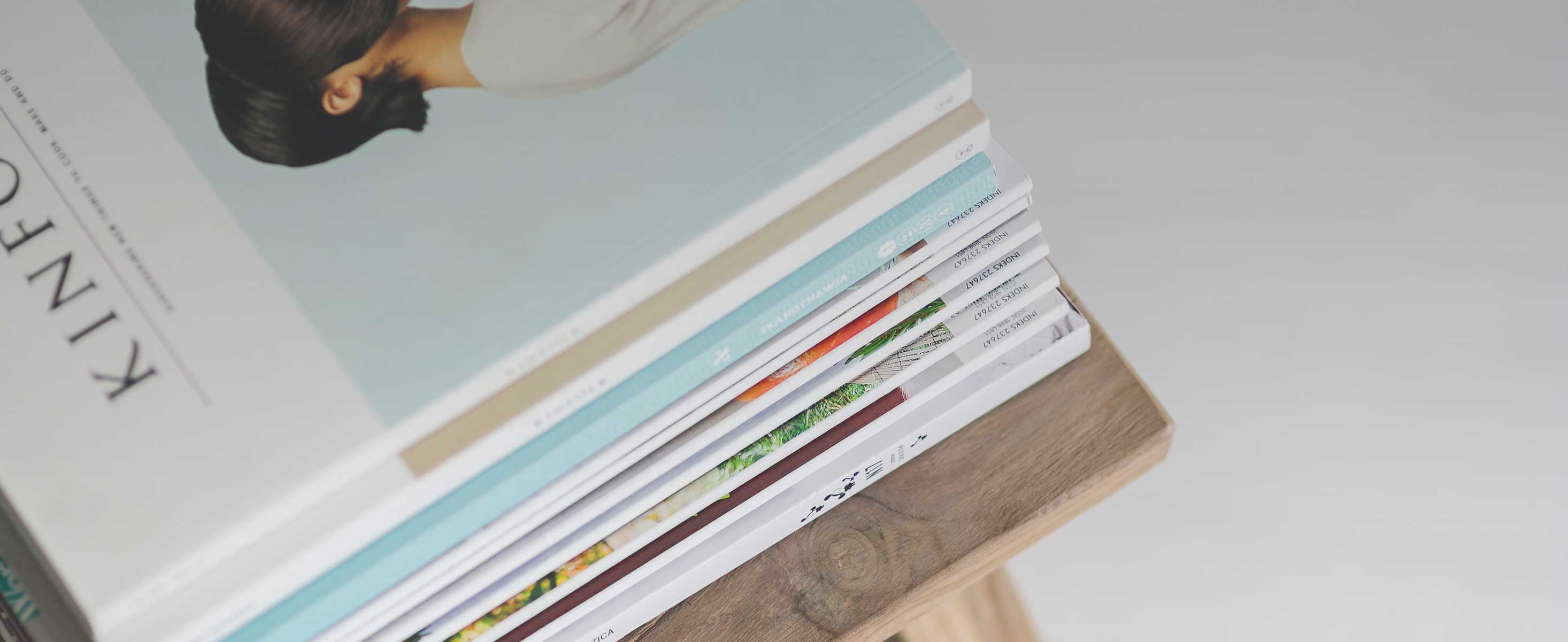
9 minute read
Managing urban environments with Digital Twin
detects energy needs and intervenes actively, avoiding unnecessary consumption. In case solar energy reserves are not adequate, the AI guided home will be able to switch off unnecessary devices automatically, or to reduce their consumption when possible, sending appropriate warning messages to the householder. Present and future applications of AI are being continuously tested, mainly in the field of Domestic Domotics, and their evolution will certainly be greatly accelerated by the forthcoming spread of 5G telecommunication wireless technology, able to transfer an unprecedented flow of information and data to predictive systems, largely superior than the ones available at present.
Managing urban environments with Digital Twin
At the beginning of the 4th Industrial Revolution, the rise of digitalization, innovative technologies, and materials as well as the emergence of new construction techniques, transformed the way that infrastructures, real estate and building assets can be planned, designed, constructed, and made operational to create a more attractive, energyefficient, comfortable, affordable, safe, and sustainable built environment. Developments in digital design, Artificial Intelligence, robotics, nanotechnology, and additive manufacturing, have finally started to move the construction industry – traditionally reluctant to innovate and slow to adopt new technologies – towards a new era. In particular, the architecture, engineering and construction (AEC) sector is facing a rapid and considerable transformation given the diffusion of BIM (Building Information Modelling), which is consistently
modifying construction workflows. In such an innovative framework, even the innovations related to the Internet of Things (IoT) and Smart Cities in general are changing urban planning and development dramatically, improving urban ‘intelligence’ and sustainability51 . The goal of digitization within the AEC sector is to build digital processes and ecosystems based on three-dimensional digital models, combining physical objects and components, and monitoring their interactions with reality, following the Digital Twin Model approach. According to Sue Weeks, a digital Twin could be defined as the transposition of a real world object to a virtual/digital representation, aimed to evaluate its functionality and perfomance52 . Digital Twins (DT) for building systems are designed as three-dimensional real-time databases, where data are encompassed within object-oriented models representing building components as well as their qualities and other useful information, aimed at simulating activities and real-time management of processes. The concept of DT implemented at urban environment scale gives rise to the concept of City Digital Twin (CDT), a virtual model which upgrades the single building approach to a higher and more complex level. The Digital Twin City is essentially able to improve and enrich the knowledge received through input data and signals coming from
51 Perera C., Zaslavsky A., Christen P., Georgakopoulos D., 2014, Sensing as a service model for smart cities supported by the Internet of Things. Transactions on Emerging Telecommunications Technologies, 25, pp. 81-93. 52 Weekes S. 2019, The rise of digital twins in smart cities, SmartCitiesWorld, on line platform (www.smartcitiesworld.net)
the continuous data flow obtained by the continuous monitoring of urban systems, namely by smart sensors, IoT, smart metering, personal mobile data and information. DT is progressively developing self-learning and predictive capabilities through Machine Learning, Artificial Intelligence, Deep Learning and Neural Networks53 . Therefore, the development of a CDT usually moves from a Building Information Model (BIM), a threedimensional database that communicates with data and sensors, acquiring some levels of self-learning using Artificial Intelligence algorithms, progressively developing predictive capabilities and allowing some level of autonomous decisions and actions based on the analyses performed54 . The Digital Twin of an urban environment can therefore be a key tool for organized data collection, storage, analysis and visualization for the daily management of urban life, and for the integrated use of Geographic Information Systems (GIS) and Building Information Modelling (BIM), expanding the information data management and processing from single buildings to territorial scale. Since the building sector remains the largest end-energy
53 In the field of machine learning, an artificial neural network (abbreviated as ANN or also as NN) is a computational model composed of artificial “neurons” inspired by the simplification of a biological neural network. Such mathematical models are used to attempt to solve artificial intelligence engineering problems such as those of technological fields (in electronics, computer science, simulation, and other disciplines). 54 ISO20944-1:2013: Information technology - Metadata Registries Interoperability and Bindings (MDR-IB) - Part 1: Framework, common vocabulary, and common provisions for conformance. Standard, International Organization for Standardization, Geneva.
consumer (up to 40% globally), all the Information and Communication Technologies (ICT) mentioned have been identified as playing an important role in reducing the energy intensity and increasing the energy efficiency of the building stock. The amount of information to be processed must be managed with ICT methodologies and solutions through an integration process that requires a “wide-scale” vision and design method: from Satellite-Land scale to the “vertical” BIM. The main approach is that of management continuity between building related representation and information and, thanks to Artificial Intelligence (AI), building systems are becoming able to integrate autonomously the data flow derived from domestic IoT devices and occupant behavior for improving performance and environmental efficiency. When AI is integrated with building systems and IoT devices, it has the potential to improve occupant experience, increase operational efficiency and optimize space and asset utilization. A vast array of information from digital devices furnishes insights about the operativity, use and conditions of a building’s infrastructure, physical internal microclimate, external climate, water, and energy use, providing dwellers with a better living experience and greater satisfaction. IoT and platforms embedded with Artificial Intelligence and machine learning make it possible to develop innovative new services for engaging with building occupants55. These systems have the potential to radically
55 Guillemin P., Friess, P. 2009, Internet of things strategic research
reduce costs through automation and optimization of operations. By taking advantage of powerful analytics and Artificial Intelligence, for example, building owners can significantly cut energy consumption and achieve ambitious cost-saving targets. After equipment performance, information is collected through sensors and smart meters, a library of benchmark data is applied, analytics are performed, and potential operational improvements are identified. Advanced analytics tools can be also used to prevent energy waste by isolating inefficient energy use. Sensor-controlled systems can monitor water distribution and use, cognitive maintenance systems can help preserve the proper functioning of critical building equipment and assets, anticipating possible failures and guiding timely maintenance interventions. A comprehensive building optimization system leverages all aspects of building and facility management. Taking these monitoring activities one step further, building equipment data collected from IoT sensors tagged by location or asset type and associated with business rules can trigger algorithms to not only detect but also predict and respond to anomalies. For instance, data transmitted from connected assets, such as boilers, pumps, air conditioning systems and elevators, are analyzed and enriched to identify anomalies, such as equipment operating outside of normal registered parameters, standardized in order to be evaluated not only
roadmap. The Cluster of European Research Projects, Technical report, European Commission - Information Society and Media DG, Brussels.
on the basis of the operative conditions provided by the manufacturer when newly installed, but also after some years of use. Devices automatically receive instructions to take corrective actions, and building AI memorizes the intervention results in order to improve the accuracy of detection and resolution for future occurrences. In the meantime, these optimized ecosystems of building technologies identify further opportunities for efficiency controls through predictive maintenance56, and building DT identifies possible inner causes, not necessarily depending on the malfunctioning of a single device, thus helping to improve the entire interconnected system, and sending appropriate communications to human maintenance teams, to the building administrator and to the landlord. AI is also able to capture data from dayby-day building operations, enabling new levels of realtime automation, giving to the buildings the capacity to “think,” engage and learn, autonomously monitoring and predicting self-maintenance needs57 . The integration of cognitive analytic sensors can also significantly improve dwellers’ living experience. IoT sensors are constantly monitoring movements, indoor air quality, temperature, and other human related parameters58, switching lights on and off, adjusting
56 Anvari-Moghaddam A., Monsef H., Rahimi-Kian A. 2015, Optimal Smart Home Energy Management Considering Energy Saving and a Comfortable Lifestyle, «IEEE Trans. Smart Grid», vol. 6, n. 1, pp. 324–332. 57 Vastamäki R., Sinkkonen I., Leinonen C. 2005, A Behavioural Model of Temperature Controller Usage and Energy Saving, «Personal and Ubiquitous Computing», pp. 250-259. 58 ISO16678:2014: Guidelines for interoperable object identification and related authentication systems to deter counterfeiting and illicit trade.
restroom water flow, obeying voice commands, learning and adapting to the tone of voice of occupants. Even breaths are monitored for carbon dioxide concentration and corrected with appropriate airflow adjustments. This kind of approach to problem-solving is related to real-time simulation modeling, which aims at reproducing the behavior of a non-linear dynamic system in a virtual environment. It serves as a digital testbed to evaluate ex-ante different strategies over a simulated timehorizon. Digital Twins can therefore involve Artificial Intelligence but also Machine learning (ML), enabling the DT system to learn from data rather than through programming on purpose. ML is a form of self-learning data model, comparable to children’s learning, driven by progressive “experience”. Data-models are incrementally refined, through data gathering, supplying “machine-learning” algorithms, where the quality referred to the algorithm is a direct function of data quality59 . Machine Learning (ML) techniques are essentially divided into two main categories: Supervised learning or Unsupervised learning. Given a sample of data and a desired output, Supervised Learning is based on the so called ‘ground truth’60 and its goal is learning on the basis of the function that best estimate expected outputs, as determined from clearly known inputs, using acceptable
Standard, International Organization for Standardization, Geneva. 59 Martínez-Prieto M. A., Cuesta C. E., Arias M., Fernández J. D. 2015, The Solid Architecture for Real-time Management of Big Semantic Data, «Future Generation Computer System», 47, pp. 62-79. 60 The term ‘Ground truthing’ in Machine Learning refers to the process of gathering a proper provable ideal expected result.
approximation and statistical experience. On the other hand, Unsupervised Learning does not have clearly identified outputs (tagged data), and the algorithm in this case self organizes data, with the aim of guiding the input data charge (non-tagged data), according to a selfconstructed internal classification. ML in fact is typically related to classification of data (input and output tagged data) or regression of data (relating input to a continuous output)61. In both regression and classification, the goal of machine learning is to find specific relationships in the input data that make it possible to produce correct outputs, where model complexity refers to the complexity of the learning function; the appropriate level of algorithm model complexity is defined by the nature/structure of the data acquired during the machine’s training. In fact, a high-complexity model, if used on a small/limited quantity of data, will overload the function (overfitting)62 , reducing the algorithm’s capacity to generalize other self-elaborated data. In this case the learning process can produce training data, without acquiring a structure in the data able to lead to a meaningful output.
61 All algorithms for Machine learning are divided into two families: algorithms of classification (AoC) and algorithms of regression (AoR). AoC essentially classify the data; AoR interpolate data. This implies that the output of a Classification model results in a class, and the output of a Regression model gives a number. 62 Overfitting happens when a model learns too much detail in the training data. This kind of “noise” negatively impacts the performance of the model on new data, since noise or random fluctuation confuse the learning process and the Machine is unable to distinguish relevant from non-relevant data, negatively impacting on the algorithms’ ability to generalize through regression.