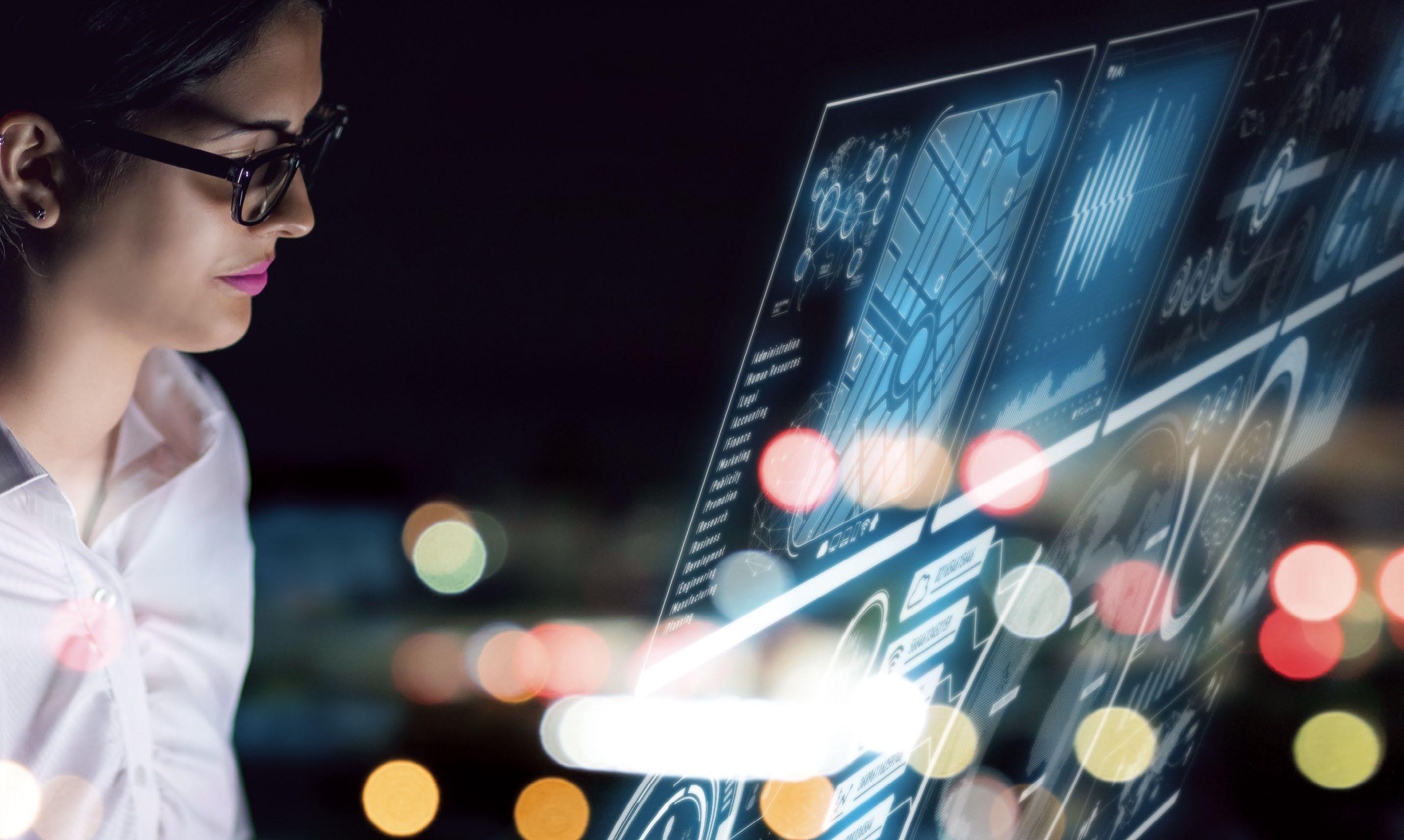
6 minute read
TECHNOLOGY Supercharging Organizational Decision Making with Artificial Intelligence
BY DAN HIGGINS
The far-reaching effects of economic volatility, social and political upheaval, and global health emergencies are being felt by organizational leaders under intensified pressure to produce results. But even under ideal circumstances, leaders know that making quick and confident decisions can be extremely difficult, particularly when they do not have access to trustworthy data. In fact, recent data from Gartner uncovered that 65 percent of leaders feel they are forced to make more complex decisions today compared to two years ago. Moreover, the same survey found that over half (53 percent) of those surveyed noted a greater need to justify or explain their decisions, highlighting a clear gap between hastened automation and a thorough comprehension of what is being automated and why.
Individuals and businesses are under pressure to make fast, consistent, and fact-based decisions, so called ‘decision intelligence’. And it’s here where we often see enterprises deploy technologies such as artificial intelligence (AI) and machine learning (ML) to augment their decision-making abilities to do just this. However, one of the most significant hurdles customers face is that organizations frequently attempt to deploy these technologies without fully comprehending the importance of context, or in other words, without a clear idea of the bigger picture driving what data is collected, examined, and how it is applied for decision making. Without context, AI predictions and decisions lack strength and dependability, potentially ushering in a range of long-term automation challenges and other setbacks.
Enter entity resolution — the process of parsing, cleaning, and standardizing data by using advanced AI and machine learning models to accurately identify entities. This process connects records related to each entity, creates a list of attributes for each entity, and generates labelled links between entities and source records, and is significantly more efficient and effective than the conventional record-to-record matching method used by MDM systems.
A single source of truth: Leveraging quality data for AI business value
When it comes to AI and ML, the data you use is everything. That’s why data scientists are laser-focused on using reliable and transparent data to make the best algorithms possible. For instance, if you build a classifier to distinguish between photos of a raven and a crow, data scientists would ideally like an input image dataset certified by an ornithologist. If they are unable to source this, then the obvious next best place to find this might be online. But this is where the risks of input errors and misclassification begin to emerge.
Another challenge is presented by inconsistent data entry, wherein a single entity may be referenced by varying names. For instance, take actor and comedian Jim Carrey. Hi name may appear in its full form, James Eugene Carrey, just as it may be listed as James Carrey, Mr. Carrey, or in a like manner. This also holds true for companies, which can be referred to by their full legal name or an abbreviated form.
It is imperative that the algorithm can recognize and learn from a multitude of diverse names and formats. The successful operation of the algorithm hinges upon its ability to recognize and learn from a broad spectrum of names and formats. To make accurate distinctions between names and said formats, the algorithm must possess the capability to learn from a complete range of them.
By harnessing powerful AI and machine learning algorithms, entity resolution efficiently processes, structures, and divides data to identify like entities in a comprehensive approach. In contrast, the standard record-torecord matching methodology that most MDM systems use is quite outdated. But with entity resolution, organizations can now bring in new entity nodes that act as essential linkages and effectively connect real-world data in a way that was previously impossible. Utilizing this technique is crucial to decision intelligence. It results in improved accuracy and efficiency when connecting data. It also allows for the matching of valuable external data sources, such as corporate registry information, that previously presented difficulties when trying to establish reliable links.
Data-driven transformation to accelerate the power of your business
Quantexa’s new research found that just 42 percent of IT decision makers in Canada, the US, and the UK have faith in their organization’s data. The study also discovered that one in eight customer records in the US is a duplicate, which means that tons of organizations struggle to differentiate between customers like me, J.E Carrey, Jim Carrey, and James Eugene Carrey.
When it comes to digital transformation, data is a musthave ingredient for improving operational efficiencies, customer value, and generating fresh revenue streams. Yet, despite the wealth of available data, organizations often struggle to derive actionable insights from it. This can make data both an asset and a considerable challenge when it comes to achieving transformational goals.
Organizations operating across industries like banking and financial services may encounter difficulties because of a data ‘context gap’, which can leave them exposed to vulnerabilities. The root of this problem can be traced back to the presence of identical datasets pertaining to the same customer that are scattered across multiple CRM and management tools and systems. Although it may be a minor duplication error — such as variations in name, address changes, or multiple phone numbers its impact on insights can be significant. For instance, if a customer’s name is spelled with just one letter out of place on one system, but not another, there is a strong chance that the organization will consider these two entries to be two unique entities, even though they refer to the same person. Such occurrences are typical of siloed data. This is in part why context is so crucial when it comes to analysis; without it, you’re essentially flying blind, which can be a major obstacle to effective decision-making.
To take your understanding of your customers to the next level, it is going to take more than just manual deduplication efforts. Manual data management isn’t just slow and laborious, but also extremely prone to human error. Although methods like Master Data Management (MDM) have been around for a while, they’ve typically fallen short in detecting these “missing links” and connecting them to an individual customer.
Clean data, clear results
Augmented and automated data-driven decision-making has become the gold standard in today’s business environment, and for good reason. Incorporating data into the decision-making process can provide valuable insights that lead to better, more successful outcomes for organizations. But that doesn’t mean that there isn’t a dark side to this rising as well — to break down potential data silos, companies must parse through a bog of duplicate redundant data, which can have a ripple effect on decisionmaking efficiency and accuracy.
This can lead to wasted resources across data, IT, and business teams creating bottle necks in a company’s ability to quickly identify risks and provide top-notch customer service. That is why achieving decision intelligence all comes down to the strong foundation of your data, making it imperative that companies establish effective and efficient data practices that protect valuable assets, optimize resources, and identify opportunities for growth.
DAN HIGGINS is the Chief Product Officer at Quantexa, a leading pioneer in Contextual Decision Intelligence.