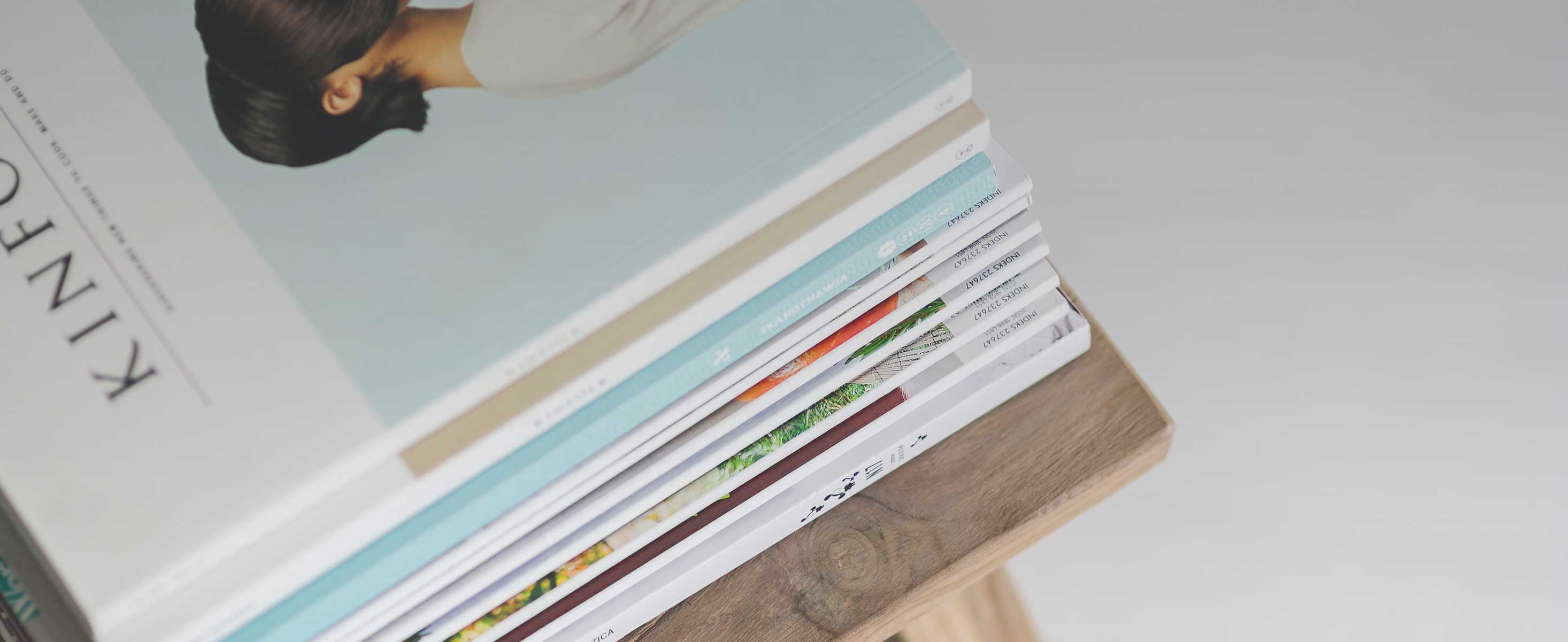
8 minute read
Artificial Intelligence and Machine Learning
first introduced to the space.
In this section, we examine the current state of the various disruptive technologies and use cases to which they are being applied. This is in no way intended to be an exhaustive listing of each vendor or product that might fall under each technology category but is more intended to provide a brief snapshot of the state of play for each and provide a glimpse into how they are being applied and a sense for their future potential.
Advertisement
An excellent definition of AI can be found at IBM’s website1 where AI is described as “enable(ing) computers and machines to mimic the perception, learning, problem-solving, and decision-making capabilities of the human mind.” Machine learning is also defined there as a branch of AI that “focuses on applications that learn from experience and improve their decision-making or predictive accuracy over time.” Deep Learning is a type of ML in which the application teaches itself to perform a task with increasing accuracy without human intervention.
AI and ML use across the commodities complex seems to have expanded rapidly in the last couple of years or so. Often combined with workflow or automation, AI and ML can form a later component of the digitalization activities taking place across the industry or, in an ad hoc mode, to help optimize specific activities, increase efficiencies and reduce costs. In some instances, it is used as a first pass attempt to automate routine tasks or in data reconciliations, leaving exceptions for human intervention. The move to this form of exception management has obvious benefits in time savings and improved process efficiencies, as well as making better use of staff’s time.
Although by no means an exhaustive list, the following use cases are among the most common examples of the deployment of AI in commodities that we are currently aware of.
Short-term market forecasting
In fact, demand and price forecasting is rapidly becoming an overcrowded market with suppliers of AI and ML enhanced short-term market data seemingly cropping up everywhere. These vendors, by using various sensing imagery such as arial and/or satellite images, and bringing together other data including macroeconomic, econometric, production and inventories data, and even social media data, can offer AI enhanced price forecasts across several classes of commodities, ranging from agriculture to electric
1 https://www.ibm.com/cloud/learn/what-is-artificial-intelligence
power. Kyos also makes use of AI when tackling forward curve models and to develop good hourly shapes. Indeed, this is where it started using AI initially.
However, these AI enhanced forecasts do rely on advanced data collection and the availability of key data will vary across the commodities complex. For example, satellite images can be useful for mapping of mines and mine inventories for major metals commodities produced in open-cast mines. A similar approach can also be used in oil and petrochemicals and agricultural production. However, for other commodities where the amounts produced and shipped are small, such tools may not offer much utility, particularly if producers wish to conceal such information by hiding stock or otherwise refusing to cooperate with data collection efforts. Nonetheless, even in markets or commodities with data gathering limitations, artificial intelligence can be used to more efficiently aggregate research, market sentiment, and historical data to arrive at more accurate predictions for commodity prices, estimate inventory drawdowns, forecast vessel movements/port activities and so on.
An example of one company using AI tools to develop improved forecasts is Enverus, the US-based data services company. The firm is utilizing ML in their short to mid-term price forecasting for power markets and has demonstrated the value ML enabled tools by apparently producing more accurate forecasts of market prices than other sources and services during the historic disruptions in the ERCOT power markets in February of 2021.
Optimizing supply chains
Efficient movement of commodities through the supply chain is crucial to profitability and is an area of application for AI that can show a quantifiable return on investment. An artificial intelligence enabled system capable of consuming and optimizing real-time logistics and pricing data can be a tremendous asset for these companies, not only in managing supply chains, but in helping make critical trading decisions. We have seen many examples of the use of AI and ML in this area including but not limited to the following functional processes: • Route optimization taking into consideration transport mode, locations, costs, time, commodity
specifics like need for refrigeration, for example, carbon footprint and so on, • Automation of nominations, balancing and scheduling using management by exception where most transactions are scheduled automatically while a smaller number of more difficult to manage transactions are identified for manual set up or checking, • Gaining insights and greater visibility into supply chains by using imagery of inventory and shipping routes/ports to predict potential issues and/or opportunities, and • Reducing the complexity around supplier
onboarding and collaboration using techniques like anomaly detection, cycle time prediction and nextbest action recommendations.
One good example we have seen of employing such technologies in supply chains is the initiative by Polestar, Vasanda and Carbon Chain to combine environmental, safety and governance (ESG) auditability with supply chains optimization around ESG impacts, including the carbon footprint of the transaction. In this application, the Vasanda component utilizes technologies to examine the ESG impact of commodity production activities, for example, using a variety of different data types including imagery.
Trading
The use of bots to perform automated and algo trading is now quite common particularly in real-time, intra-day power markets. However, this technology has been in use across many other commodities and markets to optimize and automate trading activities, particularly in financially traded commodities. In fact, an entire subcategory of ETRM software has emerged to help automate short-term power and gas trading, particularly in the European markets, specifically the software allows the use of AI/ML to trade automatically and often also to optimize asset dispatch. Vendors like Volue (Likron), Trayport, eZ-nergy, FIS, Brady, NavitaSoft, Igloo and others, are all marketing such solutions. These solutions have also begun to extend into scheduling and data visualization to support intraday trading activities. Other vendors like Enegen and eZ-nergy have gone further and innovated asset dispatch optimization solutions while others plan to do so.
Venus Technology Ventures’ Mistro, created by former traders, is another example of an AI enabled solution in that that uses natural language processing (NLP). The product is designed to understand commonly used trading language terms and, with its ML capabilities, learns quickly what types of deals a trader would typically perform. Admins and/or users can also add specific language used on their trade floor into its dictionary. Once configured, the internal dictionary picks up on keywords and phrases and translates them into something that can be leveraged for other processes and applications.
One advantage of incorporating AI solutions within commodity trading is to help increase productivity and save time through automated deal capture. Traders often use multiple forms of communication—sometimes simultaneously—to execute deals. Whether it’s through messaging platforms, emails or phone calls, it can be difficult for a trader to remember all the details of any specific deal. AI enable NLP and ML systems can help facilitate the process of gathering information from all these sources and take appropriate actions based on this information.
Techniques like NLP and ML can also be used to scan,
listen, and watch across communications, social media, and other sources to help provide early indications of market imbalances to inform trade decisions, and once acted upon, can help ensure more accurate trade capture (many transactions are entered after the fact). In this latter use case, it can also be used to help fill out additional trade data based on an understanding of the trader’s typical activities, reducing time spent entering data.
Other Areas
There are many areas that AI can, and has, been deployed in commodities. These include functions like predictive maintenance, asset management and optimization, inventory management, back office for reconciliations, settlements, invoice matching, and even in relatively obscure operational areas like optimizing fish feeding and improving crop management. SAP demonstrated a solution to reconcile invoices at a recent Commodity Management Forum that utilized AI, for example.
Increasingly, we also see a trend to manage by exception where processes are automated, and AI identifies those where human intervention is needed. Event-driven reporting, where events drive calculations, processes, reporting aided via AI, is also quickly gaining traction.
Risk Edge has been developing AI based products for some time and offers a couple of additional use cases. For one Singapore-based Agri-business that operates in over 70 countries, and supplies food and industrial raw materials to over 22,000 customers worldwide, Risk Edge delivered a web-based Planning & Analysis (FP&A) solution. Their product utilizes advanced ML algorithms to simulate its P&L and predict crop yields based on historical data. In the metals and mining industry, Risk Edge designed and built Journal Entry anomaly detection solution using machine learning algorithms and a statistical filter.
AI and automation have also been proposed and used to help users navigate through multiple systems or screens to complete working on various business processes. This is particularly useful in entities that have deployed multiple different solutions to support their operations and where managing a business process requires complex systems knowledge. As previously mentioned, it can also be used to help automate and manage by exception complex or data voluminous processes and, help to migrate to event-driven solutions whereby a specific event triggers a set of calculations (e.g., valuation based on change in price).
Customer service is another where AI is proving valuable. For example, as early as August 2015, Shell launched an AI assistant for customers of its lubricants business. Shell uses its avatars, Emma and Ethan, to help customers discover products using natural language. The Shell Virtual Assistant functions through an online chat platform through