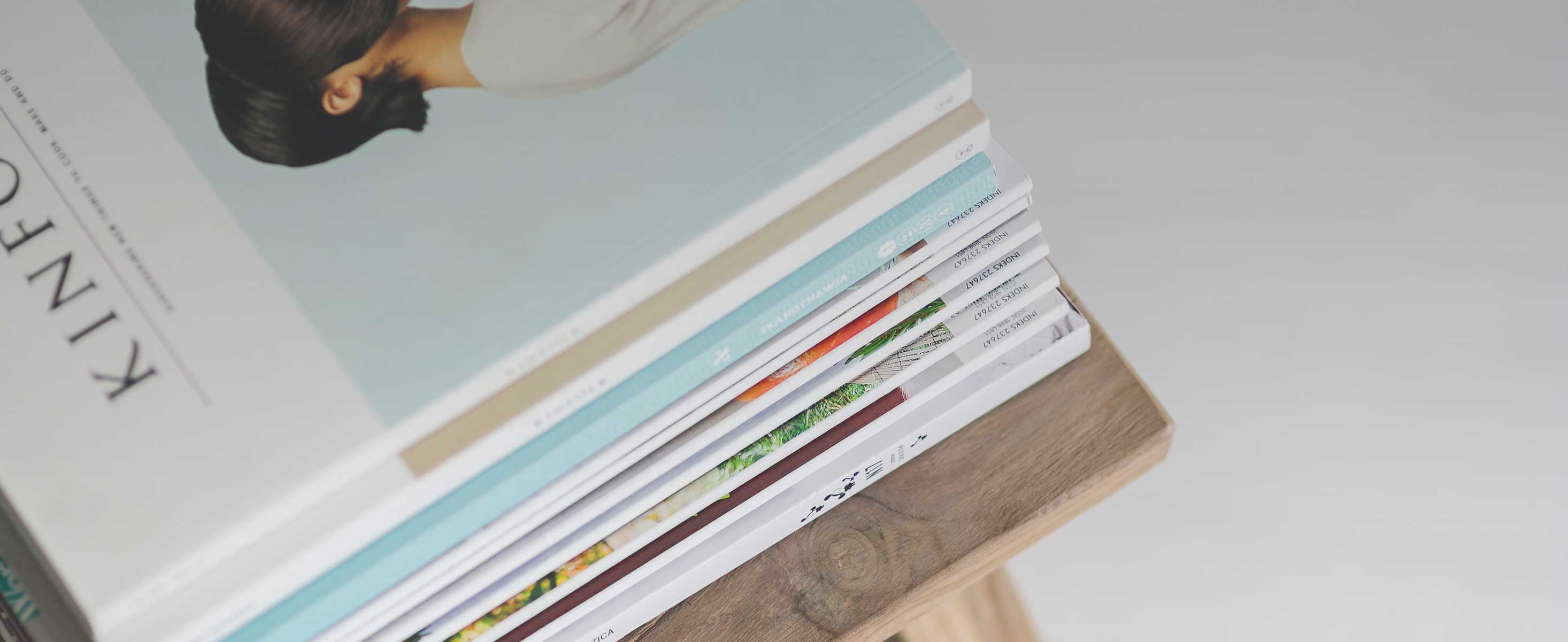
17 minute read
Part 3: The use of technologies for protecting public spaces: efficient but not sufficient
The rise in surveillance technologies over the past 20 years has given local and regional authorities a whole new range of tools they can use to better protect their public spaces, notably by enabling 24/7 moni toring, controlling access, or “nudging” public space users’ behaviour. The question for local authorities now is not so much whether to use technology, but rather which technology to use, where, when and how. This is a vast field that can appear a bit daunting in particular for medium and small municipalities that do not have the resources to keep abreast of fast-evolving technological developments. The chal lenge is to use technologies in such a way that public spaces are safer, but also remain open and inclusive while respecting fundamental freedoms and the right to privacy.
Efus’ positioning
Efus helps local and regional authorities to innovate, not only by better using new technologies but also by bringing social innovation to their crime prevention and security policies. Efus’ approach is based on the key principle that such policies, whether they concern the digital or the physical space, must include the respect of human rights, privacy and fundamental freedoms. Even though technologies transform the way police, public authorities and civil society act and react to crime, they do not change the fundamental princi ples that prevention is an effective approach to crime and that social cohesion is key to safeguarding security.
Smart technologies
Smart technologies such as facial or sound recognition are increasingly used by local authorities and other security stakeholders, notably law enforcement, to better protect public spaces. They can also be used to identify the location of victims of floods and landslides or to find people reported as missing. Efficient, although far from infallible, smart tech
nologies should not be considered as a stand-alone solution, but rather be part of a coherent, holistic security approach. They should always be used within a framework that guarantees the respect of privacy and fundamental rights.
The main smart technologies (to date) being used in public space pro tection are:
Intelligent Cameras
• Body cameras (body worn video)
• CCTV (closedcircuit television)
• Drone cameras
Biometric Technologies
• Face recognition
• Fingerprint scanners
• Voice recognition
• Iris scanners
Digital Technologies
• Algorithms, big data
• Predictive policing
Technologies that rely on data generated by citizens
Another aspect of the use of technology for public space security is that of citizen participation. Growing numbers of local authorities through out Europe, as indeed national police forces, are using social media and mobile technologies (apps) that foster direct exchanges with citizens. They can be useful in case of a crisis for authorities to gather information on the ground and inform the public in real time about an evolving situation. They can also help spot an incident and track wrongdoers. But like all other security technologies, they must be used within a clear ethical framework that guarantees fundamental rights for all and the respect of the rule of law.
Predictive tools
Even more sensitive are predictive policing tools, which rely on algo rithms to predict where crime can happen and even who could commit an offence or a crime. These technologies, which are still quite new and bound to undergo many iterations in the years to come, are beyond the remit of municipal or other local authorities as they are intended for
48 49
police. Nevertheless, local authorities should be aware of their advan tages and pitfalls because they are directly concerned by how policing is conducted on their territory and among their resident communities.
Following are some practices and insights Efus has garnered while working on this vast topic of security technologies, either through European projects (PACTESUR, Secu4All, PRoTECT and CCI) or through its ongoing, daily work, in particular its working group on Security & Innovation. The aim of this chapter is to go beyond easy narratives that are either exceedingly optimistic or pessimistic – a common pitfall when it comes to polarising topics such as predictive policing, facial recognition or subjective feelings of insecurity.
Predictive policing is the application of analytical techniques –particularly quantitative ones – to identify likely targets for police intervention and crime prevention or to solve past crimes by making statistical predictions. Predictive policing could be con sidered as a method to predict in which geographical areas there is an increased chance of criminal behaviour, but also which in dividuals and groups – through predictive profiling – are more likely to be involved in criminal activities. 29
Case study: the use of drones for public space protection in Europe
In recent years, the use of drones, or Unmanned Aircraft Systems (UAS), by public services in urban areas has significantly increased. These systems can carry out effective monitoring and surveillance functions in public spaces. They can be used to prevent potential physical attacks on critical infrastructure (power, water and life systems), airports, open-air events and concerts. Some European cities have established drone units within their municipal police to develop, implement and improve their use in public spaces. However, research is still needed to identify the best way to obtain optimal results. The use of these technologies raises concerns about the right to privacy and data protection. Additional risks are related to potential cybersecurity breaches and malicious uses, such as when drones are used for trans porting drugs or worse, weapons and bombs, or for espionage.
(1) The Drones Unit of the City of Turin (Italy)
“Drones must be considered as one among a whole range of tools and measures used as part of an overall strategy. They must be integrated with actions and technologies that already exist on the ground.”
Prediction-led process (Perry et al., 2013)
The City of Turin coordinates the DronEUnit Network, a European Drone Unit network gathering various Law Enforcement Agencies (LEAs), private companies, universities, academia and research centres whose aim is to discuss how to best use drones to improve certain services offered by municipalities to the public. It uses drones to monitor large events and large crowds, notably to spot individuals in distress and inform emergency services.
50 51
Gianfranco Todesco, Chief Commissioner, Local Police of Turin
Altered
Data
Data Fusion Prediction
Intervention
29- Efus, CCI (2020). Factsheet on Predictive Policing.
Environment
Collection
Assessment
Data Collection Analysis Police Operations Criminal Response
Turin is the first city in Europe that has two drone testing areas (one indoor, the other outdoor):
1. DoraLab, an urban park with an optimal position that guarantees security conditions for the flight of drones.
2. Città dell’aerospazio (“aerospace city”), where pilots are trained to face difficult outdoor conditions such as heavy winds.
(2) The use of drones to monitor public spaces by Police Scotland, City of Edinburgh (United Kingdom)
“All police drone activity is overt and transparent. Deployment must be for a legitimate policing purpose, be safe, legal, proportionate and necessary.”
In Police Scotland, there are two distinct and separate capabilities:
1. The Police Remotely Piloted Aircraft Systems (RPAS) Unit uses drones to keep people safe across Scotland. Drones are used as a quick and effective way to search large or sometimes inaccessible areas that would otherwise take a search team on the ground signifi cant time and resources. The RPAS Unit is of the opinion that drones are not best suited to urban areas due to building dynamics and proximity to people. Public space CCTV and ground resources are found to be the most effective in an urban search. However, drones were used to monitor airborne threats during the COP26 Climate Summit in Glasgow (November 2021)
2. The Aviation Safety and Security Unit (ASSU) employs a range of techniques to protect the public and partners from potential aerial threats. Such mitigations include airspace restrictions, geofencing and trained responders to support and protect legal drone users and to help local police with growing illegal use of drones. Such methods are deployed at sites and for events, operations and incidents.
All Police Scotland drone operations are conducted in line with a Data Protection Impact Assessment (Privacy Assessment), the EU’s General Data Protection Regulation (GDPR) and an Equalities & Human Rights Impact Assessment. Police Scotland informs the public or communi ties of a particular area of proposed police drone activity using social media, face-to-face engagement and sometimes leafleting. Community engagement is crucial as it builds trust, reassurance and confidence. All police drone activity is overt and transparent. Deployment must be for a legitimate policing purpose, be safe, legal, proportionate and necessary.
> This analysis is part of the joint interview of the cities of Edinburgh and Turin for the PACTESUR project. The full version is here
(3) The use of drones by the Municipal Police of Madrid (Spain)
In Madrid, the Air Support Section provides service across municipal departments and areas (including urban planning, firefighters and emergency medical services) and uses drones as follows:
Monitoring & surveillance: event crowd and capacity monitoring; surveillance and supervision of public events and night-time leisure parks; support to Central Security Units in events or police interven tions; monitoring of traffic offences; surveillance of large areas to prevent/locate fly-tipping, and general night observation and surveillance.
Communication: public health warnings (notably during the Covid-19 crisis).
Accident reconstruction: use of UAS for the investigation of acci dents at work (major injuries or fatalities) in hard-to-reach places; collaboration with the Accident Investigation Unit, drawing up 3D plans of accidents and reconstruction of traffic accidents.
Disaster management: collaboration with fire brigades and City Council technicians in different types of disasters, such as building explosions (gas), roof collapses, building fires, etc.
52 53
Inspector Graeme Rankin, Police Scotland, City of Edinburgh
Search & seizure: i.e. illegal marijuana plantations.
Search & rescue: search for missing persons in large areas.
> More information in the Madrid practice sheet here.
Case study: video surveillance technology and facial recognition
For the purposes of monitoring public spaces, video surveillance tech nology and facial recognition can facilitate the identification of suspi cious behaviour in urban areas. Facial recognition is arguably one of the most polemic and yet ubiquitous applications of AI-based computer vision algorithms. It is biometric technology – a process that allows us to recognise a person by a physical or behavioural characteristic. Other examples of this are fingerprint or iris recognition, voice recognition but also identification based on gait.30 Facial recognition has two func tions: the authentication and the identification of a person.
In the first case, the system compares the biometric template of a face to a single image of a particular person in order to verify whether or not it is the same person.
In the second case, the model is compared to a larger database of pictures in order to identify one person amongst many.
A third, highly controversial, function is concerned with categorisa tion – the differentiation of people into various categories, depending on individual characteristics. Such characteristics commonly include sex, age and ethnic origin.
In practice: facial recognition tests in London, London Metropolitan Police (UK)
Between 2016 and 2019, the London Metropolitan Police Service (MPS) conducted 10 Live Facial Recognition (LFR) tests in differ ent scenarios and with different watchlists of persons of interest throughout the city. The purpose of these trials was to “assess the value, viability and challenges (including technological, legal, ethical, and governance)” of the technology. The facial recognition software was integrated into cameras that would generate alerts, which officers could then assess and adjudicate.
The MPS used a watchlist of individuals of interest and their facial images as a database, which eventually included 2,401 subjects. Over the three years of testing, LFR was deployed for a total of 69 hours. With approximately 180,000 opportunities of recognition (faces that appeared in the videos), the police engaged with 27 individuals and arrested 9 in response to alerts by the facial recognition system.
The efficiency of the LFR system greatly depends on the number of individuals listed on the watchlist. The increase of the list’s size is believed to have contributed to a higher number of identifications and arrests during the second half of the trials. Compared to the “manhunt” tactic where offenders are located by deploying officers to multiple locations over long periods of time, LFR requires less re sources and can increase operational efficiency. Regarding the location of the cameras, the system was most efficient when there was a level of control over the flow of people passing through the area. The tests also showed that while the rate of false positive identi fications was statistically insignificant when it came to ethnicity, it was significant in terms of gender difference. Women had both lower false positive identification rates and lower true positive identification rates.
30- CNIL (2019). Reconnaissance faciale : pour un débat à la hauteur des enjeux.
> For a detailed description of the experimentation, see here.
54 55
The Nice Urban Supervision Centre
In practice:
facial recognition
during Carnival, Nice (France)
In 2019, the City of Nice decided to evaluate the relevance and reliability of live facial recognition during its annual Carnival. The objectives were to contribute to the securitisation of public spaces and support scientific research, based on an Israeli software called AnyVision. Forty volunteers signed up for their picture to be added to a fictitious database. The software can take into account ageing, thus recognising a person up to 20 years after the photograph was taken.
In order to comply with GDPR and respect the right to privacy and fundamental rights, the simulation was only used in a specific zone around one of the entrances of the Carnival site. The fact that the experiment was being conducted was clearly communicated to Carnival-goers through leaflets and banners. In addition, people who accepted to go through the zone were given a coloured bracelet. The experiment involved three sce narios: controlled access at one of the entrance gates of the Carnival area through facial identification, the detection of a (fictitious) person of interest in the crowd, and spotting a person of interest when they pass through the gate. In total, 5,000 people accepted to take part in the experiment over a period of three days.
A report on the experiment conducted by the City Council high lighted the high level of social acceptance of such technologies, provided they were properly regulated. As for the efficiency of the technology, the municipal police emphasised in the report that they found it useful and would use it if available.31
The Nice Urban Supervision Centre (USC), is the operational command controlling all the cameras of the city’s video surveillance network. It operates 24/7 and has three objectives:
Enhancing public security by deterring and preventing attacks on the safety of people and property. Video footage is notably used in criminal investigations and for the identification of offenders.
The prevention of natural disasters and risks; search and rescue; protection against fires. For example, CCTV is used to monitor river banks and sea coasts, allowing for both contextual and specific views of those areas without putting officers at risk. The images are also used by rescue services to identify the origin and monitor the devel opment of natural phenomena and mobilise the most appropriate response means.
Monitoring traffic and urban circulation with technology such as predictive tools to anticipate traffic conditions, thermal cameras for detecting people and obstacles to circulation, or to limit uncivilised behaviour such as double parking, as well as real-time video protec tion of the tramway network.
Considerations when developing and deploying facial recogni tion technologies
Given the complexity of this technology and the ubiquity of its potential uses, it is crucial to consider how to ensure the protection of funda mental rights and freedoms while responding to security needs. How can anonymity be preserved in public spaces? What forms of surveil lance are acceptable without raising fears in society and negatively in fluencing people’s feelings of insecurity and unsafety? While technology offers a broad range of opportunities for the protection of public spaces, these solutions have to be developed in concordance with physical protection measures and must be designed to respect privacy regulations. Efus’ working group on Security & Innovation drafted a series of considerations for local and regional authorities:
31- Nice City Council (2019). Assessment of the facial recognition experiment (only in French).
56 57
Working towards a clear legal and regulatory framework: considering the speed and complexity of new developments in facial recognition technology, the European Union is planning on re-as sessing existing legal frameworks, such as the GDPR, and consider ing new legal requirements. In its white paper on artificial intelligence, the Commission outlines aspects that these require ments are linked to: training data, keeping of records and data, infor mation to be provided, robustness and accuracy, and human oversight. Sharing local experiences, problems encountered and lessons learnt on a European level can help anchor such require ments in the real needs of European cities and regions.
Assessing the impact on fundamental rights: given that facial recognition technologies impact a whole range of fundamental rights, it is important to assess them both, to different extents, during the development and the deployment of algorithms.
Evaluating necessity and proportionality: prior to deploying facial recognition technology, a city or region must develop a clear understanding of the local urban security situation and evi dence-based knowledge. The information gathered during a safety audit can help frame considerations of necessity and proportionality in order to find the right balance between the benefits and the risks of using facial recognition. This includes evaluating which public spaces should be outfitted with the technology for what reasons and problems.
Monitoring facial recognition technology: when a law enforce ment agency uses facial recognition software, it is paramount that agents verify the results. They should evaluate whether a match is accurate and decide on an appropriate response. The accuracy and efficiency of the software itself should be monitored by independent supervisory bodies.
A proper understanding of the technology: local authorities often rely on externally developed technology. In that case it can be hard to understand how a facial recognition software works and to evaluate it. In order to ensure that fundamental rights, such as the right to non-discrimination and data protection, are integrated not only in the
deployment but also in the development of the technology, such con siderations must be part of the procurement process (FRA, 2019).
Adequate police training: depending on the quality of the software used, it is possible that law enforcement get a large number of hits. The interaction with people who were matched with a face on a watchlist needs to follow the same principles of respect as any other interaction. Again, awareness of the software’s potential fallibility and inaccuracy is important to understand that a match does not necessarily mean that a person was properly identified or authenticated. Training law enforce ment officers on how to handle such situations can be helpful to ensure calm and dignified interactions with the public.
The 2021 European Union Artificial Intelligence (AI) Act
In April 2021, the European Commission published a proposal for a regulatory framework on the use of AI. This framework was conceived as an answer to insufficient existing legislation and sets out rules to enhance transparency and minimise risks to fundamental rights. The document focuses on high-risk AI systems, including, amongst others, the use of crime forecasting software and facial recognition in urban spaces. These high-risk uses can only be put into place if they fulfil a number of require ments, such as: the use of high-quality datasets, the establish ment of appropriate documentation to enhance traceability, sharing of adequate information with the user and the design and implementation of appropriate human oversight measures.
58 59