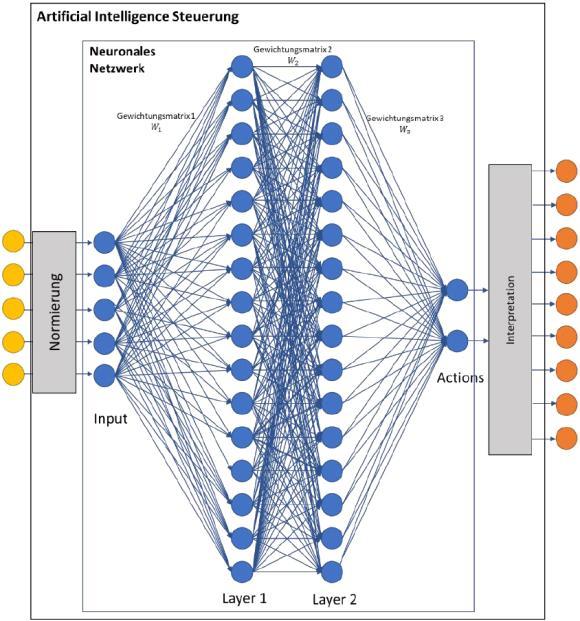
2 minute read
4.5 Artificial Intelligence (AI) Heat Pump Controller
Many buildings are now heated with heat pumps, often in combination with a geothermal heat probe. The heat pump extracts heat from the ground at a low temperature level to always ensure comfortable room temperature. Even more important than with fossil heating systems is the design of the heating system and the correct commissioning. This work has the goal to shown how a neural network can be trained by means of simulations in such a way that no parameter settings in the heat pump controller must be adjusted during commissioning. During the training phase, the AI heat pump controller has learned many different scenarios with different buildings, variable weather, and stochastic user behavior. As a result, the heat pump is operated in an energy-optimized manner without having to know the building during installation.
Advertisement
Contributors: L. Joos, S. Pfyffer, A. Witzig, D. Kempf, V. Ziebart (IAMP), N. Schmid (IAMP) Partner(s): Institut für Angewandte Mathematik und Physik (IAMP) Funding: Innosuisse / Bachelor’s Thesis Duration: 2020–2021
In the Energy Strategy 2050 of the Swiss government, the use of ambient heat will play an important role for heating. The heat pumps used for this purpose should be operated with the highest possible proportion of renewable electricity from hydropower, wind farms and photovoltaic systems. The corresponding control units should optimize energy and costs and guarantee indoor comfort at all times. To do this, they also use the storage masses of the buildings and react to weather conditions and changes in user behavior. In traditional controllers, some parameters such as the heating curve of the building or the size of a locally available photovoltaic system must be set correctly. This is done during commissioning of the heat pump or during a later check by a service technician. Within the framework of several student projects, an AI heat pump controller was developed that optimally controls the system components based on training with simulation data. The aim of the research work is that an AI heat pump controller achieves the same good performance (cost and energy optimization) as traditional controllers, but with significantly less effort during installation and commissioning. To ensure that the AI heat pump controller continuously guides the system hydraulics to the optimum operating point, it is trained with a dynamic system before delivery. In several study projects, the simulation software Polysun and the "OpenAI Gym" platform were used for the training phase. As shown in the figure to the right, a neural network consisting of two layers with 16 neurons each is used in [1]. Reinforcement learning is used to optimize the corresponding coefficients of the neural network on the basis of a large number of simulations. A cost function is defined which penalizes the careless use of energy and the undercutting of the set temperature in the building.
Figure: Neural network used in [1]. Input datapoints (signals from sensors) are symbolized with yellow circles, output data (signals to actors) are shown by orange circles.
References:
[1] Laurenz Joos, Selina Pfyffer, Reinforcement Learning für Wärmepumpensysteme mit PV, Betreuer: A. Witzig, V. Ziebart, Bachelorarbeit in Energie- & Umwelttechnik.