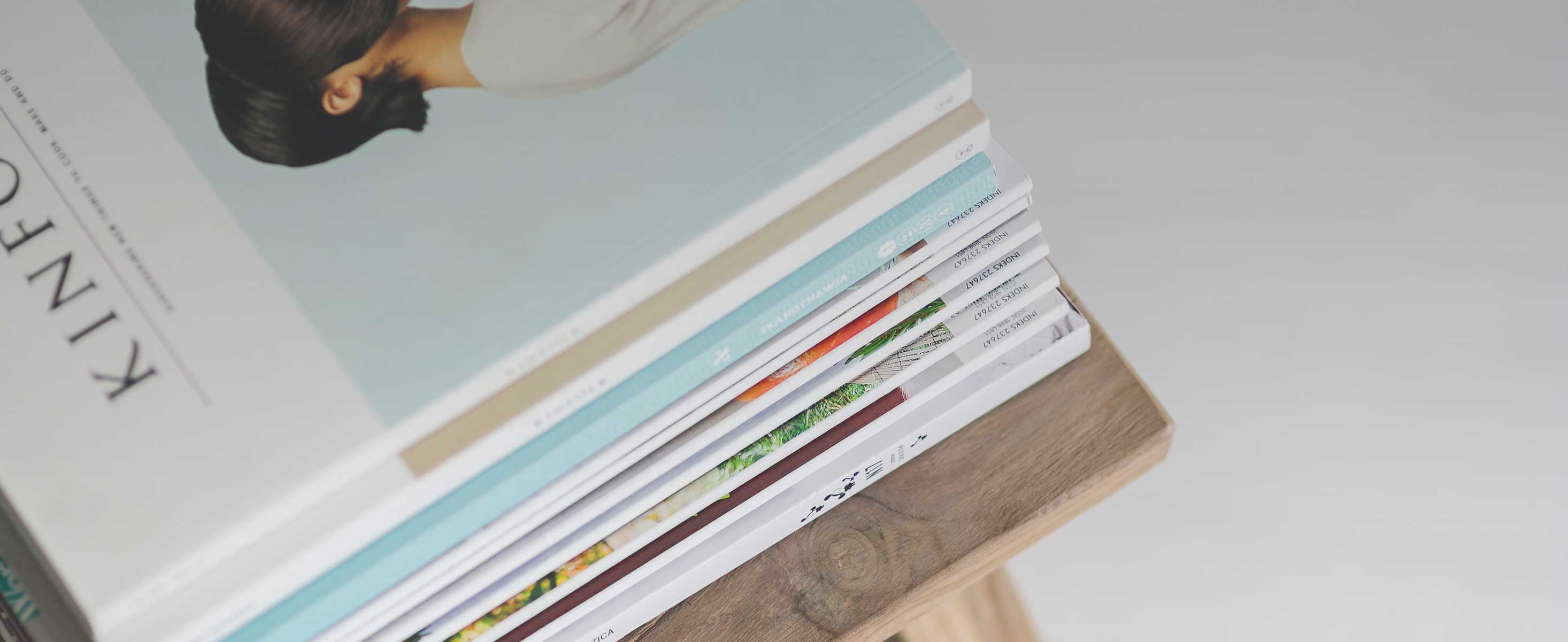
2 minute read
OVERCOMING AI SHORTCOMINGS
THERE HAS BEEN MUCH FOCUS ON WHAT TYPE OF DATA WE TRAIN THESE MACHINES on and how those algorithms work to produce actionable results. But then what? There’s a third part to this human-AI interface that is just as important as the first two—how humans and the larger systems we have in place react to this data.
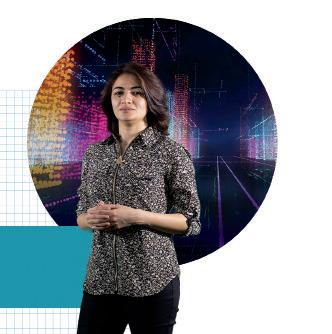
“Causal mechanisms, the reason things happen, really matter,” says Carrubba. “At Emory, we have a community beyond machine learners from a variety of specializations—from statisticians to econometricians to formal theorists, with interests across the social sciences like law, businesses, and health—who can help us anticipate things like human response.”
One such person is Razieh Nabi, assistant professor of biostatistics and bioinformatics at Rollins School of Public Health. Nabi is conducting groundbreaking research in the realm of causal inference as it pertains to AI— identifying the underlying causes of an event or behavior that predictive models fail to account for. These causes can be challenged by factors like missing or censored values, error in measurement, and dependent data.
“Machine learning and prediction models are useful in many settings, but they shouldn’t be naively deployed in critical decision making,” says Nabi. “Take when clinicians need to find the best time to initiate treatment for patients with HIV. An evidence-based answer to this question must take into account the consequences of hypothetical interventions and get rid of spurious correlations between the treatment and outcome of interest, which machine learning algorithms cannot do on their own. Furthermore, sometimes the full benefit of treatments is not realized, since patients often don’t fully adhere to the prescribed treatment plan, due to side effects or disinterest or forgetfulness. Causal inference provides us with the necessary machinery to properly tackle these kinds of challenges.”
Part of Nabi’s research has been motivated by the limitations of the methods proposed. For one example, there’s an emerging field of algorithmic fairness—the aforementioned idea that, despite the illusion that machine learning algorithms are objective, they can actually perpetuate the historical patterns of discrimination and biases reflected in the data, she says.
“In my opinion, AI and humans can complement each other well, but they can also reflect each other’s shortcomings,” Nabi says. “Algorithms rely on humans in every step of their development, from data collection and variable definition to how decisions and findings are placed into practice as policies. If you’re not using the training data carefully, it will be reflected poorly in the consequences.”
Nabi’s work combats these confounding variables by using statistical theory and graphical models to better illustrate the complete picture. “Graphical models tell the investigator what these mechanisms look like,” she says. “It’s a powerful tool when we want to know when and how we identify these confounding quantities.”
Her work is focused on health care, social justice, and public policy. But Nabi’s hope is that researchers will be able to better account for the human element when designing, applying, and interpreting the results of these predictive machine models across all fields.