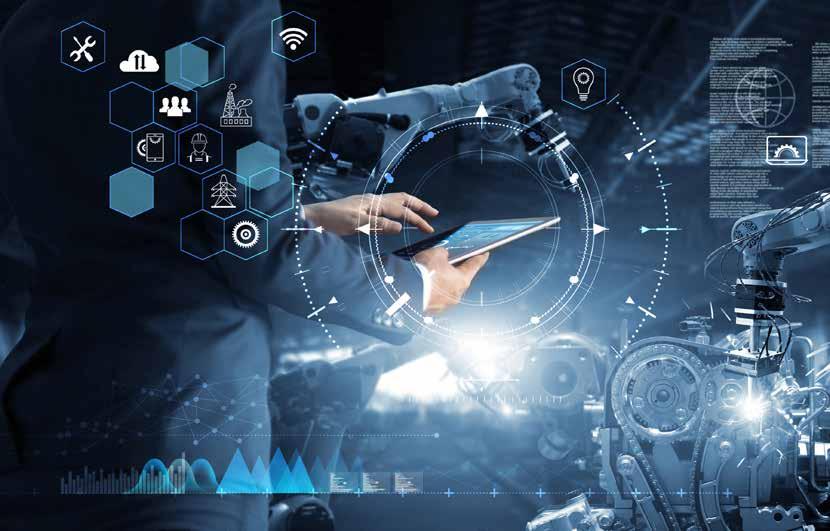
4 minute read
THE EMERGENCE OF MACHINE LEARNING OPERATIONS
from Future Ready
by cxoinsightme
NABIL ABBAS, PRINCIPAL AT BOOZ ALLEN HAMILTON, MENA, SAYS MLOPS HELP ORGANISATIONS SCALE AI FOR THE FUTURE
Over the past few years, Artificial Intelligence [AI] and Machine Learning [ML] have emerged as effective solutions to several global challenges. Amidst COVID-19, which drove businesses and governments to accelerate the digitisation of services, the influence of AI and ML has grown even more significant.
Advertisement
The International Data Corporation [IDC] estimates global spending on AI to grow from $50.1 billion in 2020 to over $110 billion in 2024. AI spend across the Middle East, Turkey and Africa (META) region is expected to grow to $1.2 billion, an increase of 24.7 per cent from last year.
As the world prepares for a postCOVID new normal, the outlook for AI appears more promising than ever. According to a survey by the AI Journal, 72 per cent of business leaders across the globe are positive about the role AI will play in the coming years. Most surveyed executives believe that AI will enable their organisations to achieve significant efficiencies and bring about innovative business models, products, and services, unlocking limitless opportunities.
AI adoption is fast gaining ground in the GCC as governments pursue ambitious innovation and cost reduction targets. For example, the UAE aims to be the world leader in AI by 2031, with AI applications expanding across priority sectors, including healthcare, transportation, education, space, and technology. Saudi Arabia, ranked first in the Arab world and 22nd globally on the global AI Index in 2020, aims to accelerate AI development in critical sectors as means to support its economic diversification agenda. The kingdom is pushing ahead swiftly with the world’s first citizen robot and a $500 billion NEOM smart city, powered by AI. Qatar continues to drive AI adoption in both public and private sectors as part of its National AI Strategy, enabling the Qatar National Vision 2030.
In fact, the AI market across the Middle East and Africa is estimated to exceed $500 million in 2022, almost doubling in size relative to 2019 estimates. The impact of such a rapid
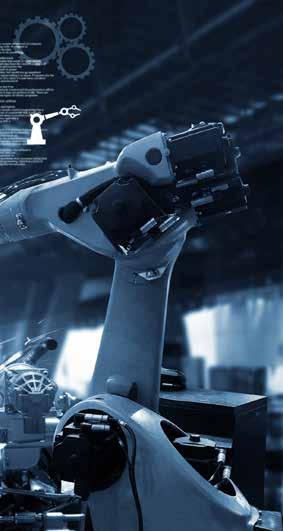
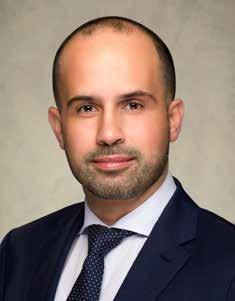
growth rate cannot be ignored. AI, and specifically its most prevalent type / enabler ML, is changing the way organisations approach problemsolving, decision-making, product development, and service provisioning. The capabilities of the technology have evolved exponentially over the past 10 years, thanks in large part to the sophistication and pervasiveness of pattern recognition algorithms, known as neural networks, advances in graphics processing units (GPUs), and decreasing cost of data storage. This progression has transformed computers from tools to trusted partners.
However, much of the power of ML is still isolated in small groups of specialised data scientists using these tools to solve specific problems within their respective organisations. This analyst-led use of ML, generally referred to as Analytical ML, is useful for better informed decision making on clearly defined, static use cases, using controlled data inputs. However, its usefulness in delivering consistent, reliable results is challenged in the real world where use cases are dynamic and data inputs are complex and varying.
An example of the challenges that Analytical ML faces in real life is model drift, which can happen due to changes in data and relationships between inputs and outputs. Model drift leads to rapid degradation of model performance and can impact business processes in a profound way.
MLOps is a standard set of practices for Machine Learning Operations that organisations can use at scale to actualise the power of AI and deliver trusted, machine-led decisions in real time. With it, complementary processes, skillsets, and technologies work together to make AI algorithms effective and useful in real world environments by offering continuous lifecycle management of ML models development, monitoring, governance, and security.
MLOps emulates the proven DevOps and DataOps models, which successfully merged the fields of software development and operations, and data technologies and operations pipelines in organisations. The below figure details how MLOps relates to other fields in the DevOps movement, and the role that each field plays in an organisation’s digital operations.
As deployment of ML models becomes more mainstream, focus will shift from Analytical ML [human-driven decisions] to Operational ML [machinemade decisions], i.e., machine learning operationalised and subsequently updated through MLOps to enable and sustain desired model performance in real life situations.
The Booz Allen report on MLOps aims to distill crucial information about fast-moving innovations to help build technology acumen from vantage points. Key takeaways for organisations include: • Transition from demonstrating
ML-enabled use cases to launching
ML-products managed from the customer perspective and working on continuously improving them • Tailor and implement an integrated
MLOps operating model with clear governance and operating processes, a focused set of meaningful KPIs, and end-to-end automation • Invest in attracting and developing
AI / ML talent, fostering an agile, collaborative culture • Strive for a flexible technology architecture to enable ML product adaptation and improvement at the right speed and scale