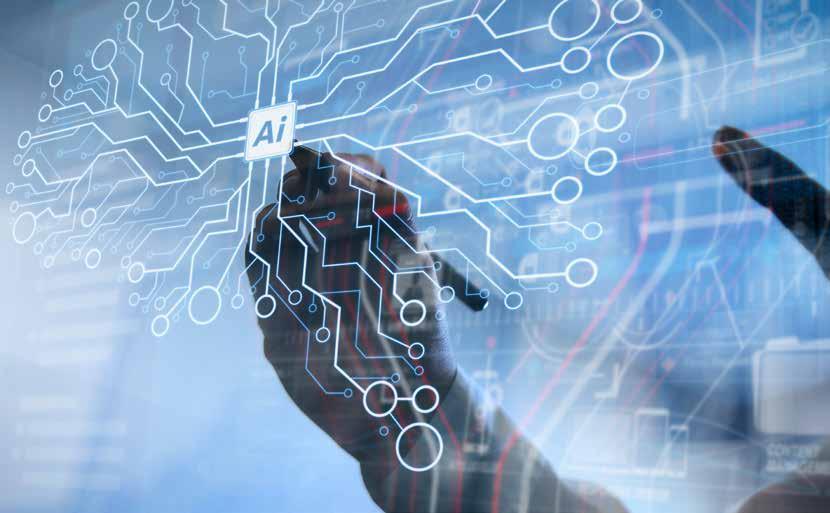
10 minute read
THE BRAINS BEHIND THE DIGITAL TWIN
from OUTLOOK 2022
by cxoinsightme
AI IS THE BRAINS BEHIND THE DIGITAL TWIN, TURNING DATA INTO INSIGHTS THAT CAN TRANSFORM A BUSINESS. THESE ANALYTICS CAN BE BROKEN DOWN INTO DISTINCT CATEGORIES – THE ‘FIVE P’S OF INDUSTRIAL AI – WHICH CAN IMPROVE OPERATIONS IN NEW AND EXCITING WAYS, SAYS JIM CHAPPELL, GLOBAL HEAD OF AI AND ADVANCED ANALYTICS, AVEVA.
Over the past 20 years, artificial intelligence (AI) has significantly transformed industry, taking an organisation’s ability to optimise processes and proactively detect and solve problems to a whole new level.
Advertisement
As a result of the increasing adoption of digital transformation, AI continues to provide benefits across a range of industrial processes. This has resulted in the extensive use of digital twins – virtual representations of physical objects, systems or factories that are created through data gathered from Internet of Things (IoT) devices, advanced computer systems and digital processes.
AI is the brain behind the digital twin. By applying various forms of AI – such as neural networks, computer vision, and machine learning – in different ways, it can create targeted solutions presented in the form of analytics.
Once a digital twin has been put into operation, AI analytics provide insights than can help everything from enhancing operations for safe and profitable processes, through to automating monitoring and control processes to ensure safety and performance.
From an industrial perspective, these can be broken down into five categories: predictive, performance, prescriptive, prognostic and perceptive analytics.
Predictive
Predictive analytics is one of the most common advanced technologies used by industry, utilising big data and machine learning to spot anomalies in processes and assets. This can highlight current inefficiencies, enabling workers to optimise processes, but also warn of future equipment failure days, weeks or even months in advance.
Thanks to this information, business are able to schedule maintenance repairs well in advance of equipment failure, limiting operational risk and saving costs by avoiding unplanned downtime.
Duke Energy, for example, was able to avoid costs of over $34m by detecting a sophisticated turbine problem that would have resulted in a catastrophic failure, potential injury to workers and extensive downtime had it occurred.
Performance
By combining industry and assetspecific algorithms, AI is able to not only identify anomalies that help an organisation discover and rectify faults before they occur, but also optimise processes for improved yield and/or operational efficiency.
Prescriptive
Prescriptive analytics takes things beyond simply alerting you to an issue – it also identifies and recommends the best course of action to resolve it.
It does this through root cause analysis and risk-based decision support, analysing the criticality and urgency of an issue to recommend actions that will optimise efficiency and profitability by minimising downtime and avoiding costly delated.
Wanting to use digital transformation to boost efficiency and sustainability throughout its operations, Ontario Power Generation (OPG) established over 100 predictive and prescriptive operating maintenance models by harnessing data from thousands of sensors throughout its plants.
This allowed the organisation to reduce risk and increase operational efficiency throughout the fleet, as well as saving $400,000 and $200,000 in two separate early warning catches.
Predictive and prescriptive analytics also enabled OPG to reduce annual maintenance hours by 3,000, freeing up stuff to work on higher value corrective tasks.
Prognostic
With prognostic analytics, neural networks, deep- and reinforcement learning enable you to forecast events such as operational performance degradation or the remaining useful life of an asset. This can help organisations to manage risk, maximise profitability and improve sustainability.
Prognostic AI can be used to optimise operations and maintenance strategies, providing risk-based insights into decisions such as whether or not an operation should attempt to run until the next planned maintenance outage or if work needs to take place more urgently. It can also help to identify specific areas for improvement.
Perceptive
Finally, perceptive analytics is all about how intelligent machines interact with their surrounding environments. Advanced technologies such as vision and audio AI, and natural language processing (NLP) are used to automatically detect relationships between sensors and devices.
For example, Schneider Electric used perceptive analytics to not only detect a fault in the main drive chain at its Lexington factory, but also an issue on the motor that runs this chain, which corresponded with the appearance of the former problem.
Through this perceptive analytics, the company was able to avoid factory downtime and related costs and has been able to reconfigure equipment to avoid similar problems occurring in the future.
Benefits of a smart factory
After recently transforming this brownfield site into a smart factory by digitising plantwide operations, Schneider Electric has seen many benefits from its advanced AI and analytics.
This has included optimisation of processes, faster, smarter decision making, improvements in labor productivity, a 6% reduction in unplanned downtime, 26% energy reduction, 78% CO2 reduction and a 20% water use reduction.
This led to the factory being awarded Advanced Lighthouse status from the World Economic Forum and becoming a showcase factory for the business: one that’s being replicated at other Schneider Electric facilities around the world.
“We feel like we’re only scratching the surface on the benefits that can accrue as a result of these new digitisation tools. We’re exploring areas that we’ve never had the opportunity to look at before,” said Mike Labhart, Senior Manager, GSC North America Smart Factory Innovation, Schneider Electric. “This opens the door to new ways of thinking about our facility and will reveal ways to improve productivity and efficiency, not just in Lexington but in other plants around the world.”
Don’t get left behind
An increasing number of industrial companies are following in the footsteps of Schneider Electric to actively leverage the benefits of AI, digital twins and analytics.
In many cases AI is no longer just an option, but rather a requirement to remain competitive, profitable and sustainable. Benefits grow with the addition of new capabilities added by the five Ps of industrial AI, which – amongst other things – help detect and prevent problems faster, better maintain operations, and optimise and enhance processes. As a result, industrial operations continue to improve in new and exciting ways. The opportunities to benefit from the five Ps of industrial AI appear virtually limitless. As AI continues to advance with every passing year, we’re excited to see what the future will hold.

BRIDGING THE GAP
GENG LIN, EVP AND CTO AT F5, SAYS A DISTRIBUTED CLOUD BRINGS ALONG THE CONCEPTS OF CROSS-CLOUD ELASTICITY WITHOUT MASSIVE COST INCREASES, TIME CONSTRAINTS ON PROVISIONING, OR ENVIRONMENTAL VARIANCES.
Despite the broad adoption of multi-cloud strategies in the enterprise, there remains a dearth of effective solutions that address the many challenges faced by organisations executing them.
One such challenge is the secure interconnection of workloads hosted by multiple providers—a problem which magnifies in intensity when more cloud vendors are added.
Of the majority (75%) of organisations deploying apps in multiple clouds, 63% use three or more clouds, according to a Propeller Insights survey. Overall, more than half (56%) find it difficult to manage workloads across different cloud providers, citing challenges with security, reliability, and—generally—connectivity.
Some of this difficulty can be attributed to competing operational models.
Each individual cloud offers services and respective APIs that are unique to the individual cloud provider—and often require customers to conform to different skillsets, policies, and approaches. Every cloud offers a software-defined network experience, but no two clouds offer the same software-defined network experience. This often leads to inconsistent configurations that affect security and performance when these cross-environment differences are not properly considered.
This interconnectivity difficulty is heightened by the introduction of cloud-native, microservices-based applications significantly increasing the number of cross-communication instances. The Propeller survey found that “over 70% of respondents say that security problems are exacerbated in multi-cloud environments by the differing security services between providers (77%), the growing number
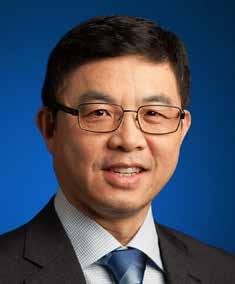
of APIs (75%), and the prevalence of microservices-based apps (72%).”
All this is driving a need—and demand—for a new approach to multicloud networking.
The challenge of multi-cloud networking
Multi-cloud networking unifies two different approaches to simplifying application delivery: • It embraces software-defined internetworking from the bottom-up.
It creates an overlay that abstracts the differences between networking environments and significantly simplifies the challenges of using multiple cloud environments together. The fixed physical infrastructure is used as a capable underlay with a standard crosscloud control plane enabling dynamic virtual networking on top of it. • It extends simple container networking into sophisticated distribution from the top down. While the industry has begun to standardise on container workloads as a de facto application unit, the relatively unsophisticated networking underneath them must be extended toward other environments. This marks the eventual emergence of a distributed cloud to assist in managing application traffic between environments.
The convergence of these two elements has already led to the creation of two layers of abstraction in customer application architectures—Kubernetes to facilitate network workload management and SDN to simplify internetworking. But the way these two approaches currently converge still causes significant customer pain.
Many organisations experience a challenge with the way these technologies require operations to adopt overly granular configurations to obtain a standardised internetworking approach when multiple clouds are involved. The approach taken by one cloud provider— even for extremely simple networking tasks like VLAN management—is distinctly different from the approach taken by another…and both may be completely foreign to the approach taken by the enterprise for its own private cloud efforts.
The way in which networks are provisioned and managed across cloud properties often leads to the need to maintain a staff of experts in the differences between the respective environments just to keep pace with network standardisation.
Distributed cloud as a solution
Adding more than one cloud provider to the mix magnifies the intensity of the problem. Clearly, there are better ways to tackle this issue by moving Kubernetes and SDN closer together, solving environmental differences, and removing the need to be a networking expert to make this all happen. At F5, we call this approach the “distributed cloud.”
Customers generally encounter this problem as their business decisions and application needs are weighed prior to selecting the “best network/cloud” for their service. This decision incorporates a variety of factors, such as cost, ability to launch, speed of deployment, or the need to be in a particular region— whatever factor the customer decides is critical to their application’s success. Rarely are network-side factors or interoperability with other clouds considered in the initial business decision. Unfortunately, this primes new challenges to occur as the application moves along its expected lifespan and other elements of the business make different decisions about cloud use.
At F5, we believe there is nothing inherently incorrect about the decisions made to use cloud technologies that are particularly suitable to business needs—even if it leads to the use of multiple vendors or environments. We do not suggest that our customers should uniquely pursue the benefits of any particular cloud provider, but to instead aim to create commonality across all of them with build-to-scale solutions that are reasonable and within the reach of customers’ network skills, application needs, and business desires. We call our approach the “distributed cloud.”
Our approach is backed by three key beliefs:
1. We understand that the network must support a model of anywhere, anytime, without the loss of quality or customer experience. 2.We assert that any internetworking cloud should be simple, complete, and consistent no matter what underlying cloud our customers might choose. 3.We believe that our customers should be able to get more value through simple, declarative, API-driven unification across control and management planes.
The distributed cloud model considers that the users of our customers’ applications must be served with the highest aspects of quality, performance, and security in near-real time. Our aim is to provide a distributed cloud that brings along the concepts of cross-cloud elasticity without massive cost increases, time constraints on provisioning, or environmental variances.
F5 has created a broad portfolio of solutions to meet these critical moments head on by providing a congruent set of technologies and practices, and we are working hard to extend this to every application in our customers’ architectures. As part of our mission to move towards more Adaptive Applications, we intend to help customers complete these transitions to allow them to move workloads to the most efficient and effective locations, regions, or cost models with ease. Without employing a staff of network wizards for each environment.