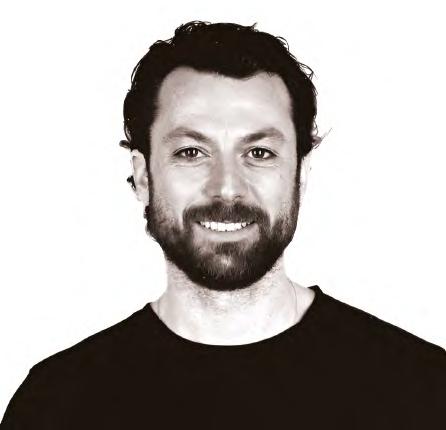
5 minute read
AI VS THE PLANET: THE ENERGY CRISIS BEHIND THE CHATBOT BOOM
AI’S INSATIABLE APPETITE FOR POWER – WHAT’S THE COST OF INTELLIGENCE?
AI’S RAPID GROWTH AND ENERGY DEMANDS
ChatGPT recently hit a massive milestone of 200 million weekly active users – double what it was this time last year. This is incredible! But with rapid growth comes enormous energy demands. On average, ChatGPT alone uses around 453.6 million kilowatt-hours per year, which costs OpenAI about $59.4 million annually. That’s just for answering prompts, not even considering the energy used for model training.
Energy Costs Per Query
Each query to ChatGPT reportedly uses around 0.0029 kilowatt-hours of energy, about ten times what a typical Google search requires. This may sound like a drop in the ocean, but when we scale this to ChatGPT’s 3 billion weekly queries, it consumes over a million kilowatthours daily. To put it into perspective, that’s enough energy to power over 43,000 U.S. homes for a day.
FRANCESCO GADALETA is a seasoned professional in the field of technology, AI and data science. He is the founder of Amethix Technologies, a firm specialising in advanced data and robotics solutions. Francesco also shares his insights and knowledge as the host of the podcast Data Science at Home
His illustrious career includes a significant tenure as the Chief Data Officer at Abe AI, which was later acquired by Envestnet Yodlee Inc. Francesco was a pivotal member of the Advanced Analytics Team at Johnson & Johnson. His professional interests are diverse, spanning applied mathematics, advanced machine learning, computer programming, robotics, and the study of decentralised and distributed systems. Francesco’s expertise spans domains including healthcare, defence, pharma, energy, and finance.
CHARGING ELECTRIC CARS OR RUNNING CHATGPT?
Imagine if we redirected all that energy from ChatGPT’s yearly consumption into fully charging electric vehicles. We’d be able to charge the entire fleet of EVs in the U.S. – all 3.3 million of them – almost twice over. These numbers are staggering, and they underscore how AI’s energy demands are approaching the same scale as some national power grids.
Training Is An Energy Monster
Training these large models is even more intense. When OpenAI trained GPT-4, it consumed about 62 million kilowatt-hours over 100 days, which is almost 50 times the power needed for GPT-3. And now, models like the OpenAI o1 are developed with even more sophisticated reasoning and training techniques, which may increase energy demands further.
Now, here’s where AI reminds me of blockchain. Both involve massive computing power – think about all those GPUs crunching data nonstop. But while blockchain often offers minimal value and bloated energy costs, AI holds genuine promise. As engineers, we need to avoid following the same energy-intensive pitfalls of blockchain tech. AI has the potential to be a meaningful force for good, but we must carefully balance its benefits with environmental costs.
FUTURE DIRECTIONS
EFFICIENCY-FOCUSED ALGORITHMS
AI algorithms today, especially deep learning models, consume massive amounts of computational power, particularly during training and inference. For AI to grow sustainably, we need algorithms optimised for efficiency from the outset.
KEY APPROACHES:
Pruning and Quantisation:
Techniques like model pruning and weight quantisation reduce the size and complexity of neural networks, making them faster and less powerhungry without significant accuracy loss. This could be a deep dive into the maths and techniques that make these approaches feasible.
Sparsity in Neural Networks:
Sparse models, where many of the network connections are zeroed out, offer a compelling way to lower computational costs.
Self-Supervised Learning:
Reducing the need for extensive labelled data and resources can lower the cost associated with data curation and processing, directly reducing the energy footprint of AI systems.
HARDWARE OPTIMISATION
Traditional processors, including GPUs, aren’t designed with AIspecific tasks in mind. Specialised hardware designed explicitly for AI tasks, like inference and model training, can drastically improve energy efficiency.
KEY INNOVATIONS:
ASICs and TPUs : Companies like Google have developed AIspecific processors (e.g., TPUs) that are highly efficient for machine learning.
Low-Power Edge Devices:
Specialised edge devices and microcontrollers, like NVIDIA’s Jetson Nano or Coral’s Edge TPU, are bringing powerful AI processing to small, low-power devices for local processing, dramatically reducing energy use.
Neuromorphic Chips: This emerging technology, inspired by the human brain, simulates neural activity with lower energy demands. Explain how these could redefine AI’s approach to resource constraints.
DECENTRALISED LEARNING
Training and inference on centralised data centres not only increases energy costs but also poses security and latency challenges. By decentralising AI processes, we can reduce energy costs and bring computation closer to the data source.
KEY METHODS:
Federated Learning: A distributed learning approach that trains AI models on edge devices, allowing data to stay local and reducing the need for extensive data transfers. This can save energy and enhance privacy.
Edge Computing with Local Inference: Moving AI computations to edge devices means models perform tasks on user devices rather than in centralised servers. This approach uses local resources, lowering the cost and energy required for data transmission.
Distributed Architectures: Peer-to-peer training architectures or grid computing setups could spread the computational load, using only nearby nodes as needed and potentially leveraging idle computing power.
CONCLUSION
Looking to the future, the energy cost of AI can’t continue unchecked. If we want sustainable growth, here are three directions for AI development that make sense:
Efficiency-Focused Algorithms: AI research must prioritise models that consume less energy, especially during inference.
Hardware Optimisation: We need chips explicitly designed for AI tasks that optimise energy use, like more efficient GPUs or specialised AI hardware.
Decentralised Learning: Moving away from giant centralised models to decentralised, smaller models that handle data locally could dramatically reduce energy consumption.