), but it was not known if such relationships exist in online learning environments. In studying the four sources of self-efficacy in underachieving students in a traditional education program, Fong and Krause (2014) concluded that it might be the sources themselves that relate directly to academic achievement. Hodges (2008) hypothesized that there might be different sources of self-efficacy in an online learning environment. There does not appear to have been any follow-up in the literature to this idea from Hodges (2008) or any study that examined all four sources directly in the context of online learning, so this study addressed that gap and followed up on Hodges’ (2008) hypothesis.
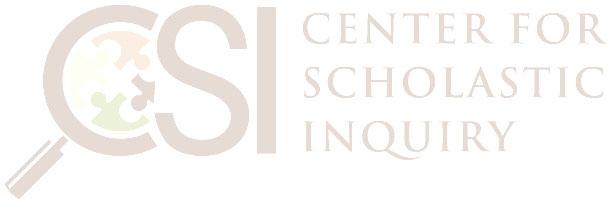
Academic Self-Efficacy
According to Bandura (1977), self-efficacy is the evaluation of one’s ability to produce a desired effect; the internal process by which people initiate and persist in a behavior. Bandura (1986) claimed that self-efficacy is context-specific, with levels that vary across domains and later described it as “a differentiated set of self-beliefs linked to distinct realms of functioning” (Bandura, 2018, p. 133), such as the realm of academics, which would yield academic selfefficacy. According to Bandura (1978), the internal self-efficacy process is influenced, but not controlled, by the environment. Changing the environment to an online setting would result in an alteration in a student’s sense of efficacy, which, also according to the model, would alter the initiation of and persistence in academic behaviors.
Unfortunately, research specific to academic self-efficacy in online learning environments has primarily focused upon technology-related factors, such as computer selfefficacy, Internet self-efficacy, and Learning Management System self-efficacy (Alqurashi, 2016). In studies that mentioned academic self-efficacy, it was identified as a mediating factor for other learning-related concepts, such as metacognitive regulation (Cho & Shen, 2013), intrinsic motivation and self-regulated learning behavior (Pardo, Han, & Ellis, 2017), and helpseeking behavior (Dayne, Hirabayashi, Seli, & Reiboldt, 2016). In the research conducted about online learning, the sources of academic self-efficacy have not been studied directly, but have rather been included in studies as an aspect of a main variable or as a treatment condition in the investigation of learning-related concepts, such as instructor feedback (Alrushiedat & Olfman, 2014; Delaval, Michinov, Le Bohec, & Le Henaff, 2015; Ladyshewsky, 2013; Lawanto, Santoso, Lawanto, & Goodridge, 2014; Pardo et al., 2017; Shen, Cho, Tsai, & Marra, 2013; Wang, Shannon, & Ross, 2013). Sources of self-efficacy. Bandura (1977) identified four sources of information that people use when evaluating their capabilities and developing their sense of efficacy: mastery experiences, vicarious experiences, verbal persuasion, and physiological states. Self-efficacy develops through the cognitive processing of this information, not from the sources directly, which means that not all experiences affect one’s level of self-efficacy. As such, self-efficacy is beliefs about skill and not always reflective of actual skill. Bandura (1986) found that the same level of capability can result in subpar, ordinary, or extraordinary performances, depending upon the level of self-efficacy.
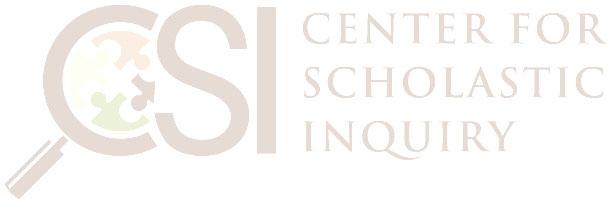
Sources of Self-efficacy in Traditional Learning Environments
Since that first experiment on the four sources with people suffering from a severe snake phobia (Bandura, 1977), mastery experience has frequently been identified as the strongest influencer of self-efficacy. Fong and Krause (2014) confirmed this conclusion in a mixed methods study on underachieving college students, with underachieving defined as a discrepancy between predicted ability and actual performance. Fong and Krause (2014) reported that selfefficacy was positively correlated with both mastery experiences and verbal persuasion, but negatively correlated with both vicarious experiences and physiological states. The group of
underachieving students reported a significant lack of mastery experiences and less verbal persuasion than the group of achieving students (Fong & Krause, 2014). The actual levels of self-efficacy did not differ between the groups, but there was a sufficiently significant difference between the sources that Fong and Krause (2014) concluded that the antecedents to efficacy are the salient factors to performance. In a later study, Zientek et al. (2017) investigated the four sources of self-efficacy in remedial math community college students. The results indicated that all four sources of selfefficacy explained the variance in the students’ math skills (Zientek et al., 2017). However, while all four sources influenced self-efficacy, mastery experience was determined to be the strongest predictor (Zientek et al., 2017). Studies with college students in Singapore (Loo & Choy, 2013) and Taiwan (Lin, 2016) also found all four sources correlated with self-efficacy. In examining the influence of all four sources of self-efficacy on academic self-efficacy, computer self-efficacy, and programming self-efficacy in undergraduate students in computing majors, Lin (2016) reported that all four sources of self-efficacy contributed significantly to the variance in academic self-efficacy. Lin (2016) also noted that more persistent students had higher scores in mastery experiences, vicarious experiences, and verbal persuasion. Loo and Choy (2013), on the other hand, found all four sources to be significantly interrelated for math students. While there was a significant positive correlation between academic performance and all four sources, mastery experience had the strongest correlation (Loo & Choy, 2013). Additionally, only mastery experience explained the significant variance in predicting academic performance (Loo & Choy, 2013). Fong and Krause (2014) included a similar note about how it may be the sources themselves that relate to academic achievement.
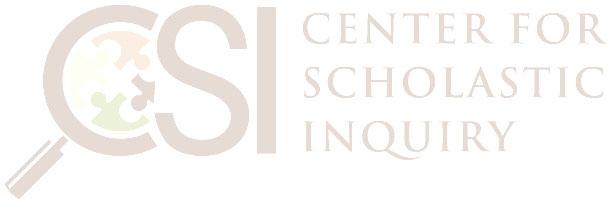
Sources of Self-efficacy in Online Learning Environments
It does not appear that the four sources of self-efficacy were examined together in the context of online learning prior to this study. Instead, prior research in the realm of online learning has examined individual sources. While research in traditional learning environments has repeatedly reported how mastery experiences is the strongest predictor of self-efficacy, only Jan (2015) reported that the strongest correlation was between prior experience and academic
self-efficacy. Other studies have similarly reported that how prior experience leads to increased self-efficacy, but they have not identified it as the strongest or most significant source of selfefficacy (Bradley, Browne, & Kelley, 2017; Hodges, 2008; Shen et al., 2013). Online learning presents a significant challenge to vicarious learning experiences since student interaction takes place online and in a disjointed manner, as opposed to the immediate face-to-face interactions available in traditional learning environments. Among undergraduate and graduate students in the United States, hybrid courses, in which students participate both inperson and online during the course, were rated more satisfactory than fully online courses (Cole, Shelley, & Swartz, 2014). The primary reason cited for the dissatisfaction with the fully online course was the lack of interaction with both instructor and fellow students (Cole et al., 2014). Even the limited interaction provided in a hybrid format was preferable to the online interaction of an exclusively online learning environment. Such studies lend support to Kozar, Lum, and Benson’s (2015) hypothesis that online learners are at a disadvantage because of the limitations placed on vicarious learning by the online environment. Another source that is affected by the online learning environment is that of verbal persuasion, particularly in the case of instructor interaction and feedback, since the online format typically keeps interactions in a textual format. A mixed methods study compared two groups of underperforming freshmen students, with one group receiving web-based, face-to-face tutoring (such as through Skype) and the other group receiving only text-based online tutoring (Wu, Lin, & Yang, 2013). Both groups showed improvement in their academic skills, but only the face-toface group reported increased in perceived efficacy (Wu et al., 2013). As for the source of physiological state, many of the studies that have reported on the role of emotions in online learning have done so in the context of studying self-regulated learning. Also, as with the research on learning-related emotions and self-efficacy in traditional learning environments, these studies have focused primarily on anxiety. For example, Pardo et al. (2017) investigated self-regulated learning and academic performance in online learning, including the variables of self-efficacy and test anxiety. The findings revealed a negative correlation between self-efficacy and test anxiety and a positive correlation between test anxiety and a negative selfregulated strategy (Pardo et al., 2017). In addition to test anxiety lowering self-efficacy and
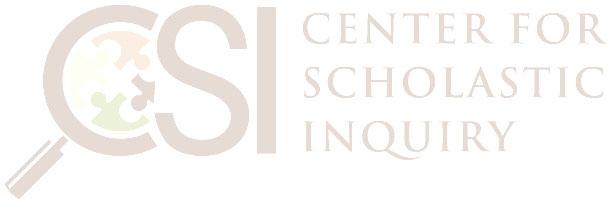
increasing the usage of negative strategies, it was also found that test anxiety has a significant negative impact on academic performance (Pardo et al., 2017). The purpose of this correlational study was to fill this gap in the literature by examining all four sources of self-efficacy together in an online learning environment, specifically focusing upon math education students and their sense of efficacy in learning math online. This study investigated if relationships exist between the four sources of self-efficacy in math and academic performance for college students who have been enrolled fully and exclusively in an online math education program for at least one year at a large private university. The research question examined was: To what extent do the four sources of math self-efficacy (mastery experiences, vicarious experiences, verbal persuasion, physiological state) predict academic performance for undergraduate math education students with at least one year of exclusively online courses at a large private university in the southwestern United States? To address this question, the following hypothesis was made: at least one of the four sources of math self-efficacy (mastery experiences, vicarious experiences, verbal persuasion, physiological state) will be a statistically significant predictor of academic performance for undergraduate math education students with at least one year of exclusively online courses at a large private university in the southwestern United States.
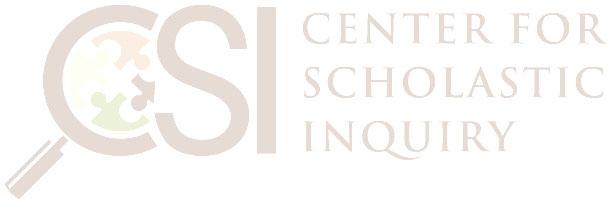
Methods
Participants
Participants (n = 93) were students enrolled in a full-time online undergraduate math education degree program at a large private university in the southwestern United States. All participants confirmed that they had been taking courses exclusively online, with no in-person courses, for at least one year. No demographic information was collected from participants.
Procedures
Data was collected using an online survey distributed via email by the university to all students enrolled in the online math education undergraduate degree program. Participants were informed of the inclusion criteria, with 103 confirming they were at least 18 years old and 100
confirming they were in the online math education program with exclusive online coursework for at least one year. All 100 consented to participate, but only 93 then completed the survey. A $5 e-gift card was offered as an incentive, with only 34 claiming the incentive at the end of the
survey.
Measure
The survey did not collect any demographic information from participants. To measure academic achievement, student cumulative grade point average (GPA) was collected via selfreport as the first question on the survey.
Sources of self-efficacy in math. The four sources of self-efficacy in math were measured using all four subscales of the Sources of Self-efficacy in Math Scale (SSEMS) (Usher & Pajares, 2009), using a 6-point Likert-type scale ranging from 1 = definitely false to 6 = definitely true. Scores for the physiological state scale were reverse coded and then averaged together to measure the physiological state source of self-efficacy in math. For the other three scales, the scores were also averaged together to create a mean measure of the mastery experiences source of self-efficacy in math, the vicarious experiences source of self-efficacy in math, and the verbal persuasion source of self-efficacy in math. Since the original instrument was designed for middle school students, the Zientek et al. (2017) modified version of the SSEMS was used. This modified version adjusted the wording of two items to better fit college students, reporting similar internal reliability to the original version (Zientek et al., 2017).
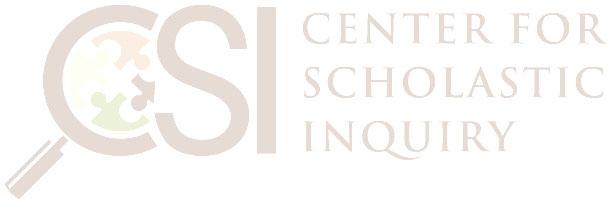
Analysis
Prior to conducting statistical tests, preliminary analyses using SPSS were run to ensure the data met the required assumptions for the planned statistical test. Basic descriptive analyses presented means and standard deviations for all variables. Scores for all variables were converted to z-scores for analysis.
Results
This section demonstrates the breakdown of the data to create results. The first section
explains the initial preliminary analysis. The next level of analysis was a multiple regression analysis. The final stage of the results was a post hoc.
Preliminary analyses. Since the SSEMS had not been used in an online learning environment in past studies, a reliability analysis was conducted on all four subscales. The mastery experiences subscale had a Cronbach’s alpha of 0.80. The vicarious experiences subscale had a Cronbach’s alpha of 0.62. The verbal persuasion subscale had a Cronbach’s alpha of 0.94. The physiological state subscale had a Cronbach’s alpha of 0.90. The scores for mastery experiences and vicarious experiences are similar to those reported by Usher and Pajares (2009) in the validation study for this instrument: 0.86 for mastery experiences and 0.68 for vicarious experiences. However, the scores for verbal persuasion and physiological state were higher than that reported by Usher and Pajares (2009): 0.82 for verbal persuasion and 0.84 for physiological state, respectively. See Table 1 (Appendix A) for comparison of Cronbach’s alpha scores from this study and the original validation study of the instrument (Usher & Pajares, 2009).
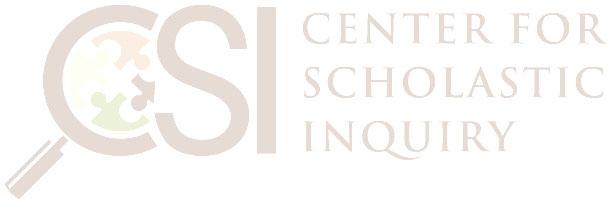
Multiple linear regression. A standard multiple regression was run to predict student GPA from each of the four sources of self-efficacy in math (mastery experiences, vicarious experiences, verbal persuasion, physiological state). Table 2 (see Appendix B) summarizes the standardized beta coefficients for the variables of mastery experiences, vicarious experiences, verbal persuasion, and physiological state. Using a statistical significance level of .05, the multiple regression model statistically significantly predicted student GPA, F(4, 83) = 3.385, p = .013, adj. R2 = .099. Only the variables of mastery experiences (β = 0.399, t = 2.782, p = .007) and verbal persuasion (β = -0.353, t = 3.107, p = .003) were statistically significant to the prediction. Vicarious experiences (β = -0.183, t = -1.51, p = .135) and physiological state (β = .0127, t = 1.047, p = 2.98) were not significant predictors. The overall model fit was R2 = 0.14. Based on findings, the null hypotheses for mastery experiences and verbal persuasion were rejected in favor of the alternative hypotheses. However, the null hypotheses for vicarious experiences and physiological state were accepted.
Post-hoc tests. The actual sample size of the study was 93 undergraduate students enrolled in an online math education program. The post-hoc achieved power analysis result was .84. Additionally, a post hoc sensitivity test was conducted with a result of .13.
Discussion
The results of this study are noteworthy because this study may be the first to examine all four sources of self-efficacy in the context of online learning. The results indicated that only two of the sources had a statistically significant predictive relationship with academic performance for online undergraduate math education students. Mastery experiences and verbal persuasion were statistically significant, while vicarious experiences and physiological state were not. The results indicated that mastery experiences was the strongest predictor of academic achievement, which aligns with a study conducted in a traditional learning environment with undergraduate engineering students (Loo & Choy, 2013). However, the results for verbal persuasion showed a negative correlation, which is different from what past studies have shown; yet it was second in strength, which does align with studies that found verbal persuasion to be both strongly interrelated with mastery experiences and second in strength of influence (Lent, Ireland, Penn, Morris, & Sappington, 2017; Loo & Choy, 2013; Usher & Pajares, 2006; Zelenak, 2015). What remains unknown is why the correlation between verbal persuasion and academic achievement was negative in the context of online learning, but positive in traditional learning environments. Hodges (2008) may be correct in his hypothesis that there may be different sources of self-efficacy in online learning environments from the four that were identified as part of the original theory. This may be particularly true due to triadic reciprocal determinism. For example, the source of vicarious experiences did not show up as statistically significant. Vicarious experiences involve watching others succeed. Such observations are readily available in traditional learning environments, but such observations are restricted, if not prevented, in online learning environments. By changing the environment, the source experience is altered, which changes its influence upon behaviors. Cole et al. (2014) noted that the primary reason that students cite for dissatisfaction with online courses is the lack of interaction with the instructor
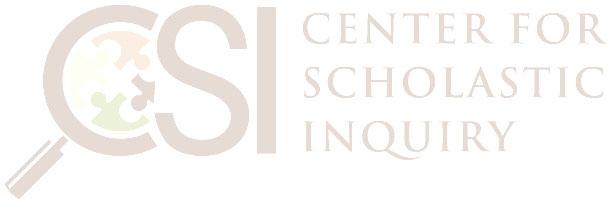
and with fellow students. Kozar et al. (2015) hypothesized that online learners are at a disadvantage because of the limitations on vicarious learning in online learning environments.
Triadic reciprocal determinism explains this change because according to that concept, changing the environment would have a reciprocal effect on the student’s personal qualities, including their sense of academic self-efficacy. An interesting implication from this study’s findings is this negative correlation between verbal persuasion and academic achievement for online undergraduate math education studies, which differs from what the original theory presented about this source experience, as well as what past research in traditional learning environments have indicated about this source experience. However, this particular finding may also be explained by the theory of triadic reciprocal determinism by way of the environmental events – behavior path, which is distinct from the environmental events – personal factors path that involves self-efficacy; meaning that changing the environment from in-person to online alters the verbal persuasion source experience in a manner that inversely affects student GPA. In a traditional setting, instructors provide various forms of feedback, including written, verbal, paraverbal, and nonverbal. In an online environment, feedback is only in written form and provided as feedback to posted work, rather than as part of in-person interactions during class time. This limited method of feedback may explain the negatively related effect in the online setting.
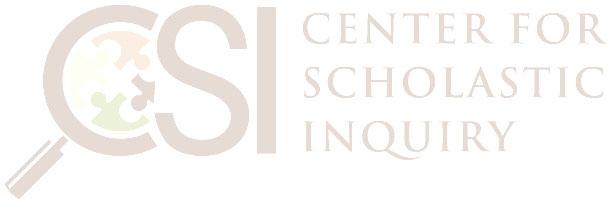
Limitations
The findings of this study are limited to the sample of online undergraduate math education majors from the private university used to recruit participants because it is a correlational study and a convenience sample was used. However, this delimitation was intentional because it allowed for control of potential confounding variables. Participants all had to be enrolled exclusively in an online program for at least a year to increase the time in which the source experiences and their sense of academic self-efficacy was adequately affected by the online learning environment, rather than any previous traditional learning environments. Participants were limited to students enrolled in the math education degree program of one university to limit the possibility of differences in programs affecting the source experiences and the grading structure. In this way, the researcher was able to confirm that all students used the same learning management software, the same curriculum and learning materials, the same online engagement requirements and means of communication with classmates and instructors,
and the same grading expectations and structure. So, although these delimitations prevent the results from being generalized beyond the sample, they also provided strength to the findings. Another limitation was the use of email recruitment and an online survey. Due to these two online-only means of recruiting participants and collecting data, it is possible that there was a self-selection recruitment bias amongst the participants, especially since only 17.5% of respondents had a GPA of less than 3.0. It appears students with higher GPAs were more inclined to opt-in to participate in the study. While this provides insight into better performing students, it fails to capture data from underperforming students. It is also possible that some participants did not provide an accurate or exact GPA since it was an anonymous online survey.
Recommendations for Future Research
Further research into the four sources of self-efficacy is recommended. While this study showed two of the four sources statistically significant in relation to academic achievement, this was possibly the only study so far that has investigated all four sources in the context of online learning. One particular area of research that is recommended is into the negative correlation between verbal persuasion and academic achievement. Research into other majors and other universities can confirm whether this negative correlation holds true outside of this study’s sample of online undergraduate math education students from one university in the southwestern United States. Further, studies into the differences between online learning environments and traditional learning environments, as well as the differences between students who choose a fully online program and those who choose a traditional program would provide insight into both the negative correlation to academic achievement found in this study. It is also recommended that exploratory research be conducted with exclusively online students to test Hodges (2008) hypothesis about the existence of additional or alternative sources of self-efficacy for online learners. The results of this study indicated that it is possible that not all four sources affect online students’ academic behavior, resulting in their GPA. It would also be useful to conduct qualitative research with online students to understand more fully the source experiences in the online learning environment, which may yield more specific directions of research for the original four sources of self-efficacy.
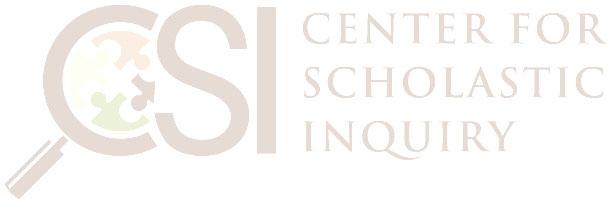
The final recommendation is the creation and implementation of a new, tested selfefficacy and sources of self-efficacy instrument specific to the context of online learning. The instruments used in this study were worded in terms of general education, rather than specifying online learning, which may have impacted the responses from participants. A new instrument would enable researchers to advance scientific knowledge for online education programs and educators.
Author Biographies
Jillian Skelton (Ed.D.) has worked in various areas in higher education and continues to enjoy studying and researching diversity, educational curriculum, leadership, and policy. She lives in the South with her family where she actively works as an editor, teacher, but also enjoys family time and hikes.
Jennifer Miller (Ph.D.) is a teacher and a problem-solver. With a decade of experience in higher education, she is currently Assistant Professor of Psychology at Tougaloo College. She is also a Licensed Professional Counselor with seventeen years of clinical experience. She regularly helps others tackle obstacles to their growth and development, which directly informs her research interests. The focus of her research has been addressing the challenges encountered by college students in the online learning environment, specifically investigating how that environment affects the experiences that inform students’ sense of efficacy and their academic behaviors.
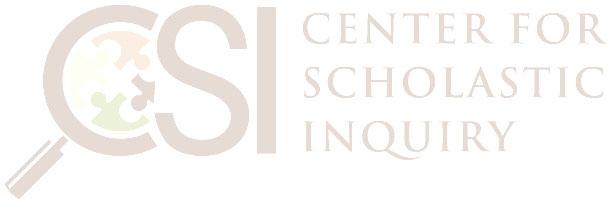
References
Allen, I. E., Seaman, J., Poulin, R., & Straut, T. T. (2016). Online report card: Tracking online education in the United States. Babson Survey Research Group. http://onlinelearningsurvey.com/reports/onlinereportcard.pdf Alqurashi, E. (2016). Self-efficacy in online learning environments: A literature review. Contemporary Issues in Education Research, 9(1), 45-52. https://www.researchgate.net/profile/Emtinan_Alqurashi/publication/296704124_Self-
Efficacy_In_Online_Learning_Environments_A_Literature_Review/links/56f55ab208ae 95e8b6d1d670.pdf Alrushiedat, N., & Olfman, L. (2014). Anchoring for self-efficacy and success: An anchored asynchronous online discussion case. Journal of Information Systems Education, 25, 107116. http://jise.org/ Alyami, M., Melyani, Z., Al Johani, A., Ullah, E., Alyami, H., Sundram, F.,… Henning, M. (2017). The impact of self-esteem, academic self-efficacy and perceived stress on academic performance: A cross-sectional study of Saudi psychology students. European Journal of Educational Sciences (EJES), 4(3), 51-63. doi:10.19044/ejes.v4no3a5 Bandura, A. (1977). Self-efficacy: Toward a unifying theory of behavioral change. Psychological Review, 84, 191-215. doi:10.1037/0033-295X.84.2.191 Bandura, A. (1978). The self system in reciprocal determinism. American Psychologist, 33, 344358. http://psycnet.apa.org/journals/amp/33/4/ Bandura, A. (1986). The explanatory and predictive scope of self-efficacy theory. Journal of Social and Clinical Psychology, 4, 359-373. doi:http://dx.doi.org.lopes.idm.oclc.org/10.1521/jscp.1986.4.3.359 Bandura, A. (2018). Towards a psychology of human agency: Pathways and reflections. Perspectives on Psychological Science, 12, 130-136. doi:10.1177/1745691617699280 Bartimote-Aufflick, K., Bridgeman, A., Walker, R., Sharma, M., & Smith, L. (2015). The study, evaluation, and improvement of university student self-efficacy. Studies in Higher Education, 41, 1918-1942. http://dx.doi.org.lopes.idm.oclc.org/10.1080/03075079.2014.999319 Bradley, R. L., Browne, B. L., & Kelley, H. M. (2017). Examining the influence of self-efficacy and self-regulation in online learning. College Student Journal, 51, 518-530. http://www.projectinnovation.com/college-student-journal.html Chen, X., & Soldner, M. (2013, November). STEM attrition: College students’ paths into and out of STEM fields [PDF file] Washington, D.C.: US Department of Education. Retrieved from https://nces.ed.gov/pubs2014/2014001rev.pdf Cho, M., & Shen, D. (2013). Self-regulation in online learning. Distance Education, 34, 290301. doi:10.1080/01587919.2013.835770
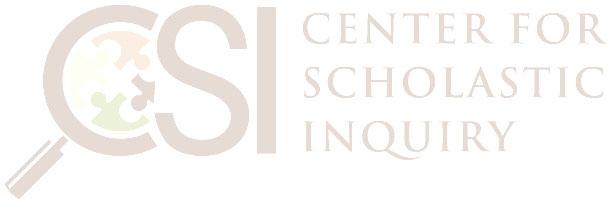
Cochran, J., Campbell, S., Baker, H., & Leeds, E. (2014). The role of student characteristics in predicting retention in online courses. Research in Higher Education, 55(1), 27-48. doi:10.1007/s11162-013-9305-8
Cole, M. T., Shelley, D. J., & Swartz, L. B. (2014). Online instruction, e-learning, and student satisfaction: A three-year study. International Review of Research in Open & Distance Learning, 15(6), 111-131. http://www.irrodl.org/index.php/irrodl/issue/view/65 Dayne, N., Hirabayashi, K., Seli, H., & Reiboldt, W. (2016). The examination of academic selfefficacy and academic help-seeking of higher education students taking an on-campus or online general education course in family and consumer sciences. Journal of Family and Consumer Sciences Education, 33(2), 13-24. http://www.natefacs.org/Pages/v33no2/v33no2Dayne.pdf De Clercq, M. D., Galand, B., Dupont, S., & Frenay, M. (2013). Achievement among first-year university students: An integrated and contextualized approach. European Journal of Psychology Education, 28, 641-662. doi:10.1007/s10212-012-0133-6 Delaval, M., Michinov, N., Le Bohec, O., & Le Henaff, B. (2015). How can students’ academic performance in statistics be improved? Testing the influence of social and temporal-self comparison feedback in web-based training environment. Interactive Learning Environments, 25(1), 35-47. http://dx.doi.org/10.1016/j.compedu.2014.11.002 Diekman, A. B., & Benson-Greenwald, T. M. (2018). Fixing STEM workforce and teacher shortages: How goal congruity can inform individuals and institutions. Policy Insights from the Behavioral and Brain Sciences, 5, 11-18. https://doi.org/10.1177/2372732217747889 Doménech-Betoret, F., Abellán-Roselló, L., & Gómez-Artiga, A. (2017). Self-efficacy, satisfaction, and academic achievement: The mediator role of students' expectancy-value beliefs. Frontiers in Psychology, 8, 1-12. doi:10.3389/fpsyg.2017.01193 Farchi, M., Cohen, A., & Mosek, A. (2014). Developing specific self-efficacy and resilience as first responders among students of social work and stress and trauma studies. Journal of Teaching in Social Work, 34, 129-146. https://doiorg.lopes.idm.oclc.org/10.1080/08841233.2014.894602
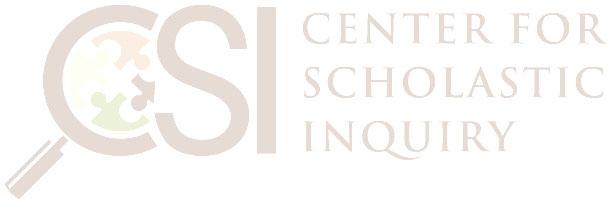
Fong, C., & Krause, J. (2014). Lost confidence and potential: A mixed methods study of underachieving college students' sources of self-efficacy. Social Psychology of Education, 17, 249-268. doi:10.1007/s11218-013-9239-1 Hodges, C. B. (2008). Self-efficacy in the context of online learning environments: A review of the literature and directions for research. Performance Improvement Quarterly, 20(3/4), 7-25. doi:10.1002/piq.20001 Høigaard, R., Kovač, V. B., Overby, N. C., & Haugen, T. (2015). Academic self-efficacy mediates the effects of school psychological climate on academic achievement. School Psychology Quarterly, 30(1), 64-74. doi:10.1037/spq0000056 Jan, S. K. (2015). The relationships between academic self-efficacy, computer self-efficacy, prior experience, and satisfaction with online learning. American Journal of Distance Education, 29(1), 30-40. doi:10.1080/08923647.2015.994366 Kozar, O., Lum, J. F., & Benson, P. (2015). Self-efficacy and vicarious learning in doctoral studies at a distance. Distance Education, 36, 448-454. doi:10.1080/01587919.2015.1081739
Ladyshewsky, R. K. (2013). Instructor presence in online courses and student satisfaction. International Journal for The Scholarship of Teaching & Learning, 7(1), 1-23. http://academics.georgiasouthern.edu/ijsotl/v7n1.html Lawanto, O., Santoso, H. B., Lawanto, K. N., & Goodridge, W. (2014). Self-regulated learning skills and online activities between higher and lower performers on a web-intensive undergraduate engineering course. Journal of Educators Online, 11(3), 1-32. http://www.eric.ed.gov/contentdelivery/servlet/ERICServlet?accno=EJ1033324 Lee, Y., & Choi, J. (2011). A review of online course dropout research: Implications for practice and future research. Education Technology Research Development, 59, 593-618. doi:10.1007/s11423-010-9177-y Lee, Y., Choi, J., & Kim, T. (2013). Discriminating factors between completers of and dropouts from online learning courses. British Journal of Educational Technology, 44, 328-337. doi:10.1111/j.1467-8535.2012.01306.x Lent, R. W., Ireland, G. W., Penn, L. T., Morris, T. R., & Sappington, R. (2017). Sources of selfefficacy and outcome expectations for career exploration and decision-making: A test of
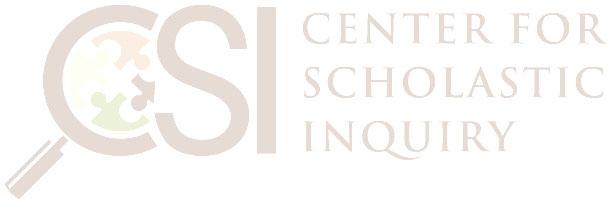
the social cognitive model of career self-management. Journal of Vocational Behavior, 99, 107-118. https://doi.org/10.1016/j.jvb.2017.01.002 Lin, G. (2016). Self-efficacy beliefs and their sources in undergraduate computing disciplines. Journal of Educational Computing Research, 53, 540-561. doi:10.1177/0735633115608440
Loo, C. W., & Choy, J. L. F. (2013). Sources of self-efficacy influencing academic performance of engineering students. American Journal of Educational Research, 1(3), 86-92. doi:10.12691/education-1-3-4
Macaskill, A., & Denovan, A. (2013). Developing autonomous learning in first year university students using perspectives from positive psychology. Students in Higher Education, 38(1), 124-142. https://doi-org.lopes.idm.oclc.org/10.1080/03075079.2011.566325 Pardo, A., Han, F., & Ellis, R. A. (2017). Combining university student self-regulated learning indicators and engagement with online learning events to predict academic performance. IEEE Transactions on Learning Technologies, 10(1), 82-92. doi:10.1109/TLT.2016.2639508
Park, J. Y., Luo, H., & Kim, W. H. (2015, October). Factors affecting students' completion: A study of an online Master's program. In Educational Innovation through Technology (EITT), 2015 International Conference of (pp. 275-278). Wuhan, China: IEEE. doi:10.1109/EITT.2015.33
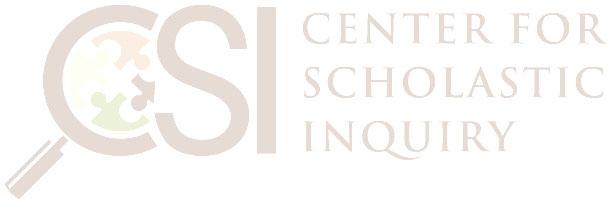
Shen, D., Cho, M., Tsai, C., & Marra, R. (2013). Unpacking online learning experiences: Online learning self-efficacy and learning satisfaction. Internet and Higher Education, 19(10), 10-17. doi:10.1016/j.iheduc.2013.04.001 Trigwell, K., Ashwin, P., & Millan, E. S. (2013). Evoked prior learning experience and approach to learning as predictors of academic achievement. British Journal of Educational Psychology, 83, 363-378. doi:10.1111/j.2044-8279.2012.02066.x Usher, E. L., & Pajares, F. (2006). Sources of academic and self-regulatory efficacy beliefs of entering middle school students. Contemporary Educational Psychology, 31, 125-141. doi:10.1016/j.cedpsych.2005.03.002 Usher, E. L., & Pajares, F. (2009). Sources of self-efficacy in mathematics: A validation study. Contemporary Educational Psychology, 34, 89-101. doi:10.1016/j.cedpsych.2008.09.002
Wang, C., Shannon, D. M., & Ross, M. E. (2013). Students’ characteristics, self-regulated learning, technology self-efficacy, and course outcomes in online learning. Distance Education, 34, 302-323. doi:10.1080/01587919.2013.835779 Wu, E., Lin, W., & Yang, S. C. (2013). An experimental study of cyber face-to-face vs. cyber text-based English tutorial programs for low-achieving university students. Computers & Education, 63, 52-61. http://dx.doi.org/10.1016/j.compedu.2012.11.018 Zelenak, M. S. (2015). Measuring the sources of self-efficacy among secondary school music students. Journal of Research in Music Education, 62, 389-404. doi:10.1177/0022429414555018
Zientek, L. R., Fong, C. J., & Phelps, J. M. (2017). Sources of self-efficacy of community college students enrolled in developmental mathematics. Journal of Further and Higher Education, 1-18. https://doi-org.lopes.idm.oclc.org/10.1080/0309877X.2017.1357071
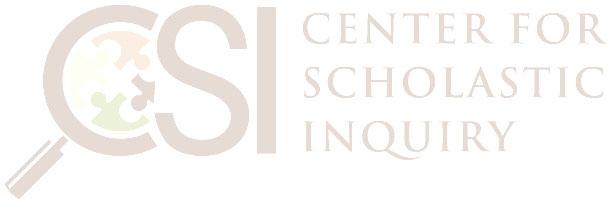
Table 1.
Appendix A
Comparison of Cronbach’s Alpha Scores of PALS and ME, VE, VP, PS scales of SSEMS
Study PALS ME VE VP PS
This Study 0.81 0.80 0.62 0.94 0.90
Validation Study 0.78 0.86 0.68 0.82 0.84
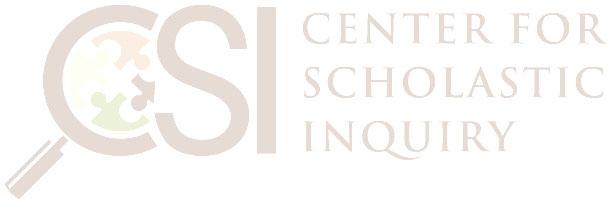
Appendix B
Table 2.
Standard Multiple Regression for RQ2 (H2)
Variable B SEB
β ME .352 .127 .399*
VE -.158 .104 -.183
VP -.300 .097 -.353*
PS -.137 .131 -.127
* p < .025, B = unstandardized regression coefficient; SEB = standard error of the coefficient; β = standardized coefficient (beta)
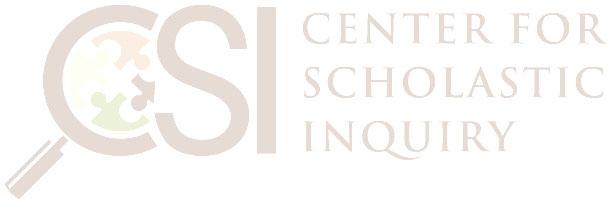