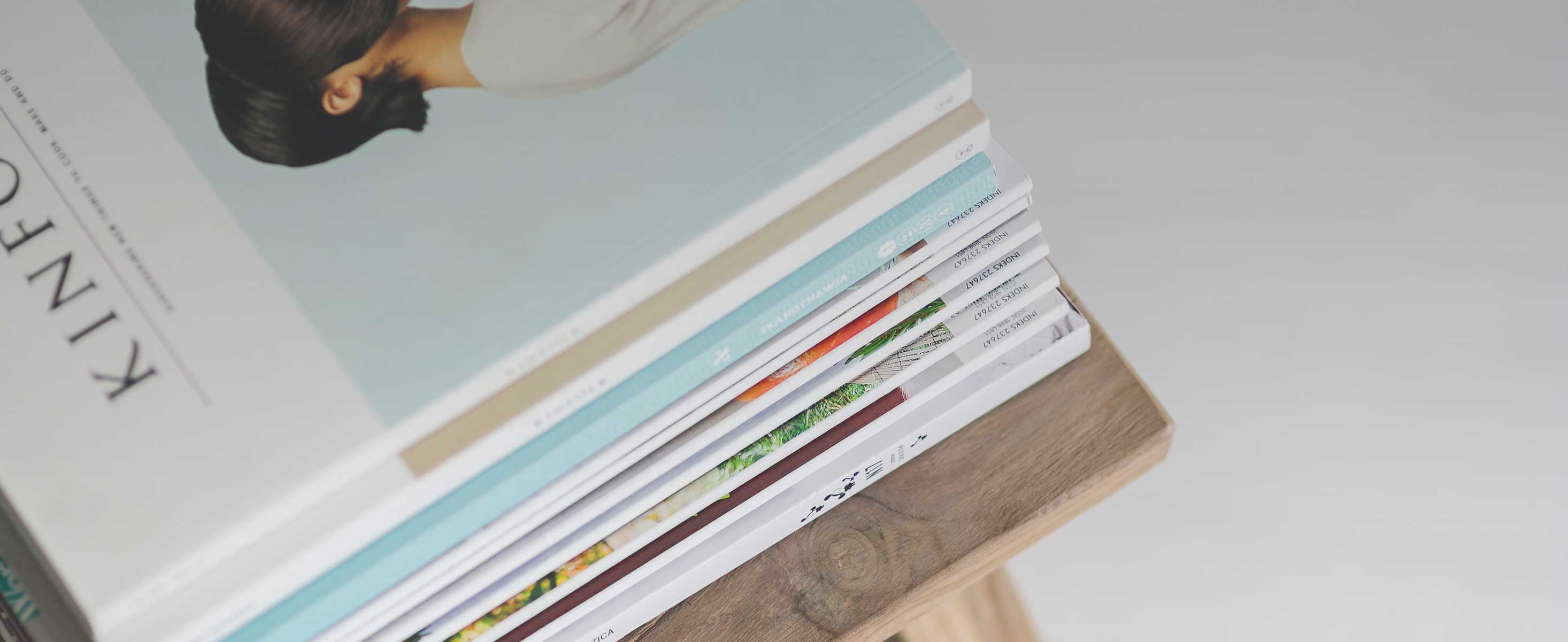
9 minute read
What’s the point in a point estimate?
What do percentage figures mean when stating prospects?
More than once, having drafted legal advice and distilled my opinion on prospects of success into a percentage Alexander MacMillan figure, the client has come back to me and questioned how I arrived at it.
The simple answer is (or in my case was) that a percentage figure is desirable since language can be ambiguous. I read with interest the CIA’s online Intelligence Community Directive 203 on Analytic Standards: paragraph 6(2)(a) of that Directive sets out difficulties arising from purely verbal advice, even seeking to align particular phrases with percentage figures. ‘Analytic products’ have particular wording mandated: “unlikely” must be used for a level of confidence in the 20-45% range, and so on. The Directive is at pains to discourage careless use of language. A percentage figure does the same job as a verbal expression of belief, but with less room for misunderstanding than words cause.
Of course, the fact that a number is apparently unambiguous should not be taken to imply that the underlying opinion enjoys any privileged status akin to mathematical certainty. Rather, a percentage is an alternative or a complementary way of expressing strength of belief. The number still simply expresses a subjective probability. That, at least, is what I replied when a client asked me to disclose my calculations for arriving at a percentage figure. In general terms, I was correct. But in hindsight I realised this answer was also inadequate to the question posed.
Beyond reasonable prospects
In my own experience, the niceties of probabilistic thinking and statistical analysis did not get covered in Bar School. In the real world, a common legal convention is that the client (and always an insurer client making a funding decision) needs to know if a legal case enjoys ‘reasonable prospects’, which – in percentage terms – is interpreted as being 51% or above. In other words: is it a green light or a red light from Counsel? The latter ‘red light’ scenario provides a high-value off-ramp for the insurer.
The highly simplified form of question makes the task of the advisor more straightforward – there is no need to go into subtle reasoning about why one alights on a particular percentage figure, beyond rehearsing what law is engaged, and stating whether the case is more likely to win or lose. Does the client engage forwards gear or reverse?
Maybe that approach to legal advice works sufficiently well in the aggregate, for the insurer’s purposes. But clients investing their own money and reputation should rightly demand much more detail: ideally the opinion of any subject matter expert should be more informative than tossing a coin (see, for example, Gardner, D. and Tetlock, P.E.’s Super forecasting: The Art and Science of Prediction Random House, 2015 where special emphasis is placed on the value-added of precision in any percentage figure when making a forecast).
If legal advice is that prospects hover around 50%, the advisor benefits from being right or nearly right irrespective of the outcome, and the client is left frustrated. What value, in fact, did expensive legal advice add? As the CIA director James Clapper notes in his Directive, “Analytic products should indicate and explain the basis for uncertainties associated with major analytic judgments […] analytic products should express judgments that would be useful to customers, and should not avoid difficult judgments in order to minimize the risk of being wrong […] Analytic judgments should express judgments as clearly and precisely as possible, reducing ambiguity by addressing the likelihood, timing, and nature of the outcome or development”
If proper legal analysis makes greater precision possible, it seems to me that the advisor should be brave and say so, even at the risk of being very wrong (should the gods see things differently from the specialist).
Naturally, prospects of success might well hover around 50% in any specific case; however, it is to our detriment if, in crafting professional advice, experts hedge their bets for fear of blowback should they get it wrong. So as experts, what can we do if we want to offer more nuanced advice whilst at the same time not overstating our certainty and exposing ourselves to risk?
Beyond point estimates
Expressing prospects as a single number has the advantage of simplicity, but it is also uninformative. A single ‘point estimate’ can be represented as a dot on a 0-100 number line. That scale tells us about strength of belief in the answer to a specific legal question. Unfortunately, the point estimate tells us nothing about the assessor’s confidence in that particular number.
For example, it is obvious that there is a world of difference between 60% prospects in a range of 55-65%, on the one hand, and 60% prospects with a range of 35-85%, on the other. The latter, wider range indicates much more uncertainty about the 60%. In that sense, situating a point estimate inside a range of values is more informative – it adds a valuable dimension to the advice. I appreciate some advisors routinely do this; it helps.
Visual legal advice
We can go a lot further than simply providing a range of values. Risk professionals insist on the value of probability distributions to quantify uncertainty. This is because reference to a range of values still fails to tell us enough about how much expert belief is distributed around values that lie outside of the quoted range. And a simple range is also still silent as to the confidence around
a particular point within that range (the distribution of belief within the range). A probability distribution is best communicated graphically, even if any graphical representation of belief risks misrepresenting subjective probability as something more concrete. The fact remains there is no more convenient way of showing the distribution of belief than with a graph of the probability density function of a continuous variable, the ‘pdf’, which makes visible the extent to which expert belief lies within a particular interval of values and exposes the quantum of risk. Conditional probabilities
If we are serious about the clear communication of legal advice, this approach also calls for more thought about how we reason about prospects of success in circumstances where the parameter of interest (in this case that percentage prospects figure) is usually conditioned on events which – in turn – impact the expert’s final assessment.
It is often the case, for example, that legal advice needs to be updated to take account of new evidence or otherwise by the admission of some material fact that causally impacts prospects. Whilst developments in disclosure as a case progresses create a clearer picture, I do not think it desirable for prospects advice to be updated arbitrarily to signal the growth or diminution in levels of confidence in a given outcome. A better alternative exists: “Bayesian updating” is a statistical approach for arriving at an opinion showing the extent to which beliefs change to take account of the new data.
Bayesian updating and DAGs
Thanks to the development of ubiquitous, inexpensive computational tools, we can easily model the dynamics of any legal issue by designing a causal graph (also known as Bayesian Belief Network/BBNs or more properly Directed Acyclic Graphs – ‘DAG’s). A DAG is particularly apposite for modelling the types of problem lawyers grapple with; this is because legal reasoning consciously lends itself to the stepwise resolution of any given problem. Using causal graphs, probabilities are derived by following a sequence of single-issue assessments of intermediary questions. This chain of reasoning is the intellectual procedure followed both in well-crafted legal opinions and by judges in their reasoned judgments. The key take-away is this: the process can be anticipated, and transparently modelled to evaluate the most likely outcome.
Bayesian approaches have gained widespread recognition for their role in Alan Turing’s deciphering of the Enigma Code, the US Navy’s use of it to find a missing H-bomb, and their usefulness in assessing the chances of a nuclear accident (see Sharon Betsch McGrayne’s The Theory that Would Not Die (New Haven and London: Yale University Press, 2011). But they are also readily available tools to tackle smaller-scale problems involving uncertainty.
Legal advice, the intelligence services, and sparse data
There are indications the intelligence community, as well as other sectors such as medical research, increasingly use causal graphs to support their decisions (see, for example, Fenton, N.E., Constantinou, A., & Neil, M. (2017). "Combining judgments with messy data to build Bayesian Network models for improved intelligence analysis and decision support". In Subjective Probability, Utility and Decision-Making Conference (SPUDM 17). Haifa, Israel; as well as McNaught, K., and Sutovsky P., “Representing Variable Source Credibility in Intelligence Analysis with Bayesian Networks”, 5th Australian Security and Intelligence Conference, Perth, 3rd-5th December 2012).
I understand this is because the intelligence services need to deal with sparse, ‘expert’ data to make decisions and recommendations. For obvious reason intelligence data is often sparse and high cost: it is based on individual expert informants and is risky to obtain. In the legal context, information is sparse for different reasons – both because disclosure is late and partial, and because expert opinion comes at high cost, given the market dictates a high price for legal expertise.
Probabilistic inputs, and the posterior distribution
Wherever data is sparse rather than ‘big’, and where the inputs include expert opinion, Bayesian techniques have unique value in providing a means to draw useful inferences. A Bayesian model can also be updated to reflect changes in the material circumstances of the case (whether new evidence or a significant concession by the other side). Bayesian analysis can accept probability distributions as its inputs, and in turn produces as an output a further probability distribution – the “posterior distribution”, rather than a point estimate.
Beyond ‘black box’ forecasting
In practical terms, this means that – approached as a Bayesian estimation problem – a legal advisor can produce advice that quantifies uncertainty for the client, identifies the significant inputs into that estimation, and in doing so provides an audit trail indicating how a given opinion was arrived at.
Better (more precise) estimates also add value for the client and the justice system because, of course, fractional prospects of success are the multiplier required to assess a case’s expected value. If we can think clearly about how we approach this element of evaluation it will assist in promoting settlement at efficient levels and avoiding unnecessary litigation.
I have found value in incorporating probabilistic programming methods into my practice and would recommend anyone interested to consider using such techniques; combined with traditional approaches to legal analysis, they offer a way to present robust and transparent advice to sophisticated clients in cases of any level of complexity.
Alexander MacMillan (St Philips Chambers)