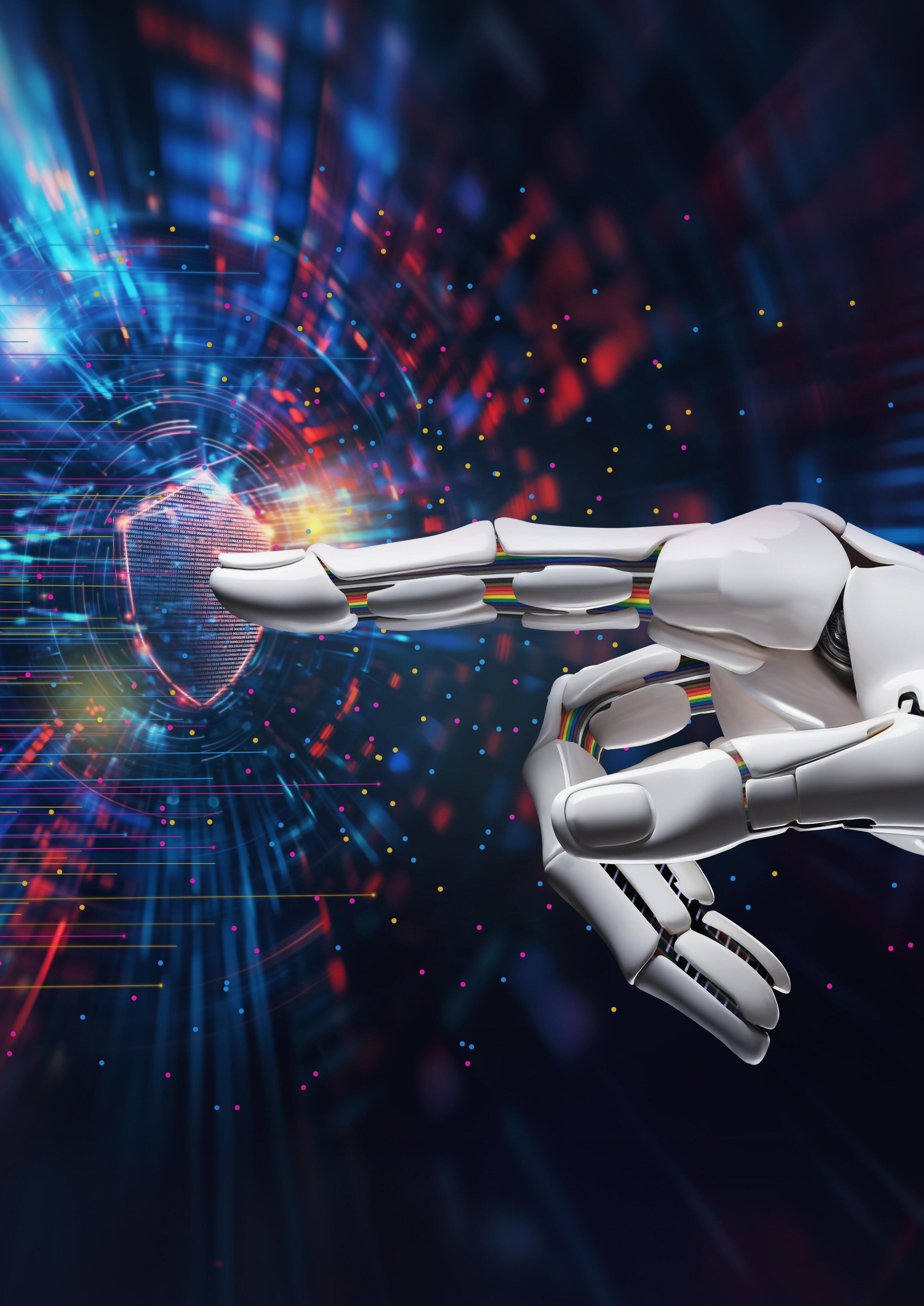
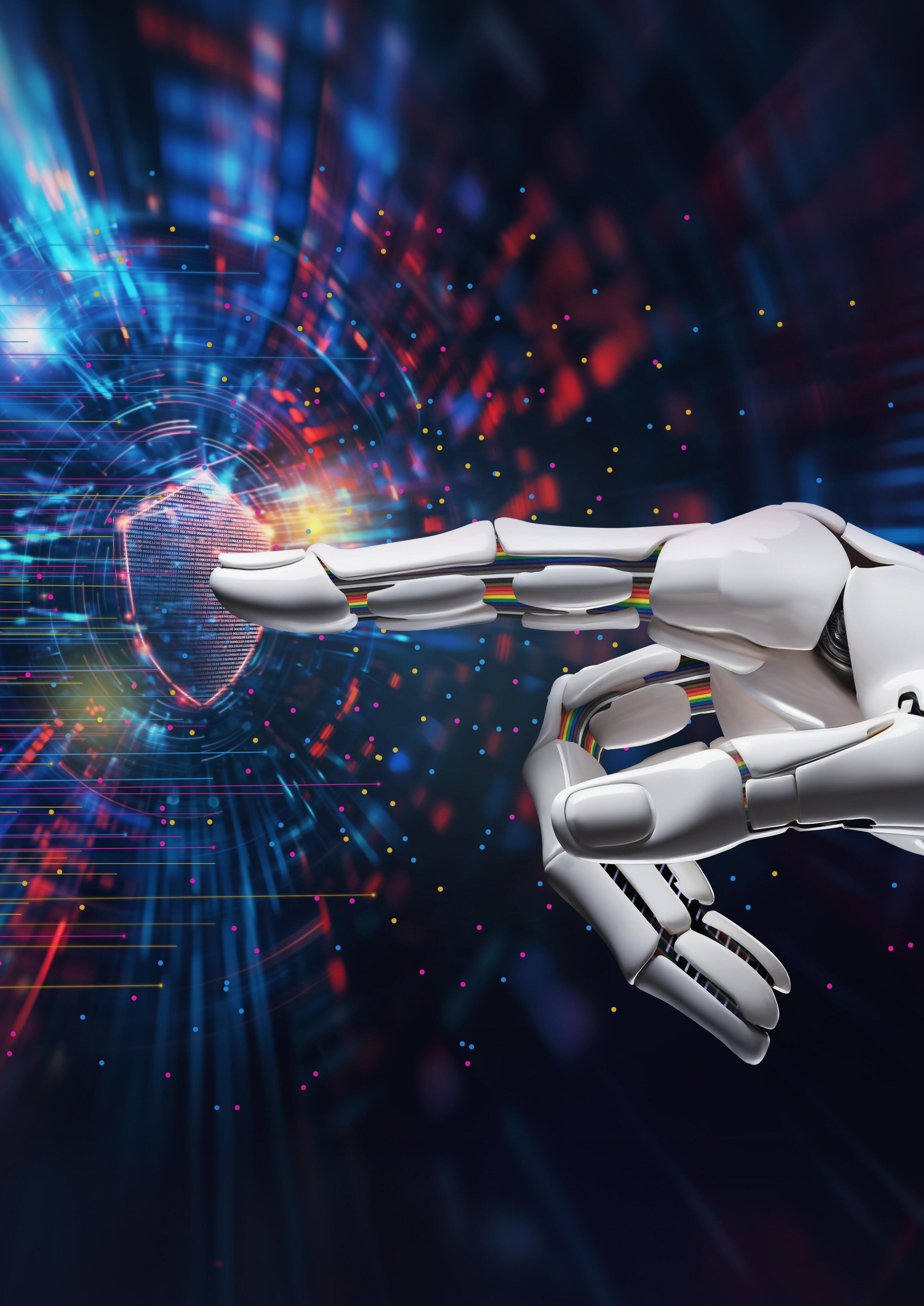
CHANGING THE GAME
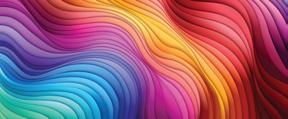
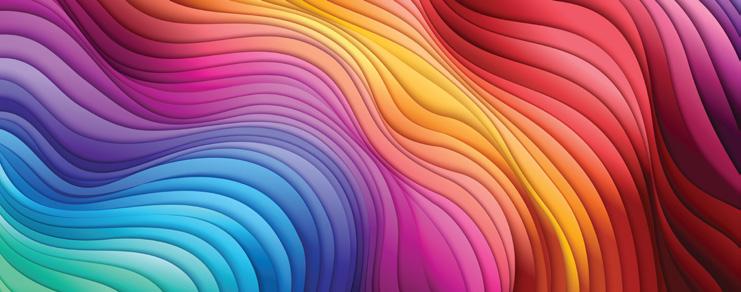
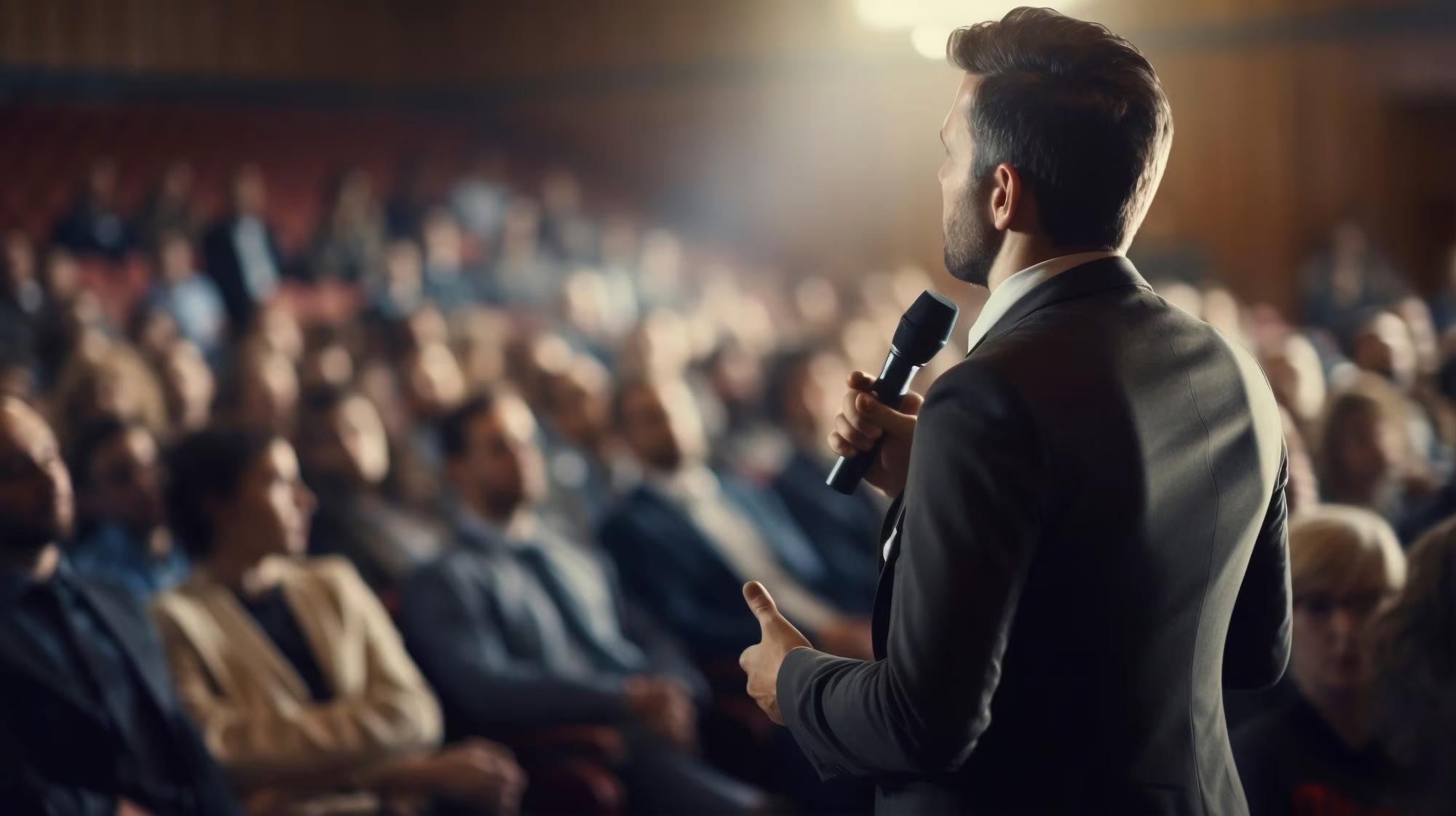
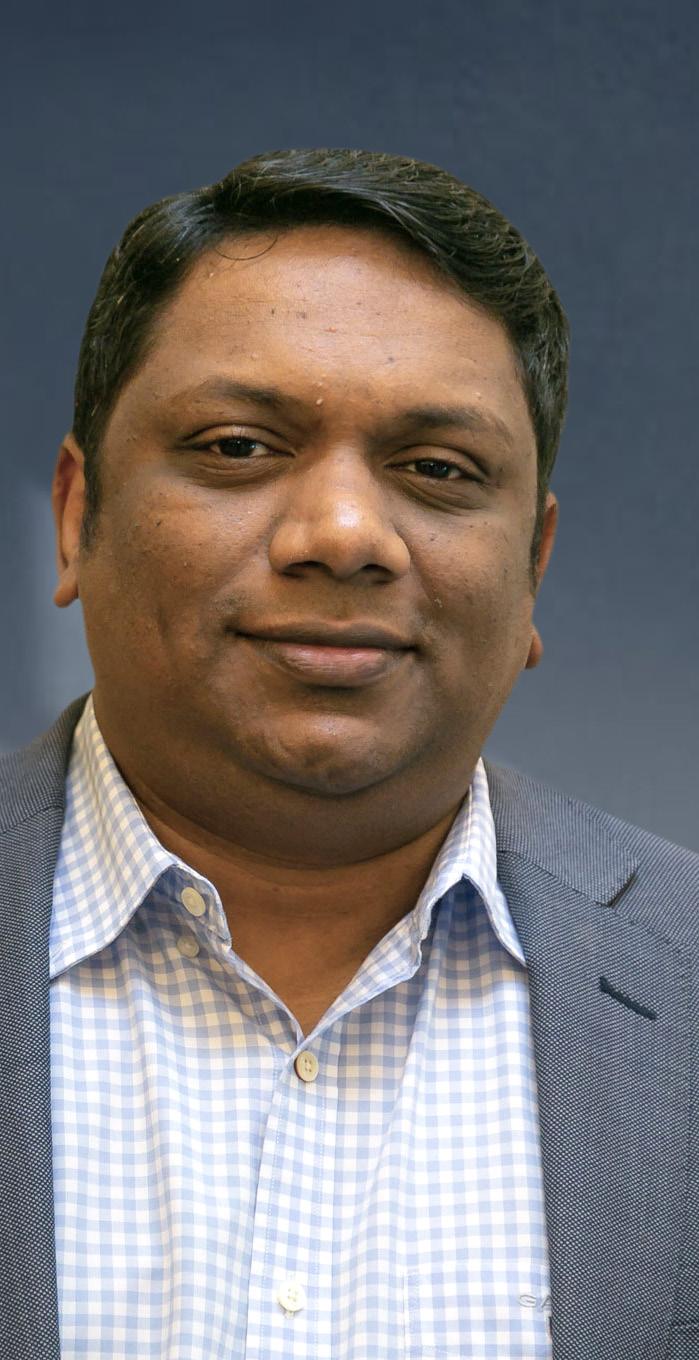
Building guardrails for AI
Should AI be regulated? This is a heated topic of discussion around the world. Recently, the European Parliament approved the AI Act, which aims to foster responsible AI development and deployment within the EU. Similarly, the United States is making continued efforts toward AI regulation, with potential legislation that could include licensing requirements and the creation of a new federal regulatory agency. In China, it is already mandatory for AI algorithms to be reviewed by the state to ensure they adhere to core socialist values.
The world remains divided on the AI regulation debate, and many fear that we may not be able to fully own or control AI. Some of the brightest minds in the industry have even warned of significant risks associated with AI, suggesting it could pose a threat to humanity’s very existence.
While the idea of AI destroying humanity sounds far-fetched, proponents of AI regulation argue that, without oversight, the impact of AI could be damaging. A case in point is deepfakes—fake but seemingly real photos and videos that are wreaking havoc on the online world.
Be that as it may, regulating AI might be easier said than done. Like any other emerging technology, AI is neither inherently good nor bad; its impact depends on how it is used. Moreover, AI is a rapidly evolving field with new use cases and innovations constantly emerging, making it challenging for regulators to keep pace. There are also concerns that AI laws might stifle innovation.
As governments, industries, and researchers work together to navigate this complex landscape, the ultimate goal should be to harness AI as a force for good—one that drives societal advancement while safeguarding ethical standards and minimizing harm. The future of AI will depend on how well we can rise to this challenge.
Thanks for reading.
JEEVAN THANKAPPAN
JeevanThankappan
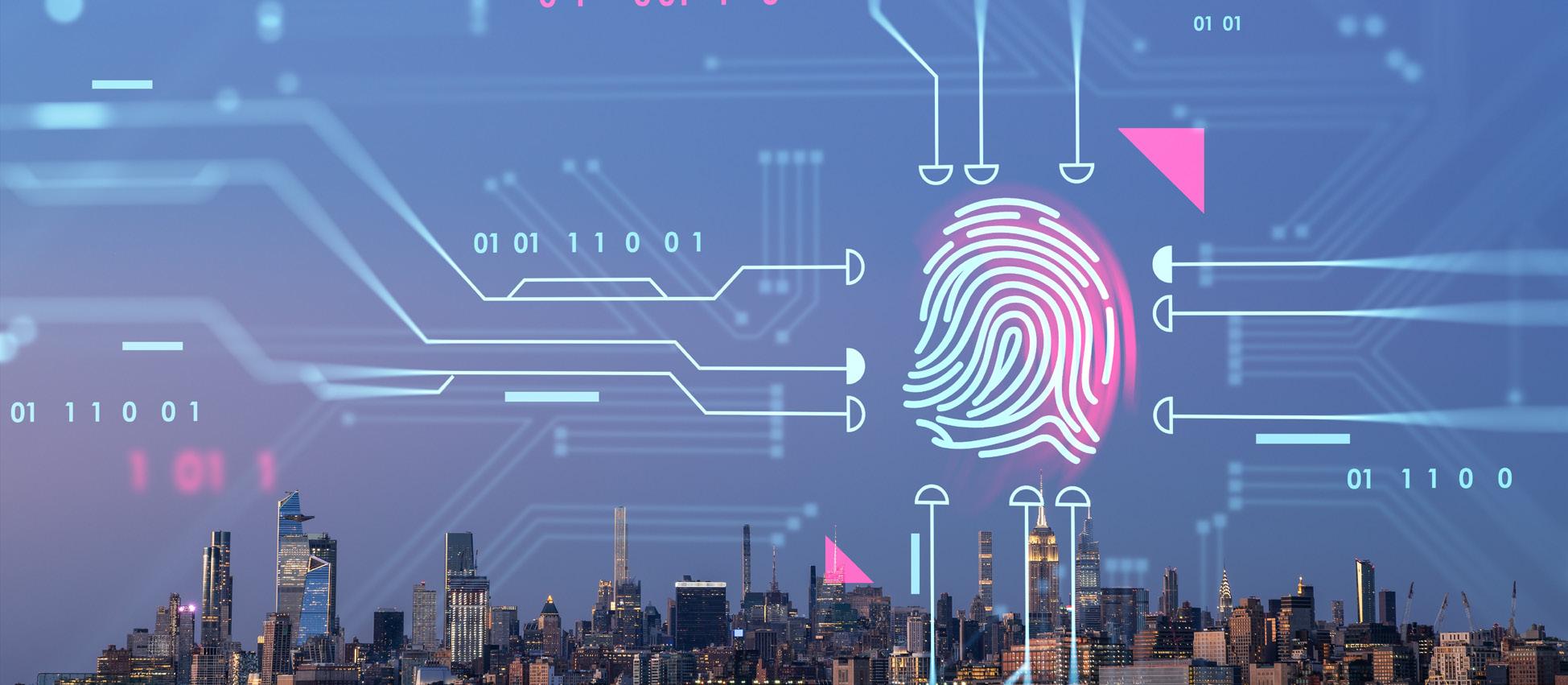
06
PIF and Google Cloud to launch advanced AI hub. PIF and Google Cloud announced a strategic partnership to create a new global AI hub in Dammmam, Saudi Arabia.
14 Leading the AI revolution. Tushar Vartak, Head of Cybersecurity at RAKBank, discusses the shift from reaction to prediction in the age of AI.
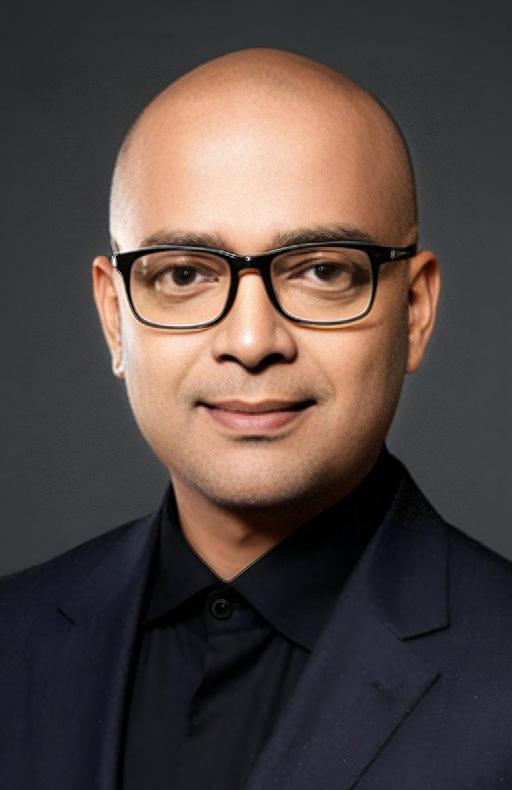
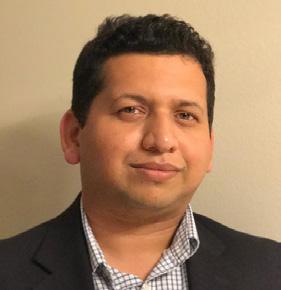
20 Bridging the gap.
Subodh Dehspande from Searce says businesses need to look at process first and AI second to unlock its true potential.
28 Spotlight on leading AI brands
We explore the top AI brands transforming the industry today
30 EU AI Act. Essential compliance strategies
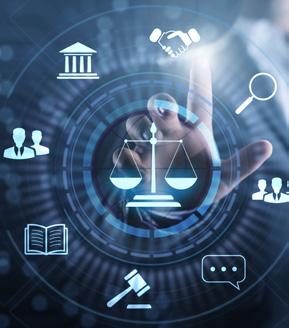
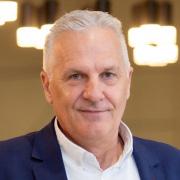
32 From experiments to investments
Exclusive interview with Chris Wiggett, Director of AI, NTT Data MEA
NORTH STAR COUNCIL
Our North Star Council serves as the editorial guiding light of the AI Times, providing strategic direction and ensuring our content remains on the cutting edge of AI innovation.
Our Members
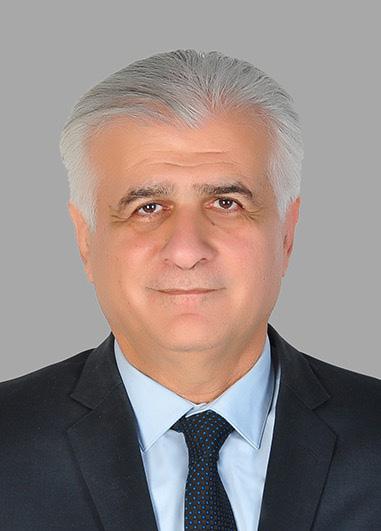
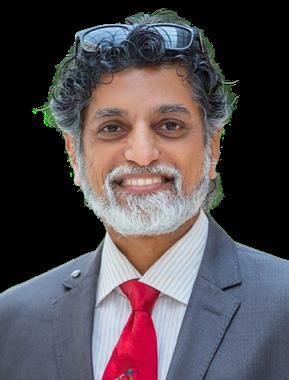
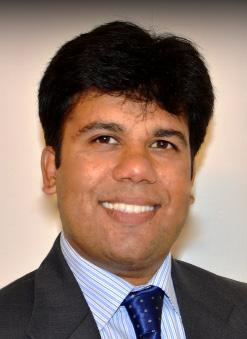
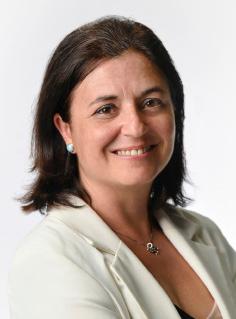
Dr. Jassim Haji President of the International Group of Artificial Intelligence
Venkatesh Mahadevan Founding Board Member CAAS
Jayanth N Kolla Founder & Partner Convergence Catalyst
Idoia Salazar Founder & President OdiseIA
If you would like to be a part of our North Star Council, please reach out to us at jeevan@gecmediagroup.com
PUBLISHER TUSHAR SAHOO tushar@gecmediagroup.com
CO-FOUNDER & CEO RONAK SAMANTARAY ronak@gecmediagroup.com
MANAGING EDITOR Jeevan Thankappan jeevan@gcemediagroup.com
ASSISTANT EDITOR SEHRISH TARIQ sehrish@gcemediagroup.com
GLOBAL HEAD, CONTENT AND STRATEGIC ALLIANCES ANUSHREE DIXIT anushree@gecmediagroup.com
CHIEF COMMERCIAL OFFICER RICHA S richa@gecmediagroup.com
PROJECT LEAD JENNEFER LORRAINE MENDOZA jennefer@gecmediagroup.com
SALES AND ADVERTISING sales@gecmediagroup.com
Content Writer KUMARI AMBIKA
IT MANAGER VIJAY BAKSHI
DESIGN TEAM CREATIVE LEAD AJAY ARYA
SR. DESIGNER SHADAB KHAN DESIGNERS
JITESH KUMAR, SEJAL SHUKLA
PRODUCTION
RITURAJ SAMANTARAY S.M. MUZAMIL
PRODUCTION CIRCULATION, SUBSCRIPTIONS info@gecmediagroup.com
DESIGNED BY

SUBSCRIPTIONS info@gecmediagroup.com
PRINTED BY Al Ghurair Printing & Publishing LLC. Masafi Compound, Satwa, P.O.Box: 5613, Dubai, UAE
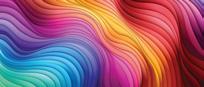
(UAE) Office No #115 First Floor , G2 Building Dubai Production City Dubai, United Arab Emirates Phone : +971 4 564 8684
(USA) 31 FOXTAIL LAN, MONMOUTH JUNCTION, NJ - 08852 UNITED STATES OF AMERICA Phone :+ 1 732 794 5918
Freshworks unveils easy-to-use AI agents
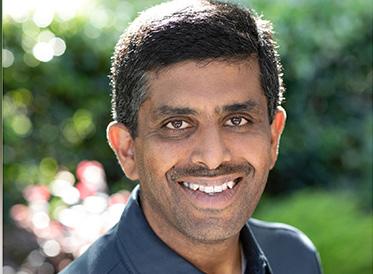
Freshworks has announced Freddy AI Agent – a new generation of easy-to-deploy and use autonomous service agents. Built to deliver exceptional customer experiences (CX) and employee experiences (EX), Freddy AI Agent can be deployed in minutes and has helped users in customer support and IT autonomously resolve an average of 45% and 40% of service requests, respectively.
“Over the last six years, we’ve seen a rise in demand for our uncomplicated, AI-
powered service solutions that make the lives of customer service and IT service managers easier and more efficient,” said Dennis Woodside, CEO and president at Freshworks.
Significant productivity and efficiency gains help unlock higher-value work, showcasing how AI is moving from an experimental tool to a driver of business outcomes across industries. Freddy AI Agent makes that possible with the following capabilities for CX and EX:
Rapid time to value. Organizations can quickly deploy Freddy AI Agent without needing to code or train models. Instead, Freddy learns from existing documents and websites. By pointing Freddy to websites and other learning materials, the agent will crawl through the resources and learn on its own.
Autonomous and always-on. Freddy AI Agent is fully autonomous and supports people on their mission to provide roundthe-clock, radically helpful, human-like conversational assistance across multiple channels.
Hyper-personalized service. Freddy AI Agent personalizes and contextualizes conversations in multiple languages across multiple channels.
Trusted and secure. Freddy AI Agent offers trustworthy, secure, enterprise-grade AI built on a bedrock of strict privacy controls to meet security and compliance standards.
PIF and Google Cloud to launch advanced AI hub in Saudi Arabia
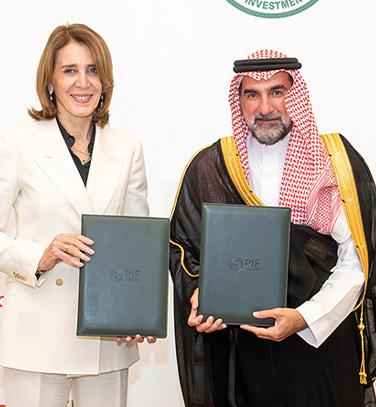
PIF and Google Cloud announced a strategic partnership to create a new global artificial intelligence (AI) hub. The new AI hub will be based near Dammam, in Saudi Arabia’s Eastern Province.
The landmark partnership, which was signed at the Future Investment Initiative 8th Edition (FII8), further establishes Saudi Arabia as a global hub and top AI destination for local and global enterprises and startups. This partnership aims to develop the Saudi workforce through AI
programs for millions of students and professionals, supporting the national objective of growing the information and communication technology (ICT) sector by 50%. Under the partnership, customers will be able to use Google Cloud’s technology to support growth across industries and increase capacity for the delivery of AI applications. Businesses and their end consumers can expect to benefit from better quality AI applications and data services, delivered faster locally.
HEALTHCARE
Globant unveils report to tackle AI myths in healthcare
Globant has launched its new ‘Move Your But’ report to drive AI adoption in the healthcare industry, showcasing AI’s potential to transform patient care and operational efficiency. The report’s findings have significant implications for the GCC region, where the healthcare market is projected to reach $135.5 billion by 2027, with AI poised to inject $320 billion into the Middle East economy by 2030.
This new report delves into AI’s impact on the healthcare industry and marks the inaugural release of an industry-focused report titled “Move Your But.”
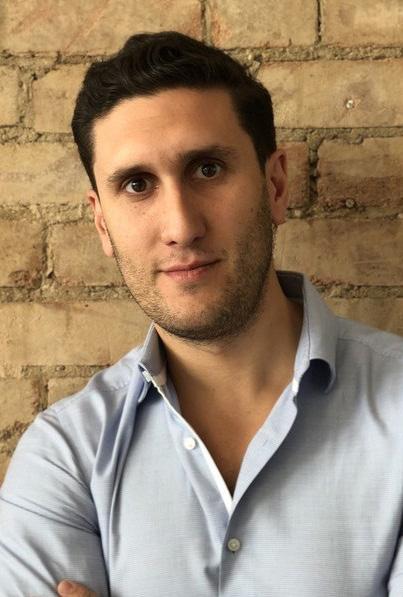
With this initiative, Globant aims to tackle the common excuses—or “buts”—that organizations use to justify not implementing AI. The findings are particularly relevant to the GCC, where the healthcare sector is rapidly evolving.
The study addresses beliefs that may hinder AI adoption and provides solutions to these challenges. For instance, despite the belief that ‘Healthcare is Personal,’ AI can enhance personalization by automating routine tasks, enabling healthcare providers to focus more on patient care. This is particularly relevant in the GCC, where the prevalence of chronic diseases like diabetes presents a significant opportunity for AI to make an impact.
“Artificial Intelligence possesses a remarkable ability to transform established paradigms, and the GCC healthcare sector is primed to benefit. With the region’s advanced digital infrastructure, AI is overcoming hurdles like complex data management and resource constraints. The potential of AI in GCC healthcare is crystal clear and is beginning to have a tangible impact. said Federico Pienovi, Chief Business Officer & CEO for APAC & MENA at Globant.
Tech Mahindra announces AI center of excellence
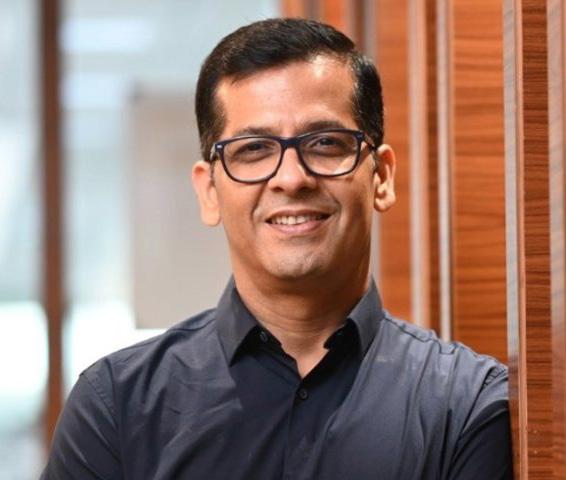
Tech Mahindra has announced the establishment of a Center of Excellence (CoE) powered by NVIDIA platforms to drive advancements in sovereign large language model (LLM) frameworks, agentic AI, and physical AI.
Based on the Tech Mahindra Optimized Framework, the CoE leverages the NVIDIA AI Enterprise software platform — including NVIDIA NeMo, NVIDIA NIM microservices and NVIDIA RAPIDS — to offer customized, enterprisegrade AI applications to help its clients adopt agentic AI in their businesses. Agentic AI significantly improves productivity by enabling AI applications to learn, reason, and take action. The CoE also uses the NVIDIA Omniverse platform to develop connected industrial AI digital twins and physical AI applications across various sectors, including manufacturing, automotive, telecommunications, healthcare, banking, financial services and insurance.
Leveraging the capabilities of the CoE, Tech Mahindra has also developed Project Indus 2.0, an advanced AI model powered by NVIDIA NeMo based on Hindi and dozens of its dialects, such as Bhojpuri, Dogri, and Maithili. Project Indus 2.0 caters to diverse sectors, including retail, banking, healthcare, and citizen services, in India. It stands out as a state-of-the-art LLM that advances Hindi and dialect conversations. In the future, Indus 2.0 aims to include agentic workflows and support multiple dialects to provide a more nuanced and effective AI solution tailored to India’s diverse linguistic and cultural landscape.
Atul Soneja, Chief Operating Officer, Tech Mahindra, said, “At Tech Mahindra, we are redefining the boundaries of AI innovation. Collaborating with NVIDIA, we are setting a new benchmark for enterprise-grade AI development by seamlessly integrating GenAI, industrial AI and sovereign large language models into the heart of global enterprises and industries.”
Atul Soneja, Chief Operating Officer, Tech Mahindra
IBM introduces Granite 3.0
IBM announced the release of its most advanced family of AI models to date, Granite 3.0. IBM’s third-generation Granite flagship language models can outperform or match similarly sized models from leading model providers on many academic and industry benchmarks, showcasing strong performance, transparency and safety.
Consistent with the company’s commitment to open-source AI, the Granite models are released under the permissive Apache 2.0 license, making them unique in the combination of performance, flexibility and autonomy they provide to enterprise clients and the community at large.
The new Granite 3.0 8B and 2B language models are designed as ‘workhorse’ models for enterprise AI, designed to be finetuned with enterprise data and seamlessly integrated across diverse business environments or workflows.
While many large language models (LLMs) are trained on publicly available data, a vast majority of enterprise data remains untapped. By combining a small Granite model with enterprise data, especially using the revolutionary alignment technique
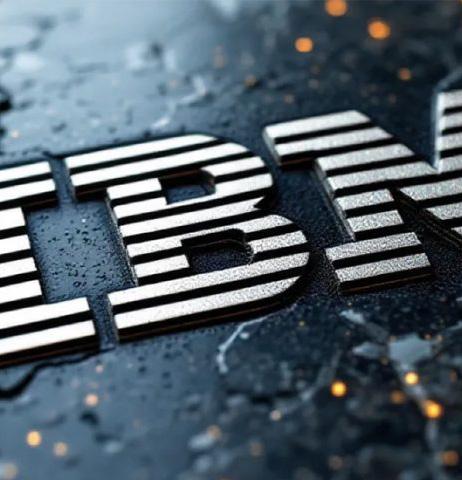
InstructLab – introduced by IBM and RedHat in May – IBM believes businesses can achieve task-specific performance that rivals larger models at a fraction of the cost.
The Granite 3.0 release reaffirms IBM’s commitment to building transparency, safety, and trust in AI products. The Granite 3.0 technical report and responsible use guide provide a description of the datasets used to train these models, details of the filtering, cleansing, and curation steps applied, along with comprehensive results of model performance across major academic and enterprise benchmarks.
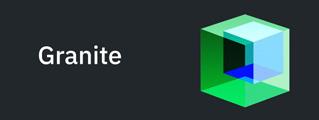
The new Granite 3.0 8B and 2B language models are designed as ‘workhorse’ models for enterprise AI.
Medcare Al Safa introduces revolutionary AI to diagnose over 30 chronic conditions
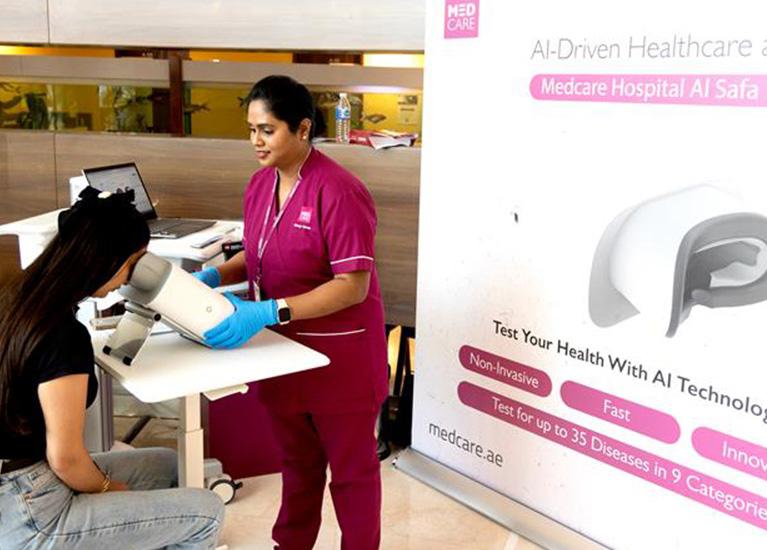
Medcare Hospitals and Medical Centers has implemented Airdoc, a multi-faceted Airtificial Intelligence (AI) retinal image interpretation system, at its Medcare Hospital Al Safa. The region’s first-of-its-kind system diagnoses 35 eye diseases and 9 chronic conditions including hypertension, anaemia, and diabetes in just three minutes,
making it the most efficient system for early finding, auxiliary diagnosis, and health risk assessment of the largest number of diseases in the human body.
The Airdoc retina scanning goes beyond traditional methods, utilising advanced technology to generate substantial data and insights.
ADNOC and AIQ Unveil Agentic AI Solution for Global Transformation
At ADIPEC 2024, ADNOC and AIQ introduced ENERGYai, a custom-built agentic artificial intelligence (AI) solution designed to accelerate the global energy transition. ENERGYai combines advanced large language models with “agentic” AI—specialized AI agents trained to handle specific tasks across ADNOC’s value chain. These AI agents can perform critical tasks with exceptional precision and autonomy, ranging from seismic data analysis to optimizing energy efficiency and enabling real-time process monitoring. The solution is designed to integrate seamlessly into ADNOC’s existing workflows, harnessing the power of machine learning and predictive analytics to enhance decision-making and drive operational efficiency. ENERGYai represents ADNOC’s commitment to pioneering sustainable, data-driven solutions that set new industry standards.
Warburg AI joins NVIDIA Inception Program
Sharjah-based Warburg AI has been invited into the prestigious NVIDIA Inception program, which enables it to access to resources like next-generation hardware and tools, as well as support from industry experts.
Warburg AI’s self-improving AI technology predicts market trends with high accuracy, helping financial institutions make informed investment decisions. For Warburg AI, joining NVIDIA Inception opens doors to cutting-edge technologies and mentorship, empowering the startup to enhance its trading models and scale rapidly.
NVIDIA Inception is a global program designed to support startups in the fields of artificial intelligence (AI), deep learning, and data science.
IFS unveils AI-driven enhancements for greater efficiency and sustainability in oil & gas
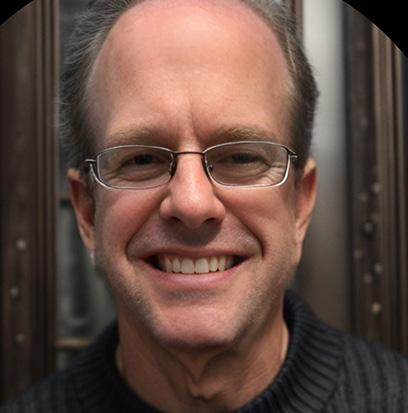
IFS has announced three product enhancements set to reimagine the way upstream operators do business. The new updates leverage the power of IFS.ai to drive new back-office efficiencies and new leasing capabilities help streamline operations and processes for clean energy projects.
First, IFS Energy & Resources, the oil and gas arm and business unit within IFS, unveiled IFS BOLO 15, the latest iteration of IFS BOLO and the next-generation oil and gas accounting solution that possesses the most processing power in the industry to handle any business scenario.
IFS BOLO 15 creates a step change in back-office efficiency through streamlined workflows, a substantial reduction in rework, and the simplification of audits. Powered by IFS.ai and layered with artificial intelligence, this software delivers significant benefits:
Modern Usability: A completely refreshed user interface (UI) that’s both familiar and intuitive, minimizing learning time
Enhanced Security: Multi-layered security that includes features at the code, networking, and data levels for unmatched protection
Second, IFS Energy & Resources customers can add new AP invoicing capabilities included with the BOLO 15 release to expand their IFS Excalibur, IFS Qbyte, IFS IDEAS, and IFS Enterprise Upstream investments. The AP invoicing module powered by IFS. ai provides open, cutting-edge automation to improve cash flow by increasing productivity through reduced processing time, improved accuracy, and optimized payment cycles.
Gupshup launches in Saudi Arabia to bring advanced conversational AI solutions to the Kingdom
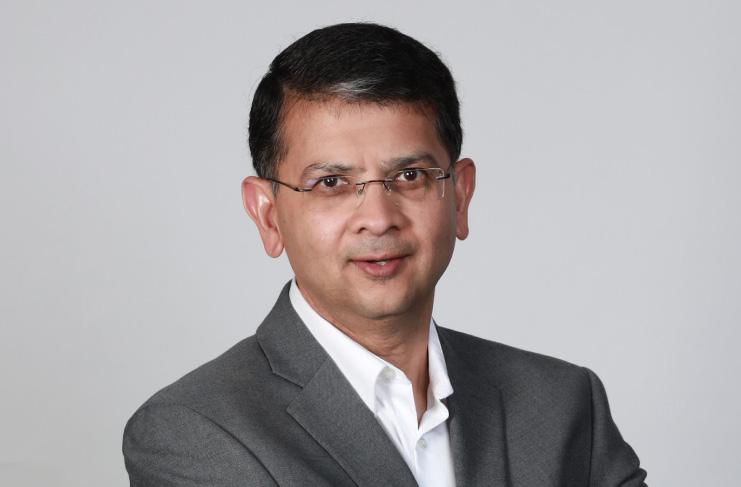
Gupshup has announced its entry into the Saudi Arabian market, marking a significant milestone in the company’s international growth journey. With the launch of Gupshup Technology Gulf Limited, its local arm, Gupshup is set to provide a wide array of conversational solutions tailored to meet the needs of Saudi businesses. Through this expansion, Gupshup will introduce its stateof-the-art Conversation Cloud platform to businesses in Saudi Arabia, empowering them to elevate customer engagement through cutting-edge conversational experiences. With this innovative solution, Saudi businesses can now seamlessly:
Digitize customer interactions using AI-powered agents that facilitate real-time, intelligent conversations.
Automate customer engagement through conversational campaigns.
Drive sales growth via conversational commerce and consultative sales strategies—all through a single, unified platform.
To meet the unique requirements of the Saudi market, Gupshup offers ACE LLM, a domain-specific Generative AI model, that enables the development of highly intelligent, human-like chatbots in Arabic. Conversations are already a key engagement channel in Saudi Arabia.
According to a survey by the Saudi Centre for Public Opinion Polling, 92% of Saudis use WhatsApp, making it an ideal channel for business communications. Gupshup’s presence in the Kingdom will allow brands to leverage its Conversation Cloud to create meaningful customer interactions.
“Saudi Arabia is a pivotal market in our global expansion strategy. We’re witnessing a remarkable surge in business messaging adoption across the Kingdom, driven by a digitally sophisticated consumer base that increasingly prefers conversational interactions with brands. Our commitment goes beyond just providing technology – we aim to be a strategic partner in KSA’s digital transformation journey, helping businesses harness the power of conversational engagement to drive growth and customer satisfaction,” said Beerud Sheth, Co-founder and CEO of Gupshup.
VAP Group set to host second edition of global AI show in Dubai
Web3 and AI consulting giant VAP Group is pleased to announce the second edition of the Global AI Show, taking place on December 12 and 13, 2024 at the Grand Hyatt Exhibition Centre, Dubai. The event will be held under the official support of the United Arab Emirates Minister
of State for Artificial Intelligence, Digital Economy and Remote Work Applications Office.
With its theme of ‘AI 2057: Accelerating Intelligent Futures’, the Global AI Show is set to host C-suite executives, ministry officials and leaders from the world’s
top companies who will explore the cutting edge technological developments across the UAE and the globe.
Beerud Sheth, Co-founder CEO of Gupshup
Wa’ed Ventures earmarks $100 million for investments in Saudi Arabia’s AI sector
Wa’ed Ventures, the $500 million venture capital fund wholly owned by Aramco, announces earmarking $100 million for early-stage AI investments, a bold move to support positioning the Kingdom as a global AI hub.
To aid with strategic deal sourcing and accelerate localisation for global startups, an advisory board consisting of globally renowned leaders in artificial intelligence (AI) has already been appointed by Wa’ed Ventures. The board members come from diverse backgrounds within the AI industry, including policymaking, research, academia, and entrepreneurship, having worked in Meta, Amazon, MIT, Oxford and other toprank institutions.
“Our strategic decision to allocate funds to AI investments is rooted in a deep understanding of the Kingdom’s growing ecosystem. By fostering innovation and supporting AI startups, we aim to accelerate the development of cuttingedge technologies that will drive economic growth, improve quality of life, and position Saudi Arabia as a global leader in artificial intelligence. This investment will not only incentivise local entrepreneurs but also support the localisation of global talent, ultimately unlocking the immense potential of AI,” said Anas Algahtani, Acting Chief Executive Officer of Wa’ed Ventures.
According to a recent report by PwC, Saudi Arabia’s gain from AI is expected to exceed other countries in the Middle East with an estimated $135 billion in value by 2030. This would position artificial intelligence as one of the leading economic drivers, composing more than 12% of the country’s total GDP by 2030.
Wa’ed’s new AI strategy marks another initiative by the fund in keeping with its commitment towards investing in highpotential AI applications and infrastructure players.
PwC Middle East partners with OpenAI to bring latest AI innovation to the region
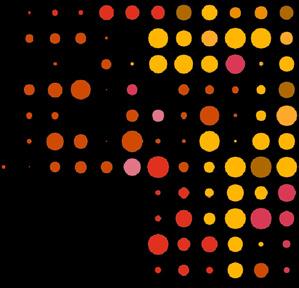
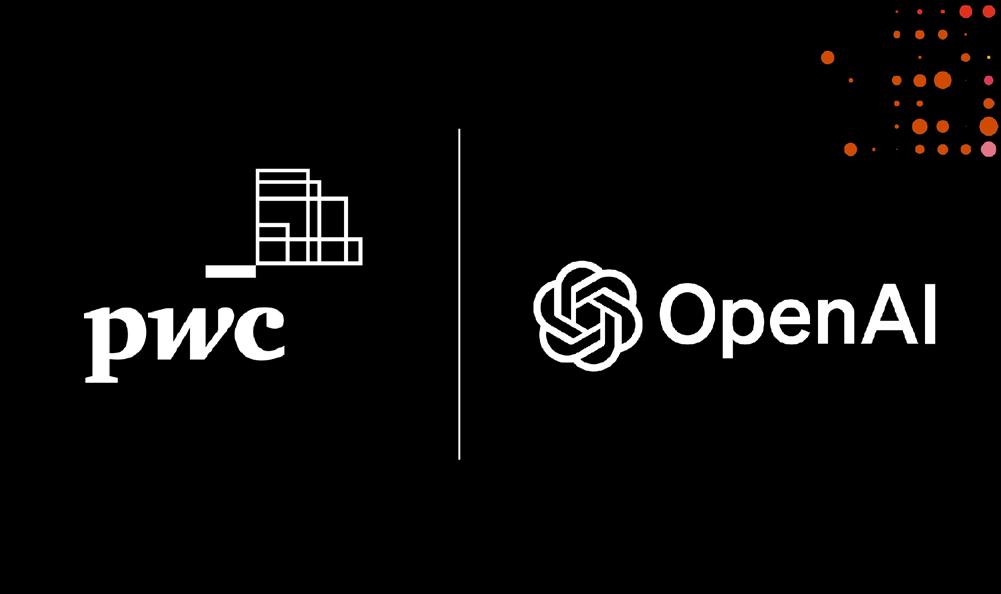
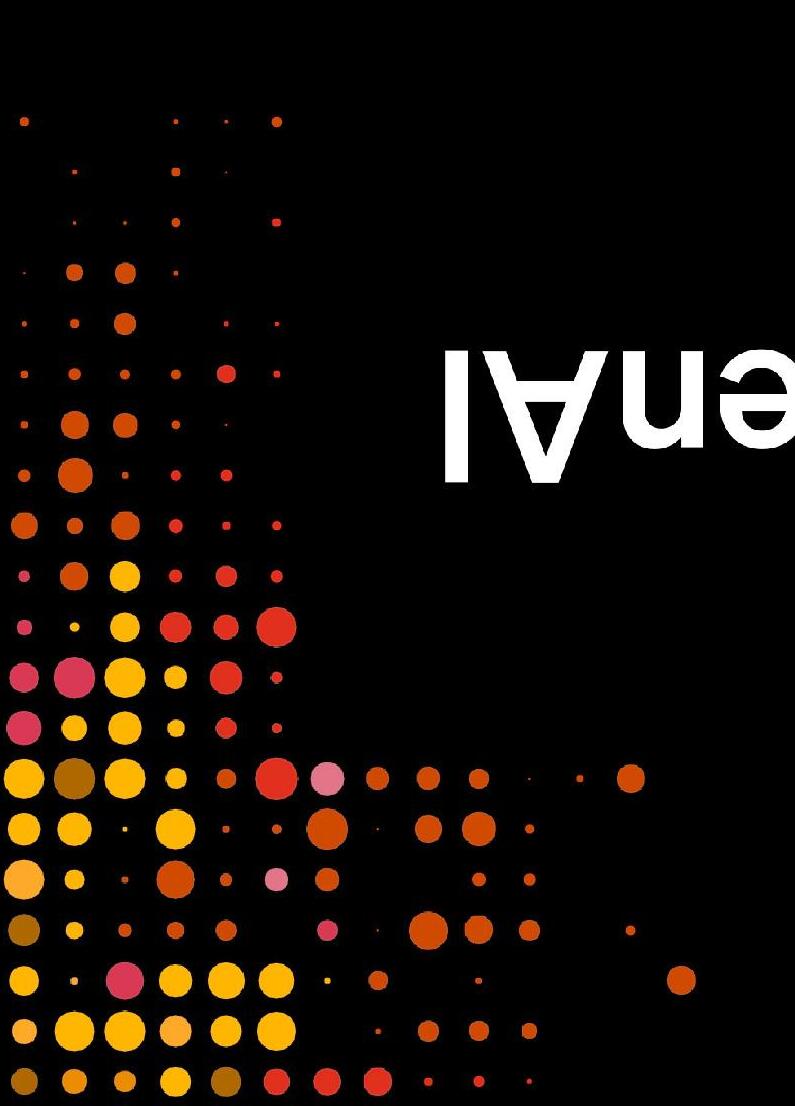
PwC Middle East announced an agreement with OpenAI, becoming the partner and the first reseller of OpenAI in the region. The partnership is the latest advancement of the firm’s investment in artificial intelligence that will enable PwC to scale AI capabilities and drive accelerated impact for organisations in the region.
At a time when business leaders across industries in the Middle East demand outcomes and business impact –and not just potential – PwC’s expanded relationship with OpenAI provides a playbook for companies looking to scale their AI infrastructure, apps, and services.
According to PwC’s 27th Annual CEO Survey, as many as 73% of CEOs in the region believe GenAI will significantly change the way their company creates, delivers, and captures value in the next three years.
The announcement builds on PwC’s successful integration of OpenAI’s capabilities for its workforce where significant positive impact has been witnessed.
Pure Storage Announces Strategic Investment and Technology Partnership with CoreWeave
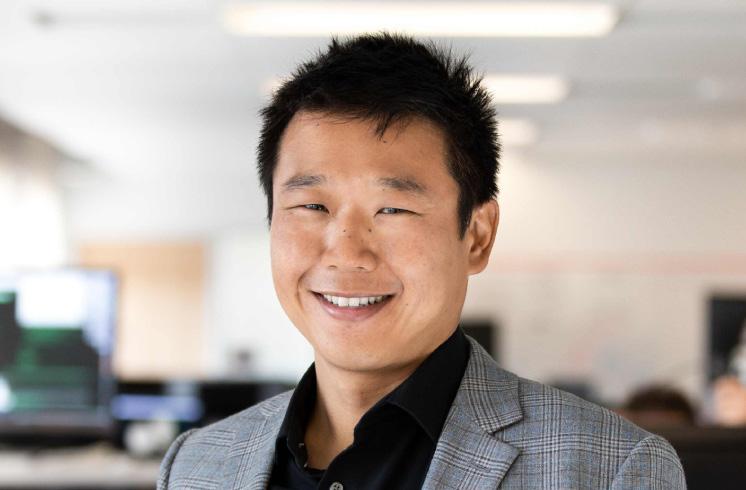
Pure Storage have announced Pure Storage’s strategic investment in CoreWeave to accelerate AI cloud services innovation. Alongside the investment, the companies unveiled a strategic partnership, enabling customers to leverage the Pure Storage platform within CoreWeave Cloud.
Building on their shared success with some of the world’s most advanced AI companies, this collaboration helps to fuel the next generation of AI innovators, driving breakthroughs with CoreWeave’s cloud services and the Pure Storage platform. By adding Pure Storage as a partner, CoreWeave recognises Pure Storage’s 15 years of innovation in flash technologies and its proven track record with some of the world’s
top AI companies.
“Our strategic collaboration with CoreWeave reflects a shared commitment to delivering AI innovation at scale and marks a major milestone in delivering the flexibility and scalability that AI-driven organisations need to thrive. Integrating the Pure Storage platform into CoreWeave’s specialised cloud service environments enables customers that require massive scale and flexibility in their infrastructure the ability to tailor their infrastructure and maximise performance on their own terms,” said Rob Lee, Chief Technology Officer, Pure Storage.
Empowering AI Supercomputers with Cutting-Edge Scale, Performance, and Flexibility
The Pure Storage platform is now available as an option within CoreWeave’s dedicated environments, which customers access through the CoreWeave Platform, a no compromise engineering solution purposebuilt for some of the world’s most compute intensive workloads. The CoreWeave Platform uses automation to simplify complexity, maximising infrastructure performance and efficiency, while Pure Storage offers a highly scalable, efficient storage solution, with joint solutions already deployed in production at supercomputing scale across thousands of GPUs. Together, they empower customers to accelerate their time to market.
ServiceNow partners with NVIDIA to accelerate enterprise adoption of Agentic AI
ServiceNow has announced a major expansion to its strategic partnership with NVIDIA to accelerate enterprise adoption of Agentic AI. The companies will use NVIDIA NIM Agent Blueprints to co develop native AI Agents within the ServiceNow platform, creating use cases fueled by business knowledge that customers simply choose to
turn on.
NVIDIA will collaborate with ServiceNow to map out multiple AI agent use cases. With six years of joint innovation on AI models, along with several previously announced strategic collaborations, ServiceNow and NVIDIA are reshaping how businesses integrate AI into their operations.
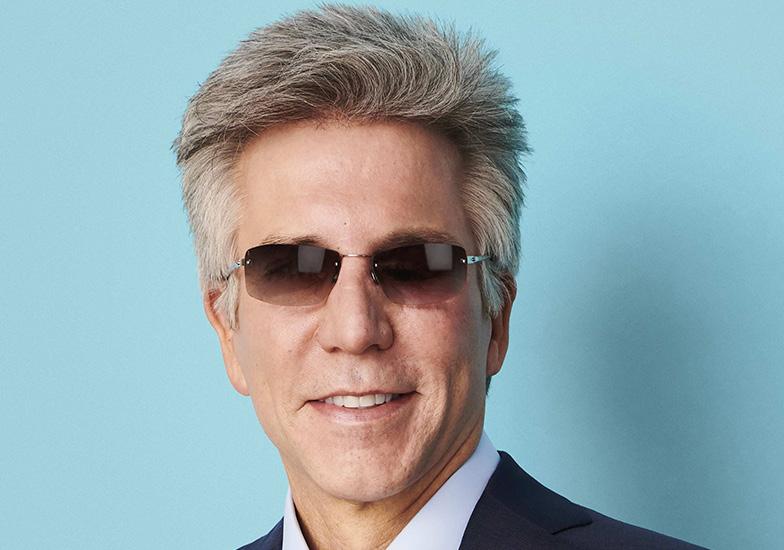
Bill McDermott, CEO, ServiceNow
Rob Lee, Chief Technology Officer, Pure Storage
BlueVerve
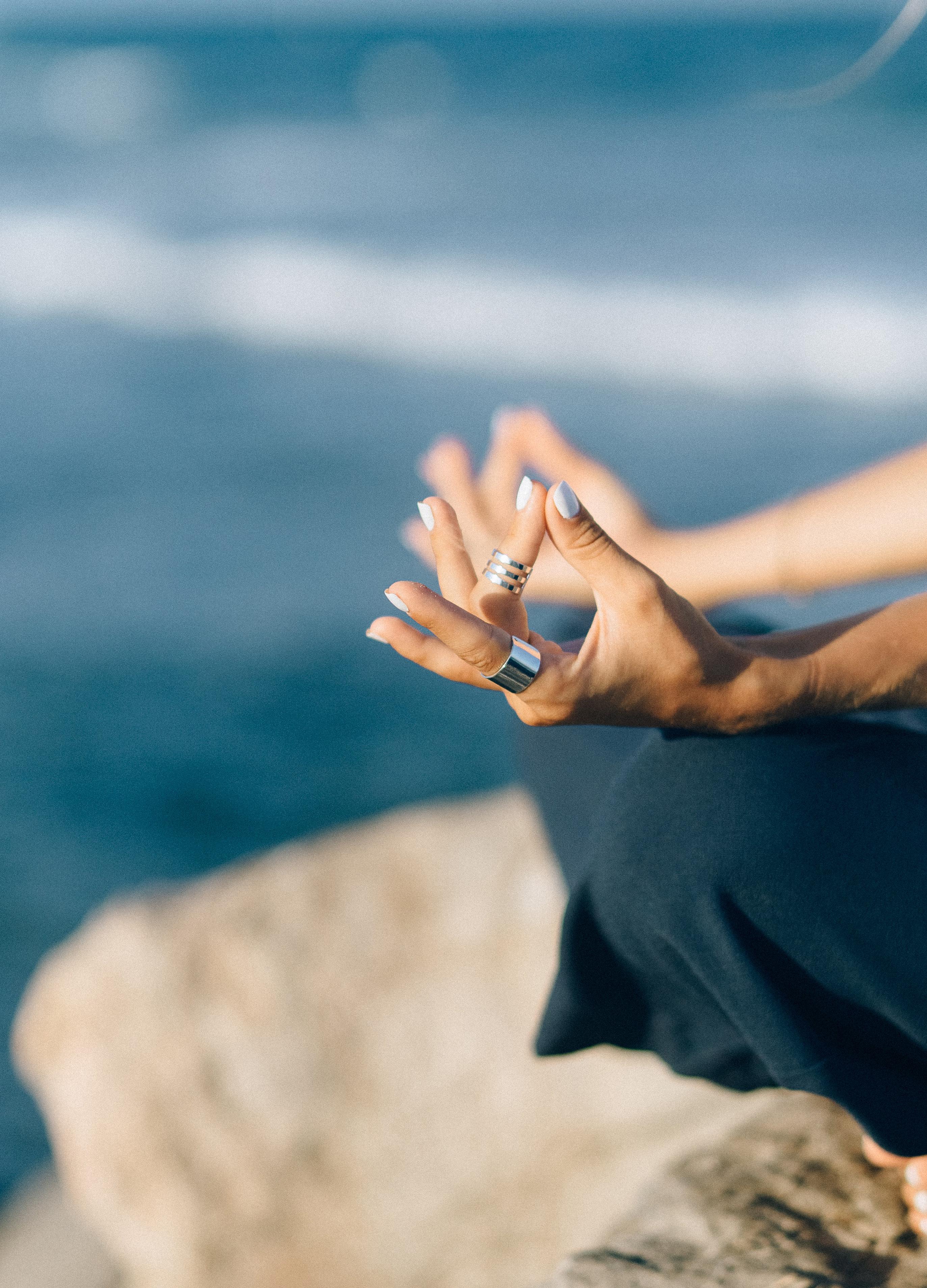
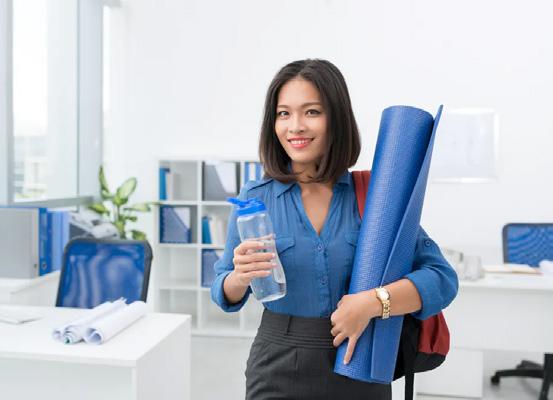

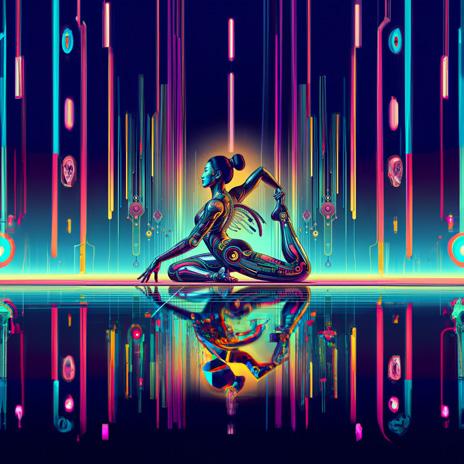
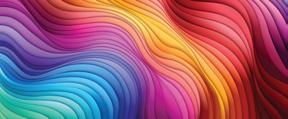
Shifting from reaction to prediction in the age of AI
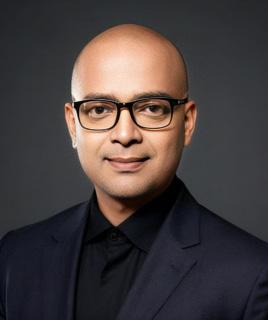
TUSHAR VARTAK
EVP & HEAD OF INFORMATION AND CYBERSECURITY AT RAKBANK, TRACKS THE EVOLUTION OF AI IN CYBERSECURITY.
Cybersecurity has progressed from basic antivirus programs to complex, multi-layered defense strategies. Today, AI is not just an aid but a core component of security that predicts and neutralizes threats before they occur.
Traditionally, cybersecurity investments have focused on prevention, detection, and response. These reactive measures have provided a solid foundation for defending against known threats but often left exploitable gaps for sophisticated attackers. AI introduces a transformative shift, enabling predictive capabilities that can anticipate and adapt to threats in real time.
HOW AI ENHANCES MODERN CYBERSECURITY SOLUTIONS
1. Anomaly Detection and Intelligent SIEM Platforms: Detecting anomalies in real time has been a persistent challenge. Traditional SIEM (Security Information and Event Management) systems provided visibility but relied on preset rules and signatures. AIenhanced SIEM platforms establish baselines of normal activity and identify deviations in real time. Whether through unexpected data transfers or unauthorized access attempts, AI flags these anomalies as potential threats, enabling earlier intervention.
2. Automated Data Classification: Manual data classification processes often led to inconsistencies and oversights. AI-driven tools now automate this process by scanning, categorizing, and labeling data based on its sensitivity. This ensures that critical information, such as client details and strategic assets, is protected with appropriate security measures, reducing the risk of data leaks and supporting compliance efforts.
3. Efficient Incident Triage and Response Recommendations: One major challenge in cybersecurity is the overwhelming volume of alerts generated by security tools, leading to analyst fatigue. AI mitigates this issue by automating incident triage, prioritizing alerts based on severity, and offering response strategies. This not only conserves time but ensures critical incidents receive prompt attention.
4. Mining Telemetry Data for Actionable Insights: The sheer volume of telemetry
data collected from network traffic, endpoint activities, and user behavior can overwhelm security teams. AIdriven solutions analyze and correlate this data at speeds beyond human capability. By processing telemetry holistically, AI can detect complex attack chains and generate actionable insights, guiding teams toward proactive measures rather than reactive responses.
PREDICTIVE CAPABILITIES: A SHIFT TOWARDS PROACTIVE SECURITY
AI’s greatest contribution to cybersecurity is its shift from reactive to predictive capabilities. Machine learning models leverage historical data, global threat intelligence, and internal telemetry to forecast potential threats and strengthen defenses preemptively.
User and Entity Behavior Analytics (UEBA) exemplifies this shift. AI tools learn typical behavior patterns within an organization and flag deviations that could indicate insider threats or compromised accounts. This proactive approach allows security teams to intervene before damage occurs.
Threat Hunting and Simulation: AIpowered threat hunting tools enable cybersecurity teams to identify subtle indicators of compromise that traditional detection systems might miss. Simulating potential attack scenarios helps teams prepare for emerging threats and prevent them from materializing.
AI’S ROLE IN MANAGING TELEMETRY AND ANOMALIES
Cybersecurity tools today generate vast amounts of telemetry data, essential for comprehensive threat monitoring but challenging to analyze manually. AI processes this data to find subtle correlations and anomalies, identifying potential threats before they escalate. By establishing baselines of normal network and user behavior, AI highlights deviations that suggest emerging threats or sophisticated attack tactics.
ADDRESSING AI-POWERED THREATS
While AI strengthens defensive capabilities, it is also being used by attackers to launch increasingly complex and adaptive threats.
This dual use requires multi-layered strategies that not only utilize AI for defense but also anticipate potential adversarial use of AI.
Anomaly Detection in Security Sentinels: AI’s ability to detect deviations from established baselines is critical for identifying stealthy attacks early. This capability helps spot signs such as unexpected data transfers or sudden privilege escalations, enabling security teams to mitigate potential damage in time.
TRENDS TO WATCH IN AIDRIVEN CYBERSECURITY
The future of AI in cybersecurity promises advancements that will shape the field:
1. Generative AI for Threat Simulation: More organizations will use AI to simulate complex attack scenarios, bolstering defenses against emerging tactics.
2. Explainable AI (XAI): As AI becomes more embedded in security operations, explainable models will be essential for providing transparency into decisions and alerts, fostering trust among analysts.
3. Enhanced AI-Human Collaboration: AI will continue to serve as a force multiplier for cybersecurity teams, managing data-heavy tasks so human analysts can focus on strategic decisionmaking.
4. Defensive AI Against Offensive AI: As attackers use AI to develop adaptive threats, defensive AI must anticipate and neutralize these threats proactively.
5. Predictive Patch Management: AI will enhance patch management by predicting vulnerabilities likely to be exploited and prioritizing patches accordingly.
AI has reshaped the cybersecurity landscape, moving the focus from reactive to proactive strategies. With capabilities that range from processing telemetry data and detecting anomalies to automating data classification and triaging incidents, AI empowers security teams to respond quickly and accurately. Balancing AI’s capabilities with human oversight will be crucial to creating adaptive, resilient, and effective security frameworks. The future of cybersecurity lies in an AI-driven approach that anticipates and adapts, keeping defenders one step ahead in an ever-evolving digital battlefield.
AI’s greatest contribution to cyber security is its shift from reactive to predictive capabilities. Machine learning models leverage historical data, global threat intelligence, and internal telemetry to forecast potential threats and strengthen defenses preemp tively.
AI IN CYBERSECURITY
Changing the game
IN AN ERA WHERE CYBERATTACKS GROW MORE SOPHISTICATED BY THE DAY, THE INTEGRATION OF AI IN CYBERSECURITY IS REDEFINING THE FIGHT AGAINST DIGITAL THREATS
AI is transforming functions worldwide, and cybersecurity is no exception. With the global market for AI-powered cybersecurity solutions projected to skyrocket to $135 billion by 2030, the impact of AI on this critical field is undeniable. Today, organizations are leveraging AI alongside traditional security tools to bolster their defenses and address emerging threats effectively.
AI brings a myriad of benefits to the cybersecurity table, including the ability to detect genuine threats more accurately than human analysts, reducing false positives and enabling organizations to prioritize responses based on real-world risks. By analyzing massive volumes of incidentrelated data at high speed, AI allows security teams to respond swiftly, containing threats before they escalate.
Nicolai Solling, Chief Technology Officer, Help AG says AI today plays a pivotal role in augmenting human intelligence, and is a key point as we move towards more automated management of routine tasks, reshaping the operational landscape of cybersecurity. This has revolutionized how we manage technologies, products, and decisionmaking processes.
AI allows analysts to concentrate on more complex issues where human expertise is essential, and AI is also directly used in performing better detection and analysis of behaviour of both users, systems
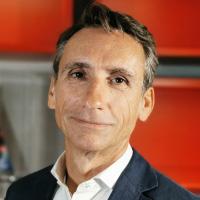
“Upon detection, automated responses can be activated to contain the threat, isolate compromised systems, and initiate incident response workflows. ”
ALAIN PENEL, VICE PRESIDENT – MIDDLE EAST, TURKEY AND CIS AT FORTINET
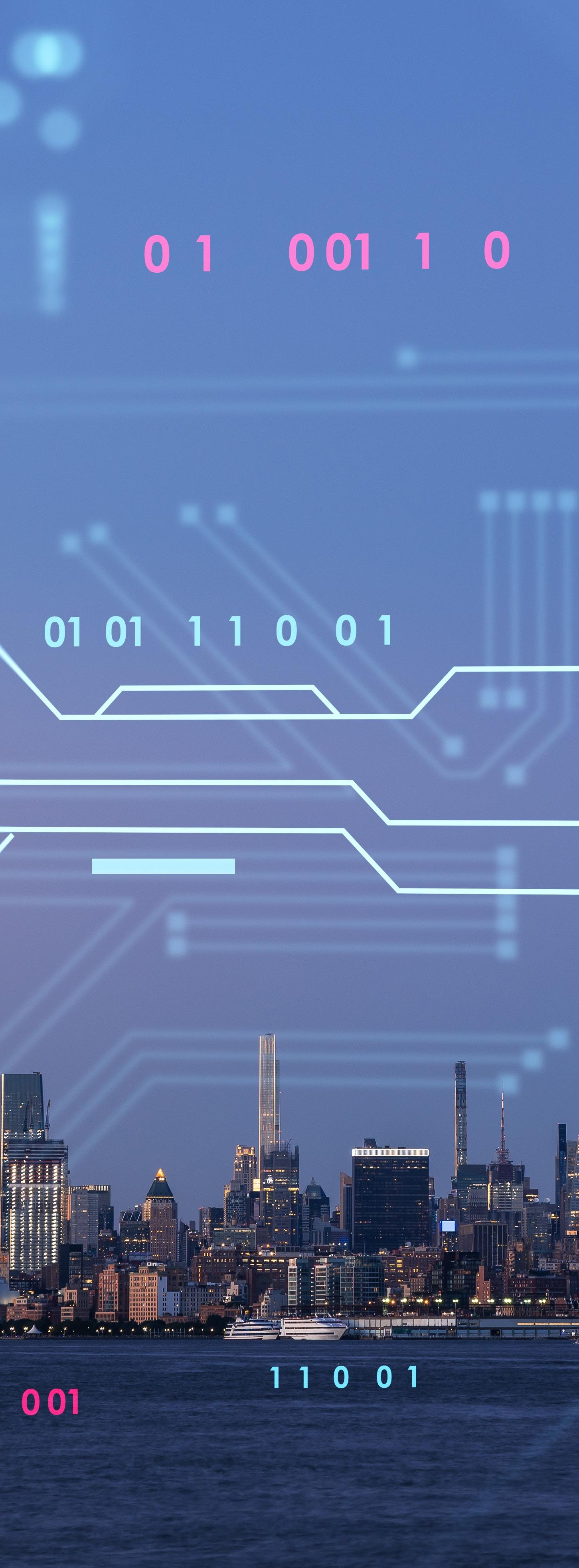
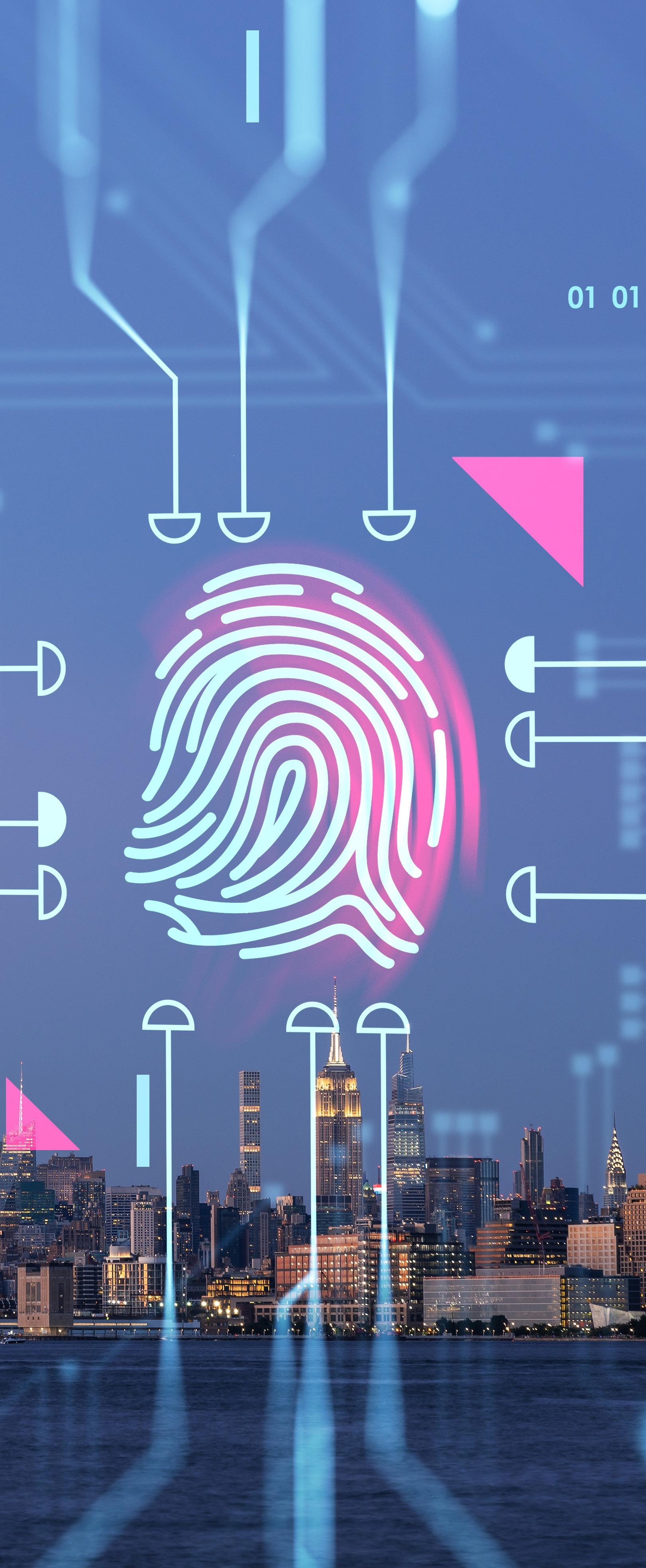
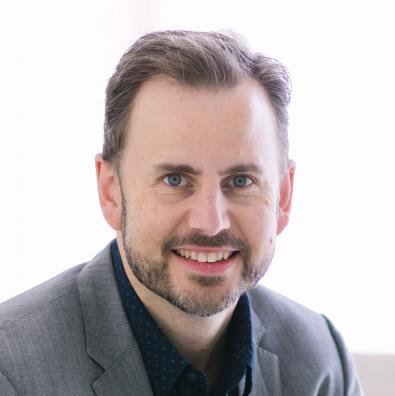
“AI today plays a pivotal role in augmenting human intelligence, and is a key point as we move towards more automated management of routine tasks, reshaping the operational landscape of cybersecurity. ”
NICOLAI SOLLING, CHIEF TECHNOLOGY OFFICER AT HELP AG
and processes, he says.
“The threat landscape has transformed significantly over the past decade,” says Roland Daccache, Senior Manager –Sales Engineering at CrowdStrike MEA. “Adversaries are leveraging technological innovations to break into organizations at record speeds, and they are increasingly shifting their focus to cloud and identitybased attacks. We are entering an era of a cyber arms race where AI will amplify the impact for both the security professional and the adversary. Organizations cannot afford to fall behind, and the legacy technology of yesterday is no match for the speed and sophistication of the modern adversary.”
Stefan Leichenauer, VP Engineering, SandboxAQ, says what makes AI a powerful tool in cybersecurity is its ability to learn from data. “Traditional approaches might use a mix of manual scans and rule-based processes, but AI can analyze large datasets to identify patterns and anomalies. For example, AI can monitor network traffic and look for suspicious behavior that might not be so obvious to the human eye or follow any sort of simple pattern.”
AI also offers a significant advantage over traditional methods by enabling faster

“For highertier threat hunters, AI facilitates algorithmic threat hunting, making it significantly easier to identify and analyze potential threats.”
RICHARD SEIERSEN, CHIEF RISK TECHNOLOGY OFFICER, QUALYS
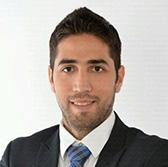
detection and response to cyberthreats. This is due to its ability to process and analyze massive volumes of data in realtime, allowing organizations to identify and mitigate risks with unprecedented speed and precision.
“They can analyze patterns and detect anomalies indicating a cybersecurity threat, such as unusual network traffic or suspicious user behavior – taking some of the manual load off threat analysis teams. Once a threat is identified, AI can automate the response by isolating affected systems, blocking suspicious IP addresses, or patching vulnerabilities,” says Saif AlRefai, Solution Engineering Manager at OPSWAT.
Alain Penel, Vice President – Middle East, Turkey and CIS at Fortinet, highlights AI-driven automation can ensure more swift responses to potential threats. Upon detection, automated responses can be activated to contain the threat, isolate compromised systems, and initiate incident response workflows. This, combined with adaptive learning, ensures that AI models continuously evolve to counter new threat vectors and attack methodologies.
For higher-tier threat hunters, AI facilitates algorithmic threat hunting, making it significantly easier to identify and analyze potential threats, according to Richard Seiersen, Chief Risk Technology Officer, Qualys. “However, while AI can streamline many processes, it cannot replace the nuanced reasoning and judgment of seasoned cybersecurity practitioners. Therefore, rather than replacing, the integration of AI enhances traditional methods by accelerating detection and response times, enabling teams to address
“Adversaries are leveraging technological innovations to break into organizations at record speeds, and they are increasingly shifting their focus to cloud and identitybased attacks. ”
ROLAND DACCACHE, SALES ENGINEERING AT CROWDSTRIKE MEA
threats more effectively while still relying on human expertise for critical decisionmaking,” he says.
Morey Haber, Chief Security Advisor, BeyondTrust, adds another perspective : “AI can detect and respond to cyber threats faster than tradition methods because of
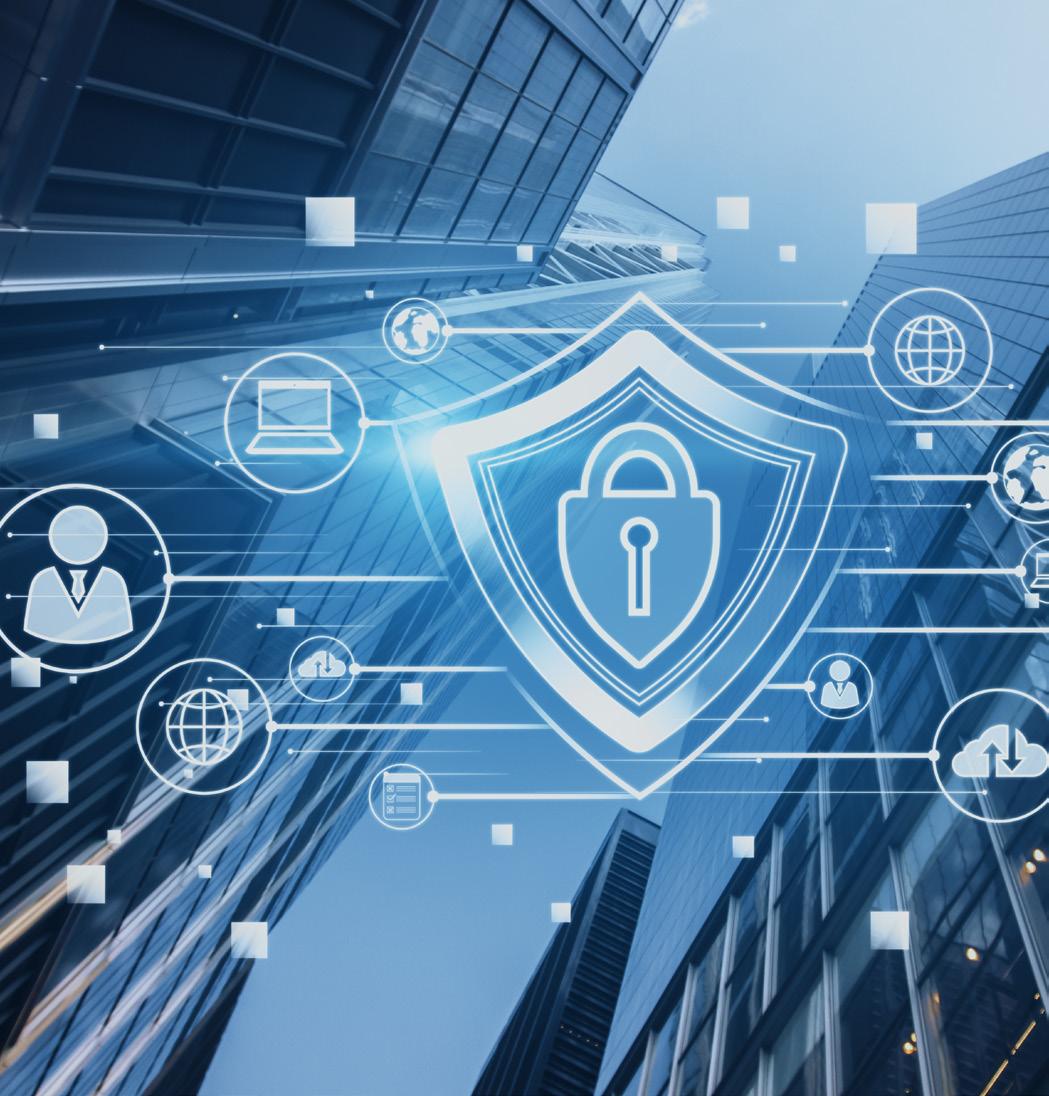
computation speed in which models can identify anomalies in vast quantities of data. For a human, threat hunting involves advanced filters, data linkage, and experience, to identify when extra information is present, attributes are incorrect, or when critical data is missing. Data analytics can perform some of these actions with signatures and rules, but AI can identify when something occurs unlike something that has “ever” been seen before.”
When AI emerged as a significant topic in cybersecurity, many believed it would empower attackers to develop super malware capable of bypassing any cybersecurity defenses. However, this has not materialized. Malware and attacks continue to rely on the same techniques and capabilities we have historically encountered.
However, Solling says there is one area where AI has fundamentally changed our industry: the economy of producing high quality attacks has been permanently altered, which means that we are now dealing with higher quality attacks at greater volumes, which in turn necessitates fundamental changes to the operational model for clients when they are thinking about cybersecurity.
A good example is generative AI, which in increasingly advanced iterations has exceptional benefits for society, but is also a powerful tool to generate more sophisticated and frequent phishing attacks.

“In the region, a recent report indicated that 92% of surveyed organizations experienced at least one successful phishing breach in 2023, up from 86% the previous year. This surge is largely due to the ease with which language-processing capabilities in AI chatbots can generate convincing, automated attacks,” points out Solling.
Industry experts say its also important to balance the benefits of AI with the need for human oversight in decision-making.
Maintaining a balance between leveraging AI for security and upholding transparency and ethical practices is crucial for the enterprise to protect consumer interests. While AI technologies strengthen cybersecurity measures, organisations must also ensure the best possible experiences to maintain trust in the system.
“To achieve this, organisations should be transparent with consumers about their use of AI applications to protect their data and mitigate security risks. Clear consent from consumers should also be required to ensure that their data is only used for its intended purpose. Organisations should also be aware that AI algorithms can be inadvertently unfair and biased in data relating to gender, race, ethnicity, educational background, and location. These biases can lead to limited access to things like fair credit scoring, investment strategies, and customer service for certain individuals. Proper application and understanding are necessary to ensure that this is not the case,” says Penel from Fortinet.
FUTURE TRENDS
Accrording to Seiersen from Qualys, we can expect significant improvements in operational and capital efficiency for defenders, as AI continues to automate routine tasks and streamline processes, says Qualys. This will free security practitioners to focus on more complex challenges, particularly those involving “irreducible uncertainty”—situations where the risk cannot be fully understood through empirical data.
Leichenauer from SanboxAQ highlights one important trend of the next five years will be the rollout of quantum-resistant encryption, also known as post-quantum cryptography. This will necessitate a massive need for risk assessments and migrations to the new quantum-resistant standards, and AI will be used to assist that process. In addition, the prevalence of AI attacks will
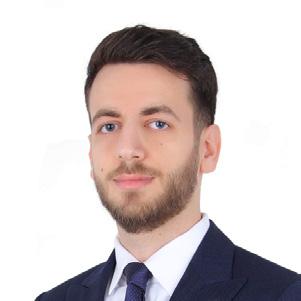
necessitate organizations rapidly moving to more secure paradigms like zero-trust architecture.
Solling from Help AG says the role of AI in automating processes will continue to expand. AI will streamline tasks like creating precise, client-ready responses by summarizing information from support tickets, allowing teams to focus on more complex, strategic issues. AI-driven security assessments, already in use, will likely
“Once a threat is identified, AI can automate the response by isolating affected systems, blocking suspicious IP addresses, or patching vulnerabilities.”
SAIF ALREFAI, SOLUTION ENGINEERING MANAGER AT OPSWAT
become even more central to identifying vulnerabilities in clients’ environments. It’s essential for organizations to leverage AI to ensure secure software development practices, as attackers may use similar tools to exploit vulnerabilities in software.
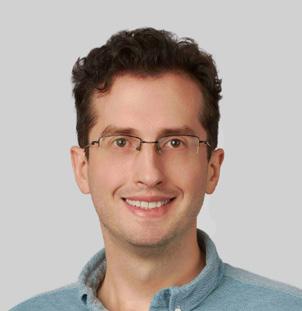
“Traditional approaches might use a mix of manual scans and rule-based processes, but AI can analyze large datasets to identify patterns and anomalies.”
STEFAN LEICHENAUER, VP ENGINEERING AT SANDBOXAQ
He adds as we forge ahead in this journey through an AI-powered environment, it’s important to note that, for all their extraordinary capabilities, AI-powered applications and large-language models (LLMs) introduce new data security challenges and expand the attack surface. Just as organizations have taken advantage of AI’s ability to streamline workflows, threat actors are using the same technology for their own benefit.
Daccache from Crowdstrike believes AI has moved from its evolutionary phase to its transformative phase. Since AI-native cybersecurity seamlessly integrates different cybersecurity solutions, we can expect it to enable organizations to use the strengths of modern, cloud-native data platforms and cutting-edge AI to analyze vast datasets, identify patterns, and strengthen security posture.
“As adversaries reach new heights of attack sophistication with AI, organizations must be equipped to meet them on the battlefield with an equal, if not superior, response. Things like conversational AI will make security teams faster, more productive and help them to learn new skills, which is critical to beat the adversaries in the emerging generative AI arms race,” he concludes.
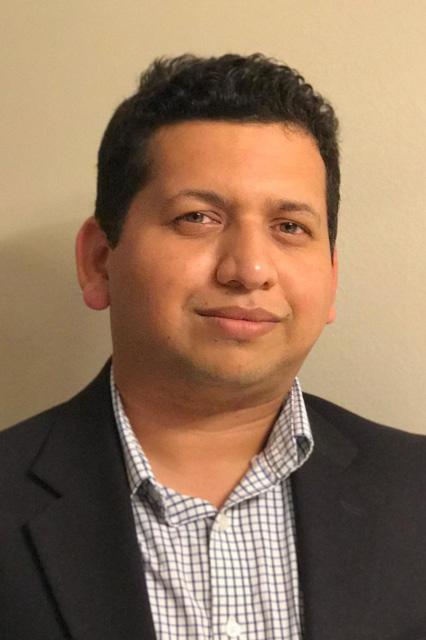
INTERVIEW
SUBODH DESHPANDE
DIRECTOR OF CLOUD
CONSULTING FOR THE MIDDLE EAST REGION, SEARCE, SAYS BUSINESSES NEED TO LOOK AT PROCESS FIRST AND AI SECOND TO UNLOCK ITS TRUE POTENTIAL.
“Bridging the gap”
The UAE has made significant strides in AI by appointing a Minister of State for AI and launching initiatives like the ‘One Million Prompters’ program. How do these initiatives reflect the UAE’s long-term vision for AI adoption across industries?
The UAE’s ambition to become a global AI leader is fast becoming a reality. Appointing a Minister of State for AI was a world first, and this encapsulates how the country is showing up to this next major technology shift. But it’s not just about being firstmovers with new takes on leadership, the UAE is investing in grassroots skills to ensure AI adoption is both broad and sustained.
The “One Million Prompters” program, a global initiative to train one million people in prompt engineering over three years, aims to equip individuals with the skills to leverage AI for innovation and economic growth.
It includes a Global Prompt Engineering Championship to identify and reward top talent, with future plans to expand the championship to include more categories and attract more participants.
Prompt engineering is fundamental for widespread AI adoption and usage. This initiative will empower individuals to utilize AI services like ChatGPT or Gemini effectively, fostering a proactive approach to problem-solving. Integrating GenAI solutions into daily life will simplify tasks, enhance efficiency, and spark curiosity, leading to further exploration and innovation. Ultimately, this initiative has the potential to inspire widespread adoption of newage technologies, encouraging individuals to leverage AI’s capabilities for societal advancement. What challenges do organizations face
in moving from proof of concept to fullscale AI deployment, and how can they overcome these obstacles?
Organizations face significant challenges when moving from an AI proof of concept (PoC) to full-scale deployment. We can break the journey down into three key phases:
Envisioning AI projects
AI PoCs typically start with one of two approaches. The approach most frequently employed, sees organizations begin with an exploration phase to understand AI’s potential within their business. Often led by C-level executives, this exploration stage can lead to longer adoption timelines, as both business leaders and technical teams must undergo a learning curve to grasp AI’s capabilities and limitations. The second approach is more use-case-driven, with a clear strategy aligned to business objectives. This model is common in enterprises with greater technological maturity, where PoCs are designed with specific goals and measurable outcomes. In such cases, teams generally work with fixed, cleaned data sets and avoid complex integrations with other systems, allowing them to measure success within a controlled environment and usually meet expected outcomes.
Navigating production challenges
As organizations shift from PoC to production, demonstrating business value becomes critical, as quantifiable results are necessary to secure resources and organizational buyin. Data quality issues also emerge as models require clean, consistent, and unbiased data to function effectively at scale. Running costs can be considerable, requiring careful planning to manage infrastructure and operational expenses. Additionally, quality assurance through rigorous testing and monitoring is essential to maintain model accuracy and reliability.
Team expertise is another essential factor, as deploying AI solutions requires skills in AI development, data engineering, and DevOps. Risk management must also be proactive, as identifying and mitigating potential issues early can prevent costly setbacks. Lastly, security becomes paramount, as protecting sensitive data and models from unauthorized access is critical.
Most businesses encounter obstacles in demonstrating clear business value, ensuring data quality, and managing costs. However, in more technologically advanced organizations, teams address these challenges with greater ease. Often, these obstacles arise when PoCs are pursued in
silos by either IT or business units, resulting in misaligned process flows and a lack of integration planning with existing systems.
Overcoming obstacles
To improve the likelihood of success, organizations should begin with a strategic, collaborative approach. Identifying processes that would benefit most from AI can help ensure projects are tackling the right issues. It’s also important to ask whether AI is the best solution or if simpler automation could suffice, and to validate the potential benefits, such as cost savings, efficiency, or improved customer satisfaction. Thought should be given to how the AI solution will integrate with existing workflows and whether end-users are prepared to adopt it. Ensuring data quality by preparing a representative dataset for production deployment is crucial to avoid performance discrepancies. Bringing together IT and business teams with the right skills and shared objectives for AI deployment can bridge gaps that might otherwise impede progress. Compliance requirements should also be considered early to avoid regulatory complications down the line.
In your experience, what are the most common gaps between AI strategies discussed in boardrooms and the realities of implementing those strategies on the ground?
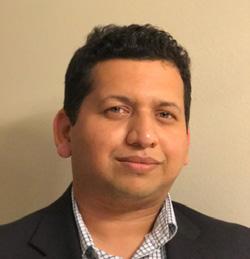
Ensuring data quality by preparing a representative dataset for production deployment is crucial to avoid performance discrepancies.
One of the most common disconnects is overestimating the business benefits of AI, with executives often expecting immediate, sizable profit increases from AI deployment. This expectation overlooks the gradual nature of AI value realization and the investment in time and resources required to achieve meaningful outcomes.
Another key gap lies in underestimating the need to prepare teams for AI projects. Successful AI implementation requires a workforce that understands and can support the technology, yet many strategies fail to prioritize team readiness, leaving a critical gap in execution. A related oversight is the assumption that relevant, high-quality data will be readily available. In reality, data quality and suitability are often major challenges, impacting the accuracy and efficacy of AI models if not addressed from the outset.
INTERVIEW
Raising the bar
Russell Hammad, Founder and CEO of Zenith Technologies, explains why Dubai’s new AI security policy is setting a benchmark for global cities.
Dubai’s new AI security policy is considered a pioneering initiative. How do you see this policy influencing AI security strategies in other global cities?
Dubai’s AI security policy sets a precedent for other global cities by establishing a comprehensive framework that balances innovation with security. This policy’s proactive approach, which integrates AI and cybersecurity measures across both physical and digital infrastructures, provides a blueprint for others to follow. It addresses the rapidly evolving threats posed by AI-driven technologies, ensuring robust safeguards are in place to mitigate risks before they materialize. As cities around the world continue to embrace AI, Dubai’s policy can serve as a model for how to implement AI-driven security in a way that enhances public safety without compromising ethical standards or individual privacy.
In your opinion, what are the main challenges cities might face when implementing AI-driven security policies on a global scale?
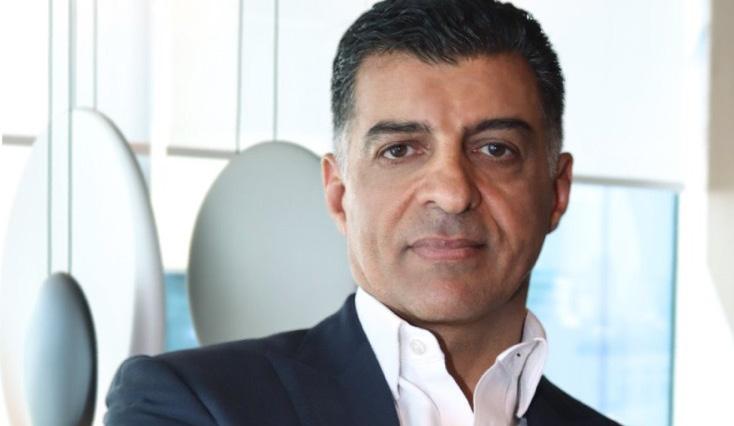
RUSSELL HAMMAD
FOUNDER AND CEO OF ZENITH TECHNOLOGIES

One of the key challenges cities face when implementing AI-driven security policies is balancing innovation with public trust and privacy concerns. As AI evolves rapidly, there is a risk that unregulated or unchecked systems could lead to invasions of privacy or bias in decision-making. Ensuring that AI-driven security solutions are transparent, ethical, and accountable is essential for fostering trust. Additionally, the technical complexity of integrating AI into existing infrastructures presents challenges, especially in terms of realtime connectivity and the need for secure, high-bandwidth networks. Cities must also address the growing risk of cyberattacks as interconnected systems become more widespread, making robust cybersecurity frameworks critical.
What are the key risks or vulnerabilities that arise when AI is integrated into urban infrastructure, and how can they be mitigated?
When AI is integrated into urban infrastructure, it introduces several key risks, such as data privacy issues, system vulnerabilities to cyberattacks, and the potential for AI to make biased decisions if not properly trained. To mitigate these risks, cities need to establish strong governance frameworks that regulate AI development and use, ensuring systems are transparent and accountable. Robust cybersecurity measures are also essential to protect against malicious attacks, especially as cities become more reliant on AI for critical functions like traffic management and law enforcement. Additionally, using a combination of empirical data and simulations during AI training can help eliminate bias and improve the accuracy of AI systems.
Dubai has made significant advancements in AI integration, such as in smart traffic management and predictive policing. What role do these AI applications play in building public trust, and how can they be secured?
AI applications in smart traffic management and predictive policing play a crucial role in enhancing public safety and efficiency, which, in turn, helps build public trust. By reducing traffic congestion, improving response times, and predicting potential criminal activities, these systems demonstrate the tangible benefits of AI integration. To ensure that public trust
continues to grow, these systems must be secured with robust cybersecurity protocols to prevent data breaches or malicious attacks. Furthermore, transparency in how these AI-driven solutions operate and how data is used will help build confidence in their fairness and effectiveness.
What measures should be in place to ensure that AI-driven public services are not only effective but also transparent and accountable to the public? How do you foresee AI transforming other public service areas in Dubai, and what security measures are essential for this transformation?
To ensure AI-driven public services are effective and transparent, it’s essential to train AI models using large, reliable sets of quantitative data. This reduces outlier results, leading to accurate and predictable outcomes, which in turn builds public trust. Transparency is further enhanced by making AI’s data and decision-making processes open and understandable, fostering accountability.
As AI automates more public services, robust security measures must be in place, including credential and biometric layers to prevent identity theft and unauthorized access. This not only protects the system but also maintains public confidence.
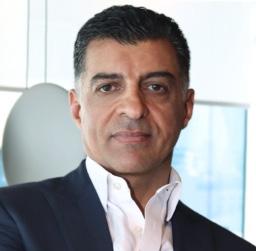
How does Zenith’s partnership with key governmental bodies, such as Dubai Police and other intelligence and security agencies, contribute to the development of AI security solutions in the region?
“One of the key challenges cities face when implementing AIdriven security policies is balancing innovation with public trust and privacy concerns.”
Zenith’s long-standing partnerships with key governmental bodies like Dubai Police have been instrumental in the development of AI security solutions in the region. These collaborations enable Zenith to align its innovative technologies with the specific needs of law enforcement, allowing for the development of tailored solutions that address both current and emerging security challenges. By working closely with these authorities, Zenith has helped deploy AI-powered systems like predictive policing and smart traffic enforcement, which enhance the ability to monitor and respond to security threats in real-time.
GUEST ARTICLE
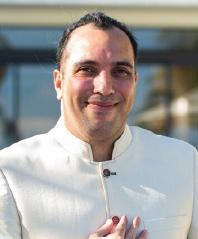
CHRIS
SHAYAN
Head of Artificial Intelligence, Backbase, explores GenAI’s transformation potential in banking and strategies for success
Groundwork for Greatness
Over the last two years, Generative AI (GenAI) has become common knowledge with the widespread adoption of tools like ChatGPT and Gemini having clearly demonstrated its immense potential. In the UAE, it is a segment that is projected to scale at an impressive CAGR of 46.47% up until 2030, when it will be a US$2.036 billion market.
In a country where the banking sector has long been at the forefront of digital transformation, we can expect this cutting-edge technology to revolutionize the industry, unlocking unparalleled opportunities to enhance customer experiences, streamline operations, and drive innovation.
ELEVATED CUSTOMER EXPERIENCES
Traditionally, customer experiences in banking have been defined by static, fragmented, and impersonal interactions — IVRs serve as perhaps the best example of this. By enabling the creation of human-quality text, image, and other creative formats, GenAI can drive a paradigm shift. The technology’s humanlike understanding of the individual needs and preferences of customers can translate to personalized product recommendations or even tailored financial advice.
As for those frustrating rules-based IVRs? GenAI could well make those a relic, replacing them with intelligent chatbots and virtual assistants that handle everything from basic Q&As to sophisticated conversations. Just as with a human agent, GenAI has the ability to effectively trawl a bank’s treasure trove of information to provide their customers with a more natural and intuitive banking experience. Imagine the next time you receive an offer from your bank. You could ask, “Why is this
a good offer for me?” and the bank’s AI-powered chatbot could engage in a humanized conversation, empathizing with your needs and providing tailored explanations.
Moreover, the impressive analytical power of AI means that it can enable banks to conduct A/B testing at scale, rapidly experimenting with different content variations to identify the most effective messaging and designs. This iterative process allows banks to optimize their content strategies, improve conversion rates, and ultimately drive business growth.
OPTIMIZING OPERATIONS AND BOOSTING EFFICIENCY
While applying GenAI to customer facing functions — in the way that was followed for previous generation chatbots — may seem the obvious path forward, it is worth considering the other side of the coin. A foundational element of elevated customer experiences is the optimization of the myriad internal processes that modern banks now depend on. After all, these efficiencies ultimately translate to customers being served faster and more effectively.
AI agents are poised to revolutionize this aspect of the banking industry as well. GenAI can automate tedious tasks currently handled by human employees, such as data entry, report generation, and customer service ticket categorization. It can empower banks to automate various workflows, freeing up valuable human resources for more strategic endeavors. Predictive analytics can drive internal optimizations for banks as well. One area primed for this application is in trend analysis. GenAI can analyze vast datasets to identify emerging trends and predict customer behavior. This information can be used to optimize marketing campaigns, cross-selling strategies, and
GLOBAL CIO EXPERTISE, DRIVING INNOVATION FOR PEOPLE AND PLANET
CONSULTING | RESEARCH | ON DEMAND
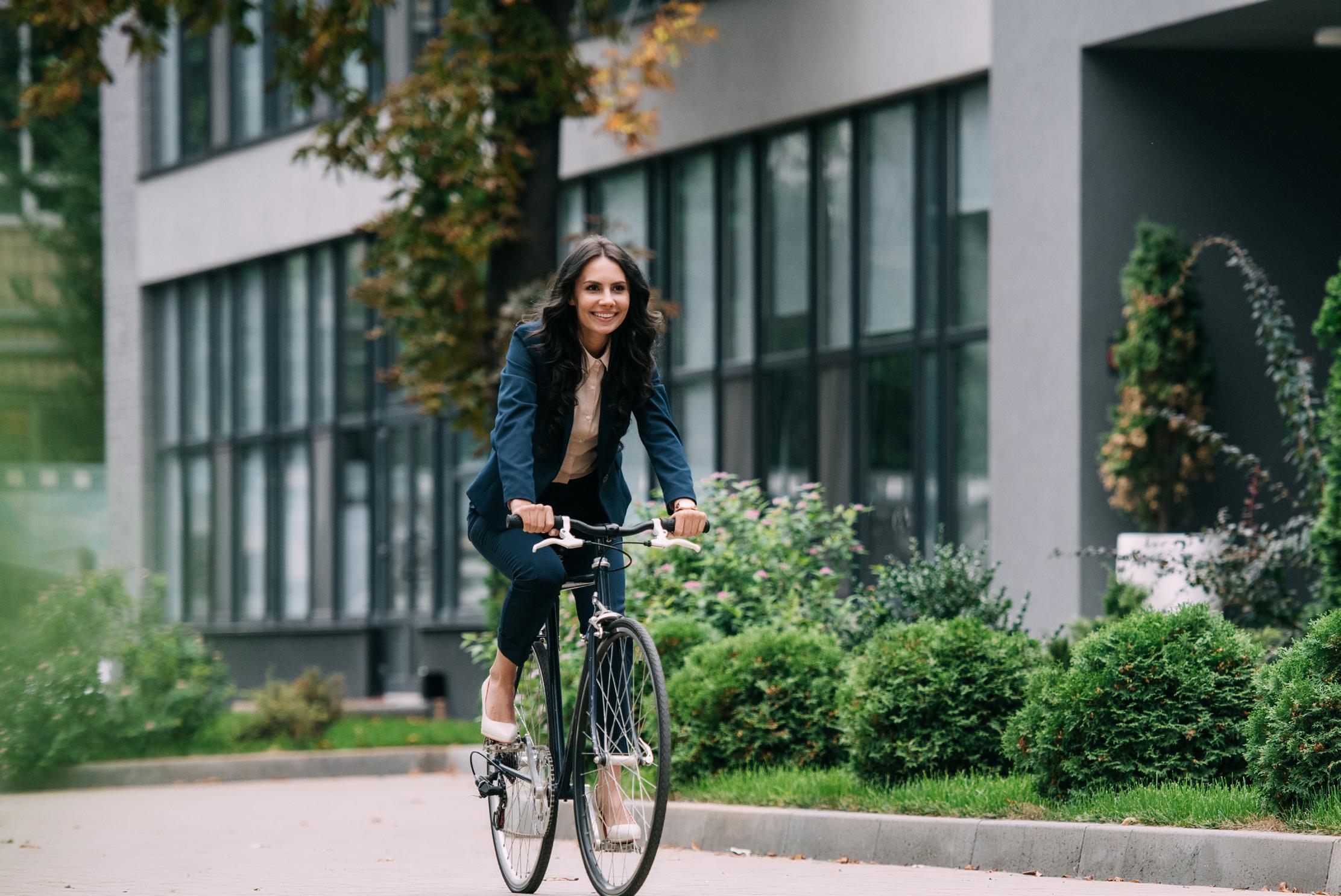
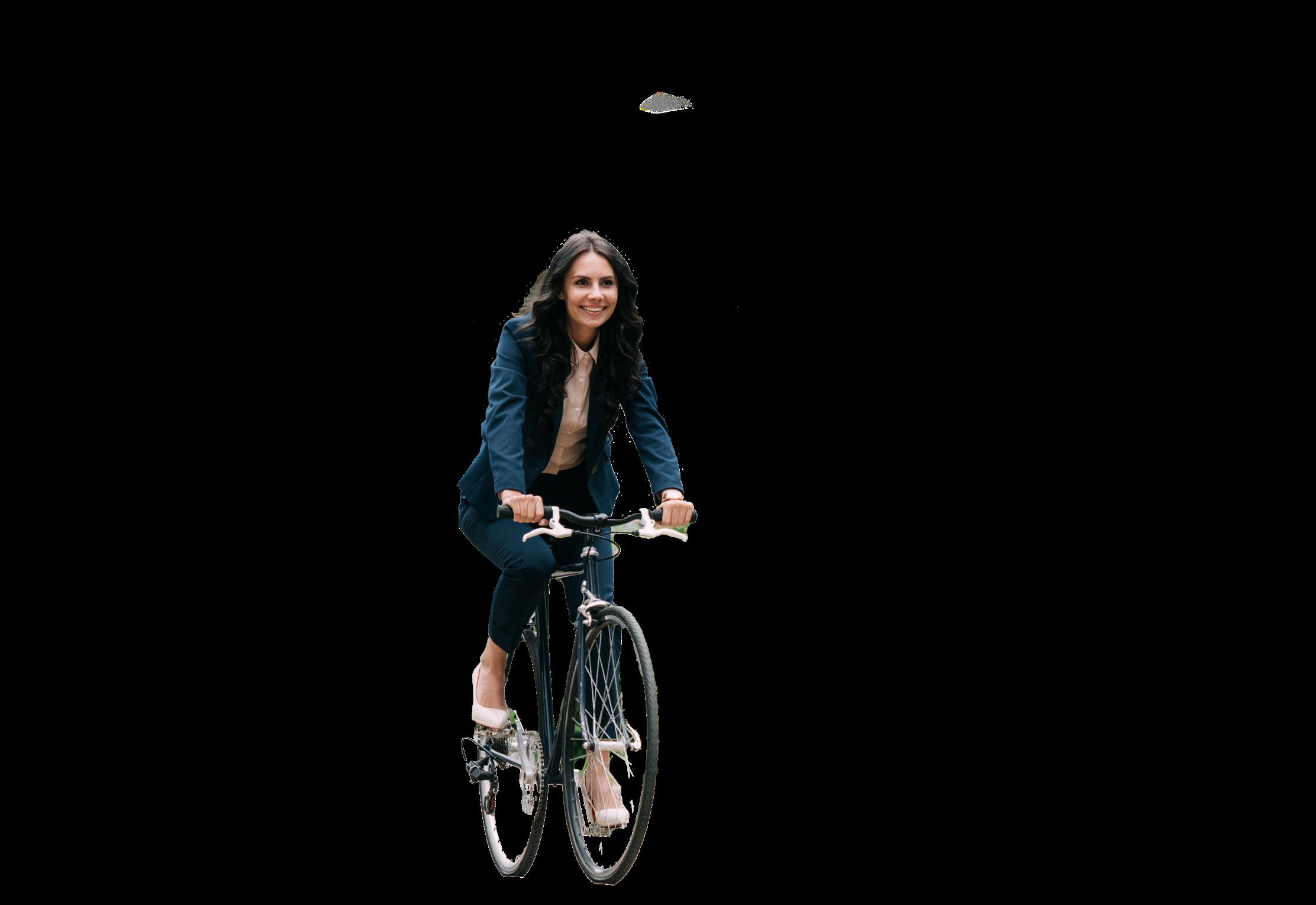
RESEARCH
INSIGHT & BENCHMARKING
EMERGING TECHNOLOGIES
GOVERNANCE
RISK & COMPLIANCE
CYBER SECURITY
DIGITAL TRANSFORMATION
DEOPS & DIGITAL INFRASTRUCTURE
ERP & CRM
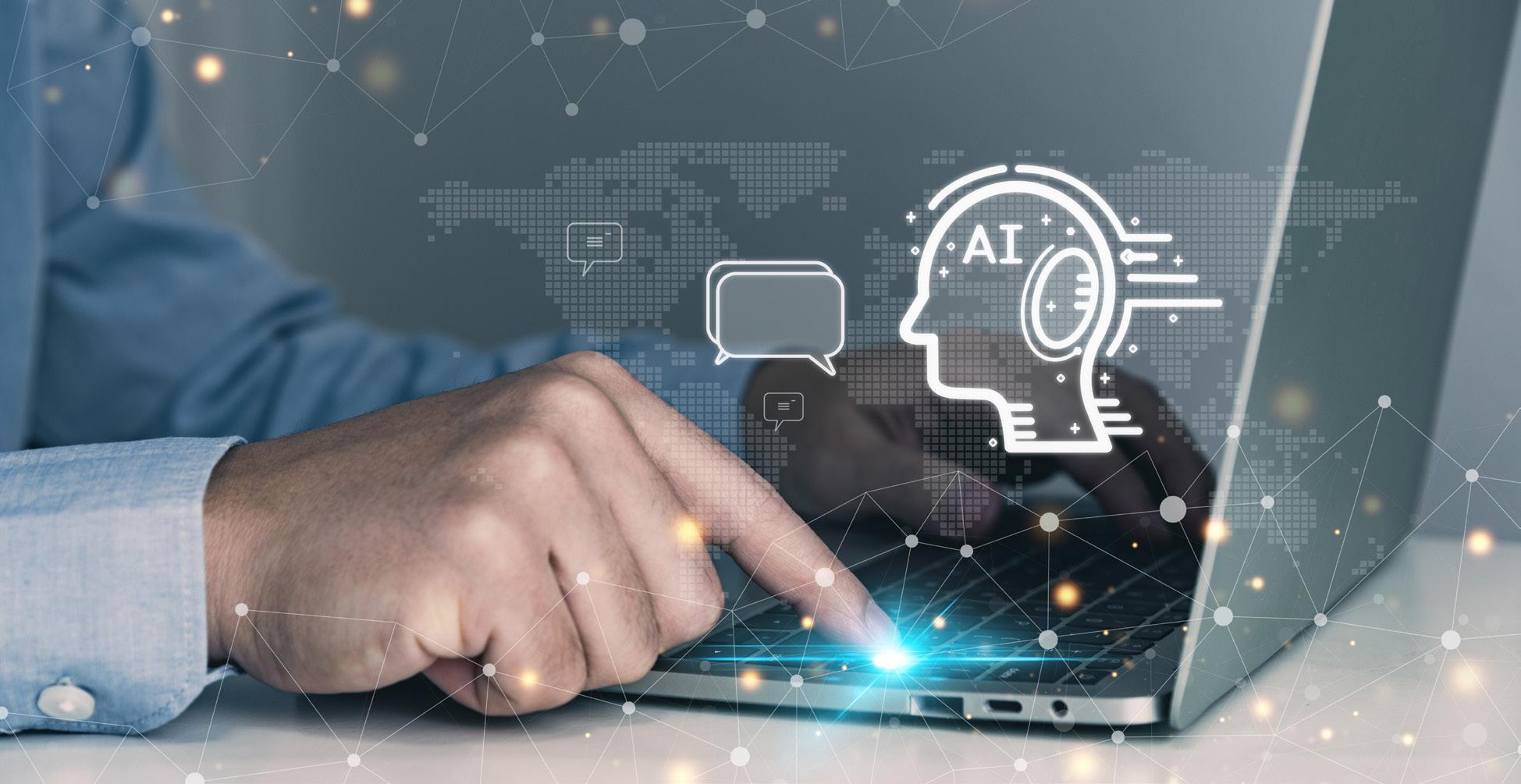
resource allocation.
With its ability to access and analyze a wider range of data points, GenAI can personalize risk assessments, leading to a more accurate picture of each customer’s creditworthiness. Similar techniques can be applied to enhance fraud prevention. These intelligent systems can analyze vast amounts of data to identify potential threats and anomalies, enabling banks to proactively mitigate risks and protect their customers from financial harm. In this way, by leveraging AI agents, banks can strengthen their security posture and build trust with their customers.
LAYING FUTURE-FOCUSED FOUNDATIONS
While the benefits of GenAI are well recognized, many banks are still poised precariously at the cusp. The industry’s previous forays into chatbots have yielded valuable learnings. Industry leaders know that without adequate preparation and maturity, such cutting-edge endeavors will do little more than drum up initial interest before being rapidly rejected. To fully realize the benefits of GenAI, banks must therefore prioritize a number of strategic initiatives.
Unsurprisingly, it all starts with
data. By seamlessly integrating data and AI capabilities, banks can create a comprehensive ecosystem where AI models can access and analyze diverse datasets. This will enable GenAI to provide more accurate and personalized insights, improving customer experiences and optimizing operational efficiency. For example, banks can combine customer transaction data with market trends to offer tailored customer onboarding or identify potential fraud risks.
Empowering AI orchestration should be another focus area for banks. Advanced capabilities like Customer Lifetime Orchestration will allow banks to orchestrate intelligent sequences of actions based on individual customer data. This in turn will enable personalized customer journeys across multiple touchpoints, maximizing product adoption and engagement. For instance, a bank might use AI to recommend relevant products at specific stages of a customer’s relationship, increasing the likelihood of cross-selling and upselling throughout their journey.
Finally, investing in a flexible and scalable Engagement Banking Platform (EBP) will empower banks to easily integrate with new and emerging AI technologies. This will ensure that their infrastructure
remains adaptable to the rapidly evolving AI landscape, allowing them to stay ahead of the curve and capitalize on future innovations. By future-proofing their infrastructure, banks can avoid the risks associated with technological obsolescence and maintain a competitive edge.
TRANSFORMING POTENTIAL TO ESSENTIAL
GenAI — whether applied directly in the service of customers, or working tirelessly behind the scenes — will increasingly become a fundamental differentiator and therefore a musthave tool in the belt of the modern bank. And while the promise of Generative AI in banking is immense, achieving success requires careful planning and strategic investment. By laying the right foundations — integrating data, prioritizing AI orchestration, and adopting flexible, scalable platforms — banks in the UAE can fully unlock the transformative power of GenAI. Those who embrace these strategies will be well-positioned to deliver superior customer experiences, optimize operations, and stay competitive in a rapidly evolving digital landscape. The groundwork for greatness is clear, and the time to act is now.
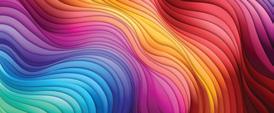
LEADING AI BRANDS TRANSFORMING THE INDUSTRY TODAY
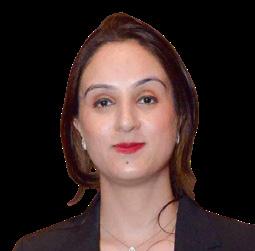
SEHRISH TARIQ
explores leading AI brands shaping the world today

• Founded: 1982
• Market Cap: Approximately $222 billion, sustained by its Creative Cloud suite and digital media solutions.
• Contributions: Adobe pioneered AI in digital media with Adobe Sensei, an AI and ML framework embedded across products like Photoshop and Premiere Pro. Sensei automates tasks such as image recognition and smart cropping, enhancing creative workflows. Recently, Adobe introduced Firefly, a generative AI tool allowing users to create images through text prompts, advancing AI in digital design.
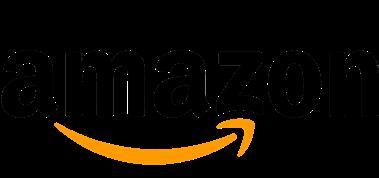
• Founded: 2017
• Valuation: Approximately $9 billion, reflecting its growing impact in defense technology.
• Contributions: Anduril uses AI to enhance national security with products like the Lattice system, which leverages computer vision and sensor fusion for real-time battlefield intelligence. Focusing on autonomous defense systems, Anduril aims to improve situational awareness and security through advanced AIdriven technology.

• Founded: 1994
• Market Cap: Roughly $2.1 trillion, attributed to dominance in e-commerce and cloud computing.
• Contributions: Amazon integrates AI into its operations, including AWS’s AI services like Amazon SageMaker for scalable ML model deployment. AI also powers Alexa, its voice assistant, and personalized product recommendations, positioning Amazon as a leader in making AI accessible across various domains.

• Founded: 2021
• Valuation: Estimated at $4 billion, with backing from Amazon and other investors.
• Contributions: Formed by former OpenAI members, Anthropic specializes in safe and ethical AI, emphasizing AI alignment to ensure that systems align with human values. Its chatbot, Claude, prioritizes safe and reliable AI interactions, underscoring Anthropic’s commitment to responsible AI research and development.
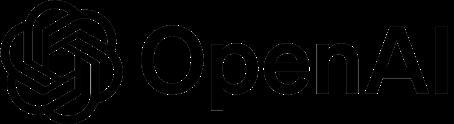
• Founded: 2015
• Valuation: Approximately $157 billion as of its latest funding round.
• Contributions: OpenAI has led the field of generative AI with models like GPT (powering ChatGPT), Codex for coding, and DALL-E for image generation. These tools have set benchmarks in NLP and AI creativity. Focused on ethical AI, OpenAI advocates for transparency and alignment with human values, contributing significantly to policy discussions on AI safety and responsibility.

• Founded: 1998
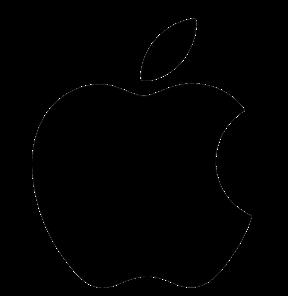
• Founded: 1976
• Market Cap: Approximately $3.4 trillion, driven by innovative hardware and a loyal consumer ecosystem.
• Contributions: Apple integrates AI across its devices, focusing on privacy and usercentric features. The Apple Neural Engine powers AI applications, including Face ID, predictive text, and health monitoring. Apple’s commitment to privacy is reflected in its preference for on-device AI processing, ensuring data security while enabling advanced personalization.
• Market Cap: Around $2.1 trillion, maintained through leading positions in search, advertising, and AI research.
• Contributions: Google is a key player in AI, particularly via DeepMind, its AI subsidiary responsible for breakthroughs like AlphaGo. Google also pioneered the Transformer architecture, essential to today’s language models, and has developed Bard, a conversational AI tool. AI powers various Google services, from language translation to cloud applications, expanding accessibility and usability of AI worldwide.
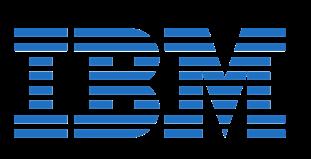
• Founded: 1911
• Market Cap: Approximately $198 billion, focused on enterprise solutions, cloud, and quantum computing.
• Contributions: IBM Watson, known for its Jeopardy victory in 2011, is widely used in healthcare, customer service, and analytics. IBM prioritizes explainable AI, which is transparent and fair, particularly for healthcare and finance. Its AI solutions include Watson Assistant and Watson Health, addressing privacy, ethics, and trustworthiness in AI for various industries.
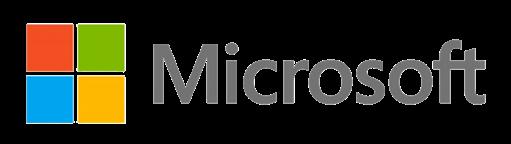
• Founded: 1975
• Market Cap: Approximately $3.1 trillion, bolstered by cloud services, software, and AI investments, including its partnership with OpenAI.
• Contributions: Microsoft’s partnership with OpenAI has brought generative AI into its products, like Microsoft 365 Copilot, enhancing tools like Word and Excel. Azure AI services provide businesses with robust AI tools. Microsoft prioritizes responsible AI, focusing on tools that empower users and businesses while ensuring ethical AI deployment.
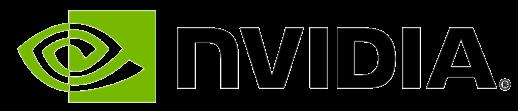
• Founded: 1993
• Market Cap: Around $3.6 trillion, leading the AI hardware and gaming sectors.
• Contributions: NVIDIA dominates AI hardware with its GPUs, crucial for highperformance model training and deployment. Its CUDA platform accelerates AI applications, and the Omniverse platform supports virtual and digital development. NVIDIA’s technology supports generative AI, deep learning, and complex applications across industries, from healthcare to automotive.
GOVERNANCE
THE EU’S AI ACT: ESSENTIAL COMPLIANCE STRATEGIES
A TEAM OF EXPERTS FROM SPECIALISING IN AI COMPLIANCE FROM THE DPO CENTER, A LEADING DATA PROTECTION OFFICER RESOURCE CENTER, EXPLORES SOME OF THE KEY STRATEGIES THAT CAN BE IMPLEMENTED TO KEEP YOUR BUSINESS AHEAD OF THE CURVE AND COMPLIANT WITH THE EU’S AI ACT.
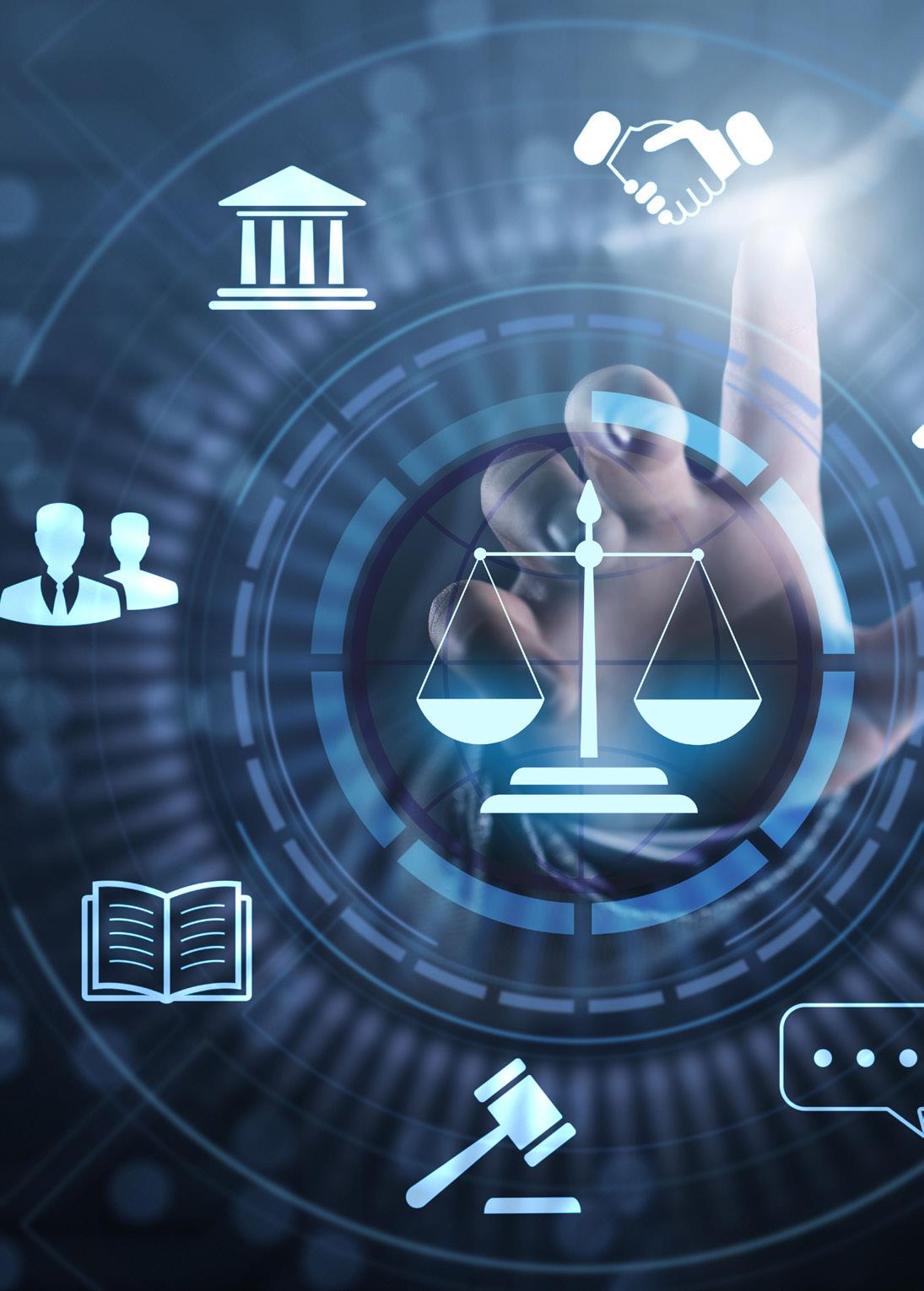
The EU AI Act will come into full effect in August 2026, but certain provisions are set to come into force earlier, such as the ban on systems that perform a range of prohibited functions.
WHAT IS THE AI ACT?
The AI Act establishes a regulatory and legal framework for the deployment, development and use of AI systems within the EU. Taking a risk-based approach, the legislation categorises AI systems according to their potential impact on safety, human rights and societal well-being. Some systems are banned entirely, while systems deemed ‘high-risk’ are subject to stricter requirements and assessments before deployment.
AI systems are categorised into different risk levels based on their potential impact, with the burden of compliance increasing proportionate to the risk. The three main categories are prohibited, high risk and low risk.
AI applications falling into the prohibited systems category are banned entirely, due to the unacceptable potential for negative consequences.
WHO MUST COMPLY WITH THE AI ACT?
Similar to the General Data Protection Regulation (GDPR), the AI Act has extraterritorial reach. This makes it a significant law with global implications that can apply to any organisation marketing, deploying or using an AI system in the EU, even if the system is developed or operated outside the EU.
There are different classifications of use, which dictate the responsibilities and expectations relating to different use cases.. The two most common classifications are likely to label businesses as either Providers or Deployers, but there are also classifications for Distributors, Importers, Product Manufacturers and what will be known as an Authorised Representative.
HOW CAN MY BUSINESS PREPARE?
For organisations developing or deploying AI systems, preparing for compliance is likely to be a complex and demanding task, especially for those managing high-risk systems. But there’s an opportunity to set the foundations of what responsible AI
innovation looks like, so businesses would do well to approach this as more than a simple box-ticking exercise, and instead as a chance to lead in the building of trust with users and regulators alike.
By embracing compliance as a catalyst for more transparent AI usage, businesses can turn regulatory demands into a competitive advantage.
STAFF AWARENESS AND TRAINING
Organisations intending to use AI systems in any capacity should take the time to consider the potential impact of those systems, and engage in an appropriate level of staff awareness training and upskilling. This is an essential element of ensuring team members recognise their roles in compliance and are equipped to implement the AI Act’s requirements.
A complete, detailed training programme should address the key requirements of the AI Act, including any role-specific details. For example, AI developers may need more indepth technical training, whilst compliance officers will focus on documentation and regulatory obligations.
ESTABLISH STRONG CORPORATE GOVERNANCE
For organisations who provide or deploy systems classified as high-risk or General Purpose AI (GPAI), a foundation of strong corporate governance is essential to demonstrate and maintain compliance.
To build and maintain this foundation of strong corporate governance, organisations should aim to pay attention to a few key areas:
• Implement effective risk and quality management systems, which are critical for overseeing and mitigating risks and help to ensure any issues are identified early and can be addressed
• Ensure robust cybersecurity and data protection practices are in place to safeguard sensitive personal data and protect against data breaches
• Develop accountability structures with clear lines of responsibility to ensure compliance efforts are coordinated and effective
• Monitor AI systems on a regular and ongoing basis, reporting on their performance and compliance status
ENSURE ROBUST CYBERSECURITY AND DATA PROTECTION PRACTICES
When it comes to establishing strong corporate governance, it’s vital to recognise the importance of robust cybersecurity and data protection, and in fact these elements are crucial for meeting the requirements of the AI Act.
For cybersecurity aspects, practices should include implementing robust infrastructure security with strict access controls, having a detailed incident response plan, and ensuring regular security audits to identify vulnerabilities.
The data protection requirements of the AI Act overlap with the General Data Protection Regulation (GDPR) in several areas, particularly around transparency and accountability.
PREPARE
FOR UPCOMING GUIDELINES AND TEMPLATES
The EU is developing specific codes of practice and templated documentation to help organisations meet their compliance obligations. Any business dealing with AI systems should be keeping an eye out for updates and further information on these documents, as they will undoubtedly prove useful in compliance measures.
ADHERE TO ETHICAL AI PRINCIPLES AND PRACTICES
For example, AI developers may need more indepth technical training, whilst compliance officers will focus on docu mentation and regulatory obligations.
Although guidelines and practical applications of the AI Act are still to be defined, its core principles are well understood, and reflected in a number of responsible and ethical AI frameworks.
Organisations considering significant AI use – especially in ways that involve personal data or affect individuals – need to understand how an AI system works, its intended use, and its limitations.
Finally, conducting a risk assessment of how the AI system may impact both individuals who interact with it and the organisation’s liability and reputation if anything should go wrong is essential.
SEEK EXPERT GUIDANCE
The AI Act is complex in nature, and with good reason. Any organisations who find themselves unsure of the extent of their obligations should seek professional advice now, in the early stages of the Act’s lifespan, in order to support your compliance journey.
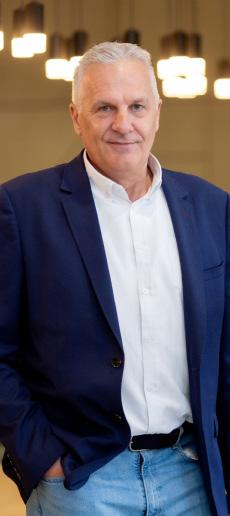
What are some of the challenges organizations face regarding GenAi implementation?
I think there are several challenges that have been faced in the past. For instance, there was an adoption challenge, and it’s taken some time to overcome that hurdle. But we also have to remember that this technology is only two years old; it hasn’t been around for very long. While AI, in general, has existed for about 70 years, Generative AI as a subset is still very new. So, if we say adoption was slow, it was actually quite rapid, all things considered.
Some of the challenges we’re seeing in the market involve the readiness of related technologies, which are beginning to play a significant role. For example, some infrastructures aren’t fully prepared yet. Many companies are dealing with legacy systems and technical debt, which can cause delays and difficulties, especially
From experiments to execution
NTT DATA HAS RELEASED THE FIRST RESULTS OF ITS EXTENSIVE ORIGINAL RESEARCH THAT REVEALS ORGANIZATIONS ARE SHIFTING FROM GENAI EXPERIMENTS TO INVESTMENTS.
CHRIS WIGGETT
DIRECTOR OF AI, NTT DATA MEA
for organizations without those systems in place and in order. However, we’re seeing a lot of progress in this area, with companies preparing to deploy Generative AI on a large scale.
Are you seeing any impactful use cases? Absolutely. I think it’s worth mentioning that, based on the report, while we know there’s a lot of experimentation happening, there’s also a very deliberate shift from experimentation to full implementation,
almost standardizing the use of this technology.
Generally speaking, from a use-case perspective, we’re seeing significant activity around service personalization. In the customer experience (CX) space, for instance, Generative AI tools, with their contextual power, allow us to achieve a highly detailed level of personalization.
In manufacturing and retail, we’re seeing applications in product development, design, and analysis, with quality control being another significant area. Risk assessment is also a key focus, as well as an increased emphasis on automation. For once, Generative AI is enabling us to merge the realms of automation and AI. In the past, we talked about Intelligent Automation, which was a combination of RPA and AI; now, we’re actually seeing these two domains come together effectively.
Across different industries—from automotive and banking to energy and healthcare—the primary objectives often include personalization in service, solution design, and product quality. In industries like manufacturing, while personalization may be less emphasized, the focus shifts toward quality control, risk assessment, and automation.
Overall, the trend across industries starts with personalization of services and solutions, followed by product design, quality control, risk assessment, and then moving down the stack to areas like demand forecasting.
Do you think Gen AI can be integrated into existing infrastructure?
Yes, I think it is a significant factor. Ideally, Gen AI is best suited for the cloud, and there are several reasons why that makes a lot of sense. First, AI—and Gen AI in particular— requires substantial computational power, which means we need robust GPU capabilities. Deploying that level of compute power on-premises can be costly, especially given the vast number of tokens these foundational models process.
From a legacy standpoint, if an organization has older, diverse databases, data integration can also pose challenges. These databases might use different formats or schemas, making it difficult to harmonize and integrate the data effectively. Scalability is another consideration; one of the cloud’s biggest advantages is the flexibility to scale up or down as needed.
Then, there’s the security and compliance
aspect. Centralizing data in one place makes it easier to manage, ensuring data protection and compliance with regulations.
In my experience, many companies are actually quite well-prepared for Gen AI. While there may still be some work to do, a lot of groundwork has already been laid.
So, do you see the cloud becoming an underpinning technology for Gen AI and AI in general?
Yes, it can. Some foundational models can indeed be deployed on-premises, but there are considerations to keep in mind. For instance, how much compute power will you actually need? How large is your data set, and what are the AI requirements? These are critical factors that can turn into budget considerations, which should not be underestimated.
Additionally, the models you can deploy on-premises may not have the same contextual power as the latest cloud-based models. From our perspective, the cloud is the optimal place for deployment, especially as cloud technologies have advanced tremendously over recent years—and even more so in recent months—in preparation for the Gen AI wave.
There’s a lot of discussion around ethical AI and responsible AI. How can enterprises navigate this landscape?
There are a few key considerations when it comes to ethical and responsible AI use. First, we need to be mindful of sustainability. There’s been a lot in the news about the power consumption of these models, with some data centers even considering nuclear plants to meet energy demands. As global citizens and people concerned with the environment, we must take these issues seriously. These models consume significant energy and water, and that’s something we must always keep in mind.
From a responsible use perspective, a lot of work is already underway. I was talking with someone from Uber just before this, and we discussed how much regulation and legislation are emerging around AI globally. This is something we must take very seriously. Regulating AI will be critically important, as these systems require oversight. From both a societal and organizational perspective, executives need to stay involved and keep a close watch on AI developments, as responsible use is essential. Irresponsible use can be exceptionally dangerous.
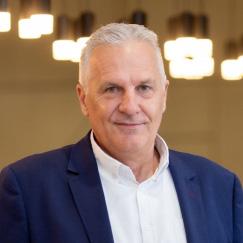
“From a legacy standpoint, if an organization has older, diverse databases, data integration can also pose challenges.”
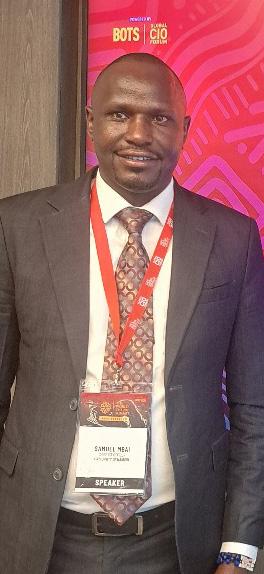
INTERVIEW
SAMUEL MBAI
CHIEF ICT OFFICER, UNIVERSITY OF NAIROBI
The future of learning
In his role as Chief ICT Officer at the University of Nairobi, Samuel Loki Mbai has championed AI-driven innovations that are reshaping educational delivery and enhancing learning outcomes. In this exclusive interview, he talks about the impact of AI in education.
How is AI enabling personalized learning experiences for students, and what are the key benefits in terms of academic outcomes?
AI in education allows us to meet students where they are, tailoring learning experiences to individual strengths, challenges, and pace. Working with the University of Nairobi and IGAD, I’ve seen firsthand how AI can transform learning by adapting course materials and feedback in real time. This helps close knowledge gaps early, boosts student confidence, and makes learning more engaging. These benefits are reflected in improved academic outcomes as students feel more supported in their unique learning journeys and are empowered to learn actively.
How is AI transforming the administrative side of education, such as grading, attendance, and communication with students and parents?
AI-driven tools have brought efficiency and transparency to administrative processes that were traditionally time-consuming. For example, automating grading systems provides students with quick feedback, and AI-based attendance tracking allows us to monitor participation in a non-intrusive way. Additionally, in my work supporting schools like Kitengela International, I’ve implemented AI-based communication tools that keep parents updated on their child’s progress, improving parental engagement and satisfaction. These changes streamline administrative tasks and enhance communication, creating a more responsive and connected educational environment.
What are the advantages and challenges of automating these tasks with AI, and how can it free up more time for teachers to focus on teaching?
Automating repetitive administrative tasks through AI has freed up valuable time for educators to focus on what matters most—teaching. By reducing the burden of grading, attendance tracking, and routine communications, teachers can invest more energy into lesson planning, personalized student support, and curriculum development. However, automation also brings challenges, such as the need to ensure data integrity and protect sensitive information. In my role with IGAD, we addressed these challenges by integrating robust data governance protocols, ensuring that automation remains a tool for
empowerment, not a source of risk.
What are the limitations of AI-powered tutors compared to human instructors, and how can these be mitigated? While AI tutors can provide consistent support and round-the-clock assistance, they lack the empathy, adaptability, and mentorship that human educators bring. AI can guide students through procedural or knowledge-based tasks but struggles with the nuances of emotional support and complex problem-solving. We can mitigate this by positioning AI as a support tool for foundational learning, while leaving complex, critical thinking and motivational support to human instructors. In my experience, a blended approach—where AI complements human instruction—creates a more effective and holistic learning environment.
What are the ethical implications of using predictive analytics in education, especially when it comes to student privacy and bias in AI algorithms? Predictive analytics introduces powerful possibilities but also significant ethical considerations. In my work with educational platforms, we prioritize transparency and fairness by rigorously testing AI models to minimize bias and ensure that algorithms respect student privacy. Predictive models must be used thoughtfully, especially when assessing student potential, as bias can have far-reaching consequences. Constant vigilance, diverse training datasets, and regular audits are key to protecting students’ rights and fostering trust in these technologies.
What role does AI play in helping educators make data-driven decisions to improve teaching methods and overall educational quality?
AI helps educators gather actionable insights from student data, enhancing instructional methods and curriculum design. For instance, through my work on e-learning platforms, I’ve seen how AI can provide insights into which learning resources are most effective, how students are engaging, and where they may need additional support. These insights allow us to continually refine educational approaches, ensuring that they are effective, relevant, and responsive to student needs. AI empowers educators to shift from reactive to proactive decision-making, ultimately raising educational standards.
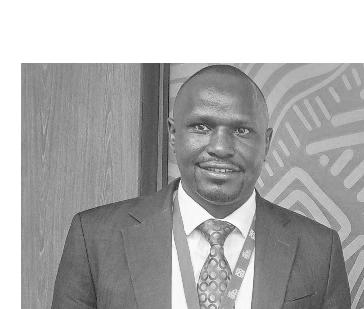
“AI empowers educators to shift from reactive to proactive decisionmaking, ultimately raising educational standards.”
Trust into the spotlight
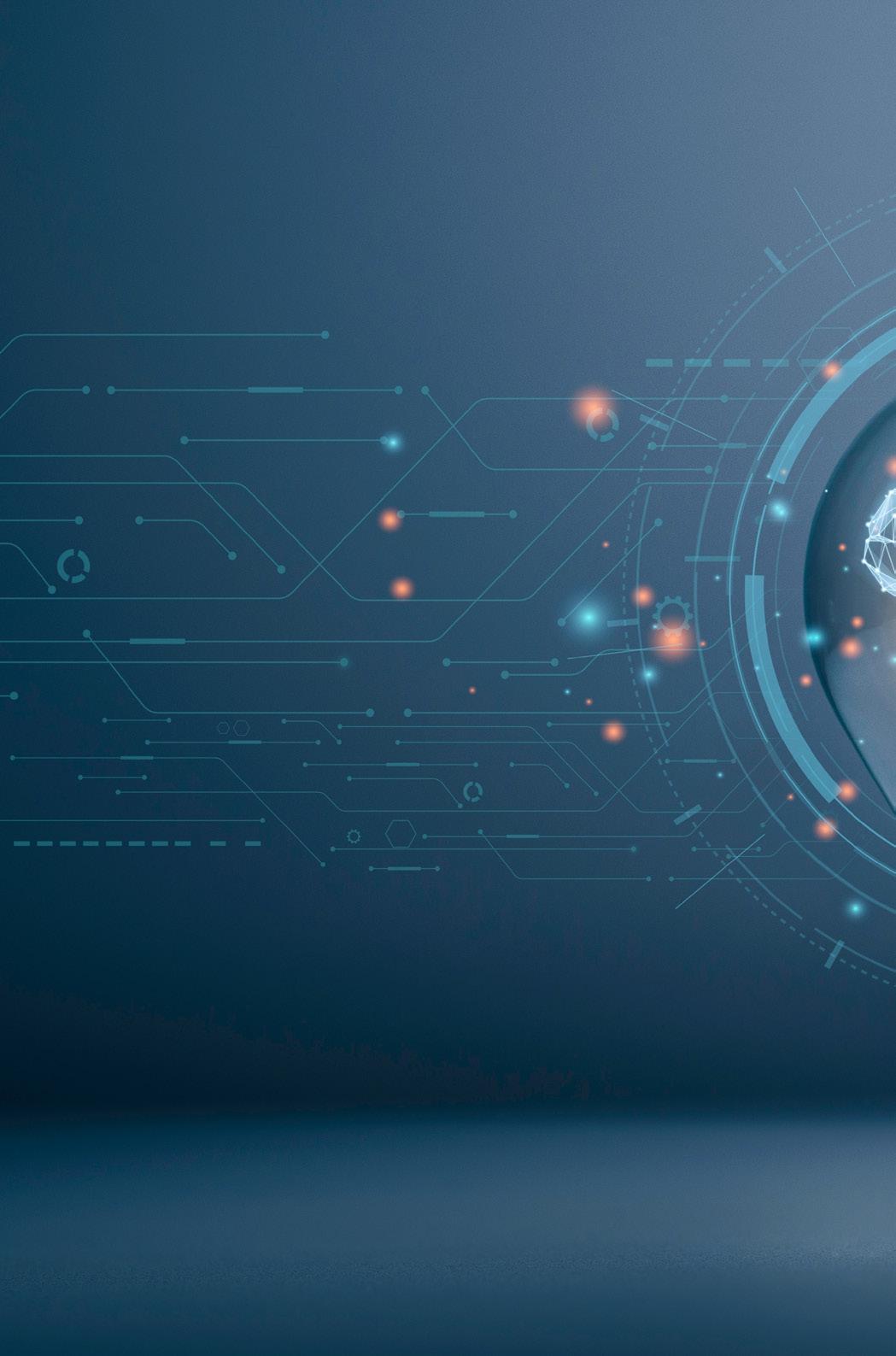
It’s evident that in the Middle East, government initiatives and action set the pace for technological advancement. Cloud-first strategies laid out by the UAE and Saudi Arabia in 2019 and 2020 respectively, have meant that today, this is now the preferred computing paradigm for many of these nations’ private enterprises. And now, the region’s forward-focused leaders have set their sights on AI. The UAE was of course the first country in the world to appoint a Minister of State for Artificial Intelligence, and that was as far back as October 2017. And this year, Saudi Arabia signaled its intentions of setting up a US$40billion AI investment fund.
The ongoing integration of AI into public services is reshaping the way governments interact with their citizens, offering unprecedented efficiencies and capabilities. But it is important to recognize that this technological leap brings with it the critical need to maintain, and even enhance, public trust in the government’s use of these capabilities. The responsible deployment of AI, combined with an unwavering commitment to transparency and security, is essential in fostering this trust.
AI’s integration into public sector functions has been both expansive and impactful. From automating routine
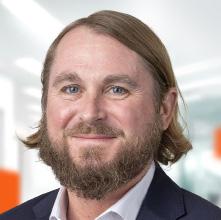
TRAVIS GALLOWAY
Head of Government Affairs at SolarWinds, writes about how governments can set the standard for AI transparency.
tasks to providing sophisticated analytics for decision-making, AI applications are becoming indispensable in areas such as law enforcement or social services. In law enforcement, predictive policing tools can help Middle East nations maintain their pristine records in maintaining social order, while on government portals, AI-driven chatbots such as the UAE’s ‘U-Ask’ can allow users to access information about government services in one place. These applications not only improve efficiencies but also enhance accuracy and responsiveness in public services.
While AI-driven applications are broadly advantageous to the public sector, AI, by its nature, raises concerns around trust: its complex algorithms can be opaque, its decision-making process impenetrable. When AI systems fail—whether through error, bias, or misuse—the repercussions for public trust can be significant. Conversely, when implemented responsibly, AI has the potential to greatly enhance trust through demonstrated efficacy and reliability. Therefore, a key principle that government entities must build their AI strategies upon is Transparency and Trust.
A ROBUST OBSERVABILITY
PROGRAM IS KEY TO BUILDING TRUST IN THE PUBLIC SECTOR’S
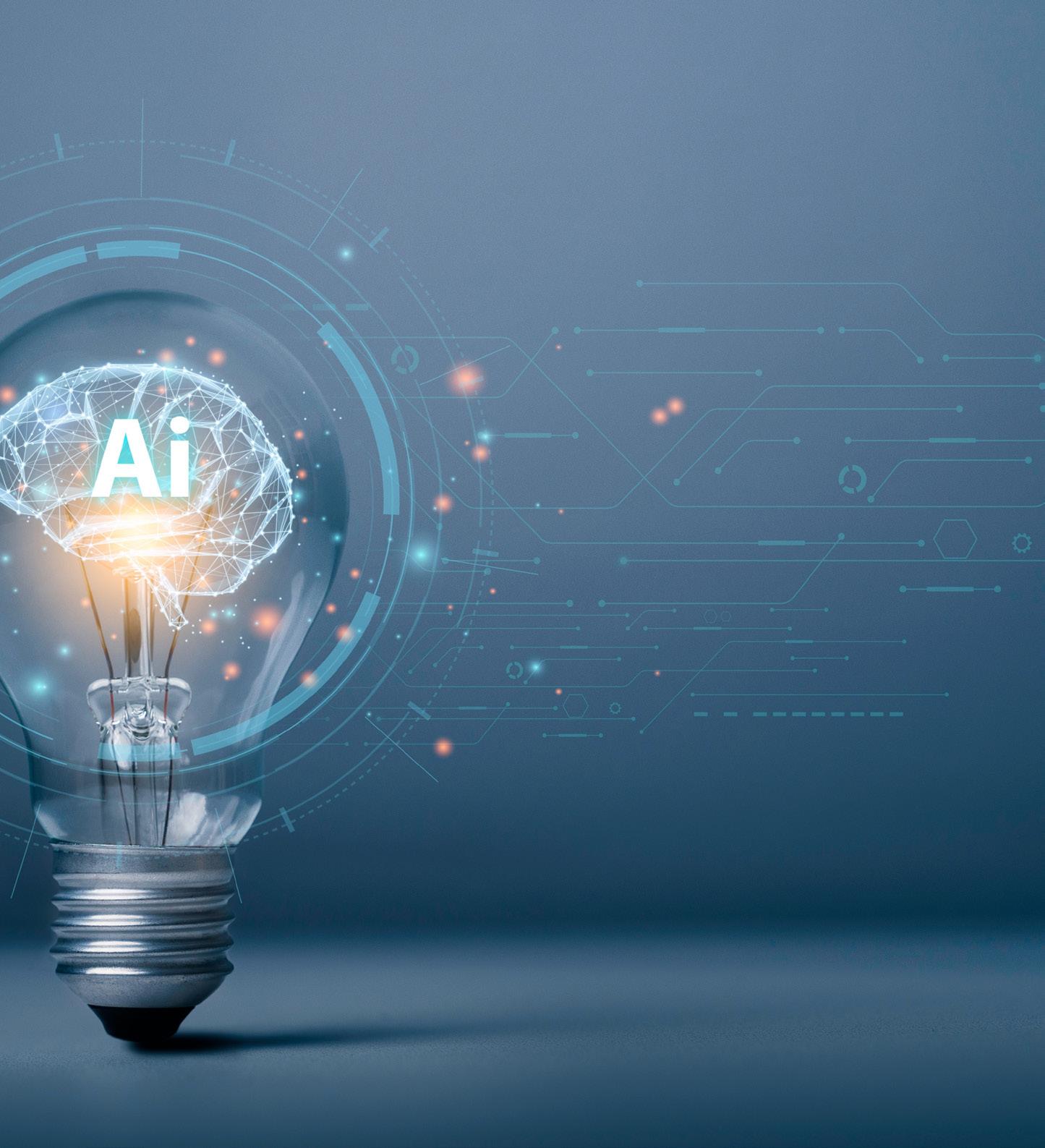
USE OF AI
A foundational way government entities can maintain accountability in their AI initiatives is by adhering to a robust observability strategy. Observability provides indepth visibility into an IT system, which is an essential resource for overseeing extensive tools and intricate public sector workloads, both on-prem and in the cloud. This capability is vital for ensuring that AI operations function correctly and ethically. By implementing comprehensive observability tools, government agencies can track AI’s decision-making processes, diagnose problems in real time, and ensure that operations remain accountable. This level of oversight is essential not only for internal management but also for demonstrating to the public that AI systems are under constant and careful scrutiny.
Observability also aids in compliance with regulatory standards by providing detailed data points for auditing and reporting purposes. This piece of the puzzle is essential for government entities that must adhere to strict governance and accountability standards. Overall, observability not only enhances the operational aspects of AI systems but also plays a pivotal role in building public trust by ensuring these systems are transparent, secure, and aligned with user needs and
regulatory requirements.
Equally critical in reinforcing public trust are robust security measures. Protecting data privacy and integrity in AI systems is paramount, as it prevents misuse and unauthorized access, but it also creates an environment where the public feels confident about depending on these systems. Essential security practices for AI systems in government entities include robust data encryption, stringent access controls, and comprehensive vulnerability assessments. These protocols ensure that sensitive information is safeguarded and that the systems themselves are secure against both external attacks and internal leaks.
The responsible deployment of AI, combined with an unwavering commitment to transparency and security, is essential in fostering this trust.
Even with these efforts, there will continuously be challenges in making sure AI builds, rather than erodes, public trust. The sheer complexity of the technology can make it hard for people to understand how AI works, which can lead to mistrust. Within government departments, resistance to change can also slow down the adoption of important transparency and security measures. Addressing these challenges requires an ongoing commitment to policy development, stakeholder engagement, and public education.
To navigate these challenges effectively, it’s paramount that governments adhere to another key principle in their design of AI systems: Simplicity and Accessibility. All strategies around implementing AI need to be thoughtful and need to make sense to all stakeholders and users. There needs to be a gradual build-up of trust in the tools rather than a jarring change, which can immediately put users on the defensive. Open communication and educating both the public and public sector personnel about AI’s capabilities and limitations can demystify the technology and aid adoption.
PwC estimates that by 2030, AI will deliver US$320billion in value to the Middle East. With governments in the region focused on growing the contribution of the digital economy to overall GDP, AI will be a fundamental enabler of their ambitions. While AI has immense potential to enhance public services, its impact on the public is complex. Government entities once again have the chance to lead by example in the responsible use of AI. And as has been the precedent, we can then expect the private sector to follow suit.
PUSH FOR AI REGULATION
A striking 87% of IT professionals in Europe, the Middle East and Africa (EMEA) would welcome stronger government regulation of artificial intelligence (AI) — according to a new survey from SolarWinds.
The survey of nearly 700 IT professionals, 297 of whom were from the EMEA region, reveals that security tops the list of AI concerns, with over twothirds (67%) emphasising the need for government measures to address security. Privacy is another major worry, with 60% of the region’s IT professionals calling for stronger rules to safeguard sensitive information. Additionally, an equal share (60%) of respondents believe government intervention is crucial to curb the spread of misinformation through AI, while nearly half (47%) support regulations focused on ensuring transparency and ethical practices in AI development.
These findings come at a time when governments in the region have begun announcing landmark initiatives around the development of frameworks to facilitate the secure and ethical implementation of AI. Notable among these are the EU’s landmark AI Act, Dubai’s unveiling of its Universal Blueprint for Artificial Intelligence which prescribes the appointment of a Chief Artificial Intelligence Officer in every government entity in the Emirate, and Saudi Arabia signaling its plans to create a US$40 billion fund to invest in artificial intelligence.
The survey further reveals a troubling lack of trust in data quality — which is essential for successful AI implementation. Only a third (33%) of EMEA respondents consider themselves ‘very trusting’ of the data quality and training used in AI systems. Additionally, 41% of the region’s IT leaders who have encountered issues with AI
attribute these problems to algorithmic errors stemming from insufficient or biased data.
As a result, data quality is identified as the third most significant barrier to AI adoption, following security and cost challenges.
Concerns about database readiness are also widespread. Just a third (33%) of EMEA IT professionals are very confident in their company’s ability to meet the increasing data demands of AI. This lack of preparedness is compounded by the fact that 43% of respondents believe their companies are not moving quickly enough to implement AI, partly due to ongoing data quality challenges.
Commenting on these findings, Rob Johnson, VP and Global Head of Solutions Engineering at SolarWinds said, “It is understandable that IT leaders are approaching AI with caution. As technology rapidly evolves, it naturally presents challenges typical of any emerging innovation. Security and privacy remain at the forefront, with ongoing scrutiny by regulatory bodies. However, it is incumbent upon organisations to take proactive measures by enhancing data hygiene, enforcing robust AI ethics and assembling the right teams to lead these efforts.”
“This proactive stance not only helps with compliance with evolving regulations but also maximises the potential of AI. Highquality data is the cornerstone of accurate and reliable AI models, which in turn drive better decision-making and outcomes. Trustworthy data builds confidence in AI among IT professionals, accelerating the broader adoption and integration of AI technologies,” Johnson added.
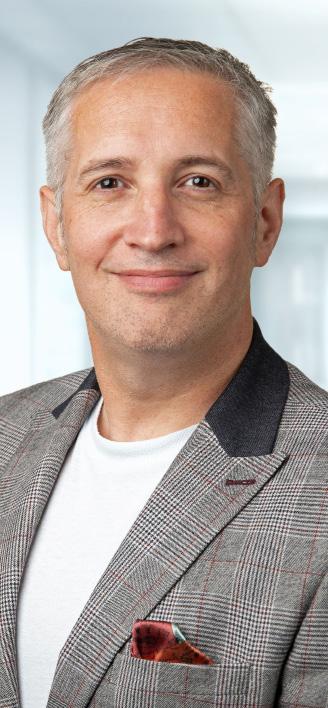
“Majority of EMEA IT professionals welcome greater AI regulation, reveals SolarWinds survey.”
ROB JOHNSON, VP AND GLOBAL HEAD OF SOLUTIONS ENGINEERING AT SOLARWINDS
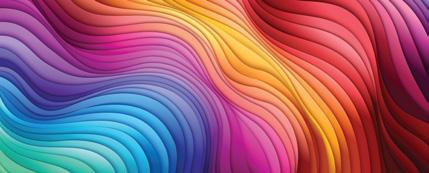
GUEST ARTICLE
Creating a positive impact
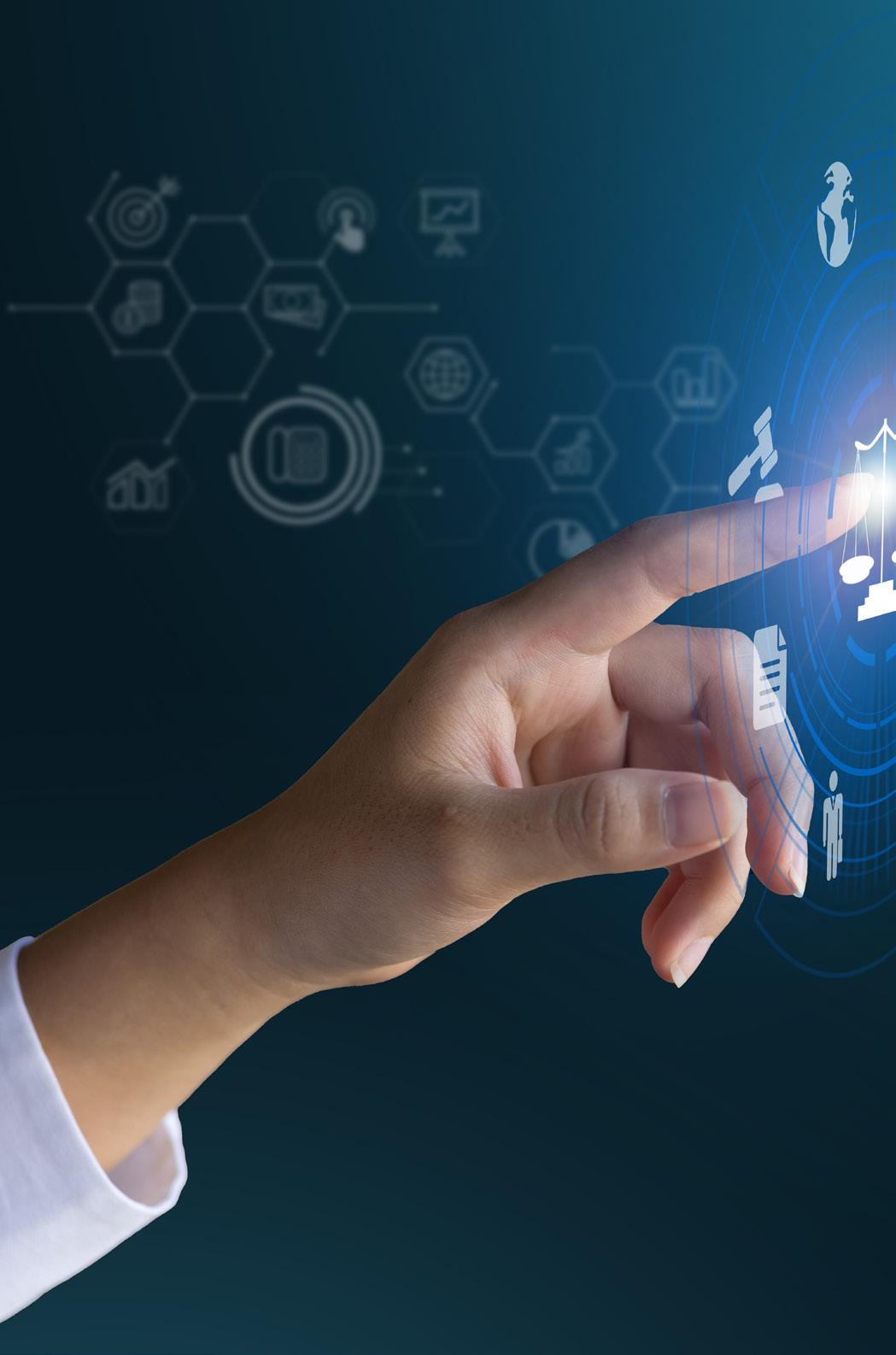
Today, AI is widely used for anything and everything; AI tools are being used to plan workouts, to code and create applications, to build self-driving cars, to transform patient care—the list goes on. While AI has been hugely beneficial in saving time, enhancing efficiency, and fostering innovation, a critical factor that is being overlooked is its impact on environmental sustainability and other environmental, social, and governance (ESG) factors.
AI’S ENERGY DEMAND
According to the Electric Power Research Institute, a non-profit research firm, AI queries require about 10 times the electricity of traditional Google queries. Think of the millions of users who type in queries every second—the energy demand adds up.
But why do AI models consume so much energy? It’s simply because of the vast amount of data and examples that are used for training an AI model. During training, AI models process large sets of data; this requires powerful hardware like GPUs, a type of electronic chip that consumes a lot of electricity. Another reason is AI’s computational power requirement, especially for large language models (LLMs), which are AI models that
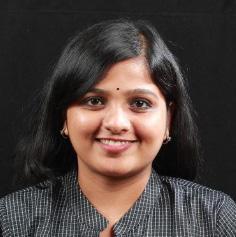
ATHIRA JAYAKUMAR
Enterprise Analyst, ManageEngine, on shaping a better tomorrow with sustainable AI.
are based on deep learning techniques designed to understand and process human language.
GPT-4 by OpenAI, BERT and Gemini by Google, and Llama by Meta are some popular examples of LLMs. Powerful LLMs might require thousands of GPUs, and manufacturing GPU chips, disposing of them, and the e-waste they generate can cause an increase in carbon emissions. A study by the University of Massachusetts Amherst found that training a single AI model can emit as much carbon as five cars over their lifetimes.
Another interesting point to note is the power consumed by the infrastructure, both hardware and software, is used to support AI computation. This includes the energy required to maintain, operate, and cool infrastructure components like data centres, servers, and network equipment. In fact, Google’s green house gas emissions in 2023 were almost 48% higher than in 2019, largely due to the energy demand tied to data centres.
ANALYSING AI’S IMPACT ON ESG FACTORS
While AI has negatively impacted the environment, it’s been said to benefit the social and governance factors under ESG—though even here there are concerns regarding gender and racial biases. In terms
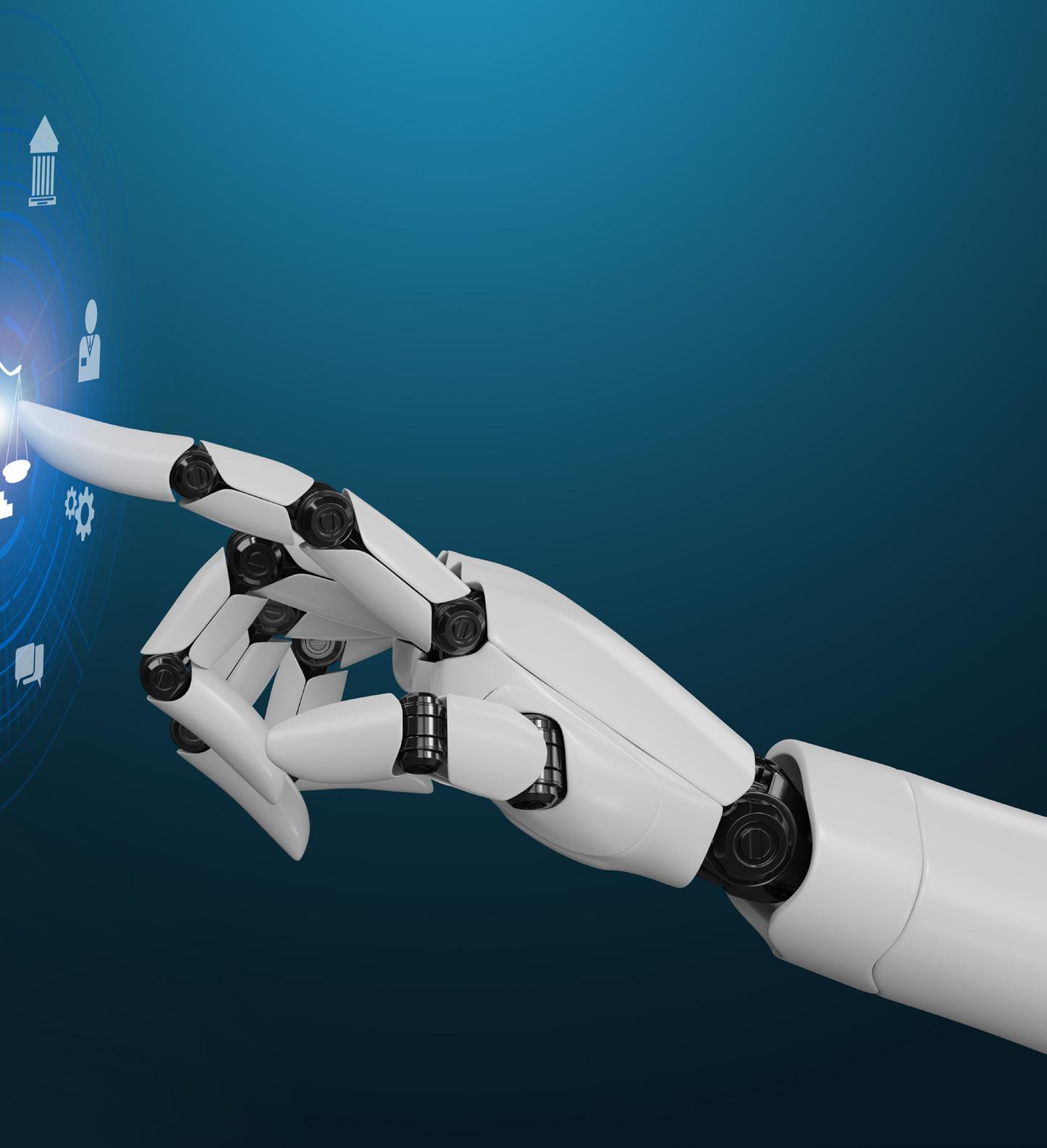
of societal factors, AI has been helpful in improving patient care, facilitating skill development, providing better access to financial services, and enhancing safety and security using threat detection systems.
In terms of governance, it has been a useful tool for analysing patterns and anomalies for fraud detection, automating administrative tasks, making datadriven decisions related to governance, and assisting in regulatory compliance management.
However, an important question that needs to be considered is, will the negative environmental impact outweigh the benefits tied to the societal and governance factors? Organisations need to carefully find a sweet spot between the environmental challenges raised and the opportunities provided by AI, especially today when consumers and investors are environmentally conscious and government and regulatory bodies are introducing stricter laws to ensure environmental sustainability.
THE NEED FOR SUSTAINABLE AI PRACTICES
Seventy-eight percent of CEOs surveyed as a part of Gartner’s 2023 CEO survey said the benefits of AI outweigh the risks, but the increasing number of organisations using AI, including generative AI (GenAI), is leading
to a AI having a growing environmental footprint. Organisations need to be wary of AI’s energy expense and take measures to mitigate its negative impact on the environment.
Organisations can still develop highperforming AI models that are sustainable by implementing a few environmentally conscious strategies. As discussed earlier, GenAI models in particular consume a lot of energy; this can be reduced to an extent if both vendors and users make minor mindset changes, beginning by using existing GenAI models or building upon them. Creating and training new models from scratch consumes a lot of energy; instead, organisations can use the training data and computing capabilities provided by existing LLM and image model providers.
Seventyeight percent of CEOs surveyed as a part of Gartner’s 2023 CEO survey said the benefits of AI outweigh the risks.
Another way to reduce energy consumption is to use energy-efficient AI models that operate effectively while maintaining the desired level of performance. AI models can be optimised by using computational techniques that consume less energy, such as pruning, quantisation, and knowledge distillation.
The pruned model uses less parameters to process model data and thus requires less memory, indirectly resulting in less power consumption. The quantisation technique lowers the precision of the parameters used in an AI model by converting model parameters—typically stored as 32-bit floating point numbers—to 8-bit integers; this means less memory is used, resulting in less energy consumption and faster operation. Finally, the knowledge distillation technique can be used to transfer the knowledge from a large, complex AI model to a smaller one. The smaller model replicates the outcome of the complex model rather than deriving the output from raw data.
The use of AI-specialised hardware is another avenue to explore: Chip manufacturers have started to explore innovative ways to design chips exclusively for training AI systems. Normally, general purpose hardware, such as GPUs, is used to train large models of data, and these consume a lot of energy. But with the increase in training demands, there’s been a push for developing hardware that is capable of handling specific AI tasks. Chips that can process AI models with increased efficiency and speed while significantly reducing the energy needed for AI computations can help ensure sustainable AI operations.
GUEST
ARTICLE
Navigating the digital shift

The GCC region makes for an exciting case study for artificial intelligence (AI). Not only was the United Arab Emirates (UAE) the first nation to establish an AI ministry, but every Gulf country now has a formal AI vision. Oman has the AI Economies Initiative, Kuwait has the National AI Strategy, Bahrain has the Ethical AI Framework, Qatar has the National AI Strategy, Saudi Arabia has the National Strategy for Data & AI, and the UAE has the National Strategy for Artificial Intelligence. The AI game is on and everyone in the region has an unwavering eye on the ball.
McKinsey research from 2023 predicted “real value” from artificial intelligence, which may add as much as US$150 billion (or 9% of combined GDP) to GCC economies. It is worth mentioning that, in May last year when the McKinsey report was published, generative AI (GenAI) was relatively new in the nontech consciousness. And yet, McKinsey’s researchers still recognised GenAI’s potential to force an upward revision of their impact projection.
One might call the rise of AI nothing less than a Digital Shift. Digital transformation itself must now necessarily include AI for
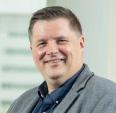
DAVID BOAST
Managing Director - MENA, Endava, writes about why GCC enterprises must modernise before AI can take flight
the business to remain competitive. But for organisations to get the most out of it they must plumb the depths of value on offer from AI and wring every ounce of value from it. They cannot do this by slapping AI on top of legacy infrastructure like hot pitch on a leaky boat. Harnessing AI to its fullest has a prerequisite — the revamp of the IT suite from top to bottom. This enterprise modernisation cannot be undertaken alone. Organisations, their vendors, and all the trusted partners in between must work shoulder-to-shoulder to build the future. The road to that future is a de-risked, cost-controlled, accurate, endto-end system transformation, supported by automated, data-driven decision-making and execution.
ROCKETS AND MOONSHOTS
Let’s return to the leaky boat. Patched with AI, legacy infrastructure may be able to remain afloat, but will never be able to take flight. That is because, at its core, the boat is still a boat. To turn it into a rocketship requires a rethink and a fundamental rebuild, from the bottom up. The rocket will be faster, and more manoeuvrable — able to keep pace with the sprinting technology landscape — but only if it is built with longterm business strategy in mind. The GCC is
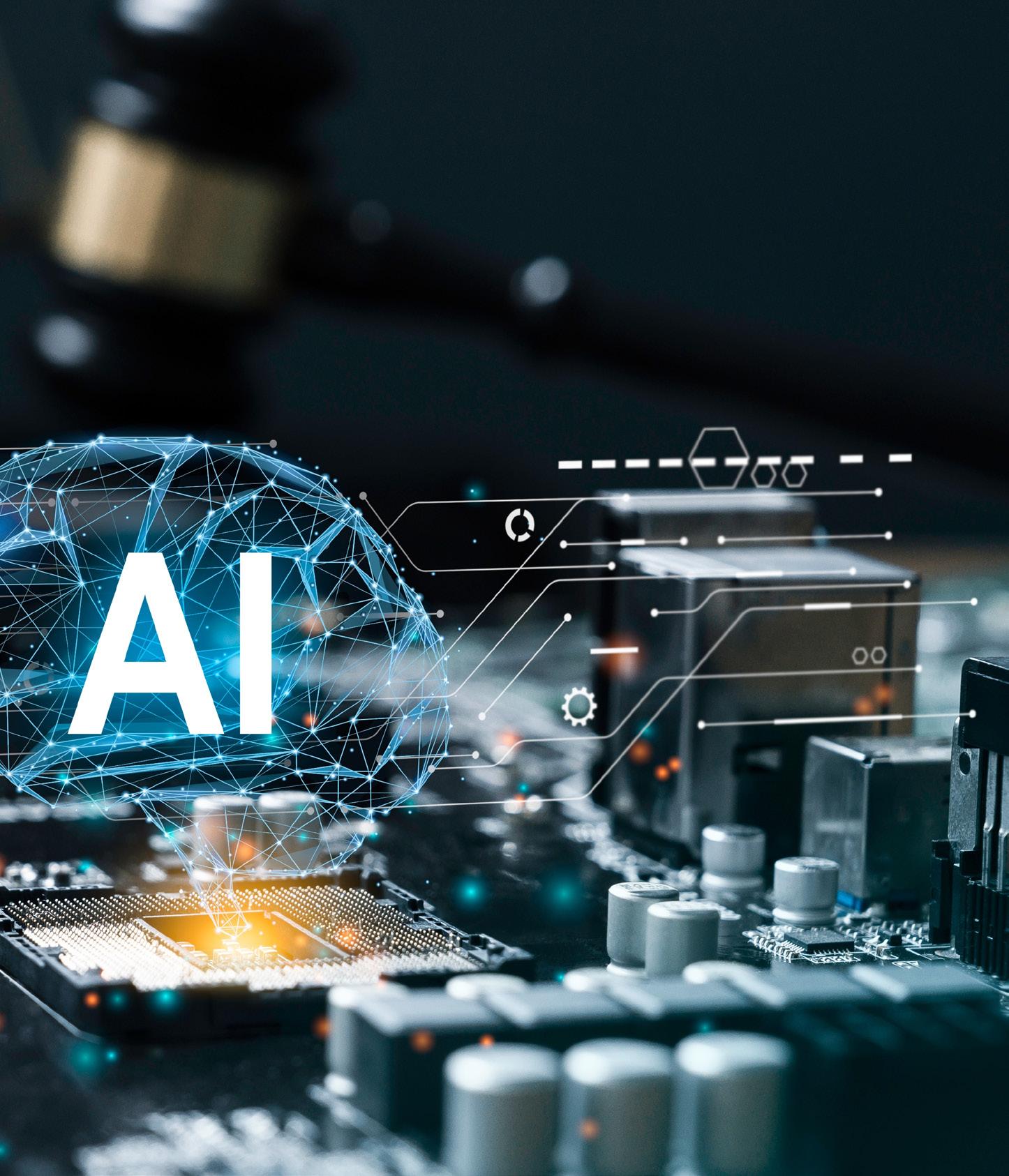
home to many dynamic markets and is part of a global digital economy that is prone to shocks, fluctuating customer demands, and emerging technologies. All this means that you must first step back from the rocket you want, and look at the boat you have.
Often, legacy systems do not capture data in straightforward, homogenised ways. As enterprise modernisation progresses, the organisation will remedy this and introduce new system architectures that make way for rapid deployment of digital experiences. Workflows and business logic will be exposed to new analyses that allow them to be quantifiably critiqued and optimised. You will note, AI has not been mentioned yet because we are still preparing its nest, feathered with up-to-date core systems, rapid-deployment capabilities, and clean data.
Great change is guaranteed to scare. Many see structural overhauls as moonshots with no guaranteed landing. Risk immobilises decision-makers. They ask, “What of the impact on our commercial standing and brand reputation if we fail?” They declare: “The risk of interrupted service is unacceptable.” These are understandable concerns and yet competitiveness will suffer if the enterprise does not modernise before
introducing AI. Agile newcomers have the opportunity to be modern because their first systems can be what they need them to be. Incumbents can be left behind if they do not face up to this.
TAKING FLIGHT
The risk of interrupted service is unaccep table.”These are under standable concerns and yet compe titiveness will suffer if the enterprise does not modernise before introducing AI.
Fortunately, change need not be the dark spectre it is perceived to be. Today, organisations have access to data-driven approaches guided by the expertise of partners that know enterprise modernisation starts with identifying the main building blocks for success. We have already mentioned these steps — clean data, composable architectures, and business intelligence about workflows and business logic. Once transparency and flexibility are in place, the enterprise can derisk the transformation process because of improved system clarity and efficiency. Roadmaps can help by laying out phased projects — cloud migration first, then a rejigging of application architecture, and so on. And finally, AI. It may seem like a convoluted route, but the AI-less steps on the journey to AI implementation are critical. The rocket has now been built. And AI? AI is the fuel. Many companies desperately want to join the AI race. But trying to catch rockets in boats is impractical. Returning to the basics of one’s architecture and being critical about what you see is what will make the difference in the race. Do you make that moonshot? Do you land in your preferred spot? Many do not. Even organisations that gain some air from AI cannot maintain altitude because of the absence of fundamentals like clean data and agile infrastructure.
And yet, many will continue to introduce AI into an inhospitable tech stack. Perhaps they were put off by enterprise modernisation because it was not the end goal they had in mind. It can be daunting to be told you must complete an initial journey before you can embark upon the one that interests you. Especially when you perceive the first journey to be both less interesting, and fraught with danger. But as we have seen, enterprise modernisation can, with proper planning, be risk-light. When it is complete, the next phase of digital transformation can commence, and you can start to thrive amid the Digital Shift. With clean data access and robust system interconnectivity, AI can finally do what you imagined it could.
GUEST ARTICLE
The AI Data Cycle
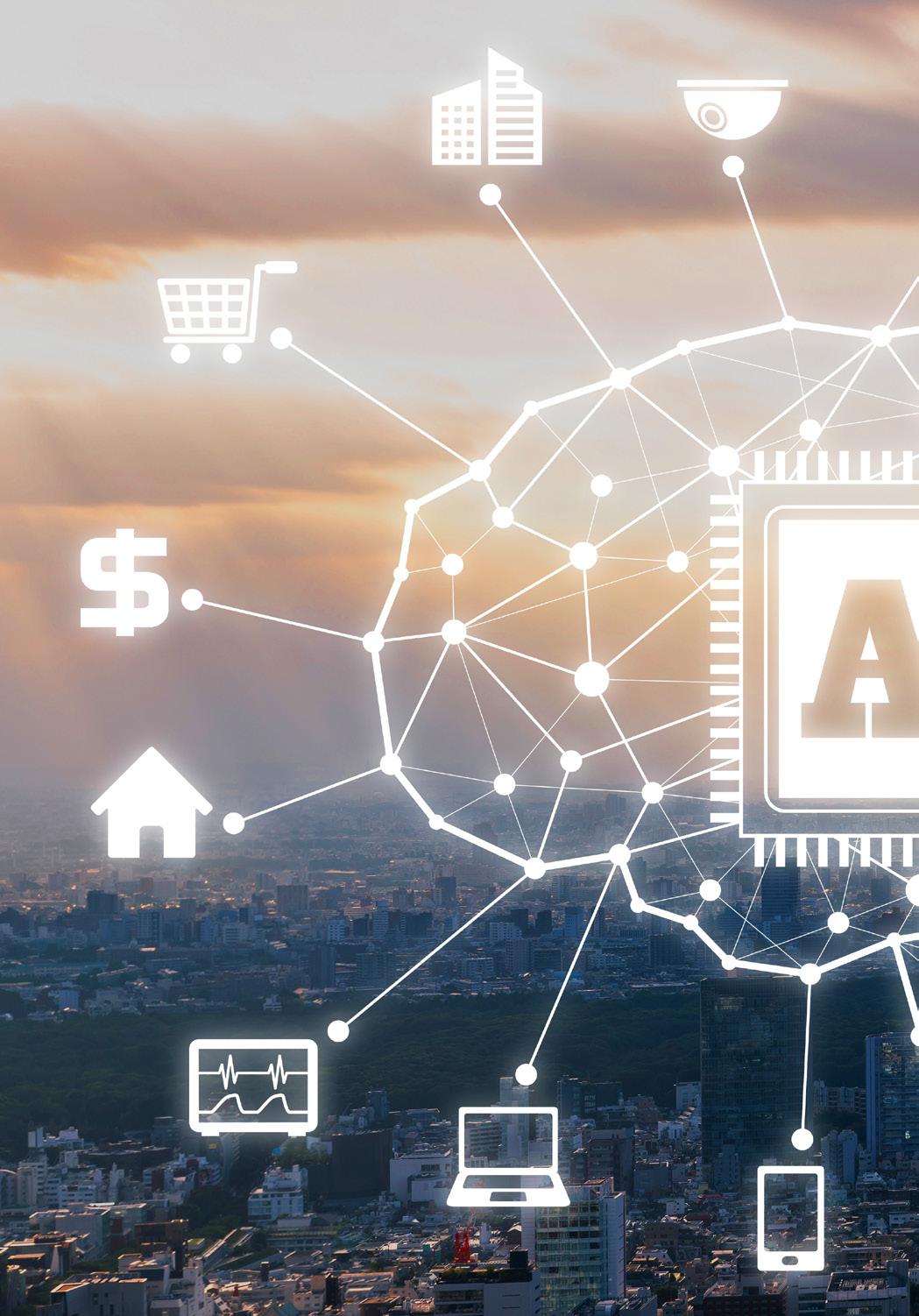
While AI is transforming lives and inspiring a world of new applications, at its core, it’s fundamentally about data utilization and data generation.
As the AI industry builds-out a massive new infrastructure to train AI models and offer AI services (inference), there are important implications related to data storage. First, storage technology plays important roles in the cost and powerefficiency of the varied stages of this new infrastructure. As AI systems process and analyze existing data, they create new data, much of which will be stored because it’s useful or entertaining. And new AI use cases and ever more sophisticated models make existing repositories and additional data sources more valuable for model context and training, powering a cycle where increased data generation fuels expanded data storage, which fuels further data generation – a virtuous AI Data Cycle. It’s important for enterprise data center planners to understand the dynamic interplay between AI and data storage. The AI Data Cycle outlines storage priorities for AI workloads at scale at each one of the six-stages. Storage component manufacturers, such as Western Digital, are tuning their product roadmaps in recognition of these accelerating AI-driven requirements to maximize performance and minimize TCO.
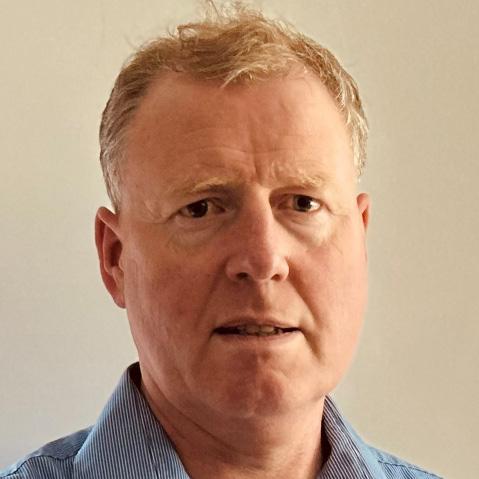
PETER HAYLES
Product Marketing Manager HDD at Western Digital, on understanding the optimal storage mix for AI workloads at scale
Let’s take a quick walk through the stages of the AI Data Cycle:
1) Raw Data Archives, Content Storage: Raw data is collected and stored from various sources securely and efficiently. The quality and diversity of collected data are critical, setting the foundation for everything that follows. Storage needs: Capacity enterprise hard disk drives (eHDDs) remain the technology of choice for lowest cost bulk data storage, continuing to deliver highest capacity per drive and lowest cost per bit.
2) Data Preparation & Ingestion: Data is processed, cleaned, and transformed for input to model training. Data center owners are implementing upgraded storage infrastructure such as fast data lakes to support preparation and ingestion.
Storage needs: All-flash storage systems incorporating high-capacity enterprise solid state drives (eSSDs) are being deployed to augment existing HDD based repositories, or within new all-flash storage tiers.
3) AI Model Training: It is during this stage where AI models are trained iteratively to make accurate predictions based on the training data. Specifically, models are trained on high-performance supercomputers,
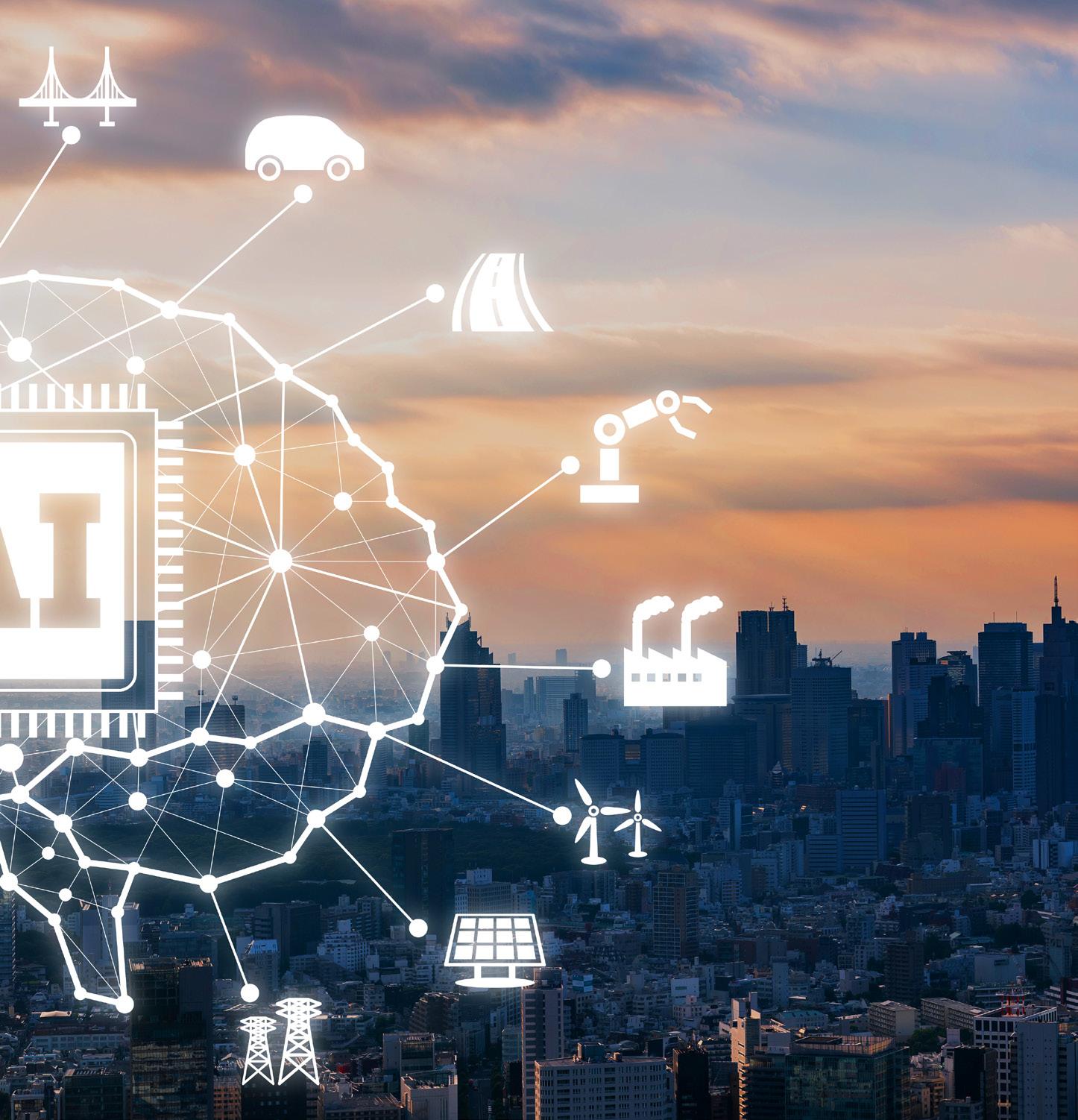
and training efficiency relies heavily on maximizing GPU utilization.
Storage needs: Very high-bandwidth flash storage near the training server is important for maximum utilization.
High-performance (PCIe® Gen. 5) and low-latency compute optimized eSSDs are designed to meet these stringent requirements.
4) Inference & Prompting: This stage involves creating user-friendly interfaces for AI models, including APIs, dashboards, and tools that combine context specific data with end-user prompts. AI models will be integrated into existing internet and client applications, enhancing them without replacing current systems. This means maintaining current systems alongside new AI compute, driving further storage needs.
Storage needs: Current storage systems will be upgraded for additional data center eHDD and eSSD capacity to accommodate AIintegration into existing processes. Similarly, larger and higher performance client SSDs (cSSDs) for PCs and laptops, and higher capacity embedded flash devices for Mobile Phones, IoT systems, and Automotive will be needed for AI-enhancements to existing applications.
5) AI Inference Engine: Stage 5 is where the magic happens in real-time. This stage involves deploying the trained models into production environments where they can analyze new data and provide real-time predictions or generate new content. The efficiency of the inference engine is crucial for timely and accurate AI responses.
Storage needs: High-capacity eSSDs for streaming context or model data to inference servers; depending on scale or response time targets, highperformance compute eSSDs may be deployed for caching; High-capacity cSSDs and larger embedded Flash modules in AI-enabled edge devices.
As AI systems process and analyze existing data, they create new data, much of which will be stored because it’s useful or entertaining.
6) New Content Generation: The final stage is where new content is created. The insights produced by the AI models often generate new data, which is stored because it proves valuable or engaging. While this stage closes the loop, it also feeds back into the data cycle, driving continuous improvement and innovation by increasing the value of data for training or analysis by future models.
Storage needs: Generated content will land back in capacity enterprise eHDDs for archival data center storage, and in high-capacity cSSDs and embedded Flash devices in AIenabled edge devices.
A SELF-PERPETUATING CYCLE OF INCREASED DATA GENERATION
This continuous loop of data generation and consumption is accelerating the need for performance-driven and scalable storage technologies for managing large AI data sets and re-factoring complex data efficiently, driving further innovation.
Ed Burns, research director at IDC noted, “The implications for storage are expected to be significant as the role of storage, and access to data, influences the speed, efficiency and accuracy of AI Models, especially as larger and higher-quality data sets become more prevalent.”
There’s no doubt that AI is the next transformational technology. As AI technologies become embedded across virtually every industry sector, expect to see storage component providers increasingly tailor products to the needs of each stage in the cycle.
Unlocking new possibilities
From voice-activated assistants such as Siri to self-driving cars, artificial intelligence (AI) is revolutionizing the way we live and work. But have you ever stopped to think about its impact on mobile connectivity, especially as 5G subscriptions will reach close to 5.6 billion in 2029? What if your smartphone could predict your data usage and optimize your network connection in real time?
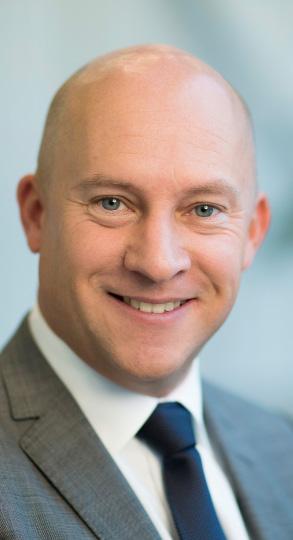
PATRICK JOHANSSON
President of Ericsson Middle East and Africa, on how AI is driving radical innovation, unprecedented growth, and sustainable development
The evolution of AI is truly mind-blowing, with incredible possibilities on the horizon. As one of the greatest transformative forces of our time, AI is revolutionizing all sectors including telecommunications. Its unprecedented efficiency and automation capabilities are reshaping the telecommunications industry, driving agility, and enabling predictive, proactive operations. Today, many service providers are leveraging AI to automate tasks and make data-driven decisions.
AI is key to developing the networks of tomorrow. It enhances communication technologies, supports extended reality (XR), reduced capability (RedCap) devices, and boosts network energy efficiency. The rise of large language models (LLMs) has also expanded the potential for generative AI use cases, bringing with it benefits that go far beyond traditional applications.
AI has been critical in the evolution of mobile connectivity, advancing the shift from best-effort mobile broadband to more predictable, performance-oriented services. This shift promises significant economic benefits, empowering businesses, governments, and societies to meet sustainability goals.
While these advancements may be remarkable, we are just beginning to unlock AI’s true power. Its impact will become even more pronounced as 5G networks become ubiquitous. That is when AI will pave the way for innovations we never imagined.
As leaders in 5G technology, we are excited about AI’s potential and have been at the forefront of its adoption. For decades, we have leveraged AI-powered solutions to manage complex network data, predict patterns, and optimize network performance. Our AI-driven predictive network management technology provides comprehensive machine learning-based diagnostics, root cause analysis, and actionable recommendations to enhance user experiences. This not only helps communication service providers (CSPs) address network anomalies but also redefines how networks are operated and maintained.
In the Middle East and Africa (MEA) region, AI plays a crucial role in ensuring reliable connectivity and advancing digital inclusion, particularly in line with ambitious national visions. We are deploying AI-enabled solutions to improve network performance, contribute to digital transformation, and tackle challenges unique to these markets. By adopting AI, we are not only solving current issues but also laying the foundation for the networks of the future.
Key to highlight as well that with AI’s vast benefits, there is a pressing need to look into cybersecurity. As 5G and ICT transition to cloud and edge computing, new vulnerabilities are emerging. The rise of generative AI and LLMs has equipped adversaries with the tools to rapidly exploit these weaknesses.
Trustworthiness also remains a challenge. AI’s reliance on probabilistic models can create uncertainty, and transparency around AI processes is crucial. To realize the full potential of AI, trust needs to be established in the development, deployment and use of AI. This is why it’s critical for us to build human trust in AI, addressing aspects spanning from explainability and human oversight to security and built-in safety mechanisms. Trustworthiness is a prerequisite for AI, and we are building it into the system by design.
Unlocking AI’s full potential will require partnerships across industries to develop secure and trustworthy solutions. As we move toward an era where networks can sense, compute, learn, and act autonomously, AI will be central to managing the explosion of data generated by billions of connected devices. This hyperconnected future will depend on strong partnerships between ICT companies and CSPs, transforming business and society through secure, efficient, and sustainable communication services.
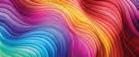
UAE - 18 FEB KSA - 24 FEB
SINGAPORE - 24 OCT
(MUMBAI) - 12 NOV
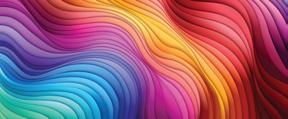
- 27 OCT
(BENGALURU) - 14 NOV
- 29 OCT