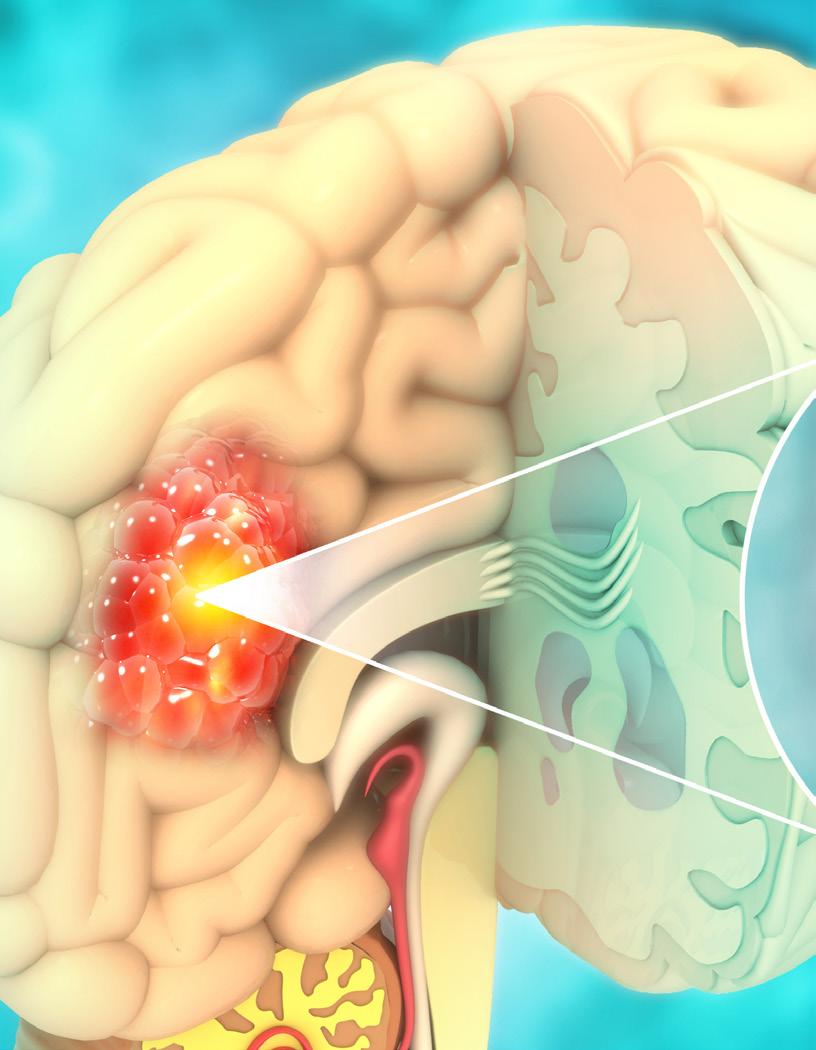
4 minute read
Predicting with precision
Machine learning maps pathways of tumour growth
BY ROBERT PRICE
Advertisement
Tumours grow in funny ways for lots of reasons. Brain geometry and skull density play a part in determining where a tumour decides to grow. Gravity, too, gives them direction: humans spend most of their lives on two feet, so tumours often tend to reach down into the brain. Add to these factors the complexity produced by blood vessels and how tumours access nutrients and oxygen and their structure—and future structure—boggles the mind.
The unpredictability of tumour growth has long been a challenge for oncologists. If clinicians could determine in which direction and at what rate a tumour is most likely to grow, they could craft more precise treatments and time interventions to maximize damage to the cancer. While not exactly the holy grail of cancer research—that would be
“the cure”—precision mapping of tumour growth is a step towards better treatment, if not the grail, and one made by advances developed by researchers in Canada.
Mapping the Brain
Glioblastoma multiforme (GBM) is a particularly nasty— and common—type of brain cancer. Survival time is around two years, tumours are hard to treat with drugs, and surgery is difficult since the fast-growing tumours can grow in parts of the brain that are impossible to reach.
In a new paper published in the Journal of Theoretical Biology, Cam Meaney, a PhD candidate at the University of Waterloo working in the field of mathematical oncology, along with his colleagues, has demonstrated that mathematical models can predict the progression of GBM. In the study, the researchers took diffusion weighted imaging of brain tumours and fed these into a machine learning algorithm called “the proliferation-invasion model (PI)” that made predictions using patient-specific parameters to deliver patient-specific projections of the future growth of the tumour.
The work began with what Meaney calls “fake brains”— synthetic, computationally generated brains that allowed the researchers to study tumour growth. The researchers— Cameron Meaney and Mohammad Kohandel at the University of Waterloo, and Sunit Das and Errol Colak at the University of Toronto and St. Michael’s Hospital—then applied the model to the clinical data of five patients diagnosed with GBM.
Using the modelling, the researchers were able to “derive evidence-based estimates for each of the PI model parameters and predictions for the future progression of the tumour, along with estimates of the parameter uncertainties.”
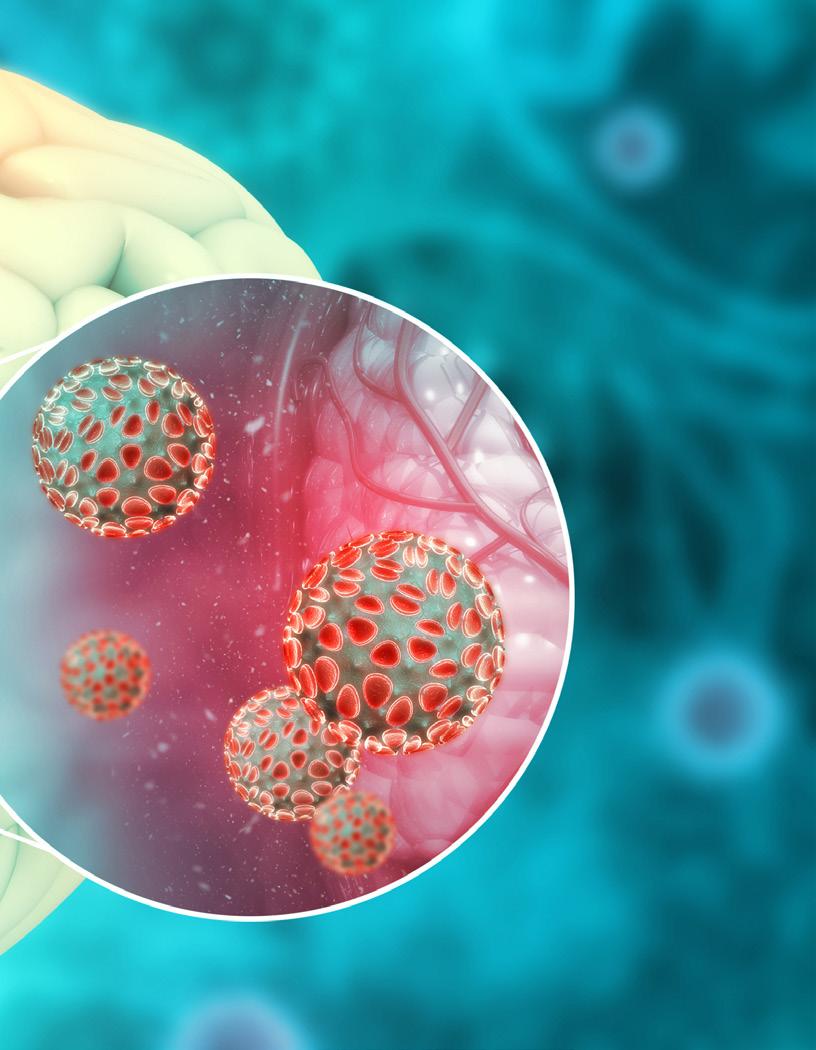
How the research plays out in the lab involves studying MRI images of brain scans and other inputs that are then fed into the algorithm coded in Python. (Samples of this code are available to researchers on Meaney’s GitHub page.) A range of inputs and possibilities are added to the scenarios—like the size of the brain, the size of the tumour, its placement, and other factors related to the patient’s health—and the machine “grows” the tumour on the screen through a series of iterations.
The algorithms have proven to be “extremely accurate within percentages of a percentage” at predicting growth of computationally-generated tumours, Meaney says. As for how these predictions will work on real patients, he says the work is developing. “It’s very difficult to assess true accuracy in a clinical setting, but based on the sort of roundabout methods that we tend to use in the field for assessing that, it’s quite accurate.”
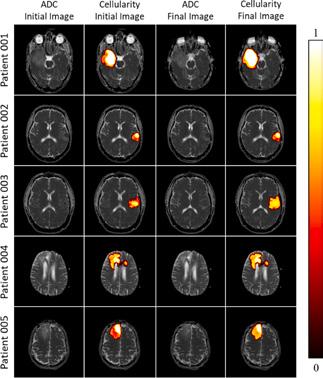
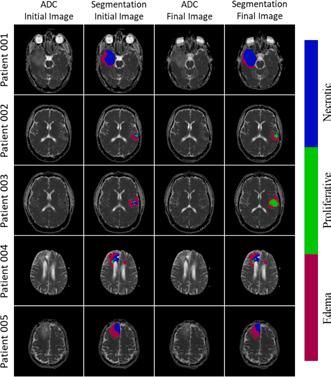
As a tool, deep learning, also called machine learning, can do more sophisticated calculations than any previous and tedious ways that researchers modelled tumour growth. “There were methods to get estimation parameters before, but they were a little, I don’t want to say primitive, because they’re quite complex, but they certainly did not incorporate anywhere near the amount of information that was available,” explains Meaney.
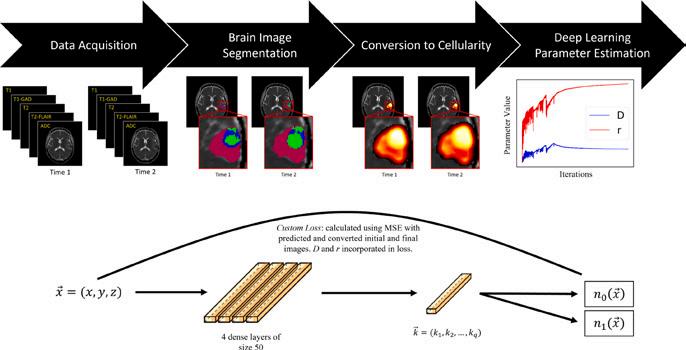
Personalizing Medicine
The study shows that mathematical tools can allow for more personalised predictions of brain tumour progression that were otherwise impossible. Instead of basing predictions on groupings of demographics, these tools allow researchers and clinicians to tailor their understanding of the disease patient-to-patient. As a tool for disease management, accurate predictions have the potential to transform treatment plans for some patients if clinicians are able to understand how big a tumour might get, how aggressive treatment should be, what sort of treatments to deploy and when, and more. To take one example, intertumoural pressure, the level of fluid pressure in the tissue, happens to be a key biomarker in patient prognosis, but it’s challenging to estimate, even though the phenomenon is well-understood. “But with a method like this,” says Meaney, “there is a path to estimating that pressure in a patient-specific way.”
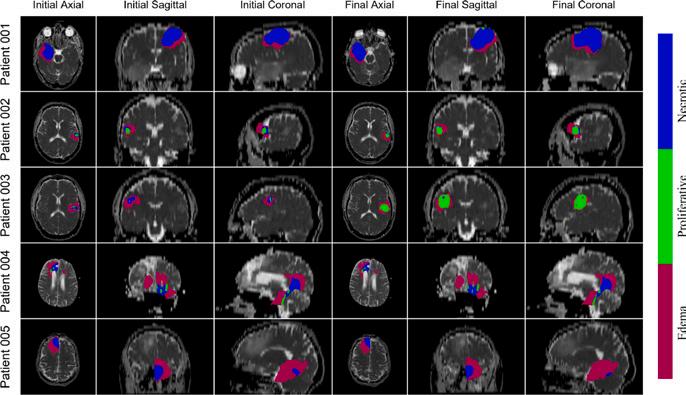
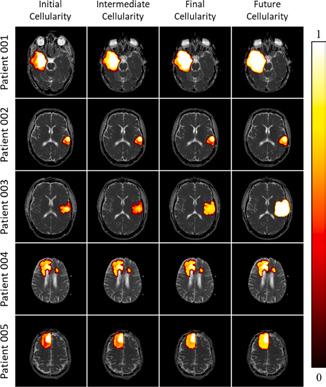
The current study didn’t include any treatments. “We were just doing the sort of characterization of the tumour itself,” explains Meaney. “The logic we’ve demonstrated in this work could totally be extended to include treatments, and that is something we’re actively looking into. That’s the obvious next step with this work.”
Teams
Work like this study highlights the highly collaborative nature of fields like mathematical oncology, which relied on the work of mathematicians, a surgeon, a radiologist, and computer scientists. “No one does any of this stuff alone,” says Meaney. “It’s extremely interdisciplinary.”
The work also reinforces the benefit of bringing quantitative researchers into other fields, like biology, where once upon a time they were strangers. “Quantitative sciences in, not only oncology, but medicine in general, are of tremendous benefit,” says Meaney. “And it’s obvious to those in the field that the more we go in this direction and incorporate these sorts of tools in our analyses, the better understanding we will have with these diseases and the better we’ll be able to treat and prevent them.”
New Tools, New Discoveries
Mathematical oncology is a burgeoning field dedicated to taking theoretical mathematics out of the esoterica of the university and using mathematical tools and artificial intelligence to optimize drug sequencing, drug combinations, radiation therapy, and immunotherapy in cancer treatment. Quantitative medicine, of which mathematical oncology is but a small subsection, is growing quickly as a field as more companies recognize the muscle power quantitative modelling teams can bring to biologists and experimentalists trying to answer difficult questions.
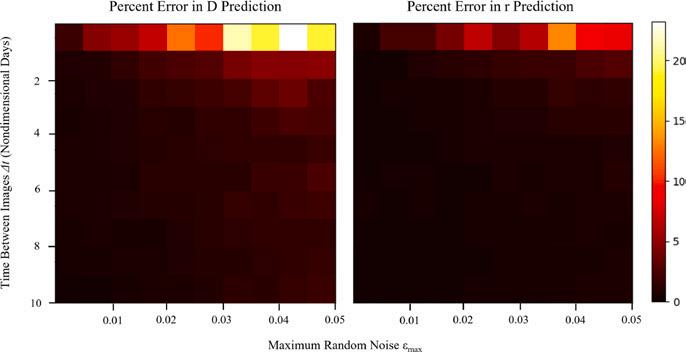
Cam Meaney, a PhD candidate at the University of Waterloo working in the field, says mathematical oncology is a product of the rapid development of the physical sciences in the 20th Century. Relativity and quantum theory and many other discoveries, can be largely attributed to the invention of sophisticated quantitative tools. Here in the 21st century, the same thing is happening in the biological sciences, as new quantitative tools, like machine learning, artificial intelligence, and diagnostic tools, improve predictions and make advances possible.
“And so for us doing that kind of research, it oftentimes feels like a lot of easy pickings,” says Meaney. “You stumble across problems and it’s just like, oh wow, I can’t believe this hasn’t been investigated quantitatively in a more comprehensive way, and yeah, those investigations, for the most part, have really borne awesome fruit.”