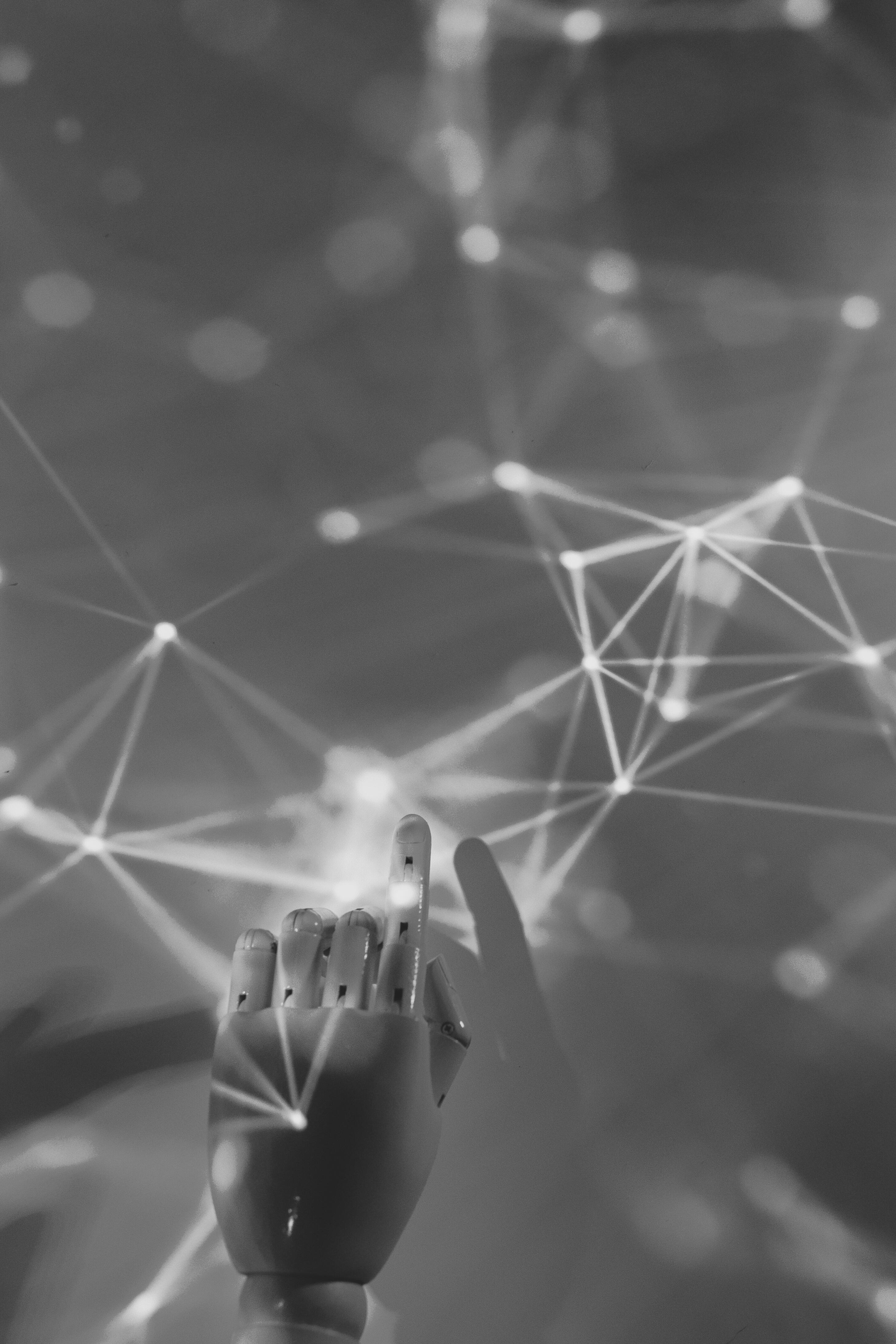
81 minute read
Regulating Government Use of Artificial Intelligence (p
Advertisement
PAGE 155
Benjamin Nober Northwestern University April 30, 2021
Abstract
Over the last decade, artificial intelligence (AI) has become a mainstay in the everyday
lives of Americans. This thesis seeks to better understand what conditions can foster greater
regulation of government use of AI systems. The present government reliance on private politics
and the relatively low level of traditional government regulation of AI poses strong technical and
ethical concerns affecting the liberties of Americans. The legislative and electoral influences
involved are critical to answer questions surrounding regulation of AI. How do legislator
preferences, interest groups, and public pressure affect whether lawmakers are incentivized to
engage in proactive regulation or to continue the current path of narrow, reactive measures? I
argue that the pace of technological change and the place of government as the direct consumer
of AI provide sizable regulatory hurdles. As a result, I hypothesize on the importance of the role
of outside influences in driving regulation of government use of AI systems.
Acknowledgements
Thank you to Richard Joseph for introducing me to the world of academic research, to
Prof. Dan Linna and Prof. John Villasenor who sparked my interest in governance and
technology, and to Prof. Laurel Harbridge-Yong for guiding me through the research and writing
process.
I. Introduction
Over the last decade, artificial intelligence (AI) has become a mainstay in the everyday
lives of Americans. Supported by increasingly cheap computational power, constantly improving
algorithms, and a vast pipeline of data now available, this technology continues to provide a
platform for those looking to innovate. Alongside this innovation, widespread concerns have
1
grown over the ethical and technological questions stemming from the technology’s broad
implementation across sectors. A major strategy by legislators at all levels of government has
been to avoid significant regulation and instead opt for private solutions. With low levels of
regulation in place concerning the technology’s use, high levels of access have led to rapid
expansion across many domains. These extend into both the public and private sectors.
Algorithms determine what news is read, increase access to legal and health services,
help law enforcement officials identify suspects, and provide countless other services.
Accompanying these advancements are new challenges created by the implementation of AI.
Ethical concerns exist concerning levels of surveillance and fairness of decision-making.
Technologies powered by AI offer an unprecedented amount of control that, when so desired,
can be used nefariously. These ethical considerations are compounded by a host of questions on
the technical nature of AI systems. The consequences of employing underdeveloped and
underregulated technologies are real. Reports of arrests made on faulty grounds due to
algorithmic failures have trickled out around the nation. Cost-saving algorithms that replace
2
1 UW Video, Kate Crawford | AI Now: Social and Political Questions for Artificial Intelligence, 2018, https://www.youtube.com/watch?v=a2IT7gWBfaE&ab _ channel=UWVideo. 2 Kashmir Hill,
“WrongfullyAccused by anAlgorithm, ” The New York Times, June 24, 2020, sec. Technology, https://www.nytimes.com/2020/06/24/technology/facial-recognition-arrest.html.
present practices in different local contexts can be dangerous. As many algorithms are created to
provide efficiency and cut costs, local contexts are often overlooked leading to wide
implementations of algorithms that worsen or ignore local conditions in some areas.3
This thesis focuses on specific uses of AI systems where the government is a direct
consumer. It seeks to better understand the present reliance on private politics, the relatively low
level of traditional government regulation of AI, and the prospects for greater regulation. Why do
legislators opt for private governance of AI? How has the initial reliance on private politics
affected subsequent governing strategies? What conditions can foster greater regulation? The
legislative and electoral influences involved are critical to answering these questions and
understanding the governing process surrounding AI. How do legislator preferences, interest
groups, and public pressure affect whether lawmakers are incentivized to engage in proactive
regulation or to continue the current path of narrow, reactive measures?
To address these questions on regulating government use of AI, I argue that there are two
main reasons that regulation is so limited. First, the pace of technological change produces a
difficult regulatory hurdle. Regulations that address yesterday’s problem may be insufficient to
address tomorrow’s challenges. Second, the government is the direct consumer of AI, which may
lead their incentives to align with those of technology companies and reduce interest in
regulation. As a result, I hypothesize that there will only be successful regulation of the use of AI
by the government when outside groups (e.g., citizens-focused interest groups) are very active on
the issue and raise awareness through public exposure. Importantly, for citizens’ groups, they
cannot use boycotts or buycotts because boycotting the government is infeasible. However, they
3 YanniAlexander Loukissas, All Data Are Local: Thinking Critically in a Data-Driven Society, 1st ed. (The MIT Press, 2019), https://mitpress.mit.edu/books/all-data-are-local.
can focus on publicity campaigns to increase the salience of the issue in the public, leading to
greater pressure from the public on elected officials.
The task of governing AI poses a difficult yet essential balancing act for legislators. On
one side is increased access to services and corporate interest in development and innovation in a
global technological race. Providing more people with smarter services and allocating resources
more efficiently could reshape aspects of governance. On the other side are public concerns as to
the intrusive nature of these technologies, infringements on Constitutional rights, and the
implications of technological shortcomings. Merit exists on both sides, and compromises to
achieve responsible use of the technology requires government action. The strategy by legislators
at all levels of government to date has largely been to avoid significant regulation and instead opt
for private solutions. The findings of this paper have implications as to what internal and external
influences on the decision-making process are important in bringing about regulation on
government use of AI.
This paper begins with a technical overview explaining a short history of AI, how it
works today, present applications of the technology, and the concerns stemming from its use.
Next, the role of interest groups in influencing legislative outcomes is explored through a review
of political science literature on public and private governance. The following section lays out
the hypothesis, which applies political science literature to the unique uses of the technology, and
the research design for the case study that follows. A case study on police use of facial
recognition technology in two US cities points to connections between regulation of government
use of AI and the role of interest groups in pushing such legislation. The final section concludes.
II. Background
This section introduces the concepts and uses of artificial intelligence, machine learning,
and deep learning before exploring the implications of their present applications. The growing
concerns over inequality and bias stemming from the technologies highlight the need for a
conversation on regulation.
A Brief Overview on Artificial Intelligence
So, what is AI? The term was first coined in 1956 and can be generally thought of as the
class of technologies that rely in some way on nonhuman decision-making. Three types of AI
4
exist in theory. The first, narrow or weak artificial intelligence, describes AI technologies able to
perform singular, specific tasks. General artificial intelligence is a class of technologies able to
think “on par with human abilities,” and superhuman AI can outperform human capabilities.5
Only narrow AI technologies have (to date) been achieved.
Fluctuating in and out of popularity in both popular culture and applied science since the
idea was first imagined, AI today has become a mainstay with the introduction of machine
learning (ML). The most popular form of the technology today, ML works by analyzing large
sets of data and making predictions based off of connections found within the data. The
technology is the ability to find complex patterns that humans may miss. In general, developers
design a program, calibrate the program on a chosen set of training data already marked with
desired outcomes, and release the trained program. For example, developing an algorithm with
the goal of recognizing handwritten numbers and translating those into a computer through
4 John Villasenor andAlicia Solow-Niederman, HoldingAlgorithmsAccountable,April 23, 2020, https://www.youtube.com/watch?v=EcF-FtXx _q8&ab _ channel=UCLASchoolofLaw. 5 Brodie O’Carroll, “WhatAre the 3 Types ofAI?AGuide to Narrow, General, and SuperArtificial Intelligence, ” Codebots, October 24, 2017, https://codebots.com/artificial-intelligence/the-3-types-of-ai-is-the-third-even-possible.
digital capture would first be written by a developer, next be trained using the National Institute
of Standards and Technology’s (NIST) public Modified National Institute of Standards and
Technology dataset containing 60,000 examples of handwritten numbers, and finally be tested for
accuracy on test data before being released to the public. Importantly, the algorithm would
continue to improve itself even after release.
At their core, ML systems operate on a variety of types of algorithms depending on what
types of connections the program intends to discover. The most popular and powerful ML
algorithm today is deep learning, which passes data through weighted connections in a series of
interconnected neural networks.6 Essentially, training in deep learning means having the weight,
or importance, of each piece of data at each layer of the algorithmic process both updated and
assigned so that the algorithm can make predictions. An important note to take away from deep
learning is that more computing power can mean better performance by the algorithm.
The amount of computational power in machines today is aweing. Moore’s Law, a
commonly referenced rule of thumb, predicts that it takes only about eighteen months for the raw
computational power of a computer, number of transistors, to double.7 Coined in 1965, this rule
of thumb has been closely correlated to the actual trend of growth in computational power.
Almost sixty years of exponential growth means that computers today are orders of magnitude
stronger than those of past decades.
Present Applications
AI technologies continue to seep into more aspects of the daily lives of people
6 Larry Hardesty,
“Explained: Neural Networks, ” MIT News | Massachusetts Institute of Technology,April 14, 2017, https://news.mit.edu/2017/explained-neural-networks-deep-learning-0414. 7 Howard Strauss, “The Future of the Web, Intelligent Devices, and Education, ” 2007, https://er.educause.edu/articles/2007/1/the-future-of-the-web-intelligent-devices-and-education.
everywhere. Applications include those that are likely benign and those that raise more ethical
concerns. For instance, AI systems control personal entertainment on digital platforms. Netflix
decides what movies to show users to increase the time users spend on a platform. Google
displays unique results for users signed into an account based on previous searches and
demographic information. Facebook creates unique newsfeeds based on user data gathered on
their platform. The average American in 2019 and 2020 spent around two and a half hours on
social media platforms per day. 8 AI systems achieve their goal of getting users to spend time on
platforms.
Beyond these more novel applications, structural shifts powered by AI systems aim to
reshape the private sector. Adopting AI systems promises to maximize accuracy, efficiency of
resource allocation, and save time and money. Healthcare professionals employ predictive
algorithms to search X-rays and MRIs. Because analyzing these images requires time-consuming
work from highly skilled professionals, the promise and initial success of employing AI to
accurately perform the task excites many. 9 Many job recruiters today save time by first sorting
job applications through AI systems before ever seeing them.10 This streamlines the ability to
find candidates who meet certain requirements without having to read through ‘unfit’ applicants.
Applications of the technology also extend into the public sector. The applications of AI
employed by the government are the primary focus of this thesis. Judges increasingly employ
predictive models to help decide sentencing lengths. Trained on data from past cases, algorithms
8 H Tankovska,
“Daily Social Media Usage Worldwide, ” Statista, February 8, 2021, https://www.statista.com/statistics/433871/daily-social-media-usage-worldwide/. 9 Patricia M. Johnson, Michael P. Recht, and Florian Knoll, “Improving the Speed of MRI withArtificial Intelligence, ” Seminars in Musculoskeletal Radiology 24, no. 1 (February 2020): 12–20, https://doi.org/10.1055/s-0039-3400265. 10 Rebecca Heilweil, “Artificial Intelligence Will Help Determine If You Get Your next Job, ” Vox, December 12, 2019, https://www.vox.com/recode/2019/12/12/20993665/artificial-intelligence-ai-job-screen.
assess the “risk of recidivism” and other factors to help give a judge context in deciding severity
of punishment. Law enforcement officials in cities and national agencies search through facial
11
recognition platforms across databases of images to better identify suspects.12 Parsing through
databases of facial images and other biometric information such as gait can allow for
better-than-human performance in positively matching individuals.13 Public benefits define
another broad area of interest where the government seeks to apply AI systems. Deciding how
14
public benefits should be appropriated creates one of the biggest areas of government
decision-making. AI systems present the opportunity to maximize efficiency along whatever
outcome a developer might desire. The lack of present transparency limits intimate knowledge of
public benefits applications of the technology, but scattered reports speak to the already-present
use of the technology in this capacity. 15
Present Concerns
AI technologies are built on top of existing systems, which opens the door for inequality
and bias. Social systems and institutions are already in place, and innovation must consider
history. Emerging technologies affect different populations in distinct manners. A quick
overview of statistics on internet access indicates how a fast-expanding technology can bolster
inequality. The United States Census Bureau in 2018 found that while 78% of Americans
subscribe to home internet, rural and low-income communities lag behind national averages by
11 Craig Schwalbe,
“AMeta-Analysis of Juvenile Justice RiskAssessment Instruments: Predictive Validity by Gender, ” Criminal Justice and Behavior 35 (July 30, 2008): 1367–81, https://doi.org/10.1177/0093854808324377. 12 Clare Garvie,Alvaro Bedoya, and Jonathan Frankle, “The Perpetual Line-Up, ” Perpetual Line Up, October 18, 2016, https://www.perpetuallineup.org/. 13 Daniel Kang, “Chinese ‘gait Recognition’Tech IDs People by How They Walk, ”AP NEWS, November 6, 2018, https://apnews.com/article/bf75dd1c26c947b7826d270a16e2658a. 14 Garvie, Bedoya, and Frankle, “The Perpetual Line-Up. ” 15 Rashida Richardson, Jason M Schultz, and Vincent M Southerland, “LitigatingAlgorithms 2019 US Report” (AI Now Institute: New York University, September 2019).
around 15%.16 White households steadily have around a 6% higher level of internet access than
Hispanic or Black households across all levels of income.17 Inequity is not unique to AI and
permeates into digital spaces in many capacities. The rapid expansion of capability and
widespread implementation of AI systems today necessitate pinpointing limitations explicit to its
current applications and implicit to using deep learning. Technical and design limitations exist
with narrow AI systems, and broader ethical worries arise from the power of these systems.
Input bias plagues deep learning. As ML relies on large data sets to learn and train
algorithms, the technology is susceptible to parroting bias built into data sets from which it
learns.18 Racial disparities in facial recognition technologies have garnered national attention.
NIST’s most recent report on discrimination documents disparities in performance across many
algorithms resulting in worse results on people of color, women, and young people aged 18-30.19
Critics have decried the use of “pale male” training data sets that underrepresent minorities,
young people, and women and overrepresent older white men.20 Training disparities have shown
that algorithms built on data skewed to represent one population more than others will
subsequently perform better on that specific population. For example, a 2018 American Civil
21
16 Michael Martin,
“Rural and Lower-Income Counties Lag Nation in Internet Subscription, ” The United States Census Bureau, December 6, 2018, https://www.census.gov/library/stories/2018/12/rural-and-lower-income-counties-lag-nation-internet-subscription.ht ml. 17 “Demographics of Internet and Home Broadband Usage in the United States, ” Pew Research Center: Internet, Science & Tech (blog), June 12, 2019, https://www.pewresearch.org/internet/fact-sheet/internet-broadband/. 18 John Villasenor, “Artificial Intelligence and Bias: Four Key Challenges, ” Brookings (blog), January 3, 2019, https://www.brookings.edu/blog/techtank/2019/01/03/artificial-intelligence-and-bias-four-key-challenges/. 19 Patrick Grother, Mei Ngan, and Kayee Hanaoka, “Face Recognition Vendor Test Part 3: Demographic Effects” (Gaithersburg, MD: National Institute of Standards and Technology, December 2019), https://doi.org/10.6028/NIST.IR.8280. 20 Joy Buolamwini, “Gender Shades, ” MIT Media Lab, 2018, https://www.media.mit.edu/projects/gender-shades/overview/. 21 Robin Materese, “NIST Evaluation ShowsAdvance in Face Recognition Software’s Capabilities, ” text, NIST, November 30, 2018, https://www.nist.gov/news-events/news/2018/11/nist-evaluation-shows-advance-face-recognition-softwares-capabili ties.
Liberties Union (ACLU) report noted 28 false positives matching Members of Congress to
criminal mugshot photos through Amazon’s facial recognition software. Included in this
22
abysmal performance were a disproportionately high number of nonwhite representatives. The
consequences of faulty technology are real, and reports of arrests made on false matches through
facial recognition searches have trickled out around the nation.23 Explaining the phenomenon are
biases in training data but also in design. Input bias can come from unintentional or intentional
biases in design. Algorithms are created to complete a task and reflect the ideas of those who
24
design them. A large underrepresentation of minority professionals in computer science and
other STEM fields means that many projects do not have diverse descriptive representation.25
This can produce unintended consequences in AI technologies. In 2015, a Black hotel guest
found it curious that a soap dispenser did not recognize his hand but would recognize his white
counterpart’s hand.26 A review by the soap dispenser’s designer discovered that the strategy used
to sense hands, bouncing light back from skin, worked significantly worse on darker skin. Input
bias from a lack of descriptive representation can thus create inequality.
The designed ability for ML algorithms to continue to evolve after being deployed leaves
room for more bias. Deep learning relies on an algorithm automatically adjusting itself over time
based on inputted data. Bias grows from this dynamic nature of AI, as systems can develop
22 Jacob Snow,
“Amazon’s Face Recognition Falsely Matched 28 Members of Congress With Mugshots, ”American Civil Liberties Union, July 26, 2018, https://www.aclu.org/blog/privacy-technology/surveillance-technologies/amazons-face-recognition-falsely-matched28. 23 Materese, “NIST Evaluation ShowsAdvance in Face Recognition Software’s Capabilities. ” 24 Ruha Benjamin, “RaceAfter Technology, ” Ruha Benjamin, accessed July 17, 2020, https://www.ruhabenjamin.com/race-after-technology. 25 Ali Karbassi, “Mission, ” WeAll Code, accessedApril 26, 2021, https://www.weallcode.org/our-story/.
26 Max Plenke,
“The Reason This ‘Racist Soap Dispenser’Doesn’t Work on Black Skin, ” Mic, 2015, https://www.mic.com/articles/124899/the-reason-this-racist-soap-dispenser-doesn-t-work-on-black-skin.
biases over time based on the information received.27 A Microsoft project to release a chatbot
onto Twitter quickly came under fire after the bot began spewing racist trope on its first day.
28
While not trained to house racial attitudes, the bot learned on its own from those who interacted
with it and evolved to mirror their attitudes. Because AI algorithms evolve constantly with new
information, bias can enter over time through regular use.
Domain specificity limits AI systems today with current technological design preventing
universal solutions. As discussed, only narrow AI presently exists. Bias further evolves in the
application of AI to diverse settings.29 Rules that govern some aspects of society are not
acceptable in other places. An AI system designed to estimate life insurance policies can use age
as a factor while this same AI system could not discriminate along age lines if it were tasked
with helping in the hiring process for those working at the same life insurance company. 30 People
have complex rules that are hard for computers to fully grasp. This presents scalability issues.31
While tempting to use an existing technology designed in one domain elsewhere, AI systems
today need to be carefully adjusted to fit new tasks. As many algorithms are created to provide
efficiency and cut costs, local contexts are often overlooked.32
Further compounding concerns is an innate and designed complexity preventing humans
27 Villasenor, “Artificial Intelligence and Bias. ”
28 Elle Hunt, “Tay, Microsoft’sAI Chatbot, Gets a Crash Course in Racism from Twitter, ” the Guardian, March 24,
2016, http://www.theguardian.com/technology/2016/mar/24/tay-microsofts-ai-chatbot-gets-a-crash-course-in-racism-fromtwitter. 29 Villasenor, “Artificial Intelligence and Bias. ” 30 “Age Discrimination | U.S. Department of Labor, ” accessedApril 28, 2021, https://www.dol.gov/general/topic/discrimination/agedisc. 31 Michael Chui et al., “Notes from theAI Frontier:Applications and Value of Deep Learning, ” McKinsey,April 17, 2018, https://www.mckinsey.com/featured-insights/artificial-intelligence/notes-from-the-ai-frontier-applications-and-value -of-deep-learning. 32 Loukissas, All Data Are Local: Thinking Critically in a Data-Driven Society.
from fully understanding AI systems. The technology is complex and designed to consider many
more factors than a human can manage.33 The complexity of AI creates a tradeoff with AI always
vulnerable to unknown problems because humans do not fully understand it.34 Just because it
appears to humans as though a certain motivation exists behind a certain algorithmic decision,
there is no guarantee that the algorithmic decision is based on what humans might think. Beyond
the complexity of deep learning, proprietary restrictions often prevent real scrutiny of algorithms.
“Black box” systems refer to those protected from audit due to proprietary restrictions.35 In the
Houston Independent School District, for example, teachers were angered after being denied the
ability to hear factors considered by an algorithm evaluating their performance.36 These
performance evaluations affected hiring and contract renewal decisions. Even after enough
backlash emerged to scrap the use of the algorithm, the factors considered by the AI system were
never revealed.
These technical and design limitations of AI systems powered by ML are compounded by
a host of questions on the ethical nature of expanded capabilities of governance. Constitutional
questions concerning levels of surveillance and fairness of decision-making as well as
complicated legal questions on accountability populate present and future worries.
Technologies powered by AI offer previously unimagined amounts of power. If not
carefully overseen, the capabilities of AI systems might be used unjustly. This raises further
33 Hardesty,
“Explained. ” 34 Ryan Calo et al., “Autonomous Systems Failures: Who Is Legally and Morally Responsible?” (School of Law, November 18, 2020), https://www.law.northwestern.edu/student-life/events/eventsdisplay.cfm?i=176039&c=2. 35 Dillon Reisman et al., “Algorithmic ImpactAssessments:APractical Framework for PublicAgency Accountability, ” Impact Assessment 13, no. 1 (April 2018): 3–30, https://doi.org/10.1080/07349165.1995.9726076. 36 Shelby Webb, “Houston Teachers to Pursue Lawsuit over Secret Evaluation System, ” HoustonChronicle.com, May 12, 2017, https://www.houstonchronicle.com/news/houston-texas/houston/article/Houston-teachers-to-pursue-lawsuit-over-sec ret-11139692.php.
concerns about the appropriate scope of government use of AI. Authoritarian regimes use facial
recognition and smart policing algorithms to surveil political dissidents.37 This creates dangerous
conditions and limits free speech and other liberties. The spread of surveillance is not unique to
authoritarian regimes, as “[a]t least seventy-five out of 176 countries globally are actively using
AI technologies for surveillance purposes”.38 Domestically, the use of AI systems for this
purpose is seen as unpopular by nearly two-thirds of Americans, who are concerned with the
amount of personal data collected by the government.39 With constant surveillance by police,
safeguarding rights to freedom of speech and other Constitutional protections becomes
necessary. Under surveillance, individuals are less likely to speak freely. 40 Precedent has shown
that the Fourth Amendment does not provide as much protection on unwarranted searches as
some would hope. Looking at the Federal Bureau of Investigation (FBI)’s database of 640
41
million pictures, many images regularly included in searches come from innocent citizens,
including the more than 146 million passport photos as of 2019.42
Algorithms powered by AI must often rely on a selected and encoded definition of
fairness which thus places these algorithms in the position of decision-makers. The concept of
fairness is subjective, and the subjective nature of defining a fair outcome makes the design of
37 Stephen Kafeero,
“Uganda Is Using Huawei’s Facial Recognition Tech to Crack down on Dissent after Protests, ” QuartzAfrica, November 27, 2020, https://qz.com/africa/1938976/uganda-uses-chinas-huawei-facial-recognition-to-snare-protesters/. 38 Steven Feldstein, “The Global Expansion ofAI Surveillance, ” Carnegie Endowment for International Peace, September 17, 2019, https://carnegieendowment.org/2019/09/17/global-expansion-of-ai-surveillance-pub-79847. 39 BrookeAuxier and Lee Rainie, “Key Takeaways onAmericans’Views about Privacy, Surveillance and Data-Sharing, ” Pew Research Center (blog), November 15, 2019, https://www.pewresearch.org/fact-tank/2019/11/15/key-takeaways-on-americans-views-about-privacy-surveillance-a nd-data-sharing/. 40 Elijah Cummings, “Facial Recognition Technology (Part II): Ensuring Transparency in Government Use, ” § House Oversight Committee (2019). 41 Cummings. 42 Gretta Goodwin, “Face Recognition Technology, ” Testimony Before the Committee on Oversight and Reform, House of Representatives, June 4, 2019.
algorithms difficult.43 Fairness, Accountability, and Transparency in ML (FAT/ML) highlights
fairness as the need to “[e]nsure that algorithmic decisions do not create discriminatory or unjust
impacts when comparing across different demographics”. A predictive policing algorithm
44
meant to assign officers to locations where crime is most likely to happen decides that it is fair to
assume that locations of past arrests can be predictive of future crimes.45 However, critics argue
that a higher presence of police leads to the creation of more data detailing a greater number of
arrests in that area where more police are assigned. Defining a fair distribution of police officers
thus relies on some subjectivity assumed by the creators of the algorithm but not universally
agreed upon.
AI systems operate in many different domains further complicating accountability.
Massive disparity exists in the consequences of getting decisions wrong across these different
domains.46 Netflix crashing because of a bug is very different than a plane crashing due in part to
software failure that includes many fatalities or someone being mistakenly identified as a
criminal by facial recognition technology. 47 A single approach at holding AI systems accountable
cannot properly encompass the variety of mediums where the technology is employed. Practical
legal limitations have emerged in attempting to hold systems accountable after failures. After an
automated car crashed into and killed a pedestrian walking her bicycle in Arizona, a Department
of Transportation investigation charged the safety driver with negligent homicide.48 Digging into
43 Deirdre K. Mulligan et al.,
“This Thing Called Fairness: Disciplinary Confusion Realizing a Value in Technology, ” Proceedings of the ACM on Human-Computer Interaction 3, no. CSCW (November 7, 2019): 119:1-119:36, https://doi.org/10.1145/3359221. 44 “Principles forAccountableAlgorithms and a Social Impact Statement forAlgorithms: FAT ML, ” accessed March 11, 2021, https://www.fatml.org/resources/principles-for-accountable-algorithms. 45 Benjamin, “RaceAfter Technology. ” 46 Villasenor and Solow-Niederman, HoldingAlgorithmsAccountable. 47 Calo et al., “Autonomous Systems Failures. ” 48 Rory Cellan-Jones, “Uber’s Self-Driving Operator Charged over Fatal Crash, ” BBC News, September 16, 2020, sec. Technology, https://www.bbc.com/news/technology-54175359.
the logs from the algorithm of the car found that it failed to stop for the pedestrian as it could not
determine whether it was seeing a person or a bike and did not properly store information
because of its confusion.49 While it was the algorithm that powered the car into the pedestrian
due to its failure, the driver faced accountability for the accident. The unprecedented situation
highlights how existing rules of governance do not yet establish full accountability for AI
systems.
AI systems create unique questions on governance. Difficulty in finding regulatory
solutions is created by the rapidly evolving landscape of digital innovation and the massive array
of domains with widely varying levels of significance. In instances where the government is the
direct consumer of AI systems, these algorithms can even become primary decision-makers. The
importance of finding solutions to regulate the technology is compounded by sweeping concerns
over bias and inequality in many forms.
49 Calo et al., “Autonomous Systems Failures. ”
III. Literature Review
In a market, governments can either impose regulations through traditional public policy
channels or provide less structure and allow for consumers or firms to guide the market.
Understanding the key influences in each of these scenarios is thus important in answering the
questions of why governance of AI has largely relied on private market regulation and under
what conditions greater government action might be expected. The two channels, traditional
public methods and private politics, may be employed simultaneously or separately by those
seeking changes in practice.50 Each will be explored in depth along with the unique challenges
stemming from regulating AI.
Traditional routes of regulation rely on public governance, either through the legislative
process or through bureaucratic rulemaking.51 For regulation initiated by elected officials, we
might expect their regulatory decisions to reflect their own views and the potentially competing
pressures on members of Congress and elected officials at all levels of government from citizens’
preferences, interest groups, political parties, and other outside actors.52 In AI, as in many issues,
the regulatory environment may be much more salient to interest groups than to constituents,
suggesting that interest groups may be influential in the direction of policy. However, if AI
regulation is made more salient to the public, legislators may be more responsive to constituency
50 David P. Baron,
“Private Politics, ” Journal of Economics & Management Strategy 12, no. 1 (2003): 31–66, https://doi.org/10.1111/j.1430-9134.2003.00031.x. 51 Christopher Carrigan and Cary Coglianese, “The Politics of Regulation: From New Institutionalism to New Governance, ” SSRN Scholarly Paper (Rochester, NY: Social Science Research Network, June 1, 2011), https://doi.org/10.1146/annurev.polisci.032408.171344; Susan Webb Yackee, “The Politics of Rulemaking in the United States, ” SSRN Scholarly Paper (Rochester, NY: Social Science Research Network, May 1, 2019), https://doi.org/10.1146/annurev-polisci-050817-092302. 52 P. Burstein andA. Linton, “The Impact of Political Parties, Interest Groups, and Social Movement Organizations on Public Policy: Some Recent Evidence and Theoretical Concerns, ” Social Forces 81, no. 2 (December 1, 2002): 380–408, https://doi.org/10.1353/sof.2003.0004.
pressures, reducing the power of business interest groups.53
With the exception of citizens-focused interest groups, the priorities of interest groups
can be contrary to those of individuals. Interest group priorities, often disproportionately
supported by corporate sponsors, do not tend to align with priorities of the public.54 Regardless
of income or class, the public agenda contains different policy issues than those pedaled by
interest groups. In the domain of AI, business or corporate interest groups behind the AI systems
may prefer little government regulation while the general public may favor greater regulation.
Scholars agree that interest groups seek to impact the legislative process, yet consensus is
split on whether access- or replacement-oriented approaches are more effective for interest
groups.55 Access-oriented models suggest that interest groups donate money to legislators to
purchase their time once in office, giving them the opportunity to contact legislators and press
their concerns. Replacement-oriented models suggest that interest groups seek to influence
electoral outcomes, helping put elected officials with like-minded views in office.
Regardless of whether they pursue a replacement or access strategy, monetary donations
are one route through which interest groups attempt to influence outcomes in policymaking.
Campaign financing is targeted as one means to have influence over policy outcomes and can
align with either a replacement or access strategy. 56 As a replacement-oriented tactic, campaign
53 Daniel M. Butler and David W. Nickerson,
“Can Learning Constituency OpinionAffect How Legislators Vote? Results from a Field Experiment, ” Quarterly Journal of Political Science 6, no. 1 (August 22, 2011): 55–83, https://doi.org/10.1561/100.00011019. 54 David C Kimball et al., “Who Cares about the LobbyingAgenda?, ” Interest Groups & Advocacy 1, no. 1 (May 1, 2012): 5–25, https://doi.org/10.1057/iga.2012.7. 55 Samuel Issacharoff and Jeremy Peterman, “Special InterestsAfter Citizens United:Access, Replacement, and Interest Group Response to Legal Change, ” Annual Review of Law and Social Science 9, no. 1 (2013): 185–205, https://doi.org/10.1146/annurev-lawsocsci-102612-133930. 56 Rui J. P. De Figueiredo and Geoff Edwards, “Does Private Money Buy Public Policy? Campaign Contributions and Regulatory Outcomes in Telecommunications, ” Journal of Economics & Management Strategy 16, no. 3 (2007): 547–76, https://doi.org/10.1111/j.1530-9134.2007.00150.x.
financing influences regulatory outcomes quickly with private money through the installation of
legislators with views aligned to those of the interest group. This method is especially effective
in local and state races, where interest groups specifically target off-cycle elections to extend the
reach of their investment.57 Interest group spending is more efficient in these smaller elections
because fewer voters are participating. Campaign spending can also be focused around an access
strategy. Financing by interest groups specifically targets members and other elected officials in
leadership positions and on committees with jurisdiction over the industry. 58 Party and committee
leaders have power over the agenda setting process, making access to these powerful members
valuable for interest groups. Much like spending in off-cycle elections, this access-oriented
approach maximizes the value of contributions by placing them where regulatory power is most
centralized. Unlike replacement-oriented methods, this access-oriented method is dynamic.
Private interest groups can quickly adapt to changing actors on relevant committees that oversee
their interests by bankrolling new members and offloading those removed.59 And in fact, research
has found that interest groups target spending at the members of those committees that regulate
their industry. 60 By donating to the gatekeepers and elected officials on key committees with
jurisdiction to regulate the industry, interest groups may seek to benefit from enhanced access to
these officeholders. This research suggests that, in the domain of AI, business-oriented interest
groups that make the technology may use donations and other strategies to limit government
57 Sarah F.Anzia,
“Election Timing and the Electoral Influence of Interest Groups, ” The Journal of Politics 73, no. 2 (April 1, 2011): 412–27, https://doi.org/10.1017/S0022381611000028. 58 Roger H. Davidson et al., “Leaders and Parties in Congress, ” in Congress and Its Members, 16th ed. (CQ Press, 2017);Alexander Fouirnaies, “WhenAreAgenda Setters Valuable?, ” American Journal of Political Science 62, no. 1 (2018): 176–91, https://doi.org/10.1111/ajps.12316. 59 Eleanor Neff Powell and Justin Grimmer, “Money in Exile: Campaign Contributions and CommitteeAccess, ” The Journal of Politics 78, no. 4 (August 3, 2016): 974–88, https://doi.org/10.1086/686615. 60 Fouirnaies, “WhenAreAgenda Setters Valuable?”
regulation if they choose.
Although scholars have amassed evidence on the spending patterns of interest groups that
are consistent with different interest group-based strategies of impacting the legislative process,
research on the policy impact of interest group activities is less conclusive, and there still
remains a gap in studies directly linking these strategies to policymaking outcomes.61 Scholars
often overstate the influence of interest groups in policy outcomes when there is little evidence
that it alone can guide legislative decisions. Many studies fail to include the effects of public
opinion alongside interest group influence altogether. 62 This lack of mention of public opinion is
worrisome, as the effects of public opinion can have strong influence over the legislative agenda,
especially at a state level.63 Even though business-oriented interest groups often have extensive
monetary resources, they are not able to guarantee their preferred outcome as many groups –
businesses and industries, as well as citizens’ groups – may align on each side, making the sides
more evenly pitted.64 Because so many interests are involved on each side, the power of any one
source of influence is hindered. In the domain of AI, business-oriented interest groups may be
active and have many monetary resources, but if they are countered by citizens’ groups and other
interest groups with their own monetary and membership resources, it is not clear which side
would prevail. Without consensus on this fundamental aspect of the financial influence of
interest groups and other outside actors, their actual role in achieving policy outcomes is unclear.
61 Marie Hojnacki et al.,
“Studying OrganizationalAdvocacy and Influence: Reexamining Interest Group Research, ” Annual Review of Political Science 15 (June 2012): 379–99. 62 Burstein and Linton, “The Impact of Political Parties, Interest Groups, and Social Movement Organizations on Public Policy. ” 63 Matthew Fellowes, Virginia Gray, and David Lowery, “What’s on the Table? The Content of State Policy Agendas, ” Party Politics 12, no. 1 (January 1, 2006): 35–55, https://doi.org/10.1177/1354068806059246. 64 Frank R. Baumgartner et al., “Money, Priorities, and Stalemate: How LobbyingAffects Public Policy, ” Election Law Journal: Rules, Politics, and Policy 13, no. 1 (March 1, 2014): 194–209, https://doi.org/10.1089/elj.2014.0242.
Further complicating the picture within the study of AI are aligning interests between
government officials and those companies providing technologies. Since AI technologies can
sometimes directly perform governmental functions, regulation would directly affect the
government’s ability to use the technology. 65 In this case, the government as a direct consumer
could create an alignment with corporate interests. That alignment limits the insights from
political science literature on interest group influence. Literature to date focuses on competing
interests of the public agenda and interest groups without considering the government as a group
with direct interest.
An alternative route of regulation focuses on private politics. In standard views of private
politics, the market produces regulation when activists in the public mobilize consumers to alter
their purchasing behavior, since this induces companies or industries to change their practices.
Two main strategies for consumers are boycotts and buycotts. Boycotts target firms by publicly
withdrawing support for a company, and buycotts do so by withholding purchases of a specific
product or products made by a producer. By pressuring firms publicly, directly, and quickly,
private politics are appealing because of the allowance for much quicker action than that through
public channels.66 These campaigns have grown in popularity recently in part due to an increase
in communication permitted by social media use.67 With better platforms for direct appeals to be
made, these campaigns continue to prove effective in many cases. For example, controversial
political comments from MyPillow CEO and founder Mike Lindell in 2020 led to widespread
65 Kate Crawford and Jason Schultz,
“AI Systems as StateActors, ” Columbia Law Review, 2019, https://columbialawreview.org/content/ai-systems-as-state-actors/. 66 David P. Baron and Daniel Diermeier, “StrategicActivism and Nonmarket Strategy, ” Journal of Economics & Management Strategy 16, no. 3 (2007): 599–634, https://doi.org/10.1111/j.1530-9134.2007.00152.x. 67 Kyle Endres and Costas Panagopoulos, “Boycotts, Buycotts, and Political Consumerism inAmerica, ” Sage Journals 4, no. 4 (November 1, 2017), https://journals.sagepub.com/doi/full/10.1177/2053168017738632.
calls to boycott the product and the popular brand subsequently being removed from sale at
leading home furnishing stores across the country. 68
This form of private politics relies on activists to monitor and sanction firms through
public campaigns targeting a chosen firm with the hope of enacting industry-wide changes.69 If
enough pressure is applied to the target, they will be incentivized into changing their practices,
and other firms will follow to avoid future targeting. To be successful, market-led governance
relies on knowledge of company practices and direct consumerism.70 Direct channels between
company and consumer are where pressure is placed to enact change.71
American governance of AI to date has largely shied away from traditional legislative
routes and instead has been heavily reliant upon private politics. Bipartisan support for the
strategy has persisted across multiple administrations.72 Even without the ability of consumers to
realistically sanction firms, public policy makers at multiple levels of government have
nonetheless kept the bulk of AI regulation in the hands of the market. However, applying popular
strategies of private politics to AI presents a problem because the consumer cannot easily boycott
a particular AI firm.73 Instead, most private regulation of AI has relied on firms alone, counting
on companies to police the use of their own technology. This strays from the traditional literature
68 Elizabeth Chang, “MyPillow Boycott: How a Product Can Spark an Identity Crisis, ” Washington Post, accessed
March 7, 2021, https://www.washingtonpost.com/lifestyle/wellness/my-pillow-lindell-boycott-customers/2021/02/12/7399aaa4-6af1 -11eb-9ead-673168d5b874 _ story.html. 69 Erin M. Reid and Michael W. Toffel, “Responding to Public and Private Politics: Corporate Disclosure of Climate Change Strategies, ” Strategic Management Journal 30, no. 11 (2009): 1157–78, https://doi.org/10.1002/smj.796. 70 Endres and Panagopoulos, “Boycotts, Buycotts, and Political Consumerism inAmerica. ” 71 James N. Druckman and Julia Valdes, “How Private PoliticsAlters Legislative Responsiveness, ” Quarterly Journal of Political Science 14, no. 1 (January 1, 2019): 115–30, https://doi.org/10.1561/100.00018066. 72 Corinne Cath et al., “Artificial Intelligence and the ‘Good Society’: The US, EU, and UKApproach, ” Science and Engineering Ethics 24, no. 2 (April 1, 2018): 505–28, https://doi.org/10.1007/s11948-017-9901-7; “Artificial Intelligence for theAmerican People, ” The White House, accessed October 11, 2020, https://www.whitehouse.gov/ai/. 73 Garvie, Bedoya, and Frankle, “The Perpetual Line-Up. ”
on the subject focusing on the threat of sanction by consumers. There are two reasons the threat
of sanction by consumers, which is standard in most models of private politics, is unrealistic in
AI. First, with the inner workings of AI systems often protected as trade secrets, there exists a
present lack of transparency and a room for “meaningful scrutiny and accountability”.74 For
instance, teachers being evaluated by algorithms on their performance are not permitted to know
what goes into their performance scores. Access to knowledge of the metrics that might get them
fired or the weight given to each component of their performance is restricted.75 Federal agencies
furtively employ facial recognition technologies to identify suspects without publishing baseline
standards or disclosure of practices.76 This lack of transparency removes traditional channels
between consumer and company that allow for private politics to target firms. Second, the
consumers of AI products include the government itself, making traditional models of private
politics limited. Implementations of AI systems often center around their use as the “core logic”
of governmental functions “contributing to the process of government decisionmaking”.77 In
2016, for example, Arkansas shifted their program from a direct review by a nurse to an
AI-powered algorithm deciding the number of benefit care hours for those on Medicaid.78 This
meant that the direct function of the government previously performed by a nurse now rested on
the proprietary and hidden assessment of a privately produced algorithm purchased by the state.
The state of Arkansas became the consumer and left those being assessed without the ability to
understand the factors used to assess their need for care hours. As a result, the public could do
little to boycott or buycott.
74 Reisman et al., “Algorithmic ImpactAssessments:APractical Framework for PublicAgencyAccountability. ”
75 Webb,
“Houston Teachers to Pursue Lawsuit over Secret Evaluation System. ” 76 Goodwin, “Face Recognition Technology. ” 77 Crawford and Schultz, “AI Systems as StateActors. ” 78 Richardson, Schultz, and Southerland, “LitigatingAlgorithms 2019 US Report. ”
An emerging body of literature in private politics delves into industry self-regulation.
Because of the limited ability within AI for consumers to directly place themselves between a
product and its user when that user is the government, it is important to consider how effective
private politics can be without the power of public interest and consumer-based boycotts or
buycotts. The concrete result of private politics in AI generally takes the form of internal codes
of conduct and industry standards informally agreed upon across companies. Scholars find that
the nature of these solutions being internal to corporations and hidden to the public means that
there is no reliable data on the strictness of adherence to the self-set standards.79 Therefore,
significant limitations exist to measuring the effectiveness of present governing strategies
because of insufficient access to intimate internal knowledge of companies. Scholarly findings
indicate that the threat alone of regulation through traditional government is not influential in
changing industry practices.80 This further limits the understanding of the effectiveness of
self-governance. Industries will generally not change their practices until public legislation is
passed. A reliance on industries to self-govern might be insufficient in guaranteeing the interests
of the public can be heard.
A review of AI’s relationship to existing political science literature on private politics
highlights the limits of effective pressure for regulatory action when there is little consumer
connection or transparency. Literature on private politics and its ability to bring regulation fails
to fully address cases of government reliance on private industry in which the public is not a
79 Andrew King,Andrea Prado, and Jorge Rivera,
“Industry Self-Regulation and Environmental Protection, ” The Oxford Handbook of Business and the Natural Environment, November 2011, https://www-oxfordhandbooks-com.turing.library.northwestern.edu/view/10.1093/oxfordhb/9780199584451.001.00 01/oxfordhb-9780199584451-e-6. 80 David Vogel, “Private Global Business Regulation, ” Annual Review of Political Science 11 (June 6, 2008), https://doi.org/10.1146/annurev.polisci.11.053106.141706.
direct consumer. The present lack of transparency regarding the use of AI technologies in
governmental capacities further limits activists’ abilities to target firms without intimate
knowledge of industry practices. Since activists are also not always consumers from firms, they
are further limited in their ability to campaign towards change.
Existing literature on regulation through public, government channels and through private
politics provides several important insights to the study of AI regulation. First, the decisions of
elected officials reflect the potentially competing pressures they face from their own interests,
constituent pressures, and interest group pressure. Second, while there are many reasons to
suspect that business-oriented groups will have some access to elected officials, particularly
when government and business interests in the domain of AI align, highly prominent issues may
weaken the power of specific industry groups and create more room for consumer groups, public
opinion, and other factors to matter. On issues where the public is uninformed or does not have
clear views, interest groups may have the ear of elected officials. When government officials
know an issue is salient and potentially electorally relevant, they may be more responsive to
constituent preferences. Third, traditional methods of private politics involving consumer
purchasing power are unlikely to be influential in the domain of AI when the primary consumer
of AI is the government itself. Combined with the lack of transparency innate to AI algorithms
and practices, this limits the ability of consumers to force regulatory change.
IV. Analytic Framework and Research Design
Expectations
In general, there remains very little government regulation over AI technologies today.
Yet these technologies have now been popular for almost a decade. Many of the adverse effects
on the public are well-documented, yet transparency into the inner workings of algorithms or
even their use in general remains sparse. AI technologies exist in different capacities across
many sectors. Technologies that aim to fundamentally alter large industries continue to innovate
in a fairly unrestricted manner. Associate Director of Stanford’s Institute for Human-Centered AI
Daniel Ho sums up the current regulatory environment surrounding AI as a “Wild West” noting:
Facial recognition technology is being adopted by banks, airlines, landlords, school principals,
and, most controversially, law enforcement, without much guiding the data quality, validation,
performance, and potential for serious bias and harm. We saw far more consensus around the
problems, than about solutions. 81
A gap clearly exists on legislative response to the growth of the technology. Why is there still so
little regulation? When might the government be willing to constrain its own use of AI
technologies?
I argue that there are two main reasons that regulation of government use of AI has been
so limited. First, the pace of technological change produces a difficult regulatory hurdle. Policy
to address today’s problem may limit the ability to address the problems that arise tomorrow.
Second, the government is the direct consumer, which may lead their incentives to align with
81 Daniel E. Ho,
“Developing Better, Less-Biased Facial Recognition Technology, ” Stanford Human-Centered Artificial Intelligence, November 9, 2020, 20, https://hai.stanford.edu/blog/developing-better-less-biased-facial-recognition-technology.
those of technology companies and reduce interest in regulation. These cases increase the
likelihood that the interests of governing bodies align with those of AI business interest groups,
and they also suggest that absent an event that increases the salience of AI technology, the public
will be ill-informed and unlikely to exert pressure on legislators to regulate AI. As a result, I
hypothesize that there will only be successful regulation of the use of AI by the government
when outside groups (e.g., citizens-focused interest groups) are very active on the issue and raise
awareness through public exposés. Importantly, for citizens’ groups, they cannot organize
boycotts or buycotts because boycotting the government is infeasible. However, they can focus
on publicity campaigns to increase the salience of the issue in the public, leading to greater
pressure from the public on elected officials as well.
This project analyzes the conditions that foster regulatory action versus inaction of AI
with a focus on the role of advocacy groups. To do so, the role of citizens’ groups and other
outside interest groups are compared when differing legislative outcomes occur.
Research Design
To assess the conditions that foster or inhibit regulation of the government’s use of AI,
this analysis relies on exploratory case studies of key explanatory variables and political
dynamics at work in the field of AI. A most similar cases strategy is used with the goal of
targeting uses of AI where a reliance exists both on private and traditional governance.82 These
cases will analyze proposed laws that are enacted as well as those that fail with the goal of better
understanding the factors that contributed to success or failure.
The complex nature of AI, its use across different domains, regulatory efforts that span
82 Jason Seawright and John Gerring,
“Case Selection Techniques in Case Study Research:AMenu of Qualitative and Quantitative Options, ” Political Research Quarterly 61, no. 2 (June 1, 2008): 294–308, https://doi.org/10.1177/1065912907313077.
cities, states and the federal government, and the confidential nature of the technology all make
this a difficult subject to study with a large-N analysis. Federal legislation remains too sparse to
be a focus. At the state level, only nineteen states introduced any legislation related to artificial
intelligence in their 2019 and 2020 sessions.83 These proposed and enacted laws span across a
vast array of subjects, thus risking comparing apples to oranges in a large-N study. There does
not presently exist any database of city laws from across the country to study. A lack of
transparency limits this further. Few reports on the use of technologies powered through AI are
available to the public due to a lack of legislation enforcing disclosure. As a result, case studies
offer the more appropriate and more feasible route for study.
When using case studies, there are a number of potential approaches. Selection of an
outlier, average outcome, or pair of similar or diverse outcomes can bring different strengths to a
study. 84 Different necessary information is required to complete each type of case study selection
effectively. In this study, selecting cases on the independent variable, interest group involvement,
creates a near impossible task. Given a lack of data on regulatory efforts or interest group
involvement, finding cases would be challenging. Moreover, selecting cases on the independent
variable would risk selecting vastly different cases for comparison. To do so would allow for
significantly more external variables to influence the legislative outcome, such as the size of the
city, the domain of the AI system, or the partisan makeup of the legislatures involved. As a
result, I leverage a weaker analysis along the dependent variable, legislative outcome. Caution
must be taken in generalizing results from the study and assuming these results would hold
83 “Legislation Related toArtificial Intelligence, ” accessed March 11, 2021, https://www.ncsl.org/research/telecommunications-and-information-technology/2020-legislation-related-to-artificial -intelligence.aspx. 84 Seawright and Gerring, “Case Selection Techniques in Case Study Research. ”
elsewhere, but the results are nonetheless valuable for providing some tentative conclusions.
V. Case Study: Law Enforcement Use of Facial Recognition in New York City and Los Angeles County
The Case
This case study explores police use of facial recognition. The technology is easily
understood, its employment raises controversy, and its use is public facing. Moreover, the
government is the direct consumer of the technology, as police departments (the government
consumer) hire private companies to power their facial recognition search databases. In general,
some legislation to regulate police use of the technology has been passed in city and state
governments with varying specific targets and levels of desired regulation. From complete bans
on facial recognition technology in San Francisco and a handful of other cities to protections on
biometric information in Illinois, restrictions exist in some manner in scattered pockets across the
country. 85 The effectiveness of these attempts at regulation is limited to the jurisdictions to which
they apply, and in most cases, existing laws can be easily circumvented.
My argument rests on two premises, both of which hold in the case of police use of facial
recognition technology. The first premise is that regulating police use of facial recognition
technology (FRT), as with AI more generally, faces challenges from the fast pace of
technological change. Consider the scope of change in just 2013 alone. Following the Boston
Bombing in April 2013, facial recognition searches failed to identify suspects given input images
85 Patrick Fowler and Haley Breedlove, “Facing the Issue: San Francisco Bans City Use of Facial Recognition
Technology, ” JD Supra, July 15, 2019, https://www.jdsupra.com/legalnews/facing-the-issue-san-francisco-bans-35144/; “740 ILCS 14/ Biometric Information PrivacyAct., ” accessed February 1, 2021, https://www.ilga.gov/legislation/ilcs/ilcs3.asp?ActID=3004&ChapterID=57.
captured through surveillance cameras.86 However, a reexamination of the same images through
leading FRT algorithms later that same year proved successful in identifying the same suspects.87
This study highlights the rapid evolution of the technology and the potential usefulness of FRT
algorithms given the right technology in place. While the Boston Bombing case points to the
potential value law enforcement may see with FRT, the speed of innovation can conversely be
problematic for effective government regulation. Legislation that regulates the use of the
technology may respond to previous problems but may be ill-suited to handle future challenges.
Following concerns surrounding surveillance levels of ordinary citizens, the San Francisco Board
of Supervisors voted to ban facial recognition from law enforcement in 2019.88 Similar bans have
been instituted in Boston, Massachusetts, Portland, Oregon, and a handful of smaller cities across
the country. 89 While these bans do well to address immediate concerns over present technological
shortcomings and surveillance concerns, they ignore the quickly changing landscape of FRT. A
growing concern online today focuses on deepfakes, images or videos altered by deep learning
algorithms to appear real even when false. As the prevalence and accuracy increases for
deepfakes, concerns mount over their ability to affect election security, invade personal privacy,
and promote identity theft or other criminal activity. 90 A need for police to monitor for false
86 Joshua Klontz andAnubhav Jain,
“ACase Study ofAutomated Face Recognition: The Boston Marathon Bombings Suspects, ” Computer 46 (November 1, 2013): 91–94, https://doi.org/10.1109/MC.2013.377. 87 Klontz and Jain. 88 Kate Conger, Richard Fausset, and Serge F. Kovaleski, “San Francisco Bans Facial Recognition Technology, ” The New York Times, May 14, 2019, sec. U.S., https://www.nytimes.com/2019/05/14/us/facial-recognition-ban-san-francisco.html. 89 Shannon Flynn, “13 Cities Where PoliceAre Banned From Using Facial Recognition Tech, ” Innovation & Tech Today (blog), November 18, 2020, https://innotechtoday.com/13-cities-where-police-are-banned-from-using-facial-recognition-tech/. 90 Alison Grace Johansen, “Deepfakes: What TheyAre and Why They’re Threatening, ” NortonLifeLock, July 24, 2020, https://us.norton.com/internetsecurity-emerging-threats-what-are-deepfakes.html.
images may arise.91 At the same time, a recently released study evaluating the improving ability
of FRT to parse through images for fake or altered files suggests that the adaptability of the
technology will prove fruitful in the future for law enforcement agencies.92 The increasing need
to address FRT in this new manner and the adaptability of development to tackle face challenge
highlights the speed at which the needs of regulation surrounding FRT can shift. Outright bans
on police use of the technology have been met with backlash for their “shortsighted” approach.93
In the case of deepfakes, a ban on the use of FRT might prevent police from properly analyzing
evidence by banning necessary technology. With rapidly evolving problems and improving
technology, effectively regulating FRT in law enforcement use becomes difficult.
The second premise is that because the government is a direct consumer, its interests are
likely to be aligned with the business-oriented groups behind the AI technology. Private
companies supplying the technology have business interests in mind and are guided by a need to
make profit. Law enforcement officials seek use of the technology to do what humans cannot.
Being able to positively identify criminals objectively through facial recognition technology
provides law enforcement an opportunity to better find suspects and establish credibility in court,
where testimony through eyewitnesses is often unreliable.94 All else equal, each group wants
more use of the technology. On the other side of the debate on access are civil liberties groups
aiming to prevent the invasive technology from advancing policing tactics and over-surveilling
91 Ryan Reynolds,
“Courts and Lawyers Struggle with Growing Prevalence of Deepfakes, ” Stanford Law School, June 9, 2020, https://law.stanford.edu/press/courts-and-lawyers-struggle-with-growing-prevalence-of-deepfakes/. 92 Mei L. Ngan et al., “Face Recognition Vendor Test (FRVT) Part 4: MORPH - Performance ofAutomated Face Morph Detection, ” March 6, 2020, https://www.nist.gov/publications/face-recognition-vendor-test-frvt-part-4-morph-performance-automated-face-mor ph. 93 Conger, Fausset, and Kovaleski, “San Francisco Bans Facial Recognition Technology. ”
94 Dirk Smeets et al.,
“Objective 3D Face Recognition: Evolution,Approaches and Challenges, ” Forensic Science International 201, no. 1–3 (September 10, 2010): 125–32, https://doi.org/10.1016/j.forsciint.2010.03.023.
the public in violation of people's privacy. A majority of Americans are worried about the level
of personal data collected by the government.95 Weary of expanding the capabilities of law
enforcement, citizen activists often cite unrestricted surveillance and increased inequality as
major concerns to the expansion of government use of facial recognition.96
Combined, the fast pace of technological change and government as a direct consumer of
facial recognition technology suggest that regulation may be unlikely. It is hard to regulate
effectively, and regulation would curtail the government’s own use of the technology. Building
from these two premises, I argue that stronger regulation of facial recognition will be more likely
when consumer-oriented groups succeed in making the issue more salient to the public, leading
elected officials to be more attentive to public preferences rather than their own interests. In the
case study that follows, I draw on news sources, studies by governmental and scholarly sources,
information directly from citizens’ groups, and information from interviews with the political
offices who authored the relevant legislation in each case. Local and national news sources are a
good medium for locating detailed information on legislation and the process behind the
enactment of bills. The National Conference of State Legislatures (NCSL) houses data on where
state laws have been passed with regard to AI.97 Because federal and state laws often interact
with local regulation of facial recognition, it is important to understand the context at each level
of government in each case.
For this case study, the Los Angeles Police Department (LAPD)/Los Angeles County
Sheriff’s Department (LASD) is compared to the New York Police Department (NYPD). The
comparison considers local and state policy and legislation impacting the use of FRT by police
95 Auxier and Rainie,
“Key Takeaways onAmericans’Views about Privacy, Surveillance and Data-Sharing. ” 96 Cummings, Facial Recognition Technology (Part II): Ensuring Transparency in Government Use. 97 “Legislation Related toArtificial Intelligence. ”
departments in each city. The similarities in size of the forces and partisan makeup of city and
state government motivate the selection of the two departments. Policing the two most populous
cities in the country, the NYPD and the LAPD/LASD employ around 36,000 and 19,000 full
time officers, respectively, forming two of the three largest law enforcement departments in the
country. 98 Both cities have overwhelmingly Democratic leanings in most city, state, and federal
elections of the past decade.99 This means the cases are similar in the partisan predisposition to
regulate. Crucially, however, the two differ in their recent regulation of the technology. Los
Angeles County currently bans third party facial recognition searches and houses all facial
recognition images for the county in a centralized database made up of only mugshots, and
California state law denies the ability for officers to run facial recognition on body camera
footage.100 The city-specific regulations are set by Los Angeles County and by the Los Angeles
Police Commission.101 A state law approved in 2019 and expiring January 1, 2023 regulates body
camera footage.102 In contrast, New York City has no explicit ban on third party searches, neither
defines the contents of its image database nor where it is housed, and does not limit searches
through body camera footage at either city or state level.103 NYPD policy is internally set by the
98 “About NYPD - NYPD, ” accessed March 15, 2021, https://www1.nyc.gov/site/nypd/about/about-nypd/about-nypd-landing.page; George Gascón, “COMPSTAT Plus -
LosAngeles Police Department, ” accessed March 15, 2021, https://www.lapdonline.org/inside _ the _ lapd/content _ basic _ view/6364; “Sheriff’s Department, ” Government, County of LosAngeles, November 15, 2016, https://lacounty.gov/residents/public-safety/sheriff/. 99 “2020 Election Results | New York State Board of Elections, ” accessed March 14, 2021, https://www.elections.ny.gov/2020ElectionResults.html; Eric McGhee, “California’s Political Geography 2020, ” Public Policy Institute of California, February 2020, https://www.ppic.org/publication/californias-political-geography/. 100 “LACRIS Facial Recognition Policy, ” 2019. 101 Kevin Rector, “Connecting for Honors Thesis Research, ” March 31, 2021.
102 Phil Ting,
“Law Enforcement: Facial Recognition and Other Biometric Surveillance, ” Pub. L. No.AB 1215 (2019), https://leginfo.legislature.ca.gov/faces/billTextClient.xhtml?bill _ id=201920200AB1215. 103 “Facial Recognition - NYPD, ” accessed March 15, 2021, https://www1.nyc.gov/site/nypd/about/about-nypd/equipment-tech/facial-recognition.page.
police department.104 Explicit transparency mandates surrounding surveillance technology do
exist in New York City, but the regulatory impact is significantly weaker than measures in Los
Angeles County. 105 Proposed state laws in New York have to date failed to ban body camera FRT
searches in a similar manner to California’s state law. 106 Thus, Los Angeles County provides a
case of greater regulation against New York City’s lower level of regulation. In both cases,
however, it is worth noting a limitation that stems from the often-hidden nature of deals between
police departments and private companies. Because transparency laws are limited, police
departments do not necessarily disclose the specifics of their use of the technology.
107
Findings
Police departments in both Los Angeles County and New York City share an interest in
using FRT. This could mean that they utilize divergent approaches due to other factors. Between
2014 and 2018, the ability to identify people through facial recognition improved markedly,
making this technology very desirable for law enforcement agencies in general and in both of
these cities.108 NYPD cites the technology’s ability in helping provide matches for “68 murders,
66 rapes, 277 felony assaults, 386 robberies, and 525 grand larcenies since 2019”.109 The
technology has been used in the city since at least 2010, and the increasing accuracy of FRT
104 “Facial Recognition - NYPD. ” 105 Vanessa Gibson, “Creating Comprehensive Reporting and Oversight of NYPD Surveillance Technologies., ” Pub. L. No. Int 0487-2018 (2020), https://legistar.council.nyc.gov/LegislationDetail.aspx?ID=3343878&GUID=996ABB2A-9F4C-4A32-B081-D6F24 AB954A0. 106 Brad Hoylman, “NY State Senate Bill S6776, ” Pub. L. No. S6776 (2019), https://www.nysenate.gov/legislation/bills/2019/s6776; Brad Hoylman, “NY State Senate Bill S7572, ” Pub. L. No. S7572 (2020), https://www.nysenate.gov/legislation/bills/2019/s7572. 107 Benjamin Nober and NYPD Legal, NYPD Facial Recognition Third-Parties, 2021. 108 Patrick J. Grother, Mei L. Ngan, and Kayee K. Hanaoka, “Ongoing Face Recognition Vendor Test (FRVT) Part 2: Identification, ” November 27, 2018, https://www.nist.gov/publications/ongoing-face-recognition-vendor-test-frvt-part-2-identification. 109 “Facial Recognition - NYPD. ”
searches clearly motivates its continued use. In Los Angeles, better policing is reiterated and
used as justification towards employing the technology. LAPD chief Michel Moore lauds FRT
as an “efficient way of working” to complete a task otherwise unmanageable by humans.110
State and local regulation of FRT limits police use of the technology for the LAPD and
LASD while no similar regulations exist for the NYPD. At the state level, California passed a
law in 2019 banning the use of FRT in policy body cameras. At the local level, the Los Angeles
Police Commission reviewed and revised Los Angeles County policies in 2020 and 2021
resulting in the banning of third party FRT searches and limiting searches to a consolidated
database of mugshot images accessible only by trained officers. Conversely, a state bill in New
York proposing a ban on FRT in police body cameras failed in 2019. Although a New York City
Act requiring more transparency by the NYPD was enacted in 2020, the regulatory impact of this
law was minimal. I hypothesize that the greater regulation in Los Angeles county than in New
York City stems from the work of citizens’ groups who increase the public salience of the issue
and thus the costs for inaction by elected officials.
Participation of citizen groups, at least to some degree, led to regulatory change
surrounding FRT in Los Angeles. The ACLU, Black Lives Matter Los Angeles, and Stop LAPD
Spying Coalition have been notably active in pushing for legislative changes and raising the
salience of this issue over multiple years. Activism has increased notably since the start of
111
summer 2020 and continues to date.112 The Office of Assemblymember Ting, the author of the
2019 California legislation that banned the use of FRT in body cameras in the state, indicated
110 Josh Cain, “LAPD Commission OKs Detectives to Use Facial Recognition, ” Government Technology, January
14, 2021, https://www.govtech.com/public-safety/LAPD-Commission-OKs-Detectives-to-Use-Facial-Recognition.html. 111 Rector, “Facial Recognition and the LAPD, ” March 31, 2021.
112 Rector.
that the ACLU even directly helped to draft the language of the bill.113 Moreover, the ACLU was
joined by numerous other citizens’ groups in their support of the bill. An expansive list of
advocate groups for the bill provided by an ACLU of San Diego and Imperial Counties press
release includes:
ACLU of California,AIDS Legal Referral Panel,API Chaya,Anti Police-Terror Coalition,Asian
LawAlliance, CaliforniaAttorneys for Criminal Justice, California Immigrant Policy Center,
California Public DefendersAssociation, Citizens Rise!, Center for Media Justice, Coalition for
Humane Immigrant Rights, Color of Change, Council onAmerican-Islamic Relations –
California, CRASH Space, Data for Black Lives, Electronic Frontier Foundation, Fight for the
Future, Harvey Milk LGBTQ Democratic Club, Indivisible CA, Justice Teams Network, Media
Alliance, NationalAssociation of Criminal Defense Lawyers, National Center for Lesbian Rights,
Oakland Privacy, RAICES, README at UCLA, RootAccess, San Jose/Silicon Valley NAACP,
Secure Justice, Transgender Law Center, Library Freedom Project, Tor Project, and X-Lab.
114
The groups in support of the bill are largely citizen-focused groups. The citizens’ groups, led by
the ACLU, played two important roles. First, the groups raised the salience of the issue to
legislators and the public. Second, the ACLU directly helped draft the regulatory text, giving the
citizens’ group an important voice in the legislation.
By contrast, the law enforcement groups who opposed the regulatory change of FRT
through its use in police body cameras were the government consumers. The bill faced
opposition from:
California Peace OfficersAssociation, California Police ChiefsAssociation, California State
113 Irene Ho,
“Question onAB1215 2019 Version History, ” February 26, 2021. 114 “California Senate Votes to Block Face Recognition on Police Body Cameras, ”ACLU of San Diego and Imperial Counties, September 11, 2019, https://www.aclusandiego.org/en/news/california-senate-votes-block-face-recognition-police-body-cameras.
Sheriffs’Association, CSAC Excess InsuranceAuthority, LosAngeles County Sheriff Peace
Officers’Association of California, and Riverside Sheriffs’Association.
115
The influence of law enforcement interests is seen even in the Democratic legislators who broke
with their party on this vote. The California bill passed the state’s Senate and Assembly mostly
along partisan lines “with a few switched votes due to closeness of law enforcement
organizations”.116 An analysis of donor histories for the eight Democratic Assembly Members
opposing the bill uncovers direct donations from various “Peace Officers” associations to seven
of the Assembly Members.117 A robust overlap of interest groups opposing the bill and Assembly
Members who received donations from these interest groups voting against the bill and against
their own party is evident. While the present analysis does not account for the fraction of
Democratic Assembly Members who may have voted in favor of the regulatory bill and also
received donations from similarly aligned Peace Officers organizations, the results are suggestive
that the interests of the government in the form of police forces can make regulation difficult.
Overall, the successful state ban on police use of FRT in body cameras in California suggests
that citizens’ group activism helped a Democratic majority counter the government alignment
with business interest, leading to the passing of Assembly Bill 1215.
Adding to the outside pressures promoting regulation of FRT in Los Angeles County has
been extensive media coverage directly resulting in public activism that has led to regulation. An
exposé in the Los Angeles Times published in September 2020 directly led to the city’s Police
115 Evan Symon,
“Recap:AB 1215 Banning Facial Recognition From Police Body Cameras, ” California Globe, September 24, 2019, https://californiaglobe.com/section-2/recap-ab-1215-banning-facial-recognition-from-police-body-cameras/. 116 Symon. 117 “CaliforniaAB1215 | 2019-2020 | Regular Session, ” LegiScan, accessedApril 27, 2021, https://legiscan.com/CA/rollcall/AB1215/id/893587.
Commission “reviewing the LAPD's use of facial recognition technology”.118 The Los Angeles
Times exposé detailed the LAPD’s continued use of FRT with evidence showing that for a
decade the department had both misled the public on their use of the technology and completed
over 30,000 FRT searches.119 The exposé further promoted regulatory change indirectly by
animating activists and citizens’ groups to increase public pressure through “public records
requests, staged protests, and encourage[ing] members and supporters to attend live meetings and
call into virtual meetings of the Police Commission and other city bodies with oversight over the
LAPD”.120 This suggests that media coverage, a factor I did not consider in my theoretical
framework, created a window of opportunity for public activism and helped eventually bring
about regulatory change.
Media investigations also directly led to a policy change of police use of FRT in Los
Angeles County, thus providing another driver of regulation I did not consider in my theoretical
framework. The Los Angeles Police Commission in November 2020 announced a policy change
banning unapproved third-party FRT searches “after it was told that documents seen by Buzzfeed
News showed more than 25 LAPD employees had performed nearly 475 searches using
Clearview AI”. The reporting revealed the continued searching through the Clearview AI
121
platform by Los Angeles County law enforcement even though prior statements by the police
force explicitly stated Clearview AI to be out of use. This policy change demonstrates how the
122
118 Rector,
“Facial Recognition and the LAPD, ” March 31, 2021. 119 Kevin Rector and Richard Winton, “Despite Past Denials, LAPD Has Used Facial Recognition Software 30,000 Times in Last Decade, Records Show, ” LosAngeles Times, September 21, 2020, https://www.latimes.com/california/story/2020-09-21/lapd-controversial-facial-recognition-software. 120 Rector, “Facial Recognition and the LAPD, ” March 31, 2021. 121 Brianna Sacks, Ryan Mac, and Caroline Haskins, “LAPD Bans Use Of Commercial Facial Recognition, ” Buzzfeed News, November 17, 2020, https://www.buzzfeednews.com/article/briannasacks/lapd-banned-commercial-facial-recognition-clearview. 122 Sacks, Mac, and Haskins.
media are another outside group who have contributed to policy regarding FRT searches.
Because of the ability to bring attention to the police department, which was already under
scrutiny surrounding its use of FRT searches, media coverage directly pressured the Los Angeles
Police Commission into regulatory action.
The Los Angeles case study shows that successful regulation has been possible. In some
cases, citizens’ groups appear to have been key players in raising the salience of the issue in the
public and with elected leaders and in writing draft legislation. In other cases, media
organizations may have created a window of opportunity that spurred citizens’ groups into action
leading to regulation. In the final case of regulation, media coverage alone produced action.
In contrast to Los Angeles, regulatory efforts have been less successful in New York City.
Citizens’ groups have been interested in regulatory change, but outcomes have either been more
limited in scope of regulation or unsuccessful. A state bill proposing a ban on FRT in police body
cameras with similar language to the successful California bill failed to gain attention in New
York after its introduction in both 2019 as S6776 and 2020 as S7572. In 2020, the bill never
made it out of the Senate’s Internet and Technology committee in which it was proposed.123 The
NYCLU was directly involved in the writing of the bill in a similar role as to what occurred with
the ACLU in California. A complete list of advocacy groups supporting or opposing the bill is
124
not publicly available, but given the content of the bill and a statement by the NYCLU, it is
reasonable to assume that the types of groups supporting and opposing the bill were similar to
those in California.125 The failure of the bill might be explained by either of two factors or a
combination of both. First, partisanship might have played some role in the failure of the New
123 Hoylman, NY State Senate Bill S7572. 124 Ben Schaefer, “Northwestern Undergraduate Thesis Research, ”April 9, 2021.
125 Schaefer.
York bill. A vote by the Senate’s 2020 Internet and Technology Committee did not advance the
bill to the Senate floor, and the bill died in committee.126 The Committee’s Democratic chair,
Diane Savino, has been aligned with more conservative policies and political groups including
New York’s Independent Democratic Conference.127 The makeup of the leadership of the Senate
Internet and Technology Committee, although Democrat-led, may suggest an ideological or
partisan motive behind the Committee’s decision not to report the bill to the Senate floor. A
second explanation might be a lack of salience from the public. Without a large public interest in
the status of the state bill preventing FRT searches on police body camera footage, S6776 in
2019 and S7572 in 2020 might have failed to gain enough public attention to bring legislative
support.
There have been some partial regulatory successes in New York City. Media attention
leading to public activism has contributed to legislative efforts to increase the transparency of the
NYPD’s use of facial recognition. The NYPD voluntarily published its broad facial recognition
policy shortly after a longform New York Times piece written in January 2020 alleged the
department’s use of third-party company Clearview AI.128 Previously, the policy was not public.
The move to disclose present practices may have come from an increase in public attention to the
subject due to the exposé. The disclosure did not amount to major changes in practice or any
stronger regulation of the department’s use of the technology, but it does indicate the potential
power of public salience surrounding the issue. Later in 2020, the Public Oversight of
126 Hoylman, NY State Senate Bill S7572. 127 David Weigel, “Analysis | The End of New York’s ‘Independent Democrats, ’Explained, ” Washington Post,April 4, 2018, https://www.washingtonpost.com/news/powerpost/wp/2018/04/04/the-end-of-new-yorks-independent-democrats-ex plained/. 128 Kashmir Hill, “The Secretive Company That Might End Privacy as We Know It, ” The New York Times, January 18, 2020, 2, https://www.nytimes.com/2020/01/18/technology/clearview-privacy-facial-recognition.html.
Surveillance Technology (POST) Act, guaranteeing increased transparency of surveillance
practices, successfully passed the New York City Council mostly along partisan lines with a few
Democratic members voting against the bill.129 The NYCLU directly helped draft the bill in 2018
when it was introduced, and in June 2020 the group was involved in “shepherding” the bill
through the city council.130 Citizen support groups for the Act also included the Stop Spying
Coalition and Black Lives Matter. 131 Given the bill’s long-dormant status in the New York City
Council and subsequent successful passage following increased public salience on FRT use by
the NYPD as well as direct action by the NYCLU and other citizens’ groups, the role of citizen
activists in leading to the passing of the bill may have been significant. However, public salience
arose only after media coverage of the city’s FRT use and the department’s subsequent internal
policy change. This suggests that increased action by citizens’ groups may lead to greater
regulation in New York City in the future, but increased action may only occur when media
coverage is also involved.
A further challenge to regulation in both Los Angeles and New York City stems from the
federal structure of United States politics, another factor I did not consider in my theoretical
framework. Federal agencies continue to be a direct consumer of private FRT systems.
Therefore, the use of the technology in both cities extends beyond local or state functions as the
NYPD and the LAPD/LASD each directly outline their willingness to communicate and
cooperate with federal agencies to perform FRT searches in their respective official FRT policy
guidelines.132 Presently, no policy regulates federal practices regarding FRT. 133 A policy
129 Gibson, Creating comprehensive reporting and oversight of NYPD surveillance technologies. 130 Schaefer, “Northwestern Undergraduate Thesis Research, ”April 9, 2021.
131 Schaefer. 132 “Facial Recognition - NYPD”; “LACRIS Facial Recognition Policy. ” 133 Crawford and Schultz, “AI Systems as StateActors. ”
announced in early 2021 by the Los Angeles Police Commission prevents any officials from
running searches on third party platforms other than the three approved by the county. 134
However, the Los Angeles County policy explicitly states a willingness to cooperate with federal
investigations.135 This presents the opportunity for third-party searches to be run on images or
videos captured in Los Angeles County. The NYPD refused to publicly comment on their use of
third party searches and has to date not responded to a media request on the topic.136 The
department outlines an ability to use databases of images outside of mugshots “if there is a
legitimate need to do so”.137 Additionally, the NYPD states their willingness to cooperate in
federal investigations. As in Los Angeles County, federal cooperation explicitly allows images
and videos captured in New York City to be searched on third-party FRT platforms. Records
obtained through Freedom of Information Act (FOIA) requests by MuckRock confirm the use of
private FRT algorithms by specific federal agencies such as the FBI and Department of
Homeland Security with specific mentions of third-party company Clearview AI.138 The
Washington Post reports that the United States Immigration and Customs Enforcement (ICE)
runs FRT searches on millions of photos not included in mugshot databases.139 Cooperation with
federal officials in both cities thus directly undercuts local policies in each of the two cities
134 Kevin Rector,
“LAPD PanelApproves New Oversight of Facial Recognition, Rejects Calls to End Program, ” Los Angeles Times, January 13, 2021, https://www.latimes.com/california/story/2021-01-12/lapd-panel-approves-new-oversight-of-facial-recognition-rejec ts-calls-to-end-program. 135 “LACRIS Facial Recognition Policy. ” 136 Nober and NYPD Legal, NYPD Facial Recognition Third-Parties. 137 “Facial Recognition - NYPD. ” 138 Beryl Lipton, “Records on ClearviewAI Reveal New Info on Police Use, ” MuckRock, accessedApril 26, 2021, https://www.muckrock.com/news/archives/2020/jan/18/clearview-ai-facial-recogniton-records/. 139 Drew Harwell and Erin Cox, “ICE Has Run Facial-Recognition Searches on Millions of Maryland Drivers, ” Washington Post, February 26, 2020, https://www.washingtonpost.com/technology/2020/02/26/ice-has-run-facial-recognition-searches-millions-maryland -drivers/.
limiting facial searches to occur only or mostly within facial image databases created locally
from mugshot images.140 The setup of multiple levels of government each being direct
consumers of private technology limits the scope of regulation at any single level of government.
Nonetheless, the hesitation of both cities to take steps to fully prevent third-party searches, even
under public pressure, indicates an alignment of government interest opposed to that of the
public.
Implications
Significant barriers affect the ability to regulate the government’s use of FRT in law
enforcement. The speed of innovation of AI in general and FRT specifically along with rapidly
evolving applications of AI systems across diverse domains limit the scope of action responsibly
available to legislators. The role of both the NYPD and Los Angeles County as well as federal
agencies as direct consumers of private FRTs and subsequent lack of regulation in the face of
public outcry might indicate a reluctance to create additional internal restrictions. Interaction and
cooperation between law enforcement agencies at the local, state, and federal levels further
highlight regulatory challenges.
Regarding consumer advocacy, partial support exists for the argument that regulation of
government use of AI will only come when there is direct involvement by citizens’ groups.
California and subsequently the LAPD and LASD have seen some regulation limiting FRT use
by police departments as a direct result of citizens’ groups mobilizing to take advantage of the
window of opportunity. New York has not. Direct involvement by a host of citizens’ groups led
to the passing of a California law banning FRT in police body cameras while a similar law has
140 “LACRIS Facial Recognition Policy”; “Facial Recognition - NYPD. ”
failed to gain public attention in New York even with some degree of backing by citizens’
groups.
The Los Angeles and New York City case comparison suggests that public salience may
matter. The hypothesis on the importance of the role of citizens’ groups entailed two key
assumptions on consumer advocacy. First, citizens’ groups would increase the public salience of
the issue. Second, increased public salience would raise the costs of inaction for elected officials.
Analysis from the case studies uncovered instances where the first assumption, citizens’ groups
increasing public salience, proved pivotal to regulatory change. However, unaccounted for in the
hypothesis was the central role of the media in increasing public salience and in directly pushing
regulatory action. Public outcry instigated by a Los Angeles Times exposé led to demonstrations
by citizens’ groups against the LAPD and its FRT usage that ultimately brought regulatory
change in the city. Regulatory change in the city also came from a direct media investigation into
the LAPD. In New York City, media coverage leading to regulatory change and media coverage
leading to citizens’ group activism has driven city regulation of FRT focused on heightened
transparency. Regulating AI might require significant involvement from citizens’ groups
including raising the salience of the issue with elected officials and developing framework
legislation. However, citizens’ groups may not always be the primary actors pushing the public
salience. This step may need independent action from media organizations. While engagement
and pressure from citizen activists brought regulation in some instances, each city did see other
instances of regulatory action as solely a result of media involvement with no role played by
public activists. This suggests the role of citizens’ groups in being necessary for regulation of
government use of AI is incorrect. A further limit to testing the importance of citizens’ groups in
driving regulation related to AI is the lack of a high quantity of legislation written to date and the
resulting need to focus the analysis along regulatory outcomes rather than interest group
involvement. The second assumption in the hypothesis is that public salience would matter
because it raised the cost of inaction for electorally-focused officials. Successful regulatory
outcomes are consistent with that argument, but the case studies did not uncover any “smoking
gun” evidence that legislators were concerned about the electoral consequences of inaction.
As governing AI appears to fall somewhere between literature on public and private
regulation, political science frameworks and theories fail to fully explain the outcome. Aspects
of both types of regulation exist, with the role of interest groups in guiding legislative outcomes
and the role of the public in guiding industry practices. Because the government is a direct
consumer of the technology, these two understandings of regulation must expand to account for
cases currently missed by both.
A final, overarching finding in this study is an alarming lack of transparency. The level of
information unavailable presently in the field that contributes to a complexity in understanding.
The lack of laws allowing for clear public knowledge of present practices regarding FRT must be
immediately addressed. Police departments, having already developed a track record of
misguidance in the past decade, continue to mislead the public on their present usage of FRT.
While Los Angeles County and New York City have serious shortcomings in information
available, the two locales are far ahead of many major cities in the country that do not publish
any public information on FRT use. Preventing these gaps in information available to the
141
public and allowing for scrutiny of present practices first requires a level of transparency not yet
141 Garvie, Bedoya, and Frankle, “The Perpetual Line-Up. ”
guaranteed or seen today.
VI. Conclusion
Given the present macro-level changes across industries resulting from AI, conversations
on shifts in governing strategies must exist. To focus these conversations, this thesis considered
instances where the government directly consumes the technology. A case study of police use of
facial recognition searches first and foremost highlights the present lack of transparency limiting
the ability for public scrutiny of the technology. Beyond this inadvertent finding, initial insights
into the role of citizen activists in shaping the regulatory field along with the aligning interests of
legislators and corporate interests in the technology sector are explored. In California, activists
played some role in changing state and city policies while in New York, less change has to date
occurred. This suggests activism might play a role in advancing public interests in the regulation
of AI. Additionally, media organizations were found to drive regulation both directly and
indirectly through promoting citizens’ groups. Instances of each role played by the media were
seen in each city. Caution should be taken in generalizing from these findings. Not enough data
presently exists to effectively compare large-N sets of data on the regulation of AI in government
uses. Will regulation in the future address these ambitious emerging technologies head-on?
Existing political science literature does not fully address regulation concerning the
technology. Traditional models of public regulation through legislation or rulemaking explain
that legislators are influenced by both the public and through corporate interests. Literature on
private politics examines how activists can alter the behavior of firms through their purchasing
power as direct consumers. Neither body addresses cases where the government is the direct
consumer nor cases where the public is left without purchasing power. An assessment of industry
self-regulation provides little insight due to the prevalence of information held unavailable to the
public.
Optimism over emerging legislation at all levels of government provides a hopeful look
for the future. The Facial Recognition and Biometric Technology Moratorium Act of 2020, the
NationalArtificial Intelligence Initiative Act of 2020, and the FUTURE of Artificial Intelligence
Act of 2020 mark some of the 175 bills introduced in the 116th congressional session related to
AI.142 Although no bills to date have been signed into law at the federal level (excluding those
providing more research funding for the technology), the sheer amount of attention that
lawmakers have given to the subject must be seen as a recognition of present shortcomings.
Further excitement stems from scholarly insight into sweeping regulatory solutions. To
directly address concerns on input bias and prevent discrimination before it happens, solutions
from environmental governance can be applied by installing algorithmic impact assessments.143
The approach is based on environmental law solutions meant to mitigate possible irreversible
damage before physical development. The strategy applies directly to the regulation of AI
systems. Algorithmic impact assessments could mitigate certain concerns by providing a detailed
report of expectations for that system from rigorous testing. The proposed solution imagines a
144
more transparent rollout of AI systems. Another legal proposal calls for the application of the
state action doctrine to increase accountability towards AI systems performing roles of
governance.145 By arguing that AI systems performing the role of the state should be accountable
to “constitutional liability,” the present gap in accountability surrounding government use of AI
142 “Legislative Search Results, ” legislation, accessedApril 27, 2021, https://www.congress.gov/search. 143 Reisman et al., “Algorithmic ImpactAssessments:APractical Framework for PublicAgencyAccountability. ”
144 Reisman et al. 145 Crawford and Schultz, “AI Systems as StateActors. ”
systems could be bridged. As more AI systems impact more sectors, the amount of literature on
better governance should only increase.
Bibliography
“740 ILCS 14/ Biometric Information Privacy Act.” Accessed February 1, 2021.
https://www.ilga.gov/legislation/ilcs/ilcs3.asp?ActID=3004&ChapterID=57.
“2020 Election Results | New York State Board of Elections.” Accessed March 14, 2021.
https://www.elections.ny.gov/2020ElectionResults.html.
“About NYPD - NYPD.” Accessed March 15, 2021.
https://www1.nyc.gov/site/nypd/about/about-nypd/about-nypd-landing.page.
“Age Discrimination | U.S. Department of Labor.” Accessed April 28, 2021.
https://www.dol.gov/general/topic/discrimination/agedisc.
Anzia, Sarah F. “Election Timing and the Electoral Influence of Interest Groups.” The Journal of
Politics 73, no. 2 (April 1, 2011): 412–27. https://doi.org/10.1017/S0022381611000028.
The White House. “Artificial Intelligence for the American People.” Accessed October 11, 2020.
https://www.whitehouse.gov/ai/.
Auxier, Brooke, and Lee Rainie. “Key Takeaways on Americans’Views about Privacy,
Surveillance and Data-Sharing.” Pew Research Center (blog), November 15, 2019.
https://www.pewresearch.org/fact-tank/2019/11/15/key-takeaways-on-americans-views-a
bout-privacy-surveillance-and-data-sharing/.
Baron, David P. “Private Politics.” Journal of Economics & Management Strategy 12, no. 1
(2003): 31–66. https://doi.org/10.1111/j.1430-9134.2003.00031.x.
Baron, David P., and Daniel Diermeier. “Strategic Activism and Nonmarket Strategy.” Journal of
Economics & Management Strategy 16, no. 3 (2007): 599–634.
https://doi.org/10.1111/j.1530-9134.2007.00152.x.
Baumgartner, Frank R., Jeffrey M. Berry, Marie Hojnacki, David C. Kimball, and Beth L. Leech.
“Money, Priorities, and Stalemate: How Lobbying Affects Public Policy.” Election Law
Journal: Rules, Politics, and Policy 13, no. 1 (March 1, 2014): 194–209.
https://doi.org/10.1089/elj.2014.0242.
Benjamin, Ruha. “Race After Technology.” Ruha Benjamin. Accessed July 17, 2020.
https://www.ruhabenjamin.com/race-after-technology.
Buolamwini, Joy. “Gender Shades.” MIT Media Lab, 2018.
https://www.media.mit.edu/projects/gender-shades/overview/.
Burstein, P., and A. Linton. “The Impact of Political Parties, Interest Groups, and Social
Movement Organizations on Public Policy: Some Recent Evidence and Theoretical
Concerns.” Social Forces 81, no. 2 (December 1, 2002): 380–408.
https://doi.org/10.1353/sof.2003.0004.
Butler, Daniel M., and David W. Nickerson. “Can Learning Constituency Opinion Affect How
Legislators Vote? Results from a Field Experiment.” Quarterly Journal of Political
Science 6, no. 1 (August 22, 2011): 55–83. https://doi.org/10.1561/100.00011019.
Cain, Josh. “LAPD Commission OKs Detectives to Use Facial Recognition.” Government
Technology, January 14, 2021.
https://www.govtech.com/public-safety/LAPD-Commission-OKs-Detectives-to-Use-Faci
al-Recognition.html.
LegiScan. “California AB1215 | 2019-2020 | Regular Session.” Accessed April 27, 2021.
https://legiscan.com/CA/rollcall/AB1215/id/893587.
ACLU of San Diego and Imperial Counties. “California Senate Votes to Block Face Recognition
on Police Body Cameras,” September 11, 2019.
https://www.aclusandiego.org/en/news/california-senate-votes-block-face-recognition-pol
ice-body-cameras.
Calo, Ryan, Madeline Clare Elish, Todd Murphey, and Dan Linna. “Autonomous Systems
Failures: Who Is Legally and Morally Responsible?” November 18, 2020.
https://www.law.northwestern.edu/student-life/events/eventsdisplay.cfm?i=176039&c=2.
Carrigan, Christopher, and Cary Coglianese. “The Politics of Regulation: From New
Institutionalism to New Governance.” SSRN Scholarly Paper. Rochester, NY: Social
Science Research Network, June 1, 2011.
https://doi.org/10.1146/annurev.polisci.032408.171344.
Cath, Corinne, Sandra Wachter, Brent Mittelstadt, Mariarosaria Taddeo, and Luciano Floridi.
“Artificial Intelligence and the ‘Good Society’: The US, EU, and UK Approach.” Science
and Engineering Ethics 24, no. 2 (April 1, 2018): 505–28.
https://doi.org/10.1007/s11948-017-9901-7.
Cellan-Jones, Rory. “Uber’s Self-Driving Operator Charged over Fatal Crash.” BBC News,
September 16, 2020, sec. Technology. https://www.bbc.com/news/technology-54175359.
Chang, Elizabeth. “MyPillow Boycott: How a Product Can Spark an Identity Crisis.” Washington
Post. Accessed March 7, 2021.
https://www.washingtonpost.com/lifestyle/wellness/my-pillow-lindell-boycott-customers/
2021/02/12/7399aaa4-6af1-11eb-9ead-673168d5b874_story.html.
Chui, Michael, James Manyika, Mehdi Miremadi, Nicolaus Henke, Rita Chung, Pieter Nel, and
Sankalp Malhotra. “Notes from the AI Frontier: Applications and Value of Deep
Learning.” McKinsey, April 17, 2018.
https://www.mckinsey.com/featured-insights/artificial-intelligence/notes-from-the-ai-fron
tier-applications-and-value-of-deep-learning.
Conger, Kate, Richard Fausset, and Serge F. Kovaleski. “San Francisco Bans Facial Recognition
Technology.” The New York Times, May 14, 2019, sec. U.S.
https://www.nytimes.com/2019/05/14/us/facial-recognition-ban-san-francisco.html.
County of Los Angeles. “Sheriff’s Department.” Government, November 15, 2016.
https://lacounty.gov/residents/public-safety/sheriff/.
Crawford, Kate, and Jason Schultz. “AI Systems as State Actors.” Columbia Law Review, 2019.
https://columbialawreview.org/content/ai-systems-as-state-actors/.
Cummings, Elijah. Facial Recognition Technology (Part II): Ensuring Transparency in
Government Use, § House Oversight Committee (2019).
Davidson, Roger H., Walter J. Oleszek, Frances E. Lee, and Eric Schickler. “Leaders and Parties
in Congress.” In Congress and Its Members, 16th ed. CQ Press, 2017.
Pew Research Center: Internet, Science & Tech. “Demographics of Internet and Home
Broadband Usage in the United States,” June 12, 2019.
https://www.pewresearch.org/internet/fact-sheet/internet-broadband/.
Druckman, James N., and Julia Valdes. “How Private Politics Alters Legislative
Responsiveness.” Quarterly Journal of Political Science 14, no. 1 (January 1, 2019):
115–30. https://doi.org/10.1561/100.00018066.
Endres, Kyle, and Costas Panagopoulos. “Boycotts, Buycotts, and Political Consumerism in
America.” Sage Journals 4, no. 4 (November 1, 2017).
https://journals.sagepub.com/doi/full/10.1177/2053168017738632.
“Facial Recognition - NYPD.” Accessed March 15, 2021.
https://www1.nyc.gov/site/nypd/about/about-nypd/equipment-tech/facial-recognition.pag
e.
Feldstein, Steven. “The Global Expansion of AI Surveillance.” Carnegie Endowment for
International Peace, September 17, 2019.
https://carnegieendowment.org/2019/09/17/global-expansion-of-ai-surveillance-pub-7984
7.
Fellowes, Matthew, Virginia Gray, and David Lowery. “What’s on the Table? The Content of
State Policy Agendas.” Party Politics 12, no. 1 (January 1, 2006): 35–55.
https://doi.org/10.1177/1354068806059246.
Figueiredo, Rui J. P. De, and Geoff Edwards. “Does Private Money Buy Public Policy?
Campaign Contributions and Regulatory Outcomes in Telecommunications.” Journal of
Economics & Management Strategy 16, no. 3 (2007): 547–76.
https://doi.org/10.1111/j.1530-9134.2007.00150.x.
Flynn, Shannon. “13 Cities Where Police Are Banned From Using Facial Recognition Tech.”
Innovation & Tech Today (blog), November 18, 2020.
https://innotechtoday.com/13-cities-where-police-are-banned-from-using-facial-recogniti
on-tech/.
Fouirnaies, Alexander. “When Are Agenda Setters Valuable?” American Journal of Political
Science 62, no. 1 (2018): 176–91. https://doi.org/10.1111/ajps.12316.
Fowler, Patrick, and Haley Breedlove. “Facing the Issue: San Francisco Bans City Use of Facial
Recognition Technology.” JD Supra, July 15, 2019.
https://www.jdsupra.com/legalnews/facing-the-issue-san-francisco-bans-35144/.
Garvie, Clare, Alvaro Bedoya, and Jonathan Frankle. “The Perpetual Line-Up.” Perpetual Line
Up, October 18, 2016. https://www.perpetuallineup.org/.
Gascón, George. “COMPSTAT Plus - Los Angeles Police Department.” Accessed March 15,
2021. https://www.lapdonline.org/inside_the_lapd/content_basic_view/6364.
Gibson, Vanessa. Creating comprehensive reporting and oversight of NYPD surveillance
technologies., Pub. L. No. Int 0487-2018 (2020).
https://legistar.council.nyc.gov/LegislationDetail.aspx?ID=3343878&GUID=996ABB2A
-9F4C-4A32-B081-D6F24AB954A0.
Goodwin, Gretta. “Face Recognition Technology.” Testimony Before the Committee on
Oversight and Reform, House of Representatives, June 4, 2019.
Grother, Patrick J., Mei L. Ngan, and Kayee K. Hanaoka. “Ongoing Face Recognition Vendor
Test (FRVT) Part 2: Identification,” November 27, 2018.
https://www.nist.gov/publications/ongoing-face-recognition-vendor-test-frvt-part-2-identi
fication.
Grother, Patrick, Mei Ngan, and Kayee Hanaoka. “Face Recognition Vendor Test Part 3:
Demographic Effects.” Gaithersburg, MD: National Institute of Standards and
Technology, December 2019. https://doi.org/10.6028/NIST.IR.8280.
Hardesty, Larry. “Explained: Neural Networks.” MIT News | Massachusetts Institute of
Technology, April 14, 2017.
https://news.mit.edu/2017/explained-neural-networks-deep-learning-0414.
Harwell, Drew, and Erin Cox. “ICE Has Run Facial-Recognition Searches on Millions of
Maryland Drivers.” Washington Post, February 26, 2020.
https://www.washingtonpost.com/technology/2020/02/26/ice-has-run-facial-recognition-s
earches-millions-maryland-drivers/.
Heilweil, Rebecca. “Artificial Intelligence Will Help Determine If You Get Your next Job.” Vox,
December 12, 2019.
https://www.vox.com/recode/2019/12/12/20993665/artificial-intelligence-ai-job-screen.
Hill, Kashmir. “The Secretive Company That Might End Privacy as We Know It.” The New
York Times, January 18, 2020.
https://www.nytimes.com/2020/01/18/technology/clearview-privacy-facial-recognition.ht
ml.
“Wrongfully Accused by an Algorithm.” The New York Times, June 24, 2020, sec. Technology.
https://www.nytimes.com/2020/06/24/technology/facial-recognition-arrest.html.
Ho, Daniel E. “Developing Better, Less-Biased Facial Recognition Technology.” Stanford
Human-Centered Artificial Intelligence, November 9, 2020.
https://hai.stanford.edu/blog/developing-better-less-biased-facial-recognition-technology.
Ho, Irene. “Question on AB1215 2019 Version History,” February 26, 2021.
Hojnacki, Marie, David C Kimball, Frank R Baumgartner, Jeffrey M Berry, and Beth L Leech.
“Studying Organizational Advocacy and Influence: Reexamining Interest Group
Research.” Annual Review of Political Science 15 (June 2012): 379–99.
Hoylman, Brad. NY State Senate Bill S6776, Pub. L. No. S6776 (2019).
https://www.nysenate.gov/legislation/bills/2019/s6776.
Hunt, Elle. “Tay, Microsoft’s AI Chatbot, Gets a Crash Course in Racism from Twitter.” the
Guardian, March 24, 2016.
http://www.theguardian.com/technology/2016/mar/24/tay-microsofts-ai-chatbot-gets-a-cr
ash-course-in-racism-from-twitter.
Issacharoff, Samuel, and Jeremy Peterman. “Special Interests After Citizens United: Access,
Replacement, and Interest Group Response to Legal Change.” Annual Review of Law and
Social Science 9, no. 1 (2013): 185–205.
https://doi.org/10.1146/annurev-lawsocsci-102612-133930.
Johansen, Alison Grace. “Deepfakes: What They Are and Why They’re Threatening.”
NortonLifeLock, July 24, 2020.
https://us.norton.com/internetsecurity-emerging-threats-what-are-deepfakes.html.
Johnson, Patricia M., Michael P. Recht, and Florian Knoll. “Improving the Speed of MRI with
Artificial Intelligence.” Seminars in Musculoskeletal Radiology 24, no. 1 (February
2020): 12–20. https://doi.org/10.1055/s-0039-3400265.
Kafeero, Stephen. “Uganda Is Using Huawei’s Facial Recognition Tech to Crack down on
Dissent after Protests.” Quartz Africa, November 27, 2020.
https://qz.com/africa/1938976/uganda-uses-chinas-huawei-facial-recognition-to-snare-pr
otesters/.
Kang, Daniel. “Chinese ‘gait Recognition’Tech IDs People by How They Walk.” AP NEWS,
November 6, 2018. https://apnews.com/article/bf75dd1c26c947b7826d270a16e2658a.
Karbassi, Ali. “Mission.” We All Code. Accessed April 26, 2021.
https://www.weallcode.org/our-story/.
Kimball, David C, Frank R Baumgartner, Jeffrey M Berry, Marie Hojnacki, Beth L Leech, and
Bryce Summary. “Who Cares about the Lobbying Agenda?” Interest Groups & Advocacy
1, no. 1 (May 1, 2012): 5–25. https://doi.org/10.1057/iga.2012.7.
King, Andrew, Andrea Prado, and Jorge Rivera. “Industry Self-Regulation and Environmental
Protection.” The Oxford Handbook of Business and the Natural Environment, November
2011.
https://www-oxfordhandbooks-com.turing.library.northwestern.edu/view/10.1093/oxford
hb/9780199584451.001.0001/oxfordhb-9780199584451-e-6.
Klontz, Joshua, and Anubhav Jain. “A Case Study of Automated Face Recognition: The Boston
Marathon Bombings Suspects.” Computer 46 (November 1, 2013): 91–94.
https://doi.org/10.1109/MC.2013.377.
“LACRIS Facial Recognition Policy,” 2019.
“LAPD Panel Approves New Oversight of Facial Recognition, Rejects Calls to End Program.”
Los Angeles Times, January 13, 2021.
https://www.latimes.com/california/story/2021-01-12/lapd-panel-approves-new-oversight
-of-facial-recognition-rejects-calls-to-end-program.
“Legislation Related to Artificial Intelligence.” Accessed March 11, 2021.
https://www.ncsl.org/research/telecommunications-and-information-technology/2020-legi
slation-related-to-artificial-intelligence.aspx.
“Legislative Search Results.” Legislation. Accessed April 27, 2021.
https://www.congress.gov/search.
Lipton, Beryl. “Records on Clearview AI Reveal New Info on Police Use.” MuckRock.
Accessed April 26, 2021.
https://www.muckrock.com/news/archives/2020/jan/18/clearview-ai-facial-recogniton-re
cords/.
Loukissas, Yanni Alexander. All Data Are Local: Thinking Critically in a Data-Driven Society.
1st ed. The MIT Press, 2019. https://mitpress.mit.edu/books/all-data-are-local.
Martin, Michael. “Rural and Lower-Income Counties Lag Nation in Internet Subscription.” The
United States Census Bureau, December 6, 2018.
https://www.census.gov/library/stories/2018/12/rural-and-lower-income-counties-lag-nati
on-internet-subscription.html.
Materese, Robin. “NIST Evaluation Shows Advance in Face Recognition Software’s
Capabilities.” Text. NIST, November 30, 2018.
https://www.nist.gov/news-events/news/2018/11/nist-evaluation-shows-advance-face-rec
ognition-softwares-capabilities.
McGhee, Eric. “California’s Political Geography 2020.” Public Policy Institute of California,
February 2020. https://www.ppic.org/publication/californias-political-geography/.
Mulligan, Deirdre K., Joshua A. Kroll, Nitin Kohli, and Richmond Y. Wong. “This Thing Called
Fairness: Disciplinary Confusion Realizing a Value in Technology.” Proceedings of the
ACM on Human-Computer Interaction 3, no. CSCW (November 7, 2019): 119:1-119:36.
https://doi.org/10.1145/3359221.
Ngan, Mei L., Patrick J. Grother, Kayee K. Hanaoka, and Jason M. Kuo. “Face Recognition
Vendor Test (FRVT) Part 4: MORPH - Performance of Automated Face Morph
Detection,” March 6, 2020.
https://www.nist.gov/publications/face-recognition-vendor-test-frvt-part-4-morph-perfor
mance-automated-face-morph.
Nober, Benjamin, and NYPD Legal. NYPD Facial Recognition Third-Parties, 2021.
NY State Senate Bill S7572, Pub. L. No. S7572 (2020).
https://www.nysenate.gov/legislation/bills/2019/s7572.
O’Carroll, Brodie. “What Are the 3 Types of AI? A Guide to Narrow, General, and Super
Artificial Intelligence.” Codebots, October 24, 2017.
https://codebots.com/artificial-intelligence/the-3-types-of-ai-is-the-third-even-possible.
Plenke, Max. “The Reason This ‘Racist Soap Dispenser’ Doesn’t Work on Black Skin.” Mic,
2015.
https://www.mic.com/articles/124899/the-reason-this-racist-soap-dispenser-doesn-t-work
-on-black-skin.
Powell, Eleanor Neff, and Justin Grimmer. “Money in Exile: Campaign Contributions and
Committee Access.” The Journal of Politics 78, no. 4 (August 3, 2016): 974–88.
https://doi.org/10.1086/686615.
“Principles for Accountable Algorithms and a Social Impact Statement for Algorithms: FAT
ML.” Accessed March 11, 2021.
https://www.fatml.org/resources/principles-for-accountable-algorithms.
Rector, Kevin. “Connecting for Honors Thesis Research,” March 31, 2021.
Rector, Kevin, and Richard Winton. “Despite Past Denials, LAPD Has Used Facial Recognition
Software 30,000 Times in Last Decade, Records Show.” Los Angeles Times, September
21, 2020.
https://www.latimes.com/california/story/2020-09-21/lapd-controversial-facial-recognitio
n-software.
Reid, Erin M., and Michael W. Toffel. “Responding to Public and Private Politics: Corporate
Disclosure of Climate Change Strategies.” Strategic Management Journal 30, no. 11
(2009): 1157–78. https://doi.org/10.1002/smj.796.
Reisman, Dillon, Jason Schultz, Kate Crawford, and Meredith Whittaker. “Algorithmic Impact
Assessments: A Practical Framework for Public Agency Accountability.” Impact
Assessment 13, no. 1 (April 2018): 3–30.
https://doi.org/10.1080/07349165.1995.9726076.
Reynolds, Ryan. “Courts and Lawyers Struggle with Growing Prevalence of Deepfakes.”
Stanford Law School, June 9, 2020.
https://law.stanford.edu/press/courts-and-lawyers-struggle-with-growing-prevalence-of-d
eepfakes/.
Richardson, Rashida, Jason M Schultz, and Vincent M Southerland. “Litigating Algorithms 2019
US Report.” AI Now Institute: New York University, September 2019.
Sacks, Brianna, Ryan Mac, and Caroline Haskins. “LAPD Bans Use Of Commercial Facial
Recognition.” Buzzfeed News, November 17, 2020.
https://www.buzzfeednews.com/article/briannasacks/lapd-banned-commercial-facial-reco
gnition-clearview.
Schaefer, Ben. “Northwestern Undergraduate Thesis Research,” April 9, 2021.
Schwalbe, Craig. “A Meta-Analysis of Juvenile Justice Risk Assessment Instruments: Predictive
Validity by Gender.” Criminal Justice and Behavior 35 (July 30, 2008): 1367–81.
https://doi.org/10.1177/0093854808324377.
Seawright, Jason, and John Gerring. “Case Selection Techniques in Case Study Research: A
Menu of Qualitative and Quantitative Options.” Political Research Quarterly 61, no. 2
(June 1, 2008): 294–308. https://doi.org/10.1177/1065912907313077.
Smeets, Dirk, Peter Claes, Dirk Vandermeulen, and John Gerald Clement. “Objective 3D Face
Recognition: Evolution, Approaches and Challenges.” Forensic Science International
201, no. 1–3 (September 10, 2010): 125–32.
https://doi.org/10.1016/j.forsciint.2010.03.023.
Snow, Jacob. “Amazon’s Face Recognition Falsely Matched 28 Members of Congress With
Mugshots.” American Civil Liberties Union, July 26, 2018.
https://www.aclu.org/blog/privacy-technology/surveillance-technologies/amazons-face-re
cognition-falsely-matched-28.
Strauss, Howard. “The Future of the Web, Intelligent Devices, and Education,” 2007.
https://er.educause.edu/articles/2007/1/the-future-of-the-web-intelligent-devices-and-educ
ation.
Symon, Evan. “Recap: AB 1215 Banning Facial Recognition From Police Body Cameras.”
California Globe, September 24, 2019.
https://californiaglobe.com/section-2/recap-ab-1215-banning-facial-recognition-from-poli
ce-body-cameras/.
Tankovska, H. “Daily Social Media Usage Worldwide.” Statista, February 8, 2021.
https://www.statista.com/statistics/433871/daily-social-media-usage-worldwide/.
Ting, Phil. Law enforcement: facial recognition and other biometric surveillance, Pub. L. No. AB
1215 (2019).
https://leginfo.legislature.ca.gov/faces/billTextClient.xhtml?bill_id=201920200AB1215.
UW Video. Kate Crawford | AI Now: Social and Political Questions for Artificial Intelligence,
2018. https://www.youtube.com/watch?v=a2IT7gWBfaE&ab_channel=UWVideo.
Villasenor, John. “Artificial Intelligence and Bias: Four Key Challenges.” Brookings (blog),
January 3, 2019.
https://www.brookings.edu/blog/techtank/2019/01/03/artificial-intelligence-and-bias-four
-key-challenges/.
Villasenor, John, and Alicia Solow-Niederman. Holding Algorithms Accountable, April 23,
2020.
https://www.youtube.com/watch?v=EcF-FtXx_q8&ab_channel=UCLASchoolofLaw.
Vogel, David. “Private Global Business Regulation.” Annual Review of Political Science 11
(June 6, 2008). https://doi.org/10.1146/annurev.polisci.11.053106.141706.
Webb, Shelby. “Houston Teachers to Pursue Lawsuit over Secret Evaluation System.”
HoustonChronicle.com, May 12, 2017.
https://www.houstonchronicle.com/news/houston-texas/houston/article/Houston-teachers-
to-pursue-lawsuit-over-secret-11139692.php.
Weigel, David. “Analysis | The End of New York’s ‘Independent Democrats,’ Explained.”
Washington Post, April 4, 2018.
https://www.washingtonpost.com/news/powerpost/wp/2018/04/04/the-end-of-new-yorks-
independent-democrats-explained/.
Yackee, Susan Webb. “The Politics of Rulemaking in the United States.” SSRN Scholarly Paper.
Rochester, NY: Social Science Research Network, May 1, 2019.
https://doi.org/10.1146/annurev-polisci-050817-092302.